Abstract
Background:
This study aimed at creating a new predictive model of significant fibrosis in chronic hepatitis B using direct and indirect parameters and comparing this model with other noninvasive models for its validation in clinical settings.Methods:
Patients (n = 81), according to the ISHAK score, were classified as mild and significant fibrosis. Serum matrix metalloproteinase-2, tissue inhibitor of metalloproteinase-2, beta-nerve growth factor levels, and indirect parameters were analyzed. To evaluate the presence of significant hepatic fibrosis, well-known conventional models were also evaluated. The cut-off values of each model were determined using receiver operating characteristic curves to distinguish patients with mild and significant fibrosis.Results:
Significant hepatic fibrosis index-1 was constructed using the following equation: (matrix metalloproteinase-2 × age × prothrombin time × direct bilirubin) / (albumin × platelet). The sensitivity and specificity for significant hepatic fibrosis index-1 were 73.3% and 95.6%, respectively. Area under the curve of significant hepatic fibrosis index-1 was 0.895 (P < 0.001), which was higher than the other models. Due to limitations of matrix metalloproteinase-2, significant hepatic fibrosis index-2 was constructed using a formula without matrix metalloproteinase-2. However, there were no significant differences between significant hepatic fibrosis index-1 and significant hepatic fibrosis index-2 or other models, except for 3 models.Conclusions:
Significant hepatic fibrosis index-1 employs a new marker; matrix metalloproteinase-2 along with routine parameters had the best diagnostic performance for significant fibrosis in patients with chronic hepatitis B. Using significant hepatic fibrosis index-1 or even significant hepatic fibrosis index-2 might be an alternative approach in place of liver biopsy to predict significant fibrosis in chronic hepatitis B cohort.Keywords
1. Background
An estimated 257 million people are living with Hepatitis B Virus (HBV) infection worldwide. Infection during adulthood leads to chronic hepatitis in less than 5% of cases (1). Liver fibrosis as part of the natural wound healing response when chronic HBV infection has caused parenchymal injury, and may further result in cirrhosis. The severity of liver fibrosis correlates with patient prognosis and determines the treatment strategy required. Liver biopsy is the gold standard in order to determine an early stage of fibrosis and hence manage the treatment required. The detection of significant fibrosis (according to Ishak score of ≥ 3) in the liver biopsy indicates whether patients should receive antiviral treatment (2).
However, liver biopsy has disadvantages due to many reasons, such as reluctance of patients, sampling failure, need of recurrent biopsies, variations between pathologists, and increased morbidity and mortality (3, 4). Furthermore, the need of rest on the hospital bed after the procedure, the usage of ultrasonography, and high risk of complications may reduce its cost effectiveness and also might be a burden of hospital services (4).
The World Health Organization (WHO) recommends using noninvasive diagnostic biomarkers, which are simple, readily, available, reliable, inexpensive, and safe to assess the stage of liver disease and eligibility for treatment [1]. Noninvasive diagnostic tests for liver fibrosis are classified as direct and indirect tests. Direct tests, such as procollagen type I and type III, type IV collagen, hyaluronic acid, laminin, Metalloproteinase (MMP), and Tissue Inhibitors of Matrix Metalloproteinase (TIMP), and transforming growth factor-β or α, directly correlate with the liver matrix produced by the hepatic stellate cells during extracellular matrix turnover in the fibrosis process. The indirect tests, such as serum alanine aminotransferase (ALT) and biochemical models, reflect alterations in liver function and they are molecules released into the bloodstream due to liver inflammation, but do not exhibit extracellular matrix turnover (5-10).
Hepatic stellate cells, activated by persistent liver damage, gain a fibroblastic phenotype and contribute to the formation of fibrous tissue (11, 12). The balance between the MMP and TIMP family is regulated by hepatic stellate cells and is important for fibrinolytic activity. The MMP-2 and its specific inhibitor (TIMP-2) have been expressed by activated hepatic stellate cells (13). As a result of this, it has been proposed that serum TIMP-2 and MMP-2 might be valuable noninvasive diagnostic tests for hepatitis B-induced liver fibrosis (14, 15). It has been shown that beta-Nerve Growth Factor (β-NGF) and its receptor were expressed in activated hepatic stellate cells (16-18).
Some of the indirect predictive models for hepatic fibrosis have been tested in patients with HBV and Hepatitis C Virus (HCV) infection, including Aspartate Transaminase (AST) to Alanine Transaminase (ALT) ratio (AAR) (19), age-Platelet (PLT) index (API) (20), AST to PLT ratio index (APRI) (21), Gamma Glutamyl Transferase (GGT) to PLT ratio index (GPRI) (22), S index (23), King’s score (24), FIB-4 model (25), Fibro-Q model (26), Forn’s index (27), Red cell Distribution Width (RDW) to PLT ratio (RPR) (28), and Fibrosis-Cirrhosis Index (FCI) (29). There are also direct models defined in these patients and the Enhanced Liver Fibrosis (ELF) test is one of them (30). Serum biomarkers of fibrosis are well validated in patients with chronic viral hepatitis with more evidence for HCV than for HBV (2).
2. Objectives
This study aimed at establishing a new noninvasive model with more accurate and reliable performance that could predict significant fibrosis in Chronic Hepatitis B (CHB) cohort. To create the model, this study evaluated serum MMP-2, TIMP-2, and β-NGF together with biochemical and hematological parameters. Furthermore, this study compared new predictive models with other noninvasive models for its validation in clinical settings.
3. Methods
3.1. Patients
Patients between 18 and 65 years of age admitted to the University of Health Sciences, Tepecik Training and Research Hospital Infectious Diseases Clinic between January and May 2017 were examined in terms of suitability for the research. Patients with CHB and in need of liver biopsy, who had not received any treatment were selected. Liver biopsy was performed for the patients, who had HBV DNA ≥ 2000 IU/mL for more than 6 months and anti HBe positivity. Chronic Hepatitis B was defined as persistence of hepatitis B surface antigen (HBsAg) positivity for more than 6 months. As a result, 81 patients were eligible for the study.
The individuals with the following disorders or conditions were excluded from the study, 1) coinfection liver disease (Hepatitis C Virus (HCV), hepatitis D virus, and human immunodeficiency virus infection), 2) non-hepatic fibrotic disease (autoimmune, alcohol or drug related, inherited or metabolic liver disease, and liver transplantation); 3) bleeding disorder; 4) chronic renal failure (stage 5 or over); 5) psychotic illnesses; 6) pregnancy; 7) consuming of alcohol more than 350 g per week for females and 420 g per week for males in the last 6 months; and 8) treatment with any anticoagulants or antiviral drugs. The patients did not receive any antiviral therapy before or during the liver biopsy. The patients, who had HBV DNA of ≥ 2000 IU/mL, according to the laboratory test results and ISHAK score of ≥ 3, according to the liver biopsy, received antiviral treatment afterwards.
Detailed information was given to all participants about the study before their participation, and their signed consents were obtained. This comparative analytical study was conducted in accordance with the Helsinki Declaration and with the approval of the local ethics committee (Tepecik Training and Research Hospital’s Ethics Committee, Decision Date-Number: 18/08/2016 - 12).
3.2. Study Design
Blood sampling and liver biopsy procedure were applied simultaneously. To obtain serum samples, blood collection tubes with gel separator were centrifuged at 1500 g for 10 minutes. At least 2 portions of serum samples were aliquoted to 1.5-mL sterile eppendorf tubes. Routine biochemical parameters in the first aliquot were analyzed immediately; the other one was stored at -20 °C until further analysis.
In the first aliquot, total protein (g/L), serum albumin (g/L), ALT (IU/L), AST (IU/L), Alkaline Phosphatase (ALP) (IU/L), GGT (IU/L), total bilirubin (mg/dL), direct bilirubin (mg/dL), triglyceride (mg/dL), cholesterol (mg/dL), and C-reactive protein (mg/L) were analyzed with AU5800 auto-analyzer (Beckman Coulter Inc., CA, USA). Alpha-Fetoprotein (AFP) (ng/mL) was analyzed with the DxI 800 auto-analyzer (Beckman Coulter Inc., CA, USA). Prothrombin Time (PT) (s) and activated Partial Thromboplastin Time (aPTT) (s) were determined by Sysmex® CS-2500 System (Sysmex Corporation, Kobe, Japan) automated coagulation analyzer. International Normalized Ratio (INR) was calculated using INR = (patient PT/mean normal PT)ISI formula. International Sensitivity Index (ISI) value of Thromborel S (Siemens Healthcare Diagnostic Products, Marburg, Germany) reagent was 0.98. The PLT (109/L), hematocrit (%), and RDW (%) were determined by Beckman Coulter LH 780 (Beckman Coulter Ireland Inc., Mervue, Galway, Ireland), a complete blood count analyzer.
In frozen and thawed samples, serum MMP-2 (ng/mL) (E-EL-H1445, Elabscience Biotechnology, Wuhan, China), TIMP-2 (pg/mL) (ELH-TIMP2, RayBiotech, Inc., Norcross, GA, USA), and β-NGF (pg/mL) (ELH-BNGF, RayBiotech, Inc., Norcross, GA, USA), levels were analyzed with the Enzyme-Linked Immunosorbent Assay (ELISA) method, according to the manufacturer’s introductions.
The ELF test, which includes the parameters of Hyaluronic Acid (HA) (ng/mL), type III Procollagen Amino-Terminal Propeptide (PIIINP) (ng/mL), and TIMP-1 (ng/mL) were also determined and used as reliable noninvasive indicators of significant hepatic fibrosis. The ELF parameters were determined using an Advia Centaur XP analyzer (Siemens Diagnostics, Erlangen, Germany) and scores were calculated by using the following formula: 2.278 + 0.851 ln(HA) + 0.751 ln(PIIINP) + 0.394 ln(TIMP-1). Expected values, according to the ISHAK score, recommended by the manufacturer was as follows: if ELF score was below 7.7, there was none or mild fibrosis; ELF over 7.7 defined moderate or severe fibrosis. With this cut-off value, AUC was 0.786 (0.755 to 0.817) (sensitivity of 88.6% and specificity of 34.6%).
To evaluate hepatic fibrosis, along with the ELF score, other well-known indexes, such as AAR, API, APRI, GPRI, S index, King’s score, FIB-4 model, Fibro-Q model, Forn’s index, RPR, and FCI were calculated, according to formulas, as shown in Table 1.
Formulas of Hepatic Fibrosis Scores
Fibrosis Model | Formula |
---|---|
King score | Age (years) × AST × INR / PLT |
FIB-4 | [Age (years) × AST] / [PLT × √ALT] |
ELF | 2.278 + 0.851 ln (HA) + 0.751 ln (PIIINP) + 0.394 ln (TIMP-1) |
API | Age (years): ≤ 30 = 0, 31 - 40 = 1, 41 - 50 = 2, 51 - 60 = 3, 61 - 70 = 4, >70 = 5; PLT: ≥ 225 = 0, 200 - 224 = 1, 175 - 199 = 2, 150 - 174 = 3, 125-149 = 4, <125 = 5; API is the sum of age and PLT (possible value 0 - 10) |
Fibro-Q | [10 × age (years) × AST × INR] / [PLT × ALT] |
Forn’s index | 7.811 - [3.131 × ln (PLT)] + [0.781 × ln (GGT)] + [3.467 × ln (age)] - [0.014 × cholesterol] |
APRI | [AST/ upper limit of normal for AST] / PLT × 100 |
GPRI | GGT / PLT |
S index | (1000 × GGT) / (PLT × Alb2) |
RPR | RDW / PLT |
FCI | (ALP × bilirubin) / (Albumin × PLT) |
AAR | AST / ALT |
3.3. Histopathological Analysis
Liver biopsy was used as the gold standard to evaluate hepatic fibrosis. Liver biopsies were obtained with an 18G biopsy needle (18G × 20 cm, Geotek Medical and Health Services, Ankara, Turkey). Biopsy samples were fixed with 10% neutral buffered formalin for at least 2 hours. Paraffin embedded tissues were serially sectioned for hematoxylin and eosin, Masson trichrome and reticulin staining. The cores containing at least 8 portal tracts were scored according to the modified ISHAK system (31). Patients whose ISHAK fibrosis score was < 3 (equivalent to < 2 METAVIR score) (n = 48) and ≥ 3 (equivalent to ≥ 2 METAVIR score) (n = 33) were classified as mild and significant fibrosis, respectively. The sections were independently examined by a single pathologist to avoid inter-observer variability.
3.4. Statistical Analysis
The SPSS version 20.0 (SPSS Inc. Chicago, USA) was used for statistical analyses. The normality of the variables was tested with the Shapiro-Wilk test. Continuous variables were presented as mean with Standard Deviation (SD) or median with Interquartile Range (IQR). If the data were normally distributed, the statistical difference between the results of patients with mild and significant fibrosis were evaluated by the unpaired t test, and if not, results were evaluated by the Mann-Whitney test. The chi-square test was used to compare categorical data. Correlations between ISHAK fibrosis score and variables were calculated using Pearson or Spearman correlation analysis, according to distribution of data. P values of < 0.05 were considered statistically significant. Binary logistic regression analysis was performed to analyze the Odds Ratio (OR) and Confidence Interval (CI) of the new predictive model associated with significant hepatic fibrosis.
The MedCalc version 14.8.1 (MedCalc Software, Mariakerke, Belgium) was used for the following statistical analyses. To distinguish patients as having mild or significant fibrosis, the Area Under Curve (AUC), sensitivity, specificity, Positive Predictive Value (PPV), and Negative Predictive Value (NPV) of each parameter were assessed by Receiver Operating Characteristic (ROC) analysis. The probable cut-off points for the optimal combination of sensitivity and specificity were determined by the Youden index. This study compared ROC curves to determine the statistical significance of the difference between AUC of 2 to 6, derived from the same data (32).
4. Results
The demographic, biochemical, and hematological parameters of 81 CHB patients, who underwent liver biopsies, are represented in Table 2. There were significant differences between the groups in terms of age yet not in sex distribution. The MMP-2 was significantly higher in patients with significant fibrosis (P = 0.003) compared with those with mild fibrosis yet there was no difference between the 2 groups regarding TIMP-2 and β-NGF. Whereas AST, GGT, total bilirubin, direct bilirubin, PT, INR, aPTT, and RDW levels in patients with significant fibrosis were significantly higher than in those with mild fibrosis, serum albumin, cholesterol, and PLT were significantly decreased in patients with significant fibrosis.
Parameters | Mild Fibrosis (n = 48) | Significant Fibrosis (n = 33) | P Valueb(Mild vs Significant Fibrosis) | R | P Valuec(Significance of R) |
---|---|---|---|---|---|
Age, year | 38.2 ± 11.7 | 55.1 ± 16.0 | < 0.001 | 0.566 | < 0.001 |
Male, n (%) | 27 (56.2) | 20 (60.6) | 0.696 | - | - |
MMP-2, ng/mL | 48.0 (45.5 - 52.0) | 52.5 (49.7 - 56.5) | 0.003 | 0.270 | 0.015 |
TIMP-2, pg/mL | 233.7 ± 49.0 | 252.6 ± 51.0 | 0.099 | 0.187 | 0.095 |
β-NGF, pg/mL | 239.8 (210.0 - 266.9) | 266.2 (195.7 - 315.6) | 0.445 | 0.105 | 0.351 |
Total protein, g/L | 7.5 (7.2 - 7.8) | 7.5 (6.9 - 7.7) | 0.122 | -0.243 | 0.033 |
Albumin, g/L | 4.4 (4.2 - 4.6) | 4.1 (3.9 - 4.5) | 0.010 | -0.407 | < 0.001 |
ALT, IU/L | 23.5 (18.5 - 32.0) | 24.5 (20.0 - 44.5) | 0.417 | 0.064 | 0.571 |
AST, IU/L | 25.0 (22.0 - 29.0) | 30.0 (24.5 - 41.0) | 0.004 | 0.303 | 0.006 |
ALP, IU/L | 82.0 (69.2 - 99.0) | 97.5 (65.0 - 116.0) | 0.660 | 0.050 | 0.661 |
GGT, IU/L | 21.0 (16.0 - 24.0) | 29.0 (20.5 - 59.5) | 0.001 | 0.460 | < 0.001 |
Total bilirubin, mg/dL | 0.75 (0.60 - 0.90) | 0.98 (0.80 - 1.53) | 0.003 | 0.319 | 0.004 |
Direct bilirubin, mg/dL | 0.21 (0.09 - 0.14) | 0.36 (0.16 - 0.53) | < 0.001 | 0.640 | < 0.001 |
Triglyceride, mg/dL | 113.0 (70.5 - 145.7) | 93.5 (70.0 - 124.5) | 0.274 | -0.156 | 0.170 |
Cholesterol, mg/dL | 194.0 (175.0 - 215.0) | 165.5 (145.5 - 190.5) | 0.002 | -0.403 | < 0.001 |
PT, s | 13.4 (12.8 - 14.0) | 14.6 (13.8 - 16.0) | < 0.001 | 0.628 | < 0.001 |
INR | 1.06 (1.01 - 1.12) | 1.13 (1.05 - 1.25) | 0.005 | 0.462 | < 0.001 |
aPTT, s | 28.1 (25.7 - 30.5) | 29.3 (28.1 - 32.1) | 0.020 | 0.309 | 0.006 |
PLT, 109/L | 225.5 (182.5 - 270.5) | 143.0 (72.5 - 203.0) | < 0.001 | -0.506 | < 0.001 |
Hematocrit, % | 41.5 (37.5 - 44.0) | 39.6 (38.2 - 43.2) | 0.298 | -0.103 | 0.365 |
RDW, % | 13.5 (12.9 - 14.3) | 14.4 (13.7 - 17.6) | < 0.001 | 0.475 | < 0.001 |
AFP, ng/mL | 2.70 (1.84 - 3.37) | 2.79 (2.27 - 3.72) | 0.253 | 0.067 | 0.557 |
CRP, mg/L | 0.3 (0.2 - 0.5) | 0.2 (0.2 - 0.6) | 0.636 | 0.069 | 0.565 |
Scores of noninvasive models in each patient group are shown in Table 3. Except AAR, all predictive noninvasive models were significantly higher in patients with significant fibrosis compared to patients with mild fibrosis.
A new combined noninvasive model, named Significant Hepatic Fibrosis Index-1 (SHFI-1) was created by choosing direct and indirect parameters that had higher significant positive and negative correlations as presented in Table 2. The SHFI-1 was determined by the following formula: (MMP-2 × age × PT × direct bilirubin) / (albumin × PLT). The SHFI-1 was significantly higher in patients with significant fibrosis compared to patients with mild fibrosis (Table 3). Significant fibrosis was distinguished from mild fibrosis when SHFI-1 was 6.82 as the cut-off value, and AUC was 0.894 (P < 0.001) (sensitivity 73.3%, specificity 95.6%, PPV 91.7%, NPV 84.3%) (Table 4). Binary logistic regression model showed that SHFI-1 could predict significant liver fibrosis in patients with CHB (OR 1.231, 95% CI 1.062-1.427, P = 0.006). The regression formula was as follows: y = -2.310 + 0.208x (R2 = 0.633, P < 0.001). With this regression equation, 85.3% of patients with significant fibrosis could be predicted. Due to the fact that MMP-2 is not routinely analyzed and its cost effectiveness is low, the SHFI-1 model might have an obstacle in clinical practice. Therefore, the model was simplified by excluding MMP-2 and renamed SHFI-2. Thus, SHFI-2 was formed as follows: (age × PT × direct bilirubin) / (albumin × PLT) formula. The SHFI-2 was also significantly higher in patients with significant fibrosis compared to patients with mild fibrosis (Table 3). In discriminating significant fibrosis versus mild fibrosis, the SHFI-2 cut-off value was set at 0.15 and the AUC cut-off was 0.885 (P < 0.001) (sensitivity 70.0%, specificity 97.8%, PPV 95.5%, and NPV 83.0%) (Table 4).
When SHFI-1 was compared with other noninvasive models, AUC of SHFI-1 was higher than SHFI-2 and the other models yet significant differences were not found, except S index, RPR, FCI, and AAR (Table 4). A comparison of the ROC curves of 6 noninvasive models with the higher AUCs, including SHFI-1 and SFHI-2, is presented in Figure 1.
Models | Mild Fibrosis (n = 48) | Significant Fibrosis (n = 33) | P Value (Mild vs Significant Fibrosis) |
---|---|---|---|
SHFI-1 | 3.05 (1.81 - 4.67) | 29.77 (5.33 - 79.98) | < 0.001 |
SHFI-2 | 0.067 (0.038 - 0.094) | 0.665 (0.098 - 1.930) | < 0.001 |
King score | 4.23 (3.24 - 6.33) | 13.49 (7.90 - 33.71) | < 0.001 |
FIB-4 | 0.84 (0.62 - 1.08) | 2.98 (1.25 - 4.37) | < 0.001 |
ELF | 8.2 (7.9 - 8.7) | 9.7 (8.8 - 11.0) | < 0.001 |
API | 2 (1 - 4) | 7 (4 - 8) | < 0.001 |
Fibro-Q | 1.78 (1.35 - 2.31) | 6.48 (2.31 - 11.03) | < 0.001 |
Forn’s index | 2.86 ± 1.46 | 6.87 ± 3.30 | < 0.001 |
APRI | 0.32 (0.23 - 0.40) | 0.75 (0.39 - 1.31) | < 0.001 |
GPRI | 0.18 (0.14 - 0.26) | 0.34 (0.25 - 1.61) | < 0.001 |
S index | 0.04 (0.03 - 0.07) | 0.10 (0.06 - 0.59) | < 0.001 |
RPR | 0.06 (0.05 - 0.07) | 0.11 (0.07 - 0.21) | < 0.001 |
FCI | 0.06 (0.04 - 0.08) | 0.16 (0.07 - 0.55) | < 0.001 |
AAR | 1.03 (0.86 - 1.23) | 1.17 (0.99 - 1.46) | 0.054 |
Models | Cutoff Value | Sensitivity, % | Specificity, % | PPV, % | NPV, % | AUC (95% CI) | P Valueb | P Valuec |
---|---|---|---|---|---|---|---|---|
SHFI-1 | 6.82 | 73.3 | 95.6 | 91.7 | 84.3 | 0.895 (0.802 - 0.954) | < 0.001 | - |
SHFI-2 | 0.15 | 70.0 | 97.8 | 95.5 | 83.0 | 0.885 (0.791 - 0.947) | < 0.001 | 0.301 |
King score | 7.44 | 80.6 | 87.2 | 80.6 | 87.2 | 0.885 (0.792 - 0.946) | < 0.001 | 0.906 |
FIB-4 | 1.09 | 87.1 | 76.6 | 71.1 | 90.0 | 0.885 (0.793 - 0.946) | < 0.001 | 0.750 |
ELF | 9.0 | 69.7 | 93.7 | 88.5 | 81.8 | 0.872 (0.779 - 0.936) | < 0.001 | 0.596 |
API | 5.00 | 67.4 | 95.7 | 91.3 | 81.8 | 0.861 (0.764 - 0.929) | < 0.001 | 0.191 |
Fibro-Q | 2.26 | 83.9 | 74.5 | 68.4 | 87.5 | 0.856 (0.758 - 0.925) | < 0.001 | 0.208 |
Forn’s index | 4.82 | 71.0 | 93.5 | 88.0 | 82.7 | 0.854 (0.755 - 0.924) | < 0.001 | 0.138 |
APRI | 0.66 | 56.2 | 95.8 | 90.0 | 76.7 | 0.829 (0.728 - 0.904) | < 0.001 | 0.199 |
GPRI | 0.22 | 81.2 | 68.1 | 63.4 | 84.2 | 0.828 (0.726 - 0.903) | < 0.001 | 0.155 |
S index | 0.05 | 80.6 | 69.6 | 64.1 | 84.2 | 0.822 (0.718 - 0.900) | < 0.001 | 0.033 |
RPR | 0.10 | 56.2 | 97.9 | 94.7 | 77.0 | 0.801 (0.697 - 0.882) | < 0.001 | 0.037 |
FCI | 0.11 | 67.7 | 86.7 | 77.8 | 80.0 | 0.781 (0.673 - 0.869) | < 0.001 | 0.023 |
AAR | 1.26 | 43.7 | 83.3 | 63.6 | 69.0 | 0.629 (0.514 - 0.735) | 0.047 | < 0.001 |
The ROC Curves of the First Six Noninvasive Models With the Higher AUCs
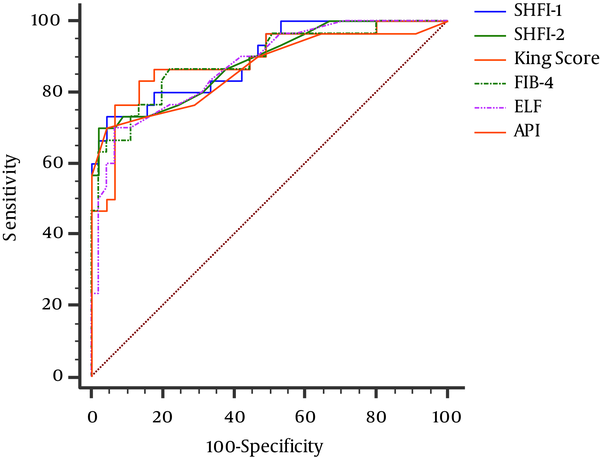
5. Discussion
This study aimed at identifying patients with significant fibrosis using noninvasive fibrosis markers, such as the direct and/or indirect models. This research showed that most of the noninvasive markers could distinguish significant fibrosis from mild fibrosis with different accuracies and significances. However, SHFI-1 as a new noninvasive fibrosis model had the highest AUC (95% CI). Since only serum MMP-2 level within the tested direct markers was associated with the hepatic fibrosis stage, this marker was included in the new noninvasive model. Age, PT, direct bilirubin, albumin, and PLT, which are indirect markers and are associated with the function and stage of hepatic disease, are also widely used within noninvasive models. In the current study, these parameters, which have the highest correlation with the degree of fibrosis were also included in the SHFI-1. According to the cut off value of 6.82 for SHFI-1, sensitivity, specificity, PPV, and NPV were respectively, 73.3%, 95.6%, 91.7%, and 84.3%. Logistic regression analysis showed that SHFI-1 may be used as a predictor of significant liver fibrosis. The OR of SHFI-1 was 1.231 and statistically significant (P = 0.006). It was assumed that SHFI-1 with this data is satisfactory to distinguish significant fibrosis. Similarly, Wu et al. indicated that models containing direct serum markers (Fibrometer, Shanghai Liver Fibrosis Group’s index, Hepascore) were more accurate predictors of significant fibrosis than models containing only indirect serum markers (APRI, FIB-4, Forn’s index) (33). However, noninvasive models are easy to calculate without additional costs, and contain validated and routinely available parameters in laboratories. Thus, a second model (SHFI-2) was formed by excluding MMP-2. The SHFI-2 showed similar performance to SHFI-1 and had better specificity (97.8%) and positive predictive value (95.5%) than all models. Thus, this final model, which is easy to practice with low cost, can be used to distinguish specific fibrosis with high specificity.
The first 5 models with the best AUC values except SHFI-1 or SHFI-2 were respectively, King score, Fib-4, ELF, API, and Fibro-Q. Most of the studies associated with these models have been firstly performed on hepatitis C patients. However, the performance of King score with 13.08 cut off value in the CHC patients was not found to be superior than the King score with 11.00 cut off value in patients with CHB. While the AUC, sensitivity, specificity, PPV, and NPV were respectively 0.783, 61%, 84%, 75%, and 72% in chronic hepatitis (CHC) patients, the AUC, sensitivity, specificity, PPV, and NPV were respectively 0.770, 60%, 83%, 66%, and 76% in CHB patients (34). Karacaer et al. found that the King score with cut off value of 5.76, had AUC of 0.807, sensitivity of 73%, specificity of 73%, PPV of 92.4%, and NPV of 36.3% [35]. This research found that optimum cut off value was 7.44, and the AUC, sensitivity, specificity, PPV, and NPV were 0.885, 80.6%, 87.2%, 80.6%, and 87.2%, respectively.
In studies performed on patients with CHB, AUC values of Fib-4 were 0.768, 0.687, 0.701, 0.720, and 0.750 for 0.73 (35), 1.09 (36), 1.02 (37), 1.7 (38), and 1.45 (39) cut off values, respectively. It was shown that AUC was 0.885 for cut off value of 1.09 and more effective for predicting significant fibrosis in patients with CHB. In the present study, the sensitivity, specificity, PPV, and NPV were 87.1%, 76.6%, 71.1%, and 90.0%, respectively.
The ELF is a biochemical test panel made up of serum markers reflecting ECM metabolism. This test panel consists of 3 parameters: HA, PIIINP, and TIMP-1. Serum levels of these parameters are used to calculate ELF score, which is used as a predictor of hepatic fibrosis. In studies, the ELF test used to identify ≥ 2 METAVİR score (equivalent to ≥ 3 ISHAK score) in patients with HBV, the AUC values were 0.901 (40), 0.820 (41) and 0.800 (42) for 8.5, 10.41, 8.75 cut off values, respectively. It was found that the 9.0 cut off value for ELF score had AUC of 0.872. The suggested cut off values might change among studies, and they may be influenced by etiologies, sample size, or ethnicity. According to the manufacturer, the AUC was 0.786 with cut-off value of 7.7. For this reason, it is reasonable to recommend that each laboratory might determine its own ELF score and cut-off values as part of good clinical practice.
Some researchers found that AUC of API was 0.680 and 0.580 (43, 44). The other ones also showed that AUC of API was 0.767 and 0.529 for 3.5 and 5.5 cut off values, respectively (34, 37). In this study, the AUC of API (0.861 for 5.0 cut off value) was higher than in other studies. The API was first implemented in patients with CHC and one of its 2 variables was age. This may explain why AUR for API was lower than other reports.
In order to Fibro-Q used for discriminating significant fibrosis, it was stated that different cut off values have different AUCs (for example 0.640 for 3.73 (39) and 0.783 for 1.6 (45). Zeng et al. showed that Fibro-Q had poor predictive value and was 0.569 of AUC (44). The current results showed that if Fibro-Q was above 2.26 for predicting significant fibrosis, AUC was 0.856. The sensitivity of Fibro-Q among all fibrosis models was the highest (83.9%).
The AUC values of Forn’s index, APRI, GPRI, S index, RPR, FCI, and AAR were lower than AUC of the first 7 models. Additionally, the AUC values of S index, RPR, FCI, and AAR had statically significant differences with AUC of SHFI-1. However, there were significant differences between mild and significant fibrosis for all models, except AAR. The AAR was more or less effective for differential diagnosis.
Liver biopsy was chosen as the reference method, yet it is known that it has some limitations. The tissues from different sites of the liver can be obstacles in the evaluation step (5). For example, a single biopsy specimen in a disease that does not affect each region of the liver equally cannot accurately reflect the characteristics of the disease since the biopsy sample represents approximately 1/50.000 of the adult liver (46). Liver biopsy is not ideal for frequent evaluations of the fibrosis stage under or after treatment due to its invasiveness (4) and this topic constitutes another limitation.
Transient Elastography (TE) is a novel and noninvasive technique for measuring liver stiffness (47). The TE includes a short procedure time, and the ability to perform the test at the bedside or in an outpatient clinic (48). The TE provides good performance for detecting significant fibrosis (40). However, applicability (80%) of TE is lower than serum biomarkers in case of obesity, ascites or operator experience. The TE also requires a specialized device with experienced operator (2). Due to the lack of TE device in the current hospital, this research was not able to show the TE results in the cohort and this was a major drawback of the study together with limited sample size. Lack of comparison of TE and SHFI-1 or SHFI-2 performances with each other is a limitation of this work.
Controversial results according to the literature might be the result of using different histopathological scoring systems (the METAVIR vs. the Ishak system), differences in patient populations or the prevalence of significant fibrosis.
Although HBV genotypes of the patients were not evaluated in this study, the HBV genotype D was the most common HBV genotype in Turkey. Hence, the researchers think that the results of the patients with HBV genotype D could be extended to other HBV genotypes (49). Additionally, since limited number of cases were tested in this study, the validation of SHFI-1 or SHFI-2 is required in large populations.
The selection of a test depends on individual patient factors, as well as the cost, accuracy, reliability, and availability of the test. The major advantage of the SHFI-2 is being an easily calculated model with low cost due to parameters being routinely analyzed in every laboratory. Especially for chronic HBV infection, treatment decision is based on the presence of necroinflammation rather than fibrosis, therefore, in such cases liver biopsy is still irreplaceable. The challenge now is to decide on how to apply validated noninvasive tests in HBV management. It is likely that a convenient combination approach (i.e., blood and imaging test as screening) will give the highest diagnostic accuracy, obviate the need for the greatest number of liver biopsies, and inform the clinician and patient regarding prognosis and the need for therapy.
References
-
1.
WHO. World Health Organization, Hepatitis B. 2017. Available from: http://www.who.int/mediacentre/factsheets/fs204/en/..
-
2.
European Association for Study of L. EASL-ALEH Clinical Practice Guidelines: Non-invasive tests for evaluation of liver disease severity and prognosis. J Hepatol. 2015;63(1):237. [PubMed ID: 25911335]. https://doi.org/10.1016/j.jhep.2015.04.006.
-
3.
Liu T, Wang X, Karsdal MA, Leeming DJ, Genovese F. Molecular serum markers of liver fibrosis. Biomark Insights. 2012;7:105-17. [PubMed ID: 22872786]. https://doi.org/10.4137/BMI.S10009.
-
4.
Fallatah SM. Successful management of complex regional pain syndrome type 1 using single injection interscalene brachial plexus block. Saudi J Anaesth. 2014;8(4):559-61. [PubMed ID: 25422619]. https://doi.org/10.4103/1658-354X.140903.
-
5.
Baranova A, Lal P, Birerdinc A, Younossi ZM. Non-invasive markers for hepatic fibrosis. BMC Gastroenterol. 2011;11:91. [PubMed ID: 21849046]. https://doi.org/10.1186/1471-230X-11-91.
-
6.
Sebastiani G, Alberti A. Non invasive fibrosis biomarkers reduce but not substitute the need for liver biopsy. World J Gastroenterol. 2006;12(23):3682-94. [PubMed ID: 16773685].
-
7.
Sebastiani G. Non-invasive assessment of liver fibrosis in chronic liver diseases: implementation in clinical practice and decisional algorithms. World J Gastroenterol. 2009;15(18):2190-203. [PubMed ID: 19437558].
-
8.
Jarcuska P, Janicko M, Veseliny E, Jarcuska P, Skladany L. Circulating markers of liver fibrosis progression. Clin Chim Acta. 2010;411(15-16):1009-17. [PubMed ID: 20399764]. https://doi.org/10.1016/j.cca.2010.04.009.
-
9.
Pinzani M, Vizzutti F, Arena U, Marra F. Technology Insight: noninvasive assessment of liver fibrosis by biochemical scores and elastography. Nat Clin Pract Gastroenterol Hepatol. 2008;5(2):95-106. [PubMed ID: 18253138]. https://doi.org/10.1038/ncpgasthep1025.
-
10.
Pinzani M, Rombouts K, Colagrande S. Fibrosis in chronic liver diseases: diagnosis and management. J Hepatol. 2005;42 Suppl(1):S22-36. [PubMed ID: 15777570]. https://doi.org/10.1016/j.jhep.2004.12.008.
-
11.
Tacke F, Weiskirchen R. Update on hepatic stellate cells: pathogenic role in liver fibrosis and novel isolation techniques. Expert Rev Gastroenterol Hepatol. 2012;6(1):67-80. [PubMed ID: 22149583]. https://doi.org/10.1586/egh.11.92.
-
12.
Rockey DC. Hepatic blood flow regulation by stellate cells in normal and injured liver. Semin Liver Dis. 2001;21(3):337-49. [PubMed ID: 11586464]. https://doi.org/10.1055/s-2001-17551.
-
13.
Iredale JP, Thompson A, Henderson NC. Extracellular matrix degradation in liver fibrosis: Biochemistry and regulation. Biochim Biophys Acta. 2013;1832(7):876-83. [PubMed ID: 23149387]. https://doi.org/10.1016/j.bbadis.2012.11.002.
-
14.
Liang B, Li Y, Zhao A, Xie F, Guo Z. Clinical utility of serum matrix metalloproteinase-2 and tissue inhibitor of metalloproteinase-2 concentrations in the assessment of liver fibrosis due to chronic hepatitis B. J Int Med Res. 2012;40(2):631-9. [PubMed ID: 22613424]. https://doi.org/10.1177/147323001204000225.
-
15.
Sumer S, Aktug Demir N, Kolgelier S, Cagkan Inkaya A, Arpaci A, Saltuk Demir L, et al. The Clinical Significance of Serum Apoptotic Cytokeratin 18 Neoepitope M30 (CK-18 M30) and Matrix Metalloproteinase 2 (MMP-2) Levels in Chronic Hepatitis B Patients with Cirrhosis. Hepat Mon. 2013;13(6). e10106. [PubMed ID: 24032040]. https://doi.org/10.5812/hepatmon.10106.
-
16.
Passino MA, Adams RA, Sikorski SL, Akassoglou K. Regulation of hepatic stellate cell differentiation by the neurotrophin receptor p75NTR. Science. 2007;315(5820):1853-6. [PubMed ID: 17395831]. https://doi.org/10.1126/science.1137603.
-
17.
Asai K, Tamakawa S, Yamamoto M, Yoshie M, Tokusashi Y, Yaginuma Y, et al. Activated hepatic stellate cells overexpress p75NTR after partial hepatectomy and undergo apoptosis on nerve growth factor stimulation. Liver Int. 2006;26(5):595-603. [PubMed ID: 16762005]. https://doi.org/10.1111/j.1478-3231.2006.01267.x.
-
18.
Kendall TJ, Hennedige S, Aucott RL, Hartland SN, Vernon MA, Benyon RC, et al. p75 Neurotrophin receptor signaling regulates hepatic myofibroblast proliferation and apoptosis in recovery from rodent liver fibrosis. Hepatology. 2009;49(3):901-10. [PubMed ID: 19072833]. https://doi.org/10.1002/hep.22701.
-
19.
Giannini E, Risso D, Botta F, Chiarbonello B, Fasoli A, Malfatti F, et al. Validity and clinical utility of the aspartate aminotransferase-alanine aminotransferase ratio in assessing disease severity and prognosis in patients with hepatitis C virus-related chronic liver disease. Arch Intern Med. 2003;163(2):218-24. [PubMed ID: 12546613].
-
20.
Liu CH, Lin JW, Tsai FC, Yang PM, Lai MY, Chen JH, et al. Noninvasive tests for the prediction of significant hepatic fibrosis in hepatitis C virus carriers with persistently normal alanine aminotransferases. Liver Int. 2006;26(9):1087-94. [PubMed ID: 17032409]. https://doi.org/10.1111/j.1478-3231.2006.01355.x.
-
21.
Sebastiani G, Vario A, Guido M, Alberti A. Sequential algorithms combining non-invasive markers and biopsy for the assessment of liver fibrosis in chronic hepatitis B. World J Gastroenterol. 2007;13(4):525-31. [PubMed ID: 17278217].
-
22.
Vardar R, Vardar E, Demiri S, Sayhan SE, Bayol U, Yildiz C, et al. Is there any non-invasive marker replace the needle liver biopsy predictive for liver fibrosis, in patients with chronic hepatitis? Hepatogastroenterology. 2009;56(94-95):1459-65. [PubMed ID: 19950810].
-
23.
Mohamadnejad M, Montazeri G, Fazlollahi A, Zamani F, Nasiri J, Nobakht H, et al. Noninvasive markers of liver fibrosis and inflammation in chronic hepatitis B-virus related liver disease. Am J Gastroenterol. 2006;101(11):2537-45. [PubMed ID: 17029616]. https://doi.org/10.1111/j.1572-0241.2006.00788.x.
-
24.
Cross TJ, Calvaruso V, Maimone S, Carey I, Chang TP, Pleguezuelo M, et al. Prospective comparison of Fibroscan, King's score and liver biopsy for the assessment of cirrhosis in chronic hepatitis C infection. J Viral Hepat. 2010;17(8):546-54. [PubMed ID: 19874477]. https://doi.org/10.1111/j.1365-2893.2009.01210.x.
-
25.
Bruno R, Sacchi P, Cima S, Maiocchi L, Patruno SF, Klersy C, et al. Correlation between FIB4, liver stiffness and metabolic parameters in patients with HIV and hepatitis C virus co-infection. Dig Liver Dis. 2011;43(7):575-8. [PubMed ID: 21596630]. https://doi.org/10.1016/j.dld.2011.03.009.
-
26.
Hsieh YY, Tung SY, Lee IL, Lee K, Shen CH, Wei KL, et al. FibroQ: an easy and useful noninvasive test for predicting liver fibrosis in patients with chronic viral hepatitis. Chang Gung Med J. 2009;32(6):614-22. [PubMed ID: 20035640].
-
27.
Forns X, Ampurdanes S, Llovet JM, Aponte J, Quinto L, Martinez-Bauer E, et al. Identification of chronic hepatitis C patients without hepatic fibrosis by a simple predictive model. Hepatology. 2002;36(4 Pt 1):986-92. [PubMed ID: 12297848]. https://doi.org/10.1053/jhep.2002.36128.
-
28.
Chen B, Ye B, Zhang J, Ying L, Chen Y. RDW to platelet ratio: a novel noninvasive index for predicting hepatic fibrosis and cirrhosis in chronic hepatitis B. PLoS One. 2013;8(7). e68780. [PubMed ID: 23874760]. https://doi.org/10.1371/journal.pone.0068780.
-
29.
Ahmad W, Ijaz B, Javed FT, Gull S, Kausar H, Sarwar MT, et al. A comparison of four fibrosis indexes in chronic HCV: development of new fibrosis-cirrhosis index (FCI). BMC Gastroenterol. 2011;11:44. [PubMed ID: 21507271]. https://doi.org/10.1186/1471-230X-11-44.
-
30.
Rosenberg WM, Voelker M, Thiel R, Becka M, Burt A, Schuppan D, et al. Serum markers detect the presence of liver fibrosis: a cohort study. Gastroenterology. 2004;127(6):1704-13. [PubMed ID: 15578508].
-
31.
Ishak K, Baptista A, Bianchi L. Histological grading and staging of chronic hepatitis. J Hepatol. 1995;22:696-9.
-
32.
DeLong ER, DeLong DM, Clarke-Pearson DL. Comparing the areas under two or more correlated receiver operating characteristic curves: a nonparametric approach. Biometrics. 1988;44(3):837-45. [PubMed ID: 3203132].
-
33.
Wu SD, Wang JY, Li L. Staging of liver fibrosis in chronic hepatitis B patients with a composite predictive model: a comparative study. World J Gastroenterol. 2010;16(4):501-7. [PubMed ID: 20101779].
-
34.
Eminler AT, Ayyildiz T, Irak K, Kiyici M, Gurel S, Dolar E, et al. AST/ALT ratio is not useful in predicting the degree of fibrosis in chronic viral hepatitis patients. Eur J Gastroenterol Hepatol. 2015;27(12):1361-6. [PubMed ID: 26352130]. https://doi.org/10.1097/MEG.0000000000000468.
-
35.
Karacaer Z, Avcı, O, Karadag, F. Y. King's Score may be More Effective in the Determination of Severe Fibrosis in Chronic Hepatitis B Infections. Viral Hepat J. 2017;23(1):20.
-
36.
Ucar F, Sezer S, Ginis Z, Ozturk G, Albayrak A, Basar O, et al. APRI, the FIB-4 score, and Forn's index have noninvasive diagnostic value for liver fibrosis in patients with chronic hepatitis B. Eur J Gastroenterol Hepatol. 2013;25(9):1076-81. [PubMed ID: 23510962]. https://doi.org/10.1097/MEG.0b013e32835fd699.
-
37.
Erdogan S, Dogan HO, Sezer S, Uysal S, Ozhamam E, Kayacetin S, et al. The diagnostic value of non-invasive tests for the evaluation of liver fibrosis in chronic hepatitis B patients. Scand J Clin Lab Invest. 2013;73(4):300-8. [PubMed ID: 23514016]. https://doi.org/10.3109/00365513.2013.773592.
-
38.
Liu XD, Wu JL, Liang J, Zhang T, Sheng QS. Globulin-platelet model predicts minimal fibrosis and cirrhosis in chronic hepatitis B virus infected patients. World J Gastroenterol. 2012;18(22):2784-92. [PubMed ID: 22719186]. https://doi.org/10.3748/wjg.v18.i22.2784.
-
39.
Coskun BD, Altinkaya E, Sevinc E. The diagnostic value of a globulin/platelet model for evaluating liver fibrosis in chronic hepatitis B patients. Rev Esp Enferm Dig. 2015;107(12):740-4.
-
40.
Kim BK, Kim HS, Park JY, Kim DY, Ahn SH, Chon CY, et al. Prospective validation of ELF test in comparison with Fibroscan and FibroTest to predict liver fibrosis in Asian subjects with chronic hepatitis B. PLoS One. 2012;7(7). e41964. [PubMed ID: 22848675]. https://doi.org/10.1371/journal.pone.0041964.
-
41.
Trembling PM, Lampertico P, Parkes J, Tanwar S, Vigano M, Facchetti F, et al. Performance of Enhanced Liver Fibrosis test and comparison with transient elastography in the identification of liver fibrosis in patients with chronic hepatitis B infection. J Viral Hepat. 2014;21(6):430-8. [PubMed ID: 24750297]. https://doi.org/10.1111/jvh.12161.
-
42.
Parikh P, Ryan JD, Tsochatzis EA. Fibrosis assessment in patients with chronic hepatitis B virus (HBV) infection. Ann Transl Med. 2017;5(3):40. [PubMed ID: 28251119]. https://doi.org/10.21037/atm.2017.01.28.
-
43.
Fung J, Lai CL, Fong DY, Yuen JC, Wong DK, Yuen MF. Correlation of liver biochemistry with liver stiffness in chronic hepatitis B and development of a predictive model for liver fibrosis. Liver Int. 2008;28(10):1408-16. [PubMed ID: 18482268]. https://doi.org/10.1111/j.1478-3231.2008.01784.x.
-
44.
Zeng X, Xu C, He D, Li M, Zhang H, Wu Q, et al. Performance of several simple, noninvasive models for assessing significant liver fibrosis in patients with chronic hepatitis B. Croat Med J. 2015;56(3):272-9. [PubMed ID: 26088852].
-
45.
Parsian H, Alizadeh M, Yahyapour Y. Clinical application of non-invasive markers of liver fibrosis. Pract Manag Chronic Viral Hepat. 2013;InTech:92-109.
-
46.
Sporea I, Sirli R, Popescu A, Cornianu M, Manciu C, Focsa M. The quality of the fragment obtained by liver biopsy for staging chronic hepatitis. J Gastrointestin Liver Dis. 2007;16(3):263-6. [PubMed ID: 17925919].
-
47.
Foucher J, Chanteloup E, Vergniol J, Castera L, Le Bail B, Adhoute X, et al. Diagnosis of cirrhosis by transient elastography (FibroScan): a prospective study. Gut. 2006;55(3):403-8. [PubMed ID: 16020491]. https://doi.org/10.1136/gut.2005.069153.
-
48.
European Association for Study of L, Asociacion Latinoamericana para el Estudio del H. EASL-ALEH Clinical Practice Guidelines: Non-invasive tests for evaluation of liver disease severity and prognosis. J Hepatol. 2015;63(1):237-64. [PubMed ID: 25911335]. https://doi.org/10.1016/j.jhep.2015.04.006.
-
49.
Tozun N, Ozdogan O, Cakaloglu Y, Idilman R, Karasu Z, Akarca U, et al. Seroprevalence of hepatitis B and C virus infections and risk factors in Turkey: a fieldwork TURHEP study. Clin Microbiol Infect. 2015;21(11):1020-6. [PubMed ID: 26163105]. https://doi.org/10.1016/j.cmi.2015.06.028.