Abstract
Background:
Individuals with breast cancer may experience multiple types of recurrence and a terminal event during their life. Follow-up may be interrupted for several reasons, including patients lost to follow-up or end of a study. Death is considered as a dependent terminal event.Objectives:
The main objective was to model the dependency between (locoregional and metastaticrelapses and death in breast cancer patients.Methods:
This retrospective cohort study was conducted in Cancer Research Center, Shahid Beheshti University of Medical Sciences on 342 patients with BC. Patients were followed up to 20 years from January 1996 until February 2015. This study considers the analysis of diseases recurrence and survival for joint modeling of three correlated evens: Local recurrence, metastasis, and death. The aims are to detect the effects of relapses on death and the correlation between local and distant recurrences. We propose a joint frailty model for multiple recurrent events with a terminal event.Results:
According to all obtained results of the fitted models, the risk of local and metastatic relapses or death increased for women with positive lymph node (N+) or for women with a grade higher than I. Also, it was found that if the association between these 3 times to events are not taken into account, we may lose a significant association. The variable HR+ was significantly correlated with the hazards of two types of recurrences, and death for both reduced and proposed models (P < 0.05).Conclusions:
We concluded that the risk of locoregional recurrences is correlated with the risk of metastatic and the risk of metastatic relapse is also correlated with death. In this special setting, it can be difficult to get an independence between locoregional and distant recurrences. Also, these results should be considered with caution, with the few number of recurrences.Keywords
Breast Cancer Disease Recurrence Survival Analysis Frailty Model
1. Background
Desired event can be experienced more than once in many studies, for each person. Such outcomes have been named as recurrent events. Such data occur mostly in longitudinal follow-up research. Example for recurrent events include bladder tumor relapse times among patients in a and recurrent heart attacks (1, 2). In other words, recurrent event data are mostly faced with clinical and observational studies regarding biomedical science. The effect of two kinds of recurrences on the survival of patients with breast cancer has been a subject of controversy for the recently decades. Many researchers assert that, in many of patients, breast cancer is a systemic illness from its starting and that node situation and local relapse are risk factors instead of the source of distant relapse (3). Breast cancer is a heterogeneous illness with various clinical behaviors and treatment outcomes, notwithstanding similarities in characteristics, like the lymph node status, tumor size, and grade. This diversity can reflect the basic molecular biology of the illness. Molecular subtypes have been correlated with multiple recurrence rates and survival of patients with breast cancers. Generally, triple negative (TN) subgroup experience higher rates of local relapse and distant metastases, also lower survival rates. With the prognostic information obtained from molecular subtype analyses, there is refinement for personalized treatment for patients with breast cancer. Major component for treatment based on molecular subtype is the better conception of local and distant relapse in the presence of terminal event (4). The effect of local and distant relapse on the outcome of patients with breast cancer has recently been a contention matter. Several authors assert that breast cancer is a systemic disease that node status and locoregional relapse are risk agents instead of the source of distant relapse. In many studies, adjuvant chemotherapy was related with decreased local and distant relapse rates and also with an increased relapse free survival rate, but not an increased whole survival rate. Various papers showed a high risk of experiencing a distant relapse after a local recurrence (5). O’Shaughnessy showed a high link between a metastatic recurrence and death (6). In several situations, recurrent events are as important measurements for determining progression of disease. According to statistical literature, non-informative censoring is commonly assumed when statistical methods are expanded or analyzing recurrent event data. In recurrent event data analysis, there are different models suggested in the matter of survival analysis, including conditional intensity models (7-9), marginal intensity models, the frailty model, and marginal means (10, 11). In so many applications, although, there is a dependent terminal event like death that stops the follow-up. For instance, patients may experience repeated hospitalizations, which are terminated by death. For this special case, terminal event (death) should be accounted for here because it is probably be correlated with recurrent events. In recent decades, statistical analysis of recurrent event in the presence of terminal event has received much interest. For recurrent event data with a terminal event, both frailty and marginal methods are the commonly existing approaches, that for considering the relationship between the recurrent and terminal events, frailty models use random effects. For instance, some authors such as Huang for recurrent events and the terminal event proposed a frailty model with proportional intensity and proportional hazards, respectively (12). Others provided a joint semi-parametric model, in which for considering the relation between the recurrent event data and terminal event, a shared gamma frailty hazard function is applied. (13). Cook studied different classes of robust procedures for modeling the recurrent event mean in the presence of terminating event (14).
In this paper, we consider two various kinds of recurrent events that can be correlated. In addition, death is assumed as a terminal event for the recurrence. Joint analyzing recurrent events with terminal event to making reliable inferences is essential. For joint-modeling of 3 correlated time-to-event responses, including locoregional relapse, distant relapse, and overall survival, a joint frailty hazard model is expanded.
2. Objectives
In the current study, we aimed at determining the risk factors correlated with the incidence of breast cancer, local recurrence, distant recurrence, and death. Moreover, this study is taking into account the dependencies between these 3 events.
3. Methods
This registry-based retrospective cohort study was conducted in Cancer Research Center, Shahid Beheshti University of Medical Sciences, using medical records of 342 patients with BC. Information of all patients with BC were registered in Cancer Research Center, Shahid Beheshti University of Medical Sciences. They were followed-up for about 20 years from January 1998 until February 2016. Based on the time protocol of the Cancer Research Center, the patient’s information was reviewed and updated. Criteria for entering each patient in the study included all patients with a definite diagnosis of breast cancer, who were followed-up at Shohada Tajrish Hospital for at least 6 months after surgery. Exclusion criteria in this study were incomplete information for each patient, this observation was made due to a defect in medical records and patient pathology reports. And also, patients who have been followed-up for up to 5 months and variables that overlap with the results of the research was removed. Finally, after a first diagnosis, a total of 342 patients were included in the study. Patients from the time of breast-conserving surgery were considered at risk of recurrence or death. In the current study 52.4% of them experienced recurrent event and 47.6% were lost to followed-up. We will describe the data first; then, we examined the further information, using a mathematical model. Lymph node involvement (node: N+ or N−), age, positive status of hormone receptors (HR+ or HR−), tumor size, HER2+ and grade were included. If at least one of axillary lymph nodes was metastatic, it is considered N+. If more than 10% of tumor cells were positive by immune histochemical analysis, a tumor was considered HR+. HER2 is a protein and if its rate is unnatural raised at the level of the tumoral cells, then the tumor is categorized HER2+ (15). In addition, the current study was extracted from a Ph.D. thesis, which was checked and approved by the Ethics Committee of the Tarbiat Modares University of Medical Sciences (IR.TMU.REC.1396.632).
3.1. Statistical Analysis
Descriptive characteristics of the patients are shown as mean (± standard deviation) and frequency (percentage) for continuous and categorical variables, respectively.
For a patient i (i = 1, 2, …, n), ni and n’i are indicative of the numbers of locoregional and metastatic, respectively; if there is no relapse for the patient, it will be zero. Here, 3 times to event responses were considered for individual i denoted by

where T1ij is the time interval between surgical resection of the tumor and the jth locoregional relapse for the ith patient. T2ij’ is also the time interval between surgical resection of the tumor to the jth metastasis too; likewise, T3i is survival time that is the time interval between surgical to death time or end of follow-up. The hazard functions for 3 times to event are:
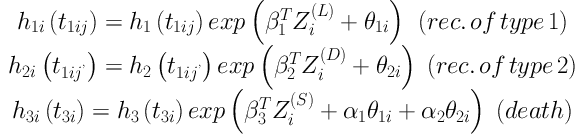
where h1(t), h2(t), and h3(t) are unknown baseline hazards for locoregional relapse, distant relapse, and survival, respectively; Z(L), Z(D), and Z(S) are local relapse, distant relapse, and survival covariates vectors, respectively, and
4. Results
This results were obtained from 342 women with BC. In this cohort study, the patients’ age in this study was between 22 and 84 years. The mean (± standard deviation) age at the diagnosis of patients with BC was 47.84 ± 11.75 years; 12.9% of the patients had stage I of a disease, 49.1% were in stage II, 35.4% were in stage III, and only 2.6% of the patients were in stage IV of disease (the most dangerous stage of the disease). Frequency distribution of variables in this study are presented in Table 1. The median follow-up period was 30.57 months with a range of 6 to 187 months that was established by the inverse Kaplan-Meier procedure. In this study, a summary of the data is presented in the Cancer Research Center. Overall, 218 (64.1%) patients did not experience a recurrence, of whom, 42 (19.7%) died. A total of 87 (25.5%) had a distant relapse, 56 (16.5%) experienced one local relapses, and 2 (< 1%) had 2 local recurrences. Actually, 41 (12.1%) of them had a distant recurrences before dying, while less than 1% of the cases experienced a local recurrence before death. In addition, 25 (7.3%) of them had experienced a local recurrence, a distant recurrences, and death. Also, within the period of follow-up, 106 (31.0%) of patients with breast cancer had died; so, the rest of patients at the end of the study were censored. Here, assuming independent censoring in each recurrences or death, many of patients that followed for a long time, finally died after a distant relapse. The number of these patients was about 12 times bigger than the other group of patients that died after a local recurrence. It implies that metastasis is a fatal event. The patients’ characteristics results are shown in Table 1. Then, the proposed model is used to assess different recurrences and survival.
4.1. Results from Joint Frailty Model
In this study, a joint frailty model with constant baseline hazard functions and 3 separate reduced models were fitted that were approximated by smoothing methods; the method used here was maximum of the penalized likelihood estimation method, in which the baseline hazard functions are approximated by smoothing methods. The results are shown in Table 2. Also, baseline hazard functions for two types of recurrences two types of recurrences, and death after a breast cancer obtained by fitting proposed multivariate frailty models are shown in Figure 1. Due to all the results obtained from the fitted models, we found that the risk of local, distant relapse, and death increased for subjects with a grade greater than I or for individuals with at least one positive lymph node (N+). For all fitted models, a significant effect was seen in tumor size (> 20 mm) for the risks of these three outcomes and for individuals younger than 40 years versus individuals older than 60, the hazard of death was not various significantly, but for individuals between 40 and 60 years, it was significantly lower compared to patients older than 60 years. The hazard of distant recurrence or local recurrence excesses for individuals was lower than 40 years versus to individuals older than 60 years. Also, the impact of tumor size for the hazard of local relapse did not specify in the reduced model. There was no relationship between HER2+ and the risk of death, but the risk of local and metastatic relapses was higher for HER2+ patients. We detected in the proposed model that for patients with tumors sizes higher than 20 mm, the risk of local, distant relapse or death increase. According to the obtained results of the reduced models, the variable tumor size significantly affects the risk of metastatic relapses and death; but, as we observed, it was not significant for the risk of local relapses. According to the obtained results from the reduced models, an underestimation in regression coefficient was observed, particularly for the death hazard function observed. The variable HR+ was significantly correlated with the risk of local recurrence, distant relapses, and death for all fitted models (P < 0.05). So, we decided not to keep these factors in the original analysis. Also, it was found that the proportionality hazard assumption by using the Cox model for variable HR+ was not consistent. This can be the reason for no-significantly of the whole period follow-up. When the follow-up period was divided into two periods: 0 to 5 years and 5 to 20 years, then this hypothesis is satisfied. For the first and second part, the effect of HR+ is significant and reduce any type of recurrences. The rest of variables satisfied the PH assumption. In these models, both η and α2 parameters are significantly different from zero; it implies a positive correlation between death and the risk of distant recurrence. The parameter α1 was not significantly different from zero, which was contrary to the parameter θ. This shows inter recurrence association between them and no dependency between the risk of death and local recurrences. The correlation coefficient P representing a strong correlation between the risk of locoregional relapses and distant relapse was significantly high and different from zero. Because distant recurrences happen before death, the correlation between local recurrences and death is not significant from the proposed model.
Baseline hazard functions for two types of recurrences, and death processes after a breast cancer obtained by fitting proposed multivariate frailty models
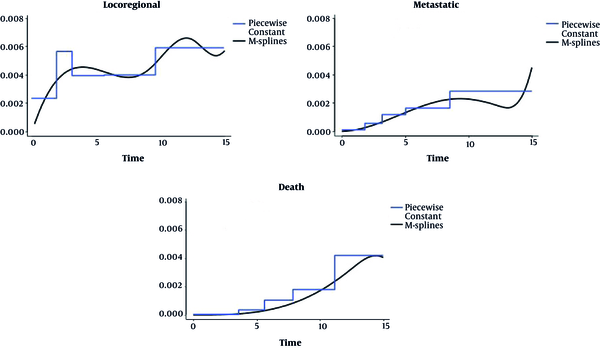
Frequency Distribution of Characteristics in Patients with Breast Cancer
Variable, Modalities | No. (%) |
---|---|
Family history | |
No | 233 (68.1) |
First degree | 52 (15.2) |
Second-degree | 57 (16.7) |
Surgery | |
BCS | 219 (64) |
MRM | 123 (36) |
Stage | |
I | 44 (12.9) |
II | 168 (49.1) |
III | 121 (35.4) |
IV | 9 (2.6) |
Grade | |
I | 37 (10.8) |
II | 191 (55.8) |
III | 114 (33.3) |
Chemotherapy | |
Yes | 330 (96.5) |
No | 12 (3.5) |
The number of involved lymph nodes | |
0 | 113 (33.1) |
1 - 3 | 127 (37.1) |
3 - 10 | 75 (21.9) |
> 10 | 27 (7.9) |
Hormone therapy | |
Without hormone therapy | 10 (2.9) |
Tamoxifen | 286 (83.6) |
Letrozole | 27 (7.9) |
Other hormonal treatments | 19 (5.6) |
Analysis of Frailty and Reduced Models of the Local and Distant Relapses and Survival in Patients with Breast Cancer
Variables, Modalities | Proposed (Piecewise Constant) | Reduced | ||
---|---|---|---|---|
HR | (95% CI) | HR | (95% CI) | |
For Local Relapses | ||||
Age, y (ref: > 55) | ||||
≤ 40 | 2.86 | (1.76 - 4.64) | 2.59 | (1.77 - 3.93) |
> 40 and ≤ 55 | 1.32 | (0.94 - 1.86) | 1.46 | (1.07 - 1.99) |
Grade (ref: I) | ||||
II | 1.63 | (1.15 - 2.30) | 1.81 | (1.21 - 2.69) |
III | 2.05 | (1.33 - 3.17) | 1.84 | (1.18 - 2.92) |
Lymph node (ref: N-) | ||||
N+ | 2.45 | (1.49 - 4.03) | 1.41 | (1.04 - 1.91) |
Tumor size, mm (ref: < 20) | ||||
≥ 20 | 1.61 | (1.15 - 2.25) | 1.34 | (0.98 - 1.86) |
HER2+ (ref: No) | ||||
Yes | 1.83 | (1.18 - 2.82) | 1.59 | (1.09 - 2.34) |
For Metastatic Relapses | ||||
Age, y (ref: > 55) | ||||
≤40 | 2.81 | (1.31 - 6.03) | 1.85 | (1.29 - 2.67) |
> 40 and ≤ 55 | 0.80 | (0.49 - 1.29) | 1.09 | (0.84 - 1.42) |
Grade (ref: I) | ||||
II | 2.79 | (1.53 - 5.09) | 1.63 | (1.16 - 2.31) |
III | 4.56 | (2.26 - 9.20) | 1.89 | (1.29 - 2.78) |
Lymph node (ref: N-) | ||||
N+ | 3.47 | (2.15 - 5.59) | 1.99 | (1.53 - 2.59) |
Tumor size, mm (ref: < 20) | ||||
≥ 20 | 3.95 | (2.37 - 6.60) | 1.55 | (1.11 - 2.15) |
HER2+ (ref: No) | ||||
Yes | 2.19 | (1.10 - 4.34) | 1.76 | (1.36 - 2.28) |
For Death | ||||
Age, y (ref: > 55) | ||||
≤ 40 | 1.22 | (0.39 - 3.80) | 0.94 | (0.64 - 1.38) |
> 40 and ≤ 55 | 0.29 | (0.14 - 0.59) | 0.66 | (0.51 - 0.84) |
Grade (ref: I) | ||||
II | 4.59 | (1.79 - 11.74) | 1.70 | (1.24 - 2.31) |
III | 14.78 | (4.76 - 45.90) | 2.36 | (1.67 - 3.33) |
Lymph node (ref: N-) | ||||
N+ | 4.33 | (2.06 - 9.08) | 1.74 | (1.37 - 2.19) |
Tumor size, mm (ref: < 20) | ||||
≥ 20 | 5.92 | (2.53 - 13.86) | 1.61 | (1.27 - 2.04) |
HER2+ (ref: No) | ||||
Yes | 2.10 | (0.75 - 5.85) | 1.33 | (0.97 - 1.83) |
η = var (vi) (SE) | 1.10 | (0.11) | ||
θ = var (ui) (SE) | 7.39 | (0.63) | ||
α1 (SE) | -0.25 | (1.45) | ||
α2 (SE) | 1.66 | (0.59) | ||
ρ (SE) | 0.99 | (0.01) |
5. Discussion
This article aimed at estimating the prognostic factors correlated with the occurrences of types of breast cancer relapses, and death. It also assesses the dependencies between these events. Different papers showed that after a locoregional recurrence, risk of experiencing a metastatic relapse is high (5). O’Shaughnessy detected a high and significant link between a metastatic event and death (6). The relation between locoregional recurrence and death has been found for young women in Elkhuizen et al. (19). This association is not clear for any women in Monteiro-Grillo et al. (20). In this study, two different types of recurrent events was considered, which could be correlated. In addition, death is considered as a terminal event too; the coefficients α1 and α2 represented the sign of the association whether, locoregional or/and metastatic relapses, and death are negatively or positively correlated significantly. The variances of the (ui, vi) indicated the dependencies between locoregional relapses, metastatic relapses and death. This method, can analysis the association between breast cancer relapses, and death. The results obtained from proposed model and three distinct reduced models are shown in Table 2. Based on all models, it can be said that the risk of two types of recurrences, or death increased for women with positive lymph node (N+) or for women with a grade higher than I. In all obtained models, the hazard of death was not significantly diverse for women younger than 40 years versus older than 55 years. Also, the risk of any kinds of recurrences excess for patients younger than 40 years compared to others. Tumor size has a significant impact for the hazard of these three outcomes. The reduced model did not discover the impact of tumor size for the risk of locoregional relapse. The risk of multiple recurrences was higher for HER2+ women, but no relationship was found with the risk of death. These models detected that the risk of two types of recurrences, or death excessed for women with tumors higher than 20 mm. The reduced models discovered only a significant impact of tumor size variable for the hazard of metastatic relapse and death; but for the risk of locoregional relapse was not significant. This shows that if the correlation between these three outcomes is not taken into account, we may lose an important association.
The variable θ was significantly various from 0, but α1 was not. This implies that there was interrelapse dependency and no correlation between the risk of locoregional recurrences and death. The coefficient P was also high and significant, demonstrating a high dependency between the risk of two types of recurrences. Because of distant relapse often happens before dying, it is not strang that this model didn’t detect the correlation between locoregional relapses and death. The risk of death is not directly correlated with the risk of locoregional relapse. Althogh, Wapnir et al. found a dependence between locoregional recurrence and death (21). In our application, few recurrences of both types were observed. The random effects ui and vi representing the relationship between locoregional recurrence and death or between distant recurrence and death, than within subject dependence.
4.1. Conclusions
In this study, we presented a multivariate frailty model that simultaneously model two types of recurrences with a dependent terminal event. The proposed model can express the dependency among these multiple recurrent events, but it could also deal with the correlation between recurrent and terminal events. Results of the current study indicated that the proposed method works well and was better than applying 3 distinct reduced models. In our application, it was difficult to get an independence between locoregional and distant metastasis relapses. This can illustrate the value of the correlation coefficient, ρ too much. It should be noticed that, these results must be considered with caution, given the few number of recurrences.
Acknowledgements
References
-
1.
Cook RJ, Lawless JF. The statistical analysis of recurrent events. Springer Science & Business Media; 2007. https://doi.org/10.1007/978-0-387-69810-6.
-
2.
Osmani F, Hajizadeh E, Mansoori P. [Use of smoothing methods for estimating the coefficients of time dependent rate models in survival analysis and its application in psoriasis disease]. Iran J Epidemiol. 2016;12(3):36-46. Persian.
-
3.
Liu L, Huang X. Joint analysis of correlated repeated measures and recurrent events processes in the presence of death, with application to a study on acquired immune deficiency syndrome. J R Stat Soc C App Stat. 2009;58(1):65-81. https://doi.org/10.1111/j.1467-9876.2008.00641.x.
-
4.
Cancer Genome Atlas N. Comprehensive molecular portraits of human breast tumours. Nature. 2012;490(7418):61-70. [PubMed ID: 23000897]. [PubMed Central ID: PMC3465532]. https://doi.org/10.1038/nature11412.
-
5.
Montagna E, Bagnardi V, Rotmensz N, Viale G, Renne G, Cancello G, et al. Breast cancer subtypes and outcome after local and regional relapse. Ann Oncol. 2012;23(2):324-31. [PubMed ID: 21525402]. https://doi.org/10.1093/annonc/mdr129.
-
6.
O'Shaughnessy J. Extending survival with chemotherapy in metastatic breast cancer. Oncologist. 2005;10 Suppl 3:20-9. [PubMed ID: 16368868]. https://doi.org/10.1634/theoncologist.10-90003-20.
-
7.
Prentice RL, Williams BJ, Peterson AV. On the regression analysis of multivariate failure time data. Biometrika. 1981;68(2):373-9. https://doi.org/10.1093/biomet/68.2.373.
-
8.
Andersen PK, Gill RD. Cox's regression model for counting processes: A large sample study. Ann Stat. 1982;10(4):1100-20. https://doi.org/10.1214/aos/1176345976.
-
9.
Zeng D, Lin DY. Efficient estimation of semiparametric transformation models for counting processes. Biometrika. 2006;93(3):627-40. https://doi.org/10.1093/biomet/93.3.627.
-
10.
Wei LJ, Lin DY, Weissfeld L. Regression analysis of multivariate incomplete failure time data by modeling marginal distributions. J Am Stat Assoc. 1989;84(408):1065-73. https://doi.org/10.1080/01621459.1989.10478873.
-
11.
Lee EW, Wei LJ, Amato DA, Leurgans S. Cox-type regression analysis for large numbers of small groups of correlated failure time observations. Survival analysis: State of the art. Netherlands: Springer; 1992. p. 237-47. https://doi.org/10.1007/978-94-015-7983-4_14.
-
12.
Huang CY, Wang MC. Joint modeling and estimation for recurrent event processes and failure time data. J Am Stat Assoc. 2004;99(468):1153-65. [PubMed ID: 24068850]. [PubMed Central ID: PMC3780991].
-
13.
Ye Y, Kalbfleisch JD, Schaubel DE. Semiparametric analysis of correlated recurrent and terminal events. Biometrics. 2007;63(1):78-87. [PubMed ID: 17447932]. https://doi.org/10.1111/j.1541-0420.2006.00677.x.
-
14.
Cook RJ, Lawless JF, Lakhal-Chaieb L, Lee KA. Robust estimation of mean functions and treatment effects for recurrent events under event-dependent censoring and termination: Application to skeletal complications in cancer metastatic to bone. J Am Stat Assoc. 2009;104(485):60-75. https://doi.org/10.1198/jasa.2009.0004.
-
15.
Huang X, Liu L. A joint frailty model for survival and gap times between recurrent events. Biometrics. 2007;63(2):389-97. [PubMed ID: 17688491]. https://doi.org/10.1111/j.1541-0420.2006.00719.x.
-
16.
Mazroui Y, Mathoulin-Pelissier S, Macgrogan G, Brouste V, Rondeau V. Multivariate frailty models for two types of recurrent events with a dependent terminal event: Application to breast cancer data. Biom J. 2013;55(6):866-84. [PubMed ID: 23929494]. https://doi.org/10.1002/bimj.201200196.
-
17.
DeSantis C, Siegel R, Bandi P, Jemal A. Breast cancer statistics, 2011. CA Cancer J Clin. 2011;61(6):409-18. [PubMed ID: 21969133]. https://doi.org/10.3322/caac.20134.
-
18.
Wu X, Baig A, Kasymjanova G, Kafi K, Holcroft C, Mekouar H, et al. Pattern of local recurrence and distant metastasis in breast cancer by molecular subtype. Cureus. 2016;8(12). e924. [PubMed ID: 28090417]. [PubMed Central ID: PMC5222631]. https://doi.org/10.7759/cureus.924.
-
19.
Elkhuizen PHM, van de Vijver MJ, Hermans J, Zonderland HM, van de Velde CJH, Leer JWH. Local recurrence after breast-conserving therapy for invasive breast cancer: High incidence in young patients and association with poor survival. Int J Radiat Oncol Biol Phys. 1998;40(4):859-67. https://doi.org/10.1016/s0360-3016(97)00917-6.
-
20.
Monteiro-Grillo I, Marques-Vidal P, Jorge M. Psychosocial effect of mastectomy versus conservative surgery in patients with early breast cancer. Clin Transl Oncol. 2005;7(11):499-503. [PubMed ID: 16373061].
-
21.
Wapnir IL, Anderson SJ, Mamounas EP, Geyer CE Jr, Jeong JH, Tan-Chiu E, et al. Prognosis after ipsilateral breast tumor recurrence and locoregional recurrences in five National Surgical Adjuvant Breast and Bowel Project node-positive adjuvant breast cancer trials. J Clin Oncol. 2006;24(13):2028-37. [PubMed ID: 16648502]. https://doi.org/10.1200/JCO.2005.04.3273.