Abstract
Context:
Contact tracing is a cornerstone community-based measure for augmenting public health response preparedness to epidemic diseases such as the current coronavirus disease 2019 (COVID-19). However, there is no an agreed data collection tool for the unified reporting of COVID-19 contact tracing efforts at the national level.Objectives:
The purpose of this research was to determine the COVID-19 Contact Tracing Minimal Dataset (COV-CT-MDS) as a prerequisite to develop a mobile-based contact tracing system for the COVID-19 outbreak.Methods:
This study was carried out in 2020 by a combination of literature review coupled with a two-round Delphi survey. First, the probable data elements were identified using an extensive literature review in scientific databases, including PubMed, Scopus, ProQuest, Science Direct, and Web of Science (WOS). Then, the core data elements were validated using a two-round Delphi survey.Results:
Out of 388 articles, 24 were eligible to be included in the study. By the full-text study of the included articles and after the Delphi survey, the designed COV-CT-MDS was categorized into two clinical and administrative data sections, nine data classes, and 81 data fields.Conclusions:
COV-CT-MDS is an efficient and valid tool that could provide a basis for collecting comprehensive and standardized data on COVID-19 contact tracing. It could also provide scientific teamwork for health care authorities, which may lead to the enhanced quality of documentation, research, and surveillance outcomes.Keywords
Contact Tracing COVID-19 Coronavirus Mobile Application Data Collection Pandemic
1. Context
Contact tracing is a principal public health practice for containing further propagation of the virus through limiting contacts between infected cases and persons adjacent to them (eg, family members, health care providers, healthcare personnel, etc.) (1-3). Contact tracing is principally significant for the COVID-19 outbreak, where a large number of carriers are silent, pre-symptomatic, or may present only mild symptoms and are thus usually not tested, despite having the potential to promulgate the disease (4). In the context of COVID-19, contact tracing is a public health response to detect and inform those individuals who may have been in close contact with an infected person every day for two weeks (5, 6). Accordingly, if an individual is confirmed positive for COVID-19, every other individual who had possibly been in close contact is tracked and recommended to go into protective self-quarantine for cutting off the transmission chain of the disease in the community (7).
To overcome the limitations of traditional contact tracing, digital-based contact tracing has been adopted (8). One promising type of digital contact tracing is the implementation of mobile-based contact tracing applications (apps). Such apps use mobile devices to promptly detect and alert users who may be in close contact with a confirmed-COVID-19 case (9). Due to the wide accessibility and affordability of mobile devices, employing mobile-based contact tracing apps can lead to making the public health process of contact tracing more efficient on a massive scale (10).
Mobile-based contact tracing systems offer a practical solution to controlling the spread of COVID-19; however, standardized data collection as one of the designing specification criteria to achieve a uniform and mass tracing app acceptance is a great challenge (11, 12). Moreover, from a data management perspective, the novelty of COVID-19 has created major gaps in data harmonization, integration, and unified reporting of disease as a basis for investigating many unfamiliar clinical aspects and outcomes of the disease, characterizing the public health threat, and supporting health authorities’ decisions (13).
The human-to-human spread of COVID-19 requires active case identification, that is, early confinement, timely testing, and treatment, besides detection and future tracking of persons who may be in close contact with infected cases (14). Meanwhile, a large number of reports inflowing the health care systems from varied networks and formats need to be validated. Current surveillance systems are generally not constructed to meet such data requirements. Moreover, vagueness and postponement of surveillance data due to isolated and heterogeneous health information systems are a barrier to data exchange among these systems, which have led to limited consistency of epidemiologic studies (15).
To our knowledge, no comprehensive data collection template currently exists that has been designed to capture high-quality, consistent, and standardized data regarding COVID-19 contact tracing.
2. Objectives
To address this priority, the current study aims to determine a minimum dataset (MDS) as an essential measure before the design and implementation of a digital contact tracing system. Accordingly, we sought to develop a COVID-19 Contact Tracing Minimal Dataset (COV-CT-MDS) based on mobile devices due to their ability to appropriately document contact tracing data during COVID-19.
3. Methods
This was a cross-sectional study conducted in 2020 following a combination of literature review and a broad discussion with a multidisciplinary team of involved healthcare experts, as follows.
3.1. Literature Review
3.1.1. Search Strategy
A systematic review was undertaken to extract the primary data elements to include in COV-CT-MDS. This systematic review was reported according to the recommendations of the Preferred Reporting Items for Systematic Reviews and Meta-Analyses (PRISMA) statement (16). PubMed, Scopus, Web of Science (WOS), Science Direct, and ProQuest databases were reviewed between 1 January 2020 and 20 December 2020 to determine the required data elements, features, and attributes for designing a mobile-based COV-CT-MDS. The following search terms were used (designed using English MeSH keywords) to maximize the output from literature findings: [COVID-19 OR Novel coronavirus OR SARS-CoV-2 OR n-CoV2] AND [Mobile phone OR Smartphone OR Cell phone OR Mobile Apps OR Mobile health] AND [Contact tracing OR Contact tracking].
3.1.2. Study Selection
Two independent researchers (M: SH and H: K-A) reviewed the titles and abstracts of the articles extracted from the initial search, and then full-text articles were obtained for detailed evaluation. Finally, we read the full text of articles and recognized potentially eligible studies to be included in the systematic review.
The following criteria were considered as the inclusion criteria:
(1) Type of a study: Original or review research papers were selected, and newspapers, reports, editorial, letters, posters, and conference papers were not examined.
(2) Date of publication: Papers published between 1 January 2020 to 20 December 2020
(3) Language: English language
(4) Text availability: Full-text papers with the keywords in the title or abstracts
(5) Content analysis: At least two of the following reporting parameters: (1) basic/general, (2) clinical, (3) para-clinical, (4) geo-locational, and (5) contact/exposure data classes.
Finally, the probable data elements to be included in the COV-CT-MDS were recorded in a checklist with two administrative and clinical sections.
3.1.3. Data Extraction
For each eligible research, the following information was extracted based on a designed data extraction form, which included the first author, country, year of publication, study design, and reporting data classes in the two non-clinical and clinical data categories. The results were organized under the following categories: (1) data categories, (2) data classes, (3) data fields, and (4) data features and attributes.
3.2. Delphi Technique
3.2.1. Questionnaire Design
After conducting the necessary literature review and receiving expert advice, we developed a questionnaire. We invited 20 experts, including five infectious diseases specialists, five virologists, five health information management (HIM), and five clinical epidemiologists, in a two-round Delphi survey. The questionnaire included the following parts: (1) demographical data, (2) clinical finding, (3) geolocation location, (4) relocation data, and (5) contact/ exposure data.
3.2.2. Data Analysis
The experts participating in the study were asked to score the tabulated data elements in terms of their importance using a five-point Likert scale (ranging from 1: “very slightly important” to 5: “highly important”). Data fields with less than 50% agreement were excluded in the first round, while those with greater than 75% agreement were included in the primary round. Those with 50% to 75% agreement were surveyed in the second round, and if there was 75% consensus over a subject, it was regarded as a final data field.
4. Results
4.1. Characteristics of Included Studies
A total of 388 articles were retrieved from the literature search. After the removal of duplicate articles and those not meeting the inclusion criteria, 24 articles that satisfied all the inclusion criteria were included in the analysis. Figure 1 summarizes the selection process (PRISMA chart).
PRISMA chart for the study selection process
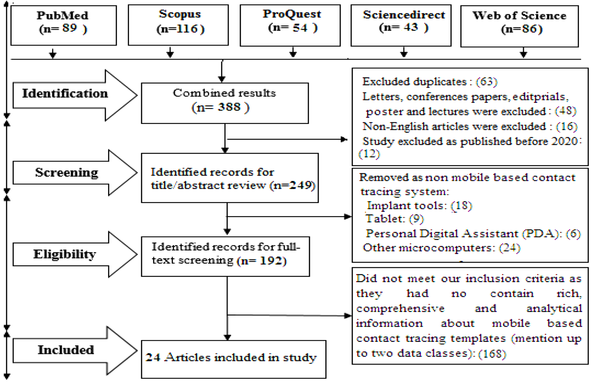
4.2. Identifying the Proposed Data Field
The proposed data fields after the literature review were divided into administrative and clinical data sections, nine data classes, and 198 data fields (Table 1).
Summary of Characteristics of Included Studies in the Systematic Review
First Author (2020) | Method | Data Classes | |||||||||
---|---|---|---|---|---|---|---|---|---|---|---|
Administrative | Clinical | ||||||||||
Basic | Geolocation | Occupational | Relocation | Contact | Exposure | Clinical | Manifestations | Vital Signs | Referral | ||
Bassi et al. (17) | Descriptive | * | * | * | |||||||
Basu (18) | Case study | * | * | * | |||||||
Davalbhakta et al. (19) | Review | * | * | * | * | ||||||
Ekong et al. (20) | Exploratory review | * | * | * | * | ||||||
Hassandoust et al. (21) | Developmental | * | * | * | * | * | |||||
Martin et al. (5) | Review | * | * | ||||||||
Parker et al. (22) | Descriptive | * | * | * | |||||||
Rahman et al. (23) | Case study | * | * | ||||||||
Shubina et al. (24) | Retrospective | * | * | ||||||||
Vuokko et al. (25) | Descriptive | * | * | * | |||||||
Prabu et al. (26) | Exploratory review | * | * | * | |||||||
Teixeira and Doetsch(27) | Descriptive | * | * | * | |||||||
Kondylakis et al. (28) | Review | * | * | * | * | ||||||
Nakamoto et al. (29) | Developmental | * | * | * | * | ||||||
Altmann et al. (30) | Retrospective | * | * | ||||||||
Dar et al. (31) | Developmental | * | * | * | |||||||
Singh et al. (32) | Review | * | * | ||||||||
Urbaczewski and Lee(33) | Retrospective | * | * | * | * | ||||||
Whaiduzzaman et al. (34) | Developmental | * | * | * | * | * | |||||
Bianconi et al. (35) | Descriptive | * | * | * | * | ||||||
Grantz et al. (36) | Prospective | * | |||||||||
Ming et al. (9) | Retrospective | * | * | * | * | * | |||||
Wirth et al. (37) | Scoping review | * | * | * | * | ||||||
Nijsingh et al. (10) | Descriptive | * | * | * |
Several data fields were excluded after the second round of Delphi. Thus, of the 198 proposed data fields, 117 fields were excluded from the study, and 81 data fields were finalized (Table 2).
Consensus Thresholds
Decision | Agreement Rate (%) | Frequency |
---|---|---|
First Round | ||
Inclusion | < 75 | 58 |
Exclusion | > 50 | 92 |
Entering in second round | 50 - 75 | 48 |
Second Round | ||
Inclusion | < 75 | 25 |
Exclusion | > 75 | 23 |
The final reporting template is composed of two data sections, nine data classes, and 81 data fields. Table 3 lists the data sections, classes, fields, their formats and values, and corresponding reference SNOMED-CT codes.
Required Data Elements for Contact Tracing
Data Element | Feature Content | Feature Format | SNOMED-CT Category | SNOMED-CT Codes |
---|---|---|---|---|
General Characteristics | ||||
Full name (11-16, 34, 36, 38, 39) | String | Observable entity | 371484003 | |
Age (5-9, 12, 14, 18, 34, 38, 40, 41) | Forced choice | Qualifier value | 764868004 | |
Gender (2, 4, 9, 10, 12, 15, 21, 36, 40, 41) | M: 1 F: 0 | Binary | Clinical finding | 703118005 |
National ID number (4, 6, 9, 13, 18, 34, 40) | xxx- xxxxxx-x | Numerical | Observable entity | 422549004 |
Citizenship (2, 15, 18, 21, 34, 36, 39, 41) | Iranian; Non-Iranian | Binary | Social concept | 275595001 |
Medical record number (4, 5, 7, 12, 13, 16, 18, 21, 31, 40) | xx-xx-xx | Numerical | Observable entity | 398225001 |
Level of education (5, 10, 12, 13, 15, 18, 36, 39, 41) | Primary; Secondary; Tertiary | Forced choice | Observable entity | 224300008 |
Marital status (5, 7, 9, 12, 13, 16, 18, 34, 36, 39, 40) | Single; Married; Widow; Other | Forced choice | Clinical finding | 87915002 |
Monthly income (3, 5, 6, 9, 13, 16, 21, 34, 36, 39, 41) | Low: < 120$; Medium: 120$ - 250$; High: > 250$ | Forced choice | Clinical finding | 424860001 |
Family relationship to index cases (5, 6, 9, 15, 34) | Nuclear family; Extended family | Binary | Social concept | 394568007 |
Phone number (4-6, 9, 13, 15, 16, 40) | +98 xxx xxx xxxx | Numerical | Observable entity | 398198004 |
Healthcare facility unique ID (5, 6, 15, 18, 21, 40) | xxxxx | Numerical | Observable entity | 713578002 |
Frontline health worker ID (4-6, 9, 10, 13, 15, 16, 36) | xxxxx | Numerical | Observable entity | 713578002 |
Relationship with the source case (5, 6, 12, 14, 15, 18, 21, 34, 40, 41) | Partner / spouse; Family member; Other | Forced choice | Clinical finding | 852071000000103 |
Geolocation Data | ||||
Place of birth (6, 14, 15, 18, 21, 40, 41) | Geographical location: Province, city, village | String | Environment/ location | 315446000 |
Resident situation (5, 6, 8, 15, 40) | Tenant; Owner; Other | Forced choice | Environment/ location | 184097001 |
Residential address (3, 4, 6, 8, 9, 14, 36, 40) | String | Observable entity | 433178008 | |
Postal code / zip code (3, 6, 8-10, 15, 36, 41) | xxxxx-xxxxx | Numerical | observable entity | 184097001 |
Place of contact (3-6, 10, 11, 14-16, 36, 41) | Workplace; Home; Public place; Other; Unknown | Forced choice | Environment/ location | 257710009 |
Location case identified (4, 6, 11, 13, 15, 21, 40) | Geographical location | String | Environment/ location | 706956001 |
Origin of travel (5, 6, 15, 16, 34, 41) | Geographical location | String | Environment/ location | 224803003 |
Travel destination (6, 10, 13, 16, 36, 39) | Geographical location | String | Environment/ location | 224807002 |
Address of healthcare organization (3, 6, 13, 21, 39-41) | String | Observable entity | 184097001 | |
Isolation/quarantine location (6, 13, 16, 40) | Self-isolation at home; Hospital; Long term care facilities; Other | Forced choice | Procedure | 1321131000000109 |
Clinical Characteristic | ||||
Symptom incidence (3, 4, 6, 7, 10, 12, 16, 31, 39, 40) | Asymptomatic; Pre symptomatic | Forced choice | Qualifier value | 264931009 |
Date of symptom onset (6, 9, 12, 13, 15, 31, 36, 39, 40) | yyyy /mm/ dd | Integer | Observable entity | 520191000000103 |
Days from exposure to symptom onset (8-10, 12, 13, 15, 36, 38, 39) | xx | Numerical | Qualifier value | 307474000 |
Days from illness onset to first admission (5, 6, 10, 12, 15, 18, 38) | xx | Numerical | Qualifier value | 307474000 |
Days from diagnosis to treatment (8, 12, 14, 40) | xx | Numerical | Qualifier value | 432213005 |
Date of diagnosis (10, 12, 14, 18, 40, 41) | yyyy /mm/ dd | Integer | Observable entity | 432213005 |
Covid-19 classification (10, 12, 18) | Confirmed; Probable; Unknown | Forced choice | Situation | 395098000 |
Covid-19 status (9, 14, 18) | Active; Inactive; Recovered | Forced choice | Clinical finding | 110278006 |
Case finding approaches | Random screening; Symptomatic case referral; Contact tracing; Other | Forced choice | Clinical finding | Country, Province/ State, City, |
Prior hospitalization (3, 5, 9, 10, 12, 14, 34, 38) | Yes; No | Numerical | Clinical finding | 314503007 |
Self Reported Clinical Manifestation | ||||
Fever/chill (4-7, 10, 13, 18, 21, 31, 40) | Yes; No | Binary | Qualifier value | 14732006 |
Cough (4, 6, 9, 10, 13, 18, 41) | Yes; No | Binary | Clinical finding | 314503007 |
Dyspnea (6, 10, 12, 14, 18, 31, 38, 39) | Yes; No | Binary | Qualifier value | 385432009 |
Respiratory distress (10, 12, 21, 38, 39) | Yes; No | Binary | Clinical finding | 386661006 |
Myalgia (9, 12, 18, 38) | Yes; No | Binary | Clinical finding | 36523521 |
Headache (10, 14, 18, 38, 39) | Yes; No | Binary | Clinical finding | 43724002 |
Nausea/ vomiting (4, 9, 14, 18, 21, 36) | Yes; No | Binary | Clinical finding | 65124004 |
GI symptoms (4, 10, 15, 16, 39, 40) | Yes; No | Binary | Clinical finding | 664563201 |
Anosmia (12, 16, 21, 34) | Yes; No | Binary | Situation | 162298006 |
Runny nose (12, 13, 15, 34, 39, 41) | Yes; No | Binary | Situation | 162062008 |
Sore throat (4, 12, 13, 16, 21, 34, 40, 41) | Yes; No | Binary | Situation | 162104009 |
Unexpected fatigue (12, 13, 15, 16, 40) | Yes; No | Binary | Clinical finding | 93559003 |
Real-Time Vital Sign Monitoring | ||||
Oxygen saturation (SO2) (13, 18, 34) | 75 < mmHg; 75 – 100 mmHg; 100 > mmHg | Forced choice | Clinical finding | 448225001 |
Heart rate (bit per minute) (10, 12, 13, 18, 34, 36, 41) | < 60 bps; 60-100 bps; > 100 bps | Forced choice | Clinical finding | 76863003 |
Blood pressure (mmHg) (10, 12, 14, 16, 31, 39) | < 120; 120-139; > 140 | Forced choice | Clinical finding | 2004005 |
Body temperature (°C) (2, 3, 12-14, 16, 21) | < 37.3; 37.3 – 39; > 39.0 | Forced choice | Clinical finding | 50177009 |
Respiratory rate (breaths per min) (2, 3, 12, 16, 21) | ≤ 24; > 24 | Forced choice | Clinical finding | 289100008 |
Occupational Criteria | ||||
Employment status (5, 7, 8, 18, 34, 41) | Unemployed; Employed | Forced choice | Clinical finding | 224363007 |
Working status (7, 16) | Full time; Part time | Forced choice | Clinical finding | 160903007 |
If employed, occupation risks (3, 8, 13, 31, 34, 38, 39) | High risk; Medium risk; Low risk | Forced choice | Event | 16090731000119102 |
Work situation during general quarantine (7, 16, 34, 38) | Not working; Working at usual place; Teleworking; Other | Forced choice | Clinical finding | 302201002 |
Work in a patient care setting (3-5, 7, 9, 13, 16, 21, 39-41) | Yes; No | Binary | Clinical finding | 302201002 |
Attending work at the time of symptom occur (4, 9, 10, 14, 34, 40) | Yes; No | Binary | Clinical finding | 83408003 |
Travel/Relocation Data | ||||
Recent travel / relocation (4, 6, 8, 10, 13, 15, 18, 34, 36, 40) | Yes; No | Binary | Situation | 473087005 |
Reason for travel (6, 9, 15, 36, 41) | Holiday business; Pilgrimage other | Forced choice | clinical finding | 161091009 |
Travel type (4, 6, 8, 18) | Domestic travel; Foreign travel | Binary | Observable entity | 441969007 |
Date of departure (3-6, 8, 9, 14, 34, 40) | dd/mm/yy | Integer | Observable entity | 810811000000107 |
Number of travels in the last 7 days (5, 6, 8, 9, 11, 16, 36, 40) | None; One - two times a week; Two - four times a week; More than five times a week | Forced choice | Qualifier value | 259083004 |
Travel to epidemic places (2, 5, 7, 9, 18, 21, 36, 40) | Yes; No | Binary | Clinical finding | 506931000000109 |
Relocation / transfer method (3, 5, 9-11, 13, 16, 36) | Public transportation; Personal transportation | Binary | Procedure | 715957006 |
Duration of travel (3, 7, 13) | Daily travel (1 day <); 1 day ≥ | Binary | Qualifier value | 69620002 |
Contact Tracing Data | ||||
Prior contact tracing experience (10, 15-18, 22, 25) | Yes; No | Binary | Procedure; | 225368008 |
If yes, prior contact tracing approach (2, 3, 7, 13) | Conventional; Automatic | Binary | Clinical finding; | 52669001 |
If Automatic, contact tracing technology (2, 3, 5-11, 14, 18, 31, 34, 40, 41) | Mobile phone; Implant tools other microcomputers | Forced choice | Qualifier value | 723991000000105 |
Contact tracer ID (3, 5, 6, 9, 11, 14, 34, 38, 39) | XXXX | Forced choice | Qualifier value | 118522005 |
Notification ID (3, 5, 6, 9, 10, 34, 39) | XXX /XXXX -X | Forced choice | Observable entity | 895571000000108 |
Contact Data | ||||
Contact type (4, 10, 12, 13, 18, 36, 38) | Primary: Person-to-person; Secondary: Person-to-surface / animal | Binary | Social concept | 70862002 |
Contact category (2-4, 14, 21, 31) | No contact; Family members; Social contact; other | Forced choice | Clinical finding | 381441000000103 |
Contact risk level (13, 15, 34, 36, 41) | Living with an infected/suspected case in the past 14 days; Prolonged direct contact in the past 14 days; Casual and indirect contact in the past 14 days; Not in contact | Forced choice | Situation | 76906009 |
Contact with care facility | Yes; No | Binary | Situation | 136569214 |
Contact frequency (3, 8, 16, 18) | Sometimes: ≥ 2 times a day; Always: 2 - 4 times a day; Repeatedly: < 4 times a day | Forced choice | Qualifier value | 735269004 |
Contact list (person) (7, 13, 34) | 5 >; 5 - 10; 10 - 30; 30 < | Forced choice | Social context | 125676002 |
Minimal distance of contact (meters) (2-4, 14, 21, 36, 41) | 2 >; 2 < | Binary | Qualifier value | 421669002 |
Date of last contact (6, 12, 14, 16, 18, 21, 36, 41) | yyyy /mm/ dd | Integer | ||
Time between contact and diagnosis (10, 15, 16, 18, 41) | Numerical | Qualifier value | 305698526 | |
Total duration of contact (minutes) (3, 7, 8, 13, 34, 36) | ≥ 15; < 15 | Binary | Qualifier value | 356624006 |
Total duration of contact (day) (3, 7, 8, 13, 34, 36) | ≥ 14; < 14 | Binary | Qualifier value | 258703001 |
5. Discussion
Contact tracing is known as a crucial surveillance measure in avoiding the spread of epidemic diseases such as the current COVID-19. During this epidemic, contact tracing data should be integrated across healthcare data collection systems at the national level (34). However, data are gathered from stand-alone recording and reporting systems largely manually generated via the contact tracing process. Data collection is a crucial strategic preparation measure for governments and health officials battling the COVID-19 epidemic (36).
The CoV-CT-MDS is a promising tool to meet some of the data necessary for epidemiology contact tracing leading to a validated template for the documentation of active case finding for public health practice and research purposes. Determining a core data set or MDS from a scientific perspective and according to the actual demands of users is the most central prerequisite for the design and development of any information system or app in the healthcare industry (38). It can be advantageous for designers and vendors of health information systems to simplify and accelerate the development of such systems and reduce the possibility of their failure (39). From this point of view, in this study, the CoV-CT-MDS can be used as a basis for the effective collection and management of data related to COVID-19 contact tracing using related information systems or apps.
In the initial months of the pandemic, contact tracing measures were recorded through manual data collection tools (eg, in Excel sheets, spreadsheet), which was a time-intensive, resource-demanding, and error-prone process (18, 40). Additionally, the conventional approaches did not always offer inclusive data about the number of investigated contacts, the nature of the relationship between cases and contacts, the number of contacts, who in turn, become cases, and the first and last days of follow-up surveillance (21, 41). To cope with these issues, it is essential to develop a contact tracing system that enables standardized data recording and accelerates the surveillance of contacts and outbreak paths (31, 42). This system allows intervallic analyses for the creation of standard reports and offers detailed epidemiological analysis for the identification of high-risk exposures and targeting of contact tracing efforts (21, 41, 43).
Implementing an active and responsive contact tracing strategy would be a valuable containing measure for avoiding the transmission of COVID-19. In this context, mobile technology enabling self-reports and smartphone applications for virtual contact tracing could be used to control disease outbreak and detect as well as quarantine COVID-19 cases and those who may have been exposed to the virus (44). For this purpose, a contact tracing system including timely and accurate data collection process and a unified case reporting template are proposed to guide healthcare authorities for proper interventions (20, 32, 36). There is, therefore, a pressing need for a unified data collection template to swiftly and prospectively collect high-quality data related to recent exposure and mobility patterns of confirmed and suspected individuals (45, 46).
The novelty of COVID-19 with frequent mutations of the virus demands numerous and unknown aspects to be investigated in prospective studies, and thus, studies related to COVID-19 contact tracing are limited at the time of writing this article (Decembers 2020). Hence, the main limitation of this living systematic review is the scarcity of available related resources and lack of data enrichment. Review of only English-language articles is another limitation of the study. However, multiple scientific databases were broadly reviewed. Future modifications, along with a Delphi survey is recommended to augment the COV-CT-MDS.
5.1. Conclusions
An effective COVID-19 contact tracing system requires reliable and timely information to guide fully informed decisions to contain the further spread of the disease by taking early preventive actions. For developing the CoV-CT-MDS, we performed an extensive literature review and expert view to identify the proposed contact tracing data fields and corresponding variables from an evidence-based perspective. The COV-CT-MDS as a unified data collection tool is the first step for developing a mobile-based contact tracing system. This template can provide valuable information for clinicians, health policymakers, and researchers for integrating the COVID-19 contact tracing efforts across Iran’s healthcare system. Given the prominence of reliable, accurate, and comprehensive data on COVID-19 surveillance measures, it is suggested that different countries design and implement a comprehensive national MDS for COVID-19 contact tracing.
References
-
1.
Gupta R, Bedi M, Goyal P, Wadhera S, Verma V. Analysis of COVID-19 tracking tool in India: Case study of Aarogya Setu mobile application. Digital government: Research and practice. Digi Govt Res Pract. 2020;1(4):1-8. https://doi.org/10.1145/3416088.
-
2.
World Health Organization. A systematic approach to monitoring and analysing public health and social measures (PHSM) in the context of the COVID-19 pandemic: underlying methodology and application of the PHSM database and PHSM Severity Index. World Health Organization. Regional Office for Europe; 2020.
-
3.
Chu DK, Akl EA, Duda S, Solo K, Yaacoub S, Schunemann H. Physical distancing, face masks, and eye protection to prevent person-to-person transmission of SARS-CoV-2 and COVID-19: A systematic review and meta-analysis. Lancet. 2020;395(10242):1973-87. https://doi.org/10.1016/j.jvs.2020.07.040.
-
4.
Hellewell J, Abbott S, Gimma A, Bosse NI, Jarvis CI, Russell TW, et al. Feasibility of controlling COVID-19 outbreaks by isolation of cases and contacts. Lancet Glob Health. 2020. https://doi.org/10.1101/2020.02.08.20021162.
-
5.
Martin T, Karopoulos G, Hernández-Ramos JL, Kambourakis G, Nai Fovino I, Choo KR. Demystifying COVID-19 digital contact tracing: A survey on frameworks and mobile apps. Wirel Commun Mob Comput. 2020;2020:1-29. https://doi.org/10.1155/2020/8851429.
-
6.
Yamamoto K, Takahashi T, Urasaki M, Nagayasu Y, Shimamoto T, Tateyama Y, et al. Health observation app for COVID-19 symptom tracking integrated with personal health records: proof of concept and practical use study. JMIR mHealth uHealth. 2020;8(7). https://doi.org/10.2196/19902.
-
7.
Ferrari A, Santus E, Cirillo D, Ponce-de-Leon M, Marino N, Ferretti MT, et al. Reproducing SARS-CoV-2 epidemics by region-specific variables and modeling contact tracing App containment. medRxiv. 2020. https://doi.org/10.1101/2020.05.14.20101675.
-
8.
Barrat A, Cattuto C, Kivelä M, Lehmann S, Saramäki J. Effect of manual and digital contact tracing on COVID-19 outbreaks: a study on empirical contact data. medRxiv. 2020. https://doi.org/10.1101/2020.07.24.20159947.
-
9.
Ming LC, Untong N, Aliudin NA, Osili N, Kifli N, Tan CS, et al. Mobile health apps on COVID-19 launched in the early days of the pandemic: Content analysis and review. JMIR mHealth uHealth. 2020;8(9). https://doi.org/10.2196/19796.
-
10.
Nijsingh N, van Bergen A, Wild V. Applying a precautionary approach to mobile contact tracing for COVID-19: The value of reversibility. J Bioeth Inq. 2020;17(4):823-7. https://doi.org/10.1007/s11673-020-10004-z.
-
11.
Yasaka TM, Lehrich BM, Sahyouni R. Peer-to-peer contact tracing: Development of a privacy-preserving smartphone app. JMIR mHealth uHealth. 2020;8(4). https://doi.org/10.2196/18936.
-
12.
Trang S, Trenz M, Weiger WH, Tarafdar M, Cheung CM. One app to trace them all? Examining app specifications for mass acceptance of contact-tracing apps. Eur J Inf Syst. 2020;29(4):415-28. https://doi.org/10.1080/0960085x.2020.1784046.
-
13.
Peddireddy AS, Xie D, Patil P, Wilson ML, Machi D, Venkatramanan S, et al. From 5Vs to 6Cs: Operationalizing epidemic data management with COVID-19 surveillance. medRxiv. 2020. https://doi.org/10.1101/2020.10.27.20220830.
-
14.
Saha S, Prakash P, Singhal S, Chauhan S, Singhal M, Chandrashekar S. Mobile app-based contact tracing during COVID-19—simple and without recall bias. Indian J Surg. 2021;83(1):390-2. https://doi.org/10.1007/s12262-021-02735-z.
-
15.
Nwawudu E, Ikwu A, Ikwu U, Oparah N, Nnorom S. Proposed design of a real-time COVID-19 pandemic contact tracing using mobile phone. Eur Sci J. 2020;16(36). https://doi.org/10.19044/esj.2020.v16n36p1.
-
16.
Moher D, Liberati A, Tetzlaff J, Altman DG. Preferred reporting items for systematic reviews and meta-analyses: The PRISMA statement. Int J Surg. 2010;8(5):336-41. https://doi.org/10.1016/j.ijsu.2010.02.007.
-
17.
Bassi A, Arfin S, John O, Jha V. An overview of mobile applications (apps) to support the coronavirus disease 2019 response in India. Indian J Med Res. 2020;151(5). https://doi.org/10.4103/ijmr.IJMR_1200_20.
-
18.
Basu S. Effective contact tracing for COVID-19 using mobile phones: an ethical analysis of the mandatory use of the Aarogya Setu application in India. Camb Q Healthc Ethics. 2020;30(2):262-71. https://doi.org/10.1017/s0963180120000821.
-
19.
Davalbhakta S, Advani S, Kumar S, Agarwal V, Bhoyar S, Fedirko E, et al. A systematic review of smartphone applications available for corona virus disease 2019 (COVID-19) and the assessment of their quality using the mobile application rating scale (MARS). J Med Sys. 2020;44(9). https://doi.org/10.1007/s10916-020-01633-3.
-
20.
Ekong I, Chukwu E, Chukwu M. COVID-19 mobile positioning data contact tracing and patient privacy regulations: exploratory search of global response strategies and the use of digital tools in Nigeria. JMIR mHealth uHealth. 2020;8(4). https://doi.org/10.2196/19139.
-
21.
Hassandoust F, Akhlaghpour S, Johnston AC. Individuals’ privacy concerns and adoption of contact tracing mobile applications in a pandemic: A situational privacy calculus perspective. J Am Med Inform Assoc. 2021;28(3):463-71. https://doi.org/10.1093/jamia/ocaa240.
-
22.
Parker MJ, Fraser C, Abeler-Dörner L, Bonsall D. Ethics of instantaneous contact tracing using mobile phone apps in the control of the COVID-19 pandemic. J Med Ethics. 2020;46(7):427-31. https://doi.org/10.1136/medethics-2020-106314.
-
23.
Rahman MT, Khan RT, Khandaker MR, Sellathurai M, Salan MSA. An automated contact tracing approach for controlling COVID-19 spread based on geolocation data from mobile cellular networks. IEEE Access. 2020;8:213554-65. https://doi.org/10.1109/access.2020.3040198.
-
24.
Shubina V, Holcer S, Gould M, Lohan ES. Survey of decentralized solutions with mobile devices for user location tracking, proximity detection, and contact tracing in the COVID-19 era. Data. 2020;5(4):87. https://doi.org/10.3390/data5040087.
-
25.
Vuokko R, Saranto K, Palojoki S. Typology-based analysis of COVID-19 mobile applications: implications for patient empowerment. Stud Health Technol Inform. 2020;275:212-6. https://doi.org/10.3233/shti200725.
-
26.
Prabu S, Velan B, Jayasudha FV, Visu P, Janarthanan K. Mobile technologies for contact tracing and prevention of COVID-19 positive cases: a cross-sectional study. Int J Pervasive Comput Commun. 2020.
-
27.
Teixeira R, Doetsch J. The multifaceted role of mobile technologies as a strategy to combat COVID-19 pandemic. Epidemiol Infect. 2020;148. https://doi.org/10.1017/s0950268820002435.
-
28.
Kondylakis H, Katehakis DG, Kouroubali A, Logothetidis F, Triantafyllidis A, Kalamaras I, et al. COVID-19 mobile apps: A systematic review of the literature. J Med Internet Res. 2020;22(12). https://doi.org/10.2196/23170.
-
29.
Nakamoto I, Jiang M, Zhang J, Zhuang W, Guo Y, Jin M, et al. Evaluation of the design and implementation of a peer-to-peer COVID-19 contact tracing mobile app (COCOA) in Japan. JMIR mHealth uHealth. 2020;8(12). e22098. https://doi.org/10.2196/22098.
-
30.
Altmann S, Milsom L, Zillessen H, Blasone R, Gerdon F, Bach R, et al. Acceptability of App-Based Contact Tracing for COVID-19: Cross-Country Survey Study. JMIR mHealth uHealth. 2020;8(8). e19857. https://doi.org/10.2196/19857.
-
31.
Dar AB, Lone AH, Zahoor S, Khan AA, Naaz R. Applicability of mobile contact tracing in fighting pandemic (COVID-19): Issues, challenges and solutions. Comput Sci Rev. 2020;38. https://doi.org/10.1016/j.cosrev.2020.100307.
-
32.
Singh HJL, Couch D, Yap K. Mobile health apps that help with COVID-19 management: scoping review. JMIR Nursing. 2020;3(1). https://doi.org/10.2196/20596.
-
33.
Urbaczewski A, Lee YJ. Information technology and the pandemic: A preliminary multinational analysis of the impact of mobile tracking technology on the COVID-19 contagion control. Eur J Inf Syst. 2020;29(4):405-14. https://doi.org/10.1080/0960085x.2020.1802358.
-
34.
Whaiduzzaman M, Hossain MR, Shovon AR, Roy S, Laszka A, Buyya R, et al. A privacy-preserving mobile and fog computing framework to trace and prevent COVID-19 community transmission. J Biomed Health Inform. 2020;24(12):3564-75. https://doi.org/10.1109/jbhi.2020.3026060.
-
35.
Bianconi A, Marcelli A, Campi G, Perali A. Efficiency of COVID-19 mobile contact tracing containment by measuring time-dependent doubling time. Phys Biol. 2020;17(6). https://doi.org/10.1088/1478-3975/abac51.
-
36.
Grantz KH, Meredith HR, Cummings DA, Metcalf CE, Grenfell BT, Giles JR, et al. The use of mobile phone data to inform analysis of COVID-19 pandemic epidemiology. Nat Commun. 2020;11(1). https://doi.org/10.1038/s41467-020-18190-5.
-
37.
Wirth FN, Johns M, Meurers T, Prasser F. Citizen-centered mobile health apps collecting individual-level spatial data for infectious disease management: scoping review. JMIR mHealth uHealth. 2020;8(11). https://doi.org/10.2196/22594.
-
38.
Shanbehzadeh M, Kazemi-Arpanahi H. Development of minimal basic data set to report COVID-19. Med J IR Iran. 2020. https://doi.org/10.47176/mjiri.34.111.
-
39.
Hashemi N, Sheikhtaheri A, Hashemi N, Rawassizadeh R. Electronic medical records for mental disorders: What data elements should these systems contain? dHealth. 2019:25-32.
-
40.
Levine R, Ghiselli M, Conteh A, Turay B, Kemoh A, Sesay F, et al. Notes from the field: Development of a contact tracing system for Ebola virus disease — Kambia district, Sierra Leone, January–February 2015. MMWR Morb Mortal Wkly Rep. 2016;65(15). https://doi.org/10.15585/mmwr.mm6515a4.
-
41.
Harper EM. Engineering a learning healthcare system: Using health information technology to develop an objective nurse staffing tool. NI 2012: 11th International congress on nursing informatics. June 23-27, 2012; Montreal, Canada. American Medical Informatics Association; 2012.
-
42.
Ding EY, Albuquerque D, Winter M, Binici S, Piche J, Bashar SK, et al. Novel method of atrial fibrillation case identification and burden estimation using the MIMIC-III electronic health data set. J Intensive Care Med. 2019;34(10):851-7. https://doi.org/10.1177/0885066619866172.
-
43.
Fateh-Moghadam P, Battisti L, Molinaro S, Fontanari S, Dallago G, Binkin N, et al. Contact tracing during phase I of the COVID-19 pandemic in the Province of Trento, Italy: Key findings and recommendations. medRxiv. 2020. https://doi.org/10.1101/2020.07.16.20127357.
-
44.
Adans-Dester CP, Bamberg S, Bertacchi FP, Caulfield B, Chappie K, Demarchi D, et al. Can mHealth technology help mitigate the effects of the COVID-19 pandemic? IEEE Open J Eng Med Biol. 2020;1:243-8. https://doi.org/10.1109/ojemb.2020.3015141.
-
45.
Drew DA, Nguyen LH, Steves CJ, Wolf J, Spector TD, Chan AT. Rapid implementation of mobile technology for real-time epidemiology of COVID-19. Science. 2020. https://doi.org/10.1101/2020.04.02.20051334.
-
46.
Ahmed K, Bukhari MA, Mlanda T, Kimenyi JP, Wallace P, Lukoya CO, et al. Novel approach to support rapid data collection, management, and visualization during the COVID-19 outbreak response in the world health organization African region: Development of a data summarization and visualization tool. JMIR public health Surve. 2020;6(4). e20355.