Abstract
Background:
The precise differentiation of schizophrenic patients with positive and negative symptoms is still challenging; hence, psychiatrists mainly focus on diagnosing schizophrenic patients with positive symptoms. However, schizophrenic patients with negative symptoms have revealed remarkably poor outcomes.Objectives:
This study aimed to differentiate schizophrenic patients with positive and negative dominant symptoms quantitatively by classifying their electroencephalography (EEG) features.Methods:
In this study, 36 patients with schizophrenia and 26 age-matched control subjects voluntarily participated. Their EEG signals were captured and characterized by elicited multiscale entropy to decode the amount of irregularities occurred in each EEG channel. The principal component analysis (PCA) was deployed to decrease the dimension of elicited features, and the reduced features were applied to three Gaussian Naïve Bayes classifiers, each of which was trained for a specific class.Results:
The classification of the three groups resulted in 77.86% accuracy, while this accuracy in the schizophrenic groups provided 65% accuracy. In the resting state, the normal and schizophrenic subjects were differentiated by a high rate (95.43%).Conclusions:
Exploiting information-theoretic features of the EEG signals over the scalp and automatic classification of these features, we can well-differentiate schizophrenic patients with different dominant symptoms. Moreover, better classification results can be achieved by passing the EEG features through PCA.Keywords
Classification Entropy EEG Schizophrenia Positive and Negative Symptoms
1. Background
Schizophrenia is a chronic and severe mental illness leading to a wide range of symptoms such as delusions, hallucinations, disorganized speech, thinking problems, and demotivation (1). Schizophrenia usually affects 1% of the general population and is traditionally diagnosed by DSM-V criteria (2). Schizophrenia is often categorized based on its dominant symptoms, including positive and negative symptoms. Positive symptoms include hallucination, delusion, and conceptual disorganization, while negative symptoms are apathy, affective blunting, and poverty of speech (3). These symptoms can be evaluated in different ways, including clinical interviews with expert psychiatrists or psychological tests such as PANSS (4). The diagnosis of schizophrenia based on dominant symptoms plays an important role in differential diagnosis, treatment plan, the selection of best medication by a doctor, and the prognosis of the disease. Although schizophrenic patients may have positive or negative symptoms, some patients have both types of symptoms, which one of them being more dominant. The diagnosis of schizophrenic patients with dominant positive symptoms is easier than the diagnosis of those with negative symptoms. The main challenge in diagnosing schizophrenic patients with negative symptoms is the similarity of their signs and symptoms with other psychiatric diseases, including major depressive disorder (MDD) and schizoid disorder personality (5). Moreover, patients with dominant-negative symptoms tend to be associated with remarkably poor outcomes and inaccurate prognosis. Accordingly, the precise categorization of schizophrenic patients based on their dominant symptoms is still challenging for psychiatrists (6). To overcome this drawback, it is better to find a quantitative biomarker for a better diagnosis of this complicated disease. Different aspects of schizophrenic patients with positive and negative syndromes have already been studied. In schizophrenic patients with both symptoms, a few brain functions and cerebrospinal fluid flow are affected and show abnormal behavior. In addition, brain structural change and deterioration in their genetics are observed (7). To monitor the electrical and functional activities of the brain, several types of equipment, including electroencephalography (EEG) systems, functional magnetic resonance imaging (fMRI), and positron emission tomography (PET), have been developed. Note that schizophrenia and its subtypes have been studied with these signals and imaging modalities (8, 9). Recent progress in neuroimaging modalities enables specialists to better understand the different aspects of various brain diseases. Structural abnormalities were also studied in schizophrenia with different types of symptomatology and revealed lower volume in frontal and temporal lobes (10).
Electroencephalography is a quantitative and physiological-based tool with a high temporal resolution and has become an important modality in studying and diagnosing various brain disorders. Since the EEG signal acquisition is cheap and has no side effects, researchers have paid attention to using this physiology-based signal to reveal different abnormalities in the brain. A few studies have been conducted to differentiate schizophrenic patients with positive and negative symptoms by analyzing their EEG signals (11-13).
Temporal synchronization and the oscillation behavior of neurons in the EEG signals are critical factors in cognition and perception tasks, both of which are impaired in schizophrenic patients. These impairments lead to abnormal EEG behaviors. However, the relationship between the gate sensory deficit and schizophrenia symptoms is still ambiguous. Keil et al. (11) recorded the EEG signals of 22 schizophrenic patients (with an equal number of dominant symptoms) and 22 age-matched controls in the presence of an auditory stimulus. They observed an increase in the Gamma-band power sensory gating in schizophrenic patients with positive symptoms. In contrast, alpha-band phase synchrony sensory gating was decreased in schizophrenic patients with negative symptoms.
Fan et al. (12) asked a group of hospitalized schizophrenic patients to do a cognitive task to compare their cognitive ability with that of the healthy subjects. The results of their test illustrated some features, such as the amplitude of EEGs and the frequency distribution of EEGs, which can guide specialists to classify schizophrenic patients with different dominant symptoms more accurately. In another study, Saletu et al. (13) assessed the brain maps of 48 schizophrenic patients and showed a severe drop and incline in their Alpha and Beta bands, respectively, compared to healthy subjects. Moreover, they compared the EEG variations of schizophrenic patients with negative and positive symptoms and observed an increase in the low-frequency EEG bands (e.g., delta/theta) of patients with dominant-negative symptoms while observed a drop in the activity of the Alpha band and an increase in the Beta band in schizophrenic patients with dominant positive symptoms. A review of the literature reveals that a few studies have aimed to discriminate schizophrenic patients with different dominant symptoms, some of which have traced the increase or decrease in the standard EEG band powers in classifying schizophrenic patients by their dominant symptoms.
As EEG signals behave irregularly and obey no specific pattern, information-theoretic measures are adopted to decode the EEG variations and reveal their content. The domain of information theory is vast and contains several issues such as coding and decoding different types of data (e.g., text, image, and video) (14) and measuring, roughness, and complexity captured in different types of data, including EEG signals, MRI images, data stream, micro-array sequences, and radio signals. One of the main measures in information theory is entropy. In this regard, several methods have been proposed to measure entropy because the value of entropy directly relates to the amount of information included in data. The well-known entropy measures are approximate entropy (ApEn), Kolmogorov entropy (15), Shannon entropy (in the time domain), spectral entropy, and multiscale entropy (MSE) (16). In a study, Shannon entropy was applied to the fMRI images to identify the nonlinear dynamics of the brain functional networks in schizophrenic patients (17). The usefulness of ApEn in diagnosing schizophrenia was studied by Taghavi et al. (18). They showed that the value of ApEn in most EEG channels significantly decreased in schizophrenic patients compared to healthy individuals. Sabeti et al. (19, 20) extracted ApEn, spectral entropy, Shanon entropy, Limpel-Ziv, fractal dimension, and auto-regressive (AR) coefficients from the EEG signals of healthy subjects and schizophrenic patients to differentiate these two groups of individuals (21, 22). They achieved a maximum classification accuracy of 91% for 20 schizophrenic patients and 20 age-matched controls. Note that the estimated value of entropy by different schemes is not equal, and some measures can reveal the EEG variations better (for a specific brain disease) than the other entropy measures.
Multiscale entropy is an efficient signal processing scheme to reveal the hidden patterns captured in relatively short time-series such as segmented EEG. Other entropy measures quantify only the irregularity of time-series on a single scale. In contrast, using a coarse-graining procedure, the MSE method quantifies the degree of complexity on multiple scales (23).
It is noteworthy to mention that extraction of entropy features (by each of the aforementioned schemes) from several EEG channels in successive time frames leads to providing high dimensional feature vectors. Moreover, some of these features are correlated. To make these features independent and also diminish the size of the features, some methods [e.g., principal component analysis (PCA)] can be used (24). The features extracted by PCA guarantee to preserve maximum information about the original features and provide new features with no dependency and much lower dimension.
After extracting low dimensional independent features, to classify the patients automatically, we need an intelligent classifier to learn patterns in the offline phase (train phase) and then classify the test features in the online phase. Since the Bayes classifier is the optimum classifier, this classifier can be used. Bayes' theorem measures the probability of each class regarding the input feature vector. In other words, for each class, a probability density function is estimated, and an input feature vector is assigned to that class whose classifier obtains a higher posterior probability. Nonetheless, we need to know the data distribution to use the general form of the Bayes classifier. Since the distribution of data is unknown, we cannot use the standard form of this optimum classier.
Nevertheless, there is a weak form of Bayes classifier, known as Gaussian Naïve Bayes (GNB), in which each dimension obeys a Gaussian distribution. Despite the simplicity of GNB, this classifier can capture the mean and variance of the features (25). GNB determines the label of each input by maximizing the posterior probability (26). If we apply the features to PCA before applying them to the classifier, we can guarantee that the input features to the GNB are independent. To classify the feature vectors of each class, we need to train an exclusive GNB classifier.
This study aimed to evaluate and separate schizophrenic patients with different symptomatology by characterizing their EEGs using the MSE method. We also differentiated schizophrenic subjects from healthy subjects using the same method. Moreover, the patient’s MSE patterns on all EEG channels were also studied and reported. Few studies have quantitatively assessed the differences in the EEG signals of schizophrenic patients with different dominant symptoms; hence, this area is still spending its infancy period, and more research is required to reveal more differences in their EEG signal patterns. To the best of the authors' knowledge, no research has yet been conducted on discriminating schizophrenic patients with different dominant symptoms by eliciting the MSE of their EEGs. Furthermore, in the recent and previous studies, researchers just showed a positive or negative correlation of the band power in different EEG bands in schizophrenic patients with positive and negative symptoms; however, they did not report the classification results. To fill up this gap, in this research, after extracting MSEs from all channels using the successive time frames, we applied PCA to these feature vectors to diminish their size and provide new independent features. Then we automatically used the reduced features of the GNB classifiers (27) to distinguish schizophrenic patients with positive and negative symptoms automatically. It should be emphasized that we aimed to classify patients automatically by adopting statistical classifiers and training them in the offline phase.
Moreover, we considered a control group and recorded their EEG signals to distinguish them from schizophrenic patients. In other words, a GNB for each class is trained, and in the test phase, the extracted features of EEG signals are applied to the three GNBs, and an input label is assigned to that class, the posterior probability of whose GNB is maximized. Another contribution of our study was to collect and prepare a new EEG dataset from 26 healthy subjects and 36 schizophrenic patients with positive and negative symptoms.
The present paper is outlined as follows. Section 2 introduces the collected EEG dataset, and the subjects and the recording protocol are specified. Then the preprocessing stage is explained, and the candidate features and classifier are expressed. Section 3 presents the empirical results of our dataset, and section 4 discusses the pros and cons of the proposed method compared to past research. The paper is finally concluded in section 5, and there are some recommendations for future research.
2. Objectives
In this paper, we have presented a fast and efficient computer-aided diagnosis (CAD) system, which can be used in private and general clinics not only to differentiate schizophrenic patients from control subjects but also to differentiate schizophrenic patients with dominant positive and negative symptoms. This research is critical since therapeutics focus more on positive symptoms; however, many patients with negative symptoms may also experience poor outcomes. Accordingly, the automatic and precise classification of schizophrenic patients with different dominant symptoms has a high impact on their treatment process. The diagnostic process is based on recording EEG channels captured by 19 electrodes. The MSE features were extracted in successive time frames from all EEG channels. By applying PCA to the elicited MSE features, they are projected into a new space with a lower dimension while the projected features are independent. Three GNB classifiers were trained for the corresponding three classes (controls, schizophrenia with positive and negative symptoms), which require independent features to be trained. The proposed CAD system provides high classification accuracy in differentiating the controls and schizophrenic patients and generates acceptable results for distinguishing between patients with positive and negative symptoms. Since there was no publicly available EEG data set for this purpose, we prepared and recorded an EEG dataset in a standard manner.
3. Methods
3.1. Data Collection
In this study, 36 patients with schizophrenia and 26 age-matched control participants (from both genders) with the age range of 25 - 60 years old participated voluntarily. All patients were diagnosed by DSM-V criteria, while the healthy participants had normal mental status. The patients were selected according to their dominant symptoms in a structural interview by an expert psychiatrist. Notably, all patients had symptoms in this study. The demographic characteristics of the participants are presented in Table 1. Inclusion criteria were determined based on the structural clinical psychiatric interview using the DSM-V criteria. The exclusion criterion was unwillingness to participate or collaborate in the research study due to some factors (e.g., irritability).
Demographic Data of Participants
Variables | Control Group | Positive Symptoms | Negative Symptoms |
---|---|---|---|
Number of subjects in each group | 26 | 18 | 18 |
Gender | |||
Male | 13 | 8 | 7 |
Female | 13 | 10 | 11 |
Age (y) | 31 ± 4.1 | 36.6 ± 8.6 | 37.8 ± 7.8 |
Duration (y) | 7.3 ± 2.5 | 8.6 ± 1.9 |
Their EEG signals were recorded via 21 electrodes (2 references and 19 scalp electrodes) molded on a cap according to the international 10 - 20 positioning system. Using the EEG recording, the subjects sat upright on a comfortable chair in the resting state with their eyes closed. The sampling frequency of the EEG setup was 500 Hz. The electrode FZ was selected as the ground electrode. The EEG recording session lasted for 35 minutes under the supervision of an EEG technician, who removed the trials contaminated by the high amplitude of noise and motion artifact.
3.2. Pre-processing
First, we change the data montage to the average montage. The recorded EEGs were filtered using a band-pass filter with 1 Hz and 55 Hz cut-off frequencies, respectively. Moreover, a notch filter was applied to remove the 50Hz component to eliminate the power line. After removing the artifacts by visual inspection, an independent component analysis (ICA) was applied to the EEG signals to remove the EMG and EOG sources. Finally, the filtered source signals were projected back on the scalp electrodes (28).
3.3. Multiscale Entropy
Multiscale entropy method for computing the entropy of biological signals was developed by Costa et al. (23). This approach computes the sample entropy on the original time-series (or "signal") and coarse-scaled series derived from the original signal. To extract MSE from a window of signal, several embedding time-series are constructed by averaging the successive samples within the non-overlapping windows of increasing length (Figure 1). Multiple scale time-series are embedded into the original signal using a coarse-graining procedure. The scale #1 series is the original time-series. The scale #2-time-series was obtained by averaging two successive values from the original series. Scale #3 was obtained by averaging every three original values, and this continues, as shown in (Figure 1).
Describing the coarse-graining procedure in determining multiscale entropy (MSE) for scales #2 and #3 (29).
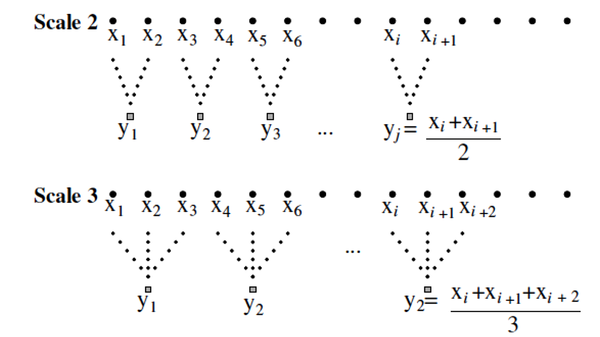
Irregularity at each scale is measured by SampEn, which is a correlation entropy version of Kolmogorov-Sinai entropy that is well suited for analyzing short and noisy experimental data(30). This library is implemented in Python, which provides high-level integrative functions like MSE (31).
3.4. Principal Component Analysis
First, the covariance matrix of the successive feature vectors should be calculated for extracting new features by PCA. Afterward, eigenvalues and their corresponding eigenvectors are determined. Then, we sort the eigenvalues in the descending order and apply an empirical threshold to the sorted values; bigger values are selected, and the eigenvectors corresponding to low eigenvalues are eliminated. This approach achieves a low number of new features with high variance (equal to high entropy).
3.5. Gaussian Naïve Bayes Classifier
Similar to the Bayes classifier, the GNB classifier is a supervised classifier, and the dimensions of input feature vectors are assumed to be independent. Accordingly, the conditional probability of each feature vector is equal to the multiplication of the conditional probability of all univariate features. Thus, GNB does not consider the covariance among the predictor variables due to the independence of features. Hence, the posterior probability (Y) given class kth for the input of X1 ..., XP is modeled according to the Bayes theorem (29).
3.6. Proposed Method
For simplicity, the roadmap of this paper is demonstrated in Figure 2. First, the signals are acquired from 19 channels and then preprocessed. After removing their noise, MSE from each channel is determined in successive windows, and they are then passed through the PCA transform to make the new features independent from each other. They are finally applied to three GNBs, each of which is trained by another class. For each input feature vector, the GNB with the highest output probability determines the class label of the input pattern.
The roadmap of the proposed scheme for distinguishing three groups of subjects. The classifiers GNB#1, GNB#2, and GNB#3 are trained for schizophrenic patients with positive symptoms, schizophrenics with negative symptoms, and control groups, respectively. Since each of these classifiers provides a probability given input data, the label of the input sample is determined by taking a maximum operator on the outputs of the three classifiers.
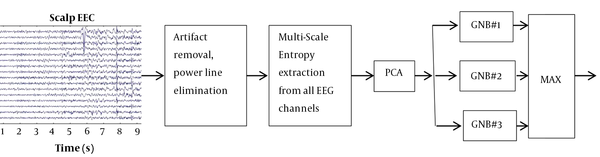
4. Results
This section presents the empirical results of applying the EEG entropy features to the classifiers. After recording the signals and applying the preprocessing method to the EEGs, signals are segmented by the window length of 1s, while successive windows have a 50% overlap. The entropy features were extracted from each windowed signal, and the features of all 19 channels belonging to identical time frames were arranged into feature vectors. The length of the recorded EEG signals is 185 seconds for each subject. Figure 3A shows the average MSE for the control group and the schizophrenic patients. The table shows P-values for schizophrenic patients with positive and negative symptoms. Moreover, Table 2 presents the extracted MSE values at each EEG channel for the three groups.
Multi-scale entropy (MSE) values. A, The average entropy of patients compared to the control group across all channels and the standard deviation of all channels are presented; B, The average entropy of patient sub-groups and control groups are presented. Moreover, the overlap between the patient and control groups is still considerable.
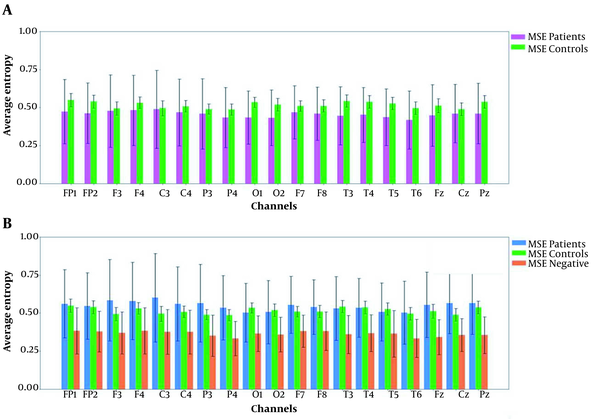
The Mean and Standard Deviation of Multiscale Entropy Features for Each Electroencephalography Channel for Each Group of Subjects a, b
Channels | Control Group | Positive Sub-group | Negative Sub-group |
---|---|---|---|
FP1 | 0.54 ± 0.04 | 0.56 ± 0.22 | 0.38 ± 0.15 |
FP2 | 0.53 ± 0.04 | 0.54 ± 0.21 | 0.37 ± 0.13 |
F3 | 0.49 ± 0.04 | 0.58 ± 0.26 | 0.36 ± 0.13 |
F4 | 0.53 ± 0.03 | 0.57 ± 0.25 | 0.38 ± 0.15 |
C3 | 0.49 ± 0.04 | 0.60 ± 0.29 | 0.37 ± 0.14 |
C4 | 0.50 ± 0.03 | 0.56 ± 0.24 | 0.37 ± 0.14 |
P3 | 0.49 ± 0.03 | 0.56 ± 0.25 | 0.35 ± 0.13 |
P4 | 0.48 ± 0.03 | 0.53 ± 0.21 | 0.33 ± 0.11 |
O1 | 0.53 ± 0.03 | 0.50 ± 0.19 | 0.36 ± 0.11 |
O2 | 0.51 ± 0.04 | 0.50 ± 0.20 | 0.35 ± 0.11 |
F7 | 0.51 ± 0.03 | 0.55 ± 0.18 | 0.38 ± 0.10 |
F8 | 0.51 ± 0.03 | 0.53 ± 0.17 | 0.38 ± 0.12 |
T3 | 0.54 ± 0.04 | 0.53 ± 0.20 | 0.36 ± 0.12 |
T4 | 0.53 ± 0.04 | 0.53 ± 0.19 | 0.36 ± 0.12 |
T5 | 0.52 ± 0.04 | 0.50 ± 0.19 | 0.36 ± 0.15 |
T6 | 0.49 ± 0.04 | 0.50 ± 0.20 | 0.33 ± 0.12 |
Fz | 0.51 ± 0.04 | 0.55 ± 0.21 | 0.34 ± 0.11 |
Cz | 0.49 ± 0.04 | 0.56 ± 0.19 | 0.35 ± 0.10 |
Pz | 0.53 ± 0.04 | 0.56 ± 0.20 | 0.35 ± 0.12 |
Total, over all channels | 0.516 ± 0.025 | 0.547 ± 0.205 | 0.364 ± 0.117 |
Moreover, Table 3 reports the P-value for the patients with positive symptoms and controls. To see the difference between the schizophrenic patients with different dominant symptoms, we compared their extracted features (Figure 3B), and their P-values are illustrated in Table 3. To decrease overlap between the MSE features of the corresponding groups, PCA is applied to the MSE features of all channels to reduce the dimension of original features by projecting them onto only a few principal components corresponding to greater eigenvalues. In this research, there were two new extracted features, which preserve data variation and information as much as possible. Moreover, we applied the extracted features to three GNBs, each separately trained for one of the classes.
Channels | Significance of P-Values Between Schizophrenic Patients and Control Subjects | |
---|---|---|
Schizophrenic Patients (Both Groups) vs. Control Group | Schizophrenic Patients with Positive Symptoms vs. Schizophrenic Patients with Negative Symptoms | |
Fp1 | × | √ |
Fp2 | × | √ |
F3 | × | √ |
F4 | × | √ |
C3 | × | √ |
C4 | × | √ |
P3 | × | √ |
P4 | × | √ |
O1 | √ | √ |
O2 | √ | √ |
F7 | × | √ |
F8 | × | √ |
T3 | √ | √ |
T4 | √ | √ |
T5 | √ | √ |
T6 | √ | √ |
Fz | × | √ |
Cz | × | √ |
Pz | √ | √ |
First, we exploit these models to predict and distinguish patients from the control group. After conducting 5-fold cross-validation, the average classification accuracy in differentiating controls from schizophrenic patients using GNBs is reported in Table 4. Then we applied the same procedure to differentiate schizophrenic patients with positive and negative symptoms. After conducting 5-fold cross-validation, the average accuracy in differentiating schizophrenic patients with different symptoms is shown in Table 4, which is not convincing. Finally, the total accuracy of this method for the three classes (controls group, positive prominent symptoms, and negative prominent symptoms) was determined (Table 4).
Classification Accuracy Between the Schizophrenic Patients with Different Dominant Symptoms and Also Between Schizophrenic Patients and Healthy Subjects
Healthy Subjects Versus Schizophrenic with Positive Symptoms Versus Schizophrenic with Negative Symptoms (Three Classes); % | Schizophrenic Patients Versus Healthy Subjects (Two Classes); % | Schizophrenic Patients with Positive and Negative Symptoms (Two Classes); % |
---|---|---|
77.8 | 94.5 | 65 |
In Figure 4, the confusion matrices are presented for the three classes on five-folds. Moreover, the boundary of the three classes for the five folds cross-validation is also demonstrated.
GNB classification on PCA features. The numerical results of the GNB classifiers in terms of confusion matrix are presented for the three classes. Moreover, boundaries formed by GNBs among the three classes (in different validation folds) and the samples of different classes on two dimensions extracted by PCA are demonstrated.
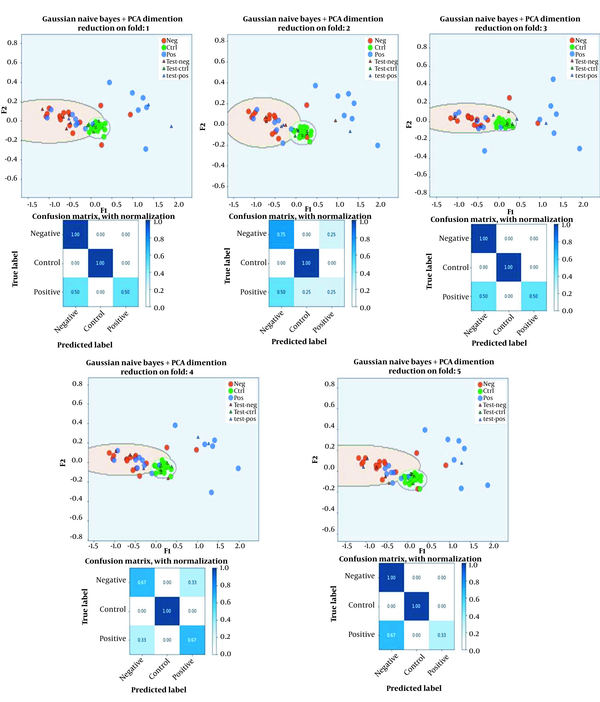
5. Discussion
In this section, we discuss similar and different methods concerning this research. First, we should emphasize that just a few studies have focused on differentiating schizophrenic patients based on their dominant symptoms. In contrast, many research studies are dedicated to classify schizophrenic patients from normal subjects or other mental diseases.
The neurobiology of schizophrenic patients with positive symptoms is different from that of patients with negative symptoms. In other words, schizophrenic patients with positive symptoms involve the dopamine and glutamate networks and affect the mesolimbic pathways. In contrast, schizophrenic patients with negative symptoms involve fronto-cortical temporal and cortico-striatal pathways (32). The results are in line with the findings reported in Tables 2 and 3. According to the tables, the entropy values on the electrodes, located above the fronto-cortical temporal and also above the limbic systems, revealed significant differences between the schizophrenic patients with positive and negative dominant symptoms.
Keil et al. (11) recorded the EEG signals of 22 schizophrenic patients and 22 age-matched controls. They found sensory gating deficits in schizophrenic patients compared to the age-matched controls. In this study, we determined high classification accuracy between the schizophrenic patients and the control group, which is in line with the findings reported in (11). In schizophrenic patients with positive symptoms, there is a positive correlation with the EEG power in the Gamma-band. Furthermore, the amount of phase synchrony sensory gating in the Alpha-band is negatively correlated in patients with negative symptoms. Although they empirically approved these correlations, they reported no classification result. Moreover, the desynchronization of the EEG signals in the Alpha-band is in line with the significance of entropy as an informative feature to classify these groups. Saletu et al. (13) investigated the EEG brain maps of 48 schizophrenic patients. They demonstrated a significant decrease in the Alpha band and a simultaneous increase in the Beta-band of the patients compared to healthy subjects. Schizophrenic patients with negative symptoms showed a frontotemporal augmentation in the delta/theta-band activity, while patients with florid symptomatology expressed opposite results. In schizophrenic patients with positive symptoms, there is a decrease in the alpha-band while Beta-band’s activity is increased. Unlike our study, these studies did not report the classification accuracy for the two groups and just reported some correlations between the standard EEG bands and their symptoms. John et al. (33) recorded the EEG signals of 28 schizophrenic patients and 25 healthy subjects by 30 electrodes. The EEG band power showed significant differences in the alpha-2, delta, and theta bands of the healthy and schizophrenic subjects.
In contrast, insignificant differences are observed in the alpha-1, alpha-2, theta, and delta bands between schizophrenic patients with positive and negative symptoms. In other words, differences in the band power in different bands lead to the difference in the irregularity and entropy of the EEGs originated from schizophrenic disease with positive and negative symptoms. This research does not report the classification results between the two groups. This is because there might be a high overlap between these correlations, which cannot lead to a considerable classification result. Begic et al. (34) investigated the EEG signals of 47 schizophrenic patients (25 patients with positive and 22 patients with negative symptoms) and 50 normal subjects over the frontal, temporal, parietal, and occipital regions (F3, F4, C3, C4, T3, T4, P3, P4, O1, and O2). In line with previous studies, differences were observed in the delta and theta bands over the frontal regions between the schizophrenic patients with positive and negative symptoms. Moreover, the differences between schizophrenic patients (both sub-groups) and age-matched controls were in the delta, theta, alpha, and beta-2 bands. Such differences in the band power of standard EEG bands imply variation in the entropy value between the schizophrenic patients with positive and negative symptoms. It should be mentioned that, similar to previous works, they reported no classification rate between the two groups.
In contrast, they are similar to our work in terms of reporting the feature values on different EEG channels. In our work, we have extracted the MSE features from each channel and elicited features from 19 channels, and reduced their dimension by PCA. We finally apply them to three Gaussian Naïve Bayes classifiers, each of which was trained for one class. We should emphasize that none of the previous work deploys an intelligent and statistical classifier to differentiate their cases automatically. It is confirmed that the entropy feature significantly varies between schizophrenic patients and healthy individuals (18, 19). The reason for choosing the entropy is that this feature can reveal the captured irregularity and the roughness of the EEG signals. Due to the differences in the interconnections between the two brain hemispheres in schizophrenia with positive and negative symptoms, different entropy values for these two schizophrenia sub-groups are expected. Although schizophrenia affects several parts of the brain, some parts are more affected because of their functional role in the brain. For example, the temporal lobe, which includes the limbic system and anatomical parts that dopaminergic fibers pass, also has better P-values on average in our method, as illustrated in Table 1.
In the second research category, the research findings illustrated that schizophrenic patients were differentiated from healthy subjects or other mental illnesses. In this regard, Boostani and Sabeti (9) showed the differences areas among the aforementioned groups by observing the fMRI and PET images of healthy subjects and schizophrenic subjects. In another study, Boostani and Sabeti (35) developed the brain map of schizophrenic and control subjects, and optimized the band power differences on 20 channels acquired from 20 schizophrenic patients and 20 age-matched controls. They could classify schizophrenic patients and control subjects using their brain map features with 84% accuracy.
Oscillatory activity among the neurons and the temporal synchronization of neurons (36) are effective factors in cognition and perception. It is demonstrated that in schizophrenic patients, the synchronization of their EEG is reduced during the visual integration and attention task (37). Visual integration means the ability to form a three-dimensional reconstruction of a spatial object (global object) from its side or local elements. Their visual integration is assessed by measuring their event-related potentials (ERP) (38), especially by characterizing the components of P100, N100, and P200. The function of visual pathways seems to be affected in schizophrenic patients.
Moreover, the synchronization in the gamma band (30 - 60Hz) declined in the schizophrenic patients (37). Nonetheless, nothing is reported about the differences among the visual evoke potential (VEP) features of patients with positive and negative symptoms. Evidently, the neural synchronization is distorted in schizophrenic patients; therefore, entropy-based EEG features from different channels can differentiate healthy subjects from schizophrenic patients (18, 19). Although schizophrenia symptoms affect the oscillatory activity of the EEG signals, the link between these symptoms and the EEG variation is unknown yet. Two different attempts have been made to differentiate schizophrenic patients and bipolar manic depression (BMD) patients. In one attempt, the synchronization of two-by-two EEG channels is used as the EEG features, and in the other one, steady-state VEP (SSVEP) have been extracted from the EEG signals and then characterize these templates to classify these two groups (39, 40). They reported convincing results when using synchronization measures and extracting the SSVEP features to distinguish schizophrenic patients finely. We hope that these two indicators, along with the functional features of brain connectivity (41), can help the psychiatrists better distinguish schizophrenic patients with positive and negative dominant symptoms.
The limitation of this study is the medium size of the participants, which was caused by the lack of time and financial issues. More studies with larger groups of participants are needed to evaluate such methods better. Moreover, the EEG signals are acquired in the resting state. If we had an EEG setup equipped with audio and visual stimuli, we could record the audio and VEP, and we may find more discriminating information on the evoke potentials of these three groups and increase their classification rate.
Acknowledgements
References
-
1.
Correll CU, Schooler NR. Negative Symptoms in Schizophrenia: A Review and Clinical Guide for Recognition, Assessment, and Treatment. Neuropsychiatr Dis Treat. 2020;16:519-34. [PubMed ID: 32110026]. [PubMed Central ID: PMC7041437]. https://doi.org/10.2147/NDT.S225643.
-
2.
American Psychiatric Association. Diagnostic and statistical manual of mental disorders. 5th ed. Washington, USA: American Psychiatric Association; 2013.
-
3.
Habtewold TD, Rodijk LH, Liemburg EJ, Sidorenkov G, Boezen HM, Bruggeman R, et al. A systematic review and narrative synthesis of data-driven studies in schizophrenia symptoms and cognitive deficits. Transl Psychiatry. 2020;10(1):244. [PubMed ID: 32694510]. [PubMed Central ID: PMC7374614]. https://doi.org/10.1038/s41398-020-00919-x.
-
4.
Kay SR, Fiszbein A, Opler LA. The positive and negative syndrome scale (PANSS) for schizophrenia. Schizophr Bull. 1987;13(2):261-76. [PubMed ID: 3616518]. https://doi.org/10.1093/schbul/13.2.261.
-
5.
Heckers S, Barch DM, Bustillo J, Gaebel W, Gur R, Malaspina D, et al. Structure of the psychotic disorders classification in DSM-5. Schizophr Res. 2013;150(1):11-4. [PubMed ID: 23707641]. https://doi.org/10.1016/j.schres.2013.04.039.
-
6.
Rabinowitz J, Levine SZ, Garibaldi G, Bugarski-Kirola D, Berardo CG, Kapur S. Negative symptoms have greater impact on functioning than positive symptoms in schizophrenia: analysis of CATIE data. Schizophr Res. 2012;137(1-3):147-50. [PubMed ID: 22316568]. https://doi.org/10.1016/j.schres.2012.01.015.
-
7.
Ho BC, Andreasen NC, Nopoulos P, Arndt S, Magnotta V, Flaum M. Progressive structural brain abnormalities and their relationship to clinical outcome: a longitudinal magnetic resonance imaging study early in schizophrenia. Arch Gen Psychiatry. 2003;60(6):585-94. [PubMed ID: 12796222]. https://doi.org/10.1001/archpsyc.60.6.585.
-
8.
Lisman J. Excitation, inhibition, local oscillations, or large-scale loops: what causes the symptoms of schizophrenia? Curr Opin Neurobiol. 2012;22(3):537-44. [PubMed ID: 22079494]. [PubMed Central ID: PMC3302967]. https://doi.org/10.1016/j.conb.2011.10.018.
-
9.
Boostani R, Sabeti M. Can Evolutionary-based Brain Map Be Used as a Complementary Diagnostic Tool with fMRI, CT and PET for Schizophrenic Patients? J Biomed Phys Eng. 2017;7(2):169-80. [PubMed ID: 28580339]. [PubMed Central ID: PMC5447254].
-
10.
Sigmundsson T, Suckling J, Maier M, Williams S, Bullmore E, Greenwood K, et al. Structural abnormalities in frontal, temporal, and limbic regions and interconnecting white matter tracts in schizophrenic patients with prominent negative symptoms. Am J Psychiatry. 2001;158(2):234-43. [PubMed ID: 11156806]. https://doi.org/10.1176/appi.ajp.158.2.234.
-
11.
Keil J, Roa Romero Y, Balz J, Henjes M, Senkowski D. Positive and Negative Symptoms in Schizophrenia Relate to Distinct Oscillatory Signatures of Sensory Gating. Front Hum Neurosci. 2016;10:104. [PubMed ID: 27014035]. [PubMed Central ID: PMC4789458]. https://doi.org/10.3389/fnhum.2016.00104.
-
12.
Fan Y, Gan Q, Feng H, Yuan L. Comparison and Analysis of EEG with Negative and Positive Symptoms of Schizophrenia. J Med Imaging & Health Infor. 2021;11(3):921-9. https://doi.org/10.1166/jmihi.2021.3339.
-
13.
Saletu B, Kufferle B, Anderer P, Grunberger J, Steinberger K. EEG-brain mapping in schizophrenics with predominantly positive and negative symptoms. Comparative studies with remoxipride/haloperidol. Eur Neuropsychopharmacol. 1990;1(1):27-36. [PubMed ID: 1983779]. https://doi.org/10.1016/0924-977x(90)90007-w.
-
14.
Feixas MA, Rigau J, Xu Q, Sbert M. Information Theory Tools for Image Processing: Synthesis Lectures on Computer Graphics and Animation. California, USA: Morgan & Claypool Publishers; 2014. 164 p. https://doi.org/10.2200/S00560ED1V01Y201312CGR015.
-
15.
Parbat D, Chakraborty M. A novel methodology to study the cognitive load induced EEG complexity changes: Chaos, fractal and entropy based approach. Biomed Signal Process Control. 2021;64:102277. https://doi.org/10.1016/j.bspc.2020.102277.
-
16.
Al-Jawahiri R, Jones M, Milne E. Spontaneous neural activity relates to psychiatric traits in 16p11.2 CNV carriers: An analysis of EEG spectral power and multiscale entropy. J Psychiatr Res. 2021;136:610-8. [PubMed ID: 33158556]. https://doi.org/10.1016/j.jpsychires.2020.10.036.
-
17.
Jia Y, Gu H. Identifying nonlinear dynamics of brain functional networks of patients with schizophrenia by sample entropy. Nonlinear Dyn. 2019;96:2327-40. https://doi.org/10.1007/s11071-019-04924-8.
-
18.
Taghavi M, Boostani R, Sabeti M, Taghavi SM. Usefulness of approximate entropy in the diagnosis of schizophrenia. Iran J Psychiatry Behav Sci. 2011;5(2):62-70. [PubMed ID: 24644448]. [PubMed Central ID: PMC3939972].
-
19.
Sabeti M, Katebi S, Boostani R. Entropy and complexity measures for EEG signal classification of schizophrenic and control participants. Artif Intell Med. 2009;47(3):263-74. [PubMed ID: 19403281]. https://doi.org/10.1016/j.artmed.2009.03.003.
-
20.
Sabeti M, Katebi SD, Boostani R, Price GW. A new approach for EEG signal classification of schizophrenic and control participants. Expert Syst Appl. 2011;38(3):2063-71. https://doi.org/10.1016/j.eswa.2010.07.145.
-
21.
Sabeti M, Boostani R, Katebi SD, Price GW. Selection of relevant features for EEG signal classification of schizophrenic patients. Biomed Signal Process Control. 2007;2(2):122-34. https://doi.org/10.1016/j.bspc.2007.03.003.
-
22.
Boostani R, Sadatnezhad K, Sabeti M. An efficient classifier to diagnose of schizophrenia based on the EEG signals. Expert Syst Appl. 2009;36(3):6492-9. https://doi.org/10.1016/j.eswa.2008.07.037.
-
23.
Costa M, Goldberger AL, Peng CK. Multiscale entropy analysis of biological signals. Phys Rev E Stat Nonlin Soft Matter Phys. 2005;71(2 Pt 1):21906. [PubMed ID: 15783351]. https://doi.org/10.1103/PhysRevE.71.021906.
-
24.
Kazemifar S, Boostani R, editors. Use of time-frequency transforms and kernel PCA to classify epileptic patients from control subjects. 6th International Conference on Intelligent Computing. 2010; Changsha, China. Springer; 2010. p. 465-72.
-
25.
Bishop CM. Pattern Recognition and Machine Learning. New York, USA: Springer; 2006.
-
26.
Ontivero-Ortega M, Lage-Castellanos A, Valente G, Goebel R, Valdes-Sosa M. Fast Gaussian Naïve Bayes for searchlight classification analysis. Neuroimage. 2017;163:471-9. [PubMed ID: 28877514]. https://doi.org/10.1016/j.neuroimage.2017.09.001.
-
27.
Lopez MM, Ramirez J, Gorriz JM, Alvarez I, Salas-Gonzalez D, Segovia F, et al. SVM-based CAD system for early detection of the Alzheimer's disease using kernel PCA and LDA. Neurosci Lett. 2009;464(3):233-8. [PubMed ID: 19716856]. https://doi.org/10.1016/j.neulet.2009.08.061.
-
28.
Albera L, Kachenoura A, Comon P, Karfoul A, Wendling F, Senhadji L, et al. ICA-based EEG denoising: a comparative analysis of fifteen methods. B Pol Acad Sci-Tech. 2012;60(3):407-18. https://doi.org/10.2478/v10175-012-0052-3.
-
29.
Swinburne R. Review: Bayes's Theorem. Rev Philos France Let. 2004;194(2):250-1.
-
30.
Richman JS, Moorman JR. Physiological time-series analysis using approximate entropy and sample entropy. Am J Physiol Heart Circ Physiol. 2000;278(6):H2039-49. [PubMed ID: 10843903]. https://doi.org/10.1152/ajpheart.2000.278.6.H2039.
-
31.
Makowski D, Pham T, Lau ZJ, Brammer JC, Lespinasse F, Pham H, et al. NeuroKit2: A Python toolbox for neurophysiological signal processing. Behav Res Methods. 2021;53(4):1689-96. [PubMed ID: 33528817]. https://doi.org/10.3758/s13428-020-01516-y.
-
32.
Nawaz U, Lee I, Beermann A, Eack S, Keshavan M, Brady R. Individual Variation in Functional Brain Network Topography is Linked to Schizophrenia Symptomatology. Schizophr Bull. 2021;47(1):180-8. [PubMed ID: 32648915]. [PubMed Central ID: PMC7824998]. https://doi.org/10.1093/schbul/sbaa088.
-
33.
John JP, Rangaswamy M, Thennarasu K, Khanna S, Nagaraj RB, Mukundan CR, et al. EEG power spectra differentiate positive and negative subgroups in neuroleptic-naive schizophrenia patients. J Neuropsychiatry Clin Neurosci. 2009;21(2):160-72. [PubMed ID: 19622687]. https://doi.org/10.1176/jnp.2009.21.2.160.
-
34.
Begic D, Hotujac L, Jokic-Begic N. Quantitative EEG in 'positive' and 'negative' schizophrenia. Acta Psychiatr Scand. 2000;101(4):307-11. [PubMed ID: 10782551].
-
35.
Boostani R, Sabeti M. Optimising brain map for the diagnosis of schizophrenia. Int J Biomed Eng Technol. 2018;28(2):105-19.
-
36.
Afrasiabi S, Boostani R, Masnadi-Shirazi MA. Differentiation of pain levels by deploying various electroencephalogram synchronization features and a dynamic ensemble selection mechanism. Physiol Meas. 2020;41(11). [PubMed ID: 33108779]. https://doi.org/10.1088/1361-6579/abc4f4.
-
37.
Wynn JK, Roach BJ, Lee J, Horan WP, Ford JM, Jimenez AM, et al. EEG findings of reduced neural synchronization during visual integration in schizophrenia. PLoS One. 2015;10(3). e0119849. [PubMed ID: 25785939]. [PubMed Central ID: PMC4364708]. https://doi.org/10.1371/journal.pone.0119849.
-
38.
Sabeti M, Boostani R, Moradi E. Event related potential (ERP) as a reliable biometric indicator: A comparative approach. Array. 2020;6:100026. https://doi.org/10.1016/j.array.2020.100026.
-
39.
Alimardani F, Cho J, Boostani R, Hwang H. Classification of bipolar disorder and schizophrenia using steady-state visual evoked potential based features. IEEE Access. 2018;6:40379-88. https://doi.org/10.1109/ACCESS.2018.2854555.
-
40.
Alimardani F, Boostani R, Azadehdel M, Ghanizadeh A, Rastegar K. Presenting a new search strategy to select synchronization values for classifying bipolar mood disorders from schizophrenic patients. Eng Appl Artif Intell. 2013;26(2):913-23. https://doi.org/10.1016/j.engappai.2012.06.010.
-
41.
Zhao Z, Li J, Niu Y, Wang C, Zhao J, Yuan Q, et al. Classification of Schizophrenia by Combination of Brain Effective and Functional Connectivity. Front Neurosci. 2021;15:651439. [PubMed ID: 34149345]. [PubMed Central ID: PMC8209471]. https://doi.org/10.3389/fnins.2021.651439.