Abstract
Background:
The sudden spread of COVID-19 raised significant concerns about the potential impact of the pandemic on healthcare systems in low- and middle-income nations. The length of hospital stay is a critical health metric with far-reaching implications in healthcare systems and a matter of resource allocation. Hospital beds, ventilators, medications, and the invaluable time and expertise of medical professionals are all finite resources. Understanding the factors influencing the length of hospitalization is essential for the efficient allocation of these resources and the provision of high-quality care.Objectives:
To explore the demographic features and clinical presentations of COVID-19 patients and investigate the relationship between patient characteristics and hospitalization length by employing advanced statistical models.Methods:
This was a retrospective study in Bam City, Kerman province, on 2096 patients who were admitted to the Pastor Hospital of Bam City with the diagnosis of COVID-19 between March and September 2021. The criterion for inclusion was either laboratory confirmation of SARS-CoV-2 infection by swab sampling or clinical diagnosis. No exclusion criteria were applied. Several variables, including age, gender, history of drug abuse, comorbid diseases, chronic illnesses, and clinical symptoms were gathered. A univariate analysis was performed, and significant parameters were subsequently included in the final multivariable model. We used SPSS 22, STATA 13, and R 4.1.3 software for statistical analysis.Results:
A total of 2096 patients admitted to the Pastor Hospital of Bam City from March to September 2021 diagnosed with COVID-19 were included in this study. The results of a negative binomial regression model showed that the factors affecting hospitalization length in COVID-19 patients were advanced age, male gender, contact with COVID-19 patients, PO2, body temperature, cancer, fever, heart disease, coughing severity, and respiratory distress.Conclusions:
Elder COVID-19 patients had relatively longer hospitalization periods. Moreover, hospitalization duration was longer in males, those who had contact with a COVID-19 patient, and patients with PO2 below 93 %, higher body temperature and fever, coughing, respiratory distress, heart diseases, and cancer compared to others. Therefore, it is recommended to monitor COVID-19 patients with heart disease and cancer more cautiously. Investigating the factors associated with long hospitalization is important for the suitable management of available resources and demands for hospital beds.Keywords
Hospitalization Duration Underlying Diseases Demographic Variables Count Regression Covid-19
1. Background
As a novel disease, COVID-19 is closely related to SARS viral species. The first cases of this virus infection were discovered in Wuhan, China, on December 8, 2019 (1). Due to the rapid global spread of the virus, the World Health Organization (WHO) declared a pandemic on March 11, 2020 (2). As of May 26, 2023, there have been 766,895,075 confirmed cases of COVID-19 worldwide, with 6,935,889 reported fatalities. In the same timeframe, Iran’s Ministry of Health reported 7,611,138 confirmed cases and 146,230 deaths (3).
While most COVID-19 patients present with mild symptoms, those with moderate-to-severe disease can rapidly progress to a fatal condition due to complications such as acute respiratory failure, multiorgan failure, disseminated intravascular coagulation (DIC), and septic shock (4). Data from countries where the virus initially propagated indicated that 5–16% of infected individuals required admission to the intensive care unit (ICU), inflicting a substantial burden on healthcare systems and deteriorating their capacity for admitting and treating patients. Therefore, some countries found it necessary to prioritize patients (5, 6). The available information strongly suggests that the lack of hospital admission is associated with higher mortality among individuals with COVID-19 (7).
The sudden surge in COVID-19 cases raises serious concerns about the potential collapse of healthcare systems in low- and middle-income nations that have limited resources. Understanding and forecasting demands for hospital beds and predicting the duration of each patient’s need for medical care are crucial for prioritizing patients, making timely decisions, and implementing backup plans (8, 9). It is important to be informed of the length of hospitalization and the factors influencing this parameter for predicting future demands on hospital resources and implementing strategies to shorten hospitalization length and reduce patient costs. According to Rees et al., the length of hospitalization in COVID-19 patients ranged from 4 to 53 days in China and from 4 to 21 days outside China (10).
It is crucial to identify the variables that affect a patient’s length of hospital stay so that effective interventions and treatments can be provided. Numerous studies have assessed the epidemiology, clinical features, and preventive and control measures for COVID-19, allowing researchers and decision-makers to better comprehend the disease (11, 12). There is inadequate research, however, on the factors that can influence the length of hospitalization in COVID-19 patients.
2. Objectives
According to studies on COVID-19, the risk of severe disease or death is higher in patients with underlying comorbidities, such as cancer, hypertension, diabetes, heart disease, kidney disease, liver disease, blood disorders, lung diseases, and others (1). This study was undertaken to describe the demographic features and clinical presentations of COVID-19 patients admitted to the Pastor Hospital of Bam City, located in Kerman province of Iran. Bam city is situated 195 km southeast of Kerman in the eastern region of the province. Additionally, we aimed to investigate the relationship between patient characteristics and the length of hospitalization using advanced statistical modeling, including count regression models, for the first time in this region.
3. Methods
This retrospective study was based on the analysis of medical records and was conducted in Bam City, Kerman province, Iran. A total of 2096 patients admitted to the Pastor Hospital of Bam due to COVID-19 diagnosis between March and September 2021 were studied.
Patients with confirmed laboratory diagnosis of SARS-CoV-2 (swab samples) or clinical diagnosis based on symptoms or consistent radiological findings were included. No exclusion criteria were applied; however, patients with incomplete information were excluded during data analysis.
The data collection process encompassed several variables, including age (in years), gender, history of drug abuse, and clinical symptoms such as fever, coughing, convulsions, diarrhea, chest pain, muscular pain, anorexia, respiratory distress, decreased level of consciousness, respiratory rate, PO2 levels, history of contact with a COVID-19 patient, and the number of days since the onset of symptoms. Additionally, comorbidities like cancer, liver disease, diabetes, hematological disorders, pregnancy, heart disease, kidney problems, asthma, lung disease, nervous disorders, chronic illnesses, and hypertension were recorded. Univariate analysis was initially conducted, and significant factors associated with the study’s outcomes were finally identified in a multivariable model.
3.1. Multicollinearity
In multiple regression models, multicollinearity refers to a linear relationship between independent variables. Multicollinearity may render some previously identified significant variables to become non-significant. One technique for detecting multicollinearity is the variance inflation factor (VIF) (13). In our study, we computed this index for each independent variable, and the mean VIF was obtained as 1.08, which indicated no multicollinearity and confirmed the reliability of our results.
3.2. Poisson Regression Model
The response variable in our study was hospitalization length (i.e., a count variable). It is important to note that, according to the literature, we decided to use a Poisson regression model in this scenario (given the presence of one or more independent variables) to predict the dependent variable (14).
Before using the Poisson regression model to analyze the data, it is essential to check certain assumptions to ensure the fit of this model, which is one of the critical assumptions pertaining to the relationship between the mean and variance (15, 16). Typically, in count data, the variance tends to exceed the mean (17, 18). In statistics, this condition is referred to as over-dispersion. When over-dispersion is observed, the application of the Poisson model may result in biased estimation and very small P-values (17). An alternative model for situations where the dependent variable does not adhere to a Poisson distribution is the negative binomial regression model (19). In our study, the response variable did not show a Poisson distribution. Therefore, we employed the negative binomial regression model for data analysis. The negative binomial regression model is similar to the Poisson regression model, in which the log of the expected response is predicted using a linear combination of predictors (20-22).
ln (Yi) = β0 + β1 X1i + ⋯ + βk Xki
3.3. Coefficient Interpretation
Coefficient interpretation in count models is easier based on the incidence rate ratio (IRR), which is similar to the odds ratio (OR) used for the interpretation coefficients in logistic regression models. For continuous predictor variables, we should interpret how a one-unit decrease or increase in the independent variable is associated with an increase or decrease in the count of the dependent variable. For categorical predictor variables, the interpretation is based on determining the percentage of decrease or increase in the counts of one group compared to the reference category or group (14, 23). The data were analyzed using SPSS 22, STATA 13, and R version 4.1.3.
4. Results
Demographic variables and clinical features of COVID-19 patients have been described in Table 1. The mean age of the patients was 48.07 ± 19.47 years. The mean (3.94) and variance (9.06) of the outcome variable were not equal, implying a poor fit of the Poisson model to our data. This was affirmed when the over-dispersion test retrieved a statistically significant parameter (overdispersion parameter = 1.959, P < 0.0001). So, as explained earlier, the negative binomial regression model is an alternative approach in this situation.
Descriptive Statistics of Demographic Variables and Clinical Features of Patients with COVID-19 in Bam City, Kerman Province, Eastern Iran a
Variables | Descriptive Statistics | Variables | Descriptive Statistics |
---|---|---|---|
Age | 48.07 ± 19.47 | Kidney disease | |
Hospitalization length (days) | 3.94 ± 3.01 | Yes | 25 (1.2) |
Days passed from the onset of symptoms | 4.99 ± 3.39 | No | 2071 (98.8) |
Respiratory rate | Heart disease | ||
≤ 22 | 165 (7.9) | Yes | (7.4) |
> 22 | 1931 (92.1) | No | 1940 (92.6) |
Contact with COVID-19 patient | Abdominal pain | ||
Yes | 867 (41.4) | Yes | 40 (1.9) |
No | 1229 (58.6) | No | 2056 (98.1) |
Gender | Neuronal disorders | ||
Male | 951 (45.4) | Yes | 19 (0.9) |
Female | 1145 (54.6) | No | 2077 (99.1) |
PO2 | Diarrhea | ||
< 93% | 760 (36.3) | Yes | 2012 (96) |
> 93% | 1336 (63.7) | No | 84 (4) |
Opium use | Fever | ||
Yes | 61 (2.9) | Yes | 1125 (53.7) |
No | 2035 (97.1) | No | 971 (46.3) |
Cancer | Cough | ||
Yes | 17 (0.8) | Yes | 842 (40.2) |
No | 2079 (99.2) | No | 1254 (59.8) |
Other chronic diseases | Muscular pain | ||
Yes | 181 (8.6) | Yes | 426 (79.7) |
No | 1915 (91.4) | No | 1670 (20.3) |
Diabetes | Respiratory distress | ||
Yes | 256 (12.2) | Yes | 902 (43) |
No | 1840 (87.8) | No | 1194 (57) |
Headache | Chest pain | ||
Yes | 245 (11.7) | Yes | 104 (5) |
No | 1851 (88.3) | No | 1992 (%95) |
Pregnancy | Vomiting | ||
Yes | 34 (1.6) | Yes | 73 (3.5) |
No | 2062 (98.4) | No | 2023 (96.5) |
The results of the negative binomial regression model (Table 2) revealed that several factors could significantly influence the duration of hospitalization in COVID-19 patients, including age (P < 0.0001), gender (P = 0.006), contact with a COVID-19 patient (P = 0.002), PO2 (P < 0.0001), cancer (P = 0.008), heart disease (P = 0.024), fever (P = 0.004), coughing (P < 0.0001), and respiratory distress (P = 0.019). According to the IRRs obtained, the incidence rate of hospitalization increased by 1.003 times for every one-year increase in age and was 1.08 times higher in males than in females. In patients who had contact with a COVID-19 patient, the incidence rate of hospitalization was 1.09 times higher than other patients, considering other variables to be constant. Additionally, patients with PO2 levels below 93% had a 1.34-time higher incidence rate of hospitalization compared to those with PO2 levels above 93%. Patients with cough, fever, and respiratory distress had hospitalization incidence rates of 1.13, 1.09, and 1.07 times higher compared to patients without these symptoms, respectively. Furthermore, COVID-19 patients with cancer and heart disease had hospitalization incidence rates of 1.44 and 1.134 times higher than others. Figure 1 shows the parameter coefficients retrieved by the negative binomial regression model.
The Results of Negative Binomial Regression for Demographic Variables and Clinical Features of Patients with COVID-19 in Bam City, Kerman Province, Eastern Iran a
Variables | Coefficients | Standard Error | P-Value | %95 CI | %95 CI | |||
---|---|---|---|---|---|---|---|---|
Lower | Upper | IRR | Lower | Upper | ||||
Intercept | - 0.241 | 0.248 | 0.332 | - 0.245 | 0.726 | 1.272 | 0.778 | 2.058 |
Age | 0.0035 | 0.0008 | < 0.0001* | 0.002 | 0.005 | 1.003 | 1.002 | 1.005 |
Days passed from the onset of symptoms | 0.0055 | 0.0041 | 0.189 | - 0.0027 | 0.014 | 1.005 | 0.997 | 1.014 |
Contact with COVID-19 patient (Ref = No) | 0.089 | 0.028 | 0.002* | 0.033 | 0.146 | 1.094 | 1.034 | 1.157 |
PO2 (Ref >93%) | 0.294 | 0.030 | < 0.0001* | 0.234 | 0.354 | 1.342 | 1.265 | 1.425 |
Respiratory rate | - 0.023 | 0.054 | 0.669 | -0.129 | 0.083 | 0.977 | 0.878 | 1.086 |
Male gender (Ref=female) | 0.077 | 0.028 | 0.006* | 0.022 | 0.133 | 1.081 | 1.022 | 1.143 |
Opium use | 0.019 | 0.083 | 0.815 | - 0.143 | 0.182 | 1.020 | 0.865 | 1.199 |
Cancer | 0.367 | 0.138 | 0.008* | 0.095 | 0.638 | 1.443 | 1.098 | 1.893 |
Diabetes | - 0.009 | 0.0430 | 0.836 | - 0.093 | 0.075 | 0.991 | 0.911 | 1.078 |
Pregnancy | 0.017 | 0.116 | 0.882 | - 0.210 | 0.245 | 1.017 | 0.808 | 1.274 |
Heart disease | 0.126 | 0.055 | 0.024* | 0.016 | 0.234 | 1.134 | 1.017 | 1.265 |
Kidney disease | 0.204 | 0.142 | 0.151 | - 0.074 | 0.483 | 1.227 | 0.932 | 1.630 |
Neuronal disorders | 0.210 | 0.160 | 0.189 | - 0.103 | 0.525 | 1.234 | 0.907 | 1.702 |
Other chronic diseases | 0.0005 | 0.049 | 0.991 | - 0.095 | 0.097 | 1.000 | 0.909 | 1.102 |
Fever | 0.084 | 0.029 | 0.004* | 0.028 | 0.141 | 1.088 | 1.028 | 1.152 |
Cough | 0.123 | 0.029 | < 0.0001* | 0.066 | 0.180 | 1.131 | 1.068 | 1.197 |
Muscular pain | 0.043 | 0.035 | 0.226 | - 0.027 | 0.114 | 1.044 | 0.973 | 1.120 |
Respiratory distress | 0.069 | 0.029 | 0.019* | 0.011 | 0.127 | 1.072 | 1.011 | 1.136 |
Diarrhea | 0.048 | 0.076 | 0.526 | - 0.101 | 0.197 | 1.049 | 0.905 | 1.219 |
Chest pain | 0.008 | 0.067 | 0.903 | - 0.124 | 0.141 | 1.008 | 0.882 | 1.150 |
Abdominal pain | - 0.130 | 0.111 | 0.239 | - 0.348 | 0.087 | 0.878 | 0.704 | 1.088 |
Headache | - 0.027 | 0.045 | 0.551 | - 0.115 | 0.061 | 0.973 | 0.891 | 1.063 |
Vomiting | 0.017 | 0.080 | 0.824 | - 0.140 | 0.176 | 1.018 | 0.868 | 1.191 |
The coefficient plot of negative binomial regression
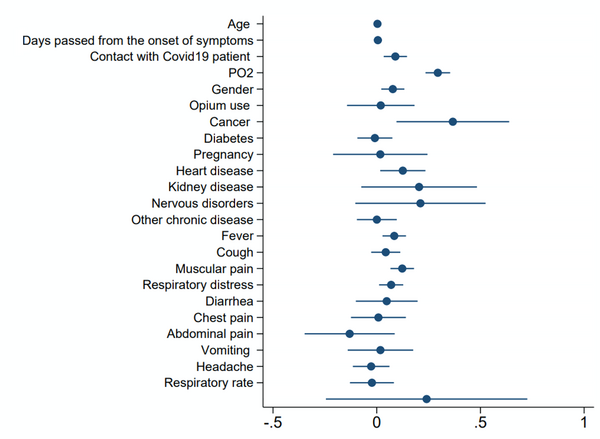
4.1. Goodness-of-fit
The goodness-of-fit test is crucial for assessing how well a statistical model aligns with the respective data, ensuring the accuracy of predictions and conclusions. For this aim, we employed two tests (i.e., the link test and the likelihood ratio test) (24). The non-significance of the hat-square value in the link test suggested that the negative binomial model appropriately fitted into the data (Hat square = 0.101, P = 0.692). Also, the results of the likelihood ratio test (chi-squared value = 369.49, P < 0.0001) also strongly indicated that the negative binomial model was more appropriate than the Poisson model.
5. Discussion
According to our findings, the mean± SD of hospitalization length in COVID-19 patients was 3.94±3.01 days. The most influential factors affecting the duration of hospitalization in these patients included age, gender, history of contact with COVID-19 patients, PO2 levels, the presence of comorbidities (cancer, heart disease), fever, coughs, and respiratory distress.
The mean duration of hospitalization in COVID-19 patients has been reported to vary across different countries, from 6 days in Saudi Arabia (25) and 7 days in Peru (1) to 8.5 days in the Mediterranean region (25). This observed variation can be attributed to various factors, including demographic characteristics, the severity of the disease, and different criteria used for admitting or discharging patients (26). In a report from Belgium, hospitalization duration varied from 3 to 10.4 days, and age was identified to be a significant determinant (27), which was consistent with our observation. The impact of age on the length of hospitalization is not unexpected, as it has been affirmed in many studies (25, 28). The mechanism behind the increase in hospitalization duration with age may be attributed to more severe disease, weakened immune system, and a higher prevalence of chronic diseases in elders (29). Additionally, similar to other studies, we observed that the length of hospital stay was longer in males than in females (30). Contact with COVID-19 patients was another significant factor affecting the length of hospital stay. Such contacts increase the risk of infection (31), which may justify the extended duration of hospitalization.
Our study uncovered that patients suffering from respiratory distress had prolonged hospitalizations, which was similar to the findings of other studies (32-35). Respiratory distress showed a direct impact on the length of hospitalization in our study and was recognized as one of the significant contributors to the high mortality rate among COVID-19 patients (36, 37). Another factor associated with the duration of hospital stay was fever. Fever has been the most commonly used indicator for screening suspected COVID-19 patients (38-40), and it is a prevalent symptom of this infection (41).
Although diabetes is a common comorbidity among COVID-19 patients (42), we did not find a significant association between the length of hospital stay and diabetes, which was consistent with the findings of Wu et al. (43). Also, cardiac disease is among the top ten comorbidities associated with COVID-19, and patients with heart disease have been reported to be at a higher risk of developing severe COVID-19 and requiring hospitalization (44).
In our study, cancer was another significant factor affecting the length of hospital stay in COVID-19 patients. This finding aligned with a survey that examined the relationship between depression and cancer in COVID-19-infected patients (45). Additionally, a systematic review showed that SARS-CoV-2 contraction could lead to the aggravation of disease and poor outcomes in patients with cancer (46), which could evidently extend the length of hospital stay.
Our study has several limitations, including limited available data and lack of access to some important information, such as treatments administered and COVID-19 severity, requiring future comprehensive studies to assess these variables.
In conclusion, this retrospective study on COVID-19 patients showed a positive association between the length of hospital stay and parameters such as age, PO2 level, respiratory rate, body temperature, underlying cancer, contact with COVID-19-infected patients, coughing, and respiratory distress. It is recommended to monitor COVID-19 patients with underlying heart disease or cancer more closely. Investigating the risk factors affecting the length of hospitalization in COVID-19 patients is important for the suitable management of available health resources, fair allocation of hospital beds, and effective resolution of relevant challenges. Our findings provide valuable insights for decision-makers to become prepared for future epidemic waves of SARS-Cov-2 and its new variants and predict the demand for hospital beds.
References
-
1.
Mejia F, Medina C, Cornejo E, Morello E, Vasquez S, Alave J, et al. Oxygen saturation as a predictor of mortality in hospitalized adult patients with COVID-19 in a public hospital in Lima, Peru. PLoS One. 2020;15(12). e0244171. [PubMed ID: 33370364]. [PubMed Central ID: PMC7769479]. https://doi.org/10.1371/journal.pone.0244171.
-
2.
World Health Organization. Virtual Press Conference on COVID‑19. 2020. Available from: https://www.who.int/publications/m/item/covid-19-virtual-press-conference-transcript---14-december-2020.
-
3.
World Health Organization. WHO Coronavirus (COVID-19) Dashboard. 2023. Available from: https://covid19.who.int/.
-
4.
Gorlinger K, Dirkmann D, Gandhi A, Simioni P. COVID-19-associated coagulopathy and inflammatory response: What do we know already and what are the knowledge gaps? Anesth Analg. 2020;131(5):1324-33. [PubMed ID: 33079850]. [PubMed Central ID: PMC7389937]. https://doi.org/10.1213/ANE.0000000000005147.
-
5.
Grasselli G, Pesenti A, Cecconi M. Critical care utilization for the COVID-19 outbreak in lombardy, italy: Early experience and forecast during an emergency response. JAMA. 2020;323(16):1545-6. [PubMed ID: 32167538]. https://doi.org/10.1001/jama.2020.4031.
-
6.
Guan WJ, Ni ZY, Hu Y, Liang WH, Ou CQ, He JX, et al. Clinical characteristics of coronavirus disease 2019 in China. N Engl J Med. 2020;382(18):1708-20. [PubMed ID: 32109013]. [PubMed Central ID: PMC7092819]. https://doi.org/10.1056/NEJMoa2002032.
-
7.
Wu Z, McGoogan JM. Characteristics of and important lessons from the coronavirus disease 2019 (covid-19) outbreak in china: Summary of a report of 72314 cases from the chinese center for disease control and prevention. JAMA. 2020;323(13):1239-42. [PubMed ID: 32091533]. https://doi.org/10.1001/jama.2020.2648.
-
8.
Rodriguez-Morales AJ, Cardona-Ospina JA, Gutierrez-Ocampo E, Villamizar-Pena R, Holguin-Rivera Y, Escalera-Antezana JP, et al. Clinical, laboratory and imaging features of COVID-19: A systematic review and meta-analysis. Travel Med Infect Dis. 2020;34:101623. [PubMed ID: 32179124]. [PubMed Central ID: PMC7102608]. https://doi.org/10.1016/j.tmaid.2020.101623.
-
9.
Rosenbaum L. Facing covid-19 in italy - ethics, logistics, and therapeutics on the epidemic's front line. N Engl J Med. 2020;382(20):1873-5. [PubMed ID: 32187459]. https://doi.org/10.1056/NEJMp2005492.
-
10.
Rees EM, Nightingale ES, Jafari Y, Waterlow NR, Clifford S, B. Pearson CA, et al. COVID-19 length of hospital stay: A systematic review and data synthesis. BMC Med. 2020;18(1):270. [PubMed ID: 32878619]. [PubMed Central ID: PMC7467845]. https://doi.org/10.1186/s12916-020-01726-3.
-
11.
Wang D, Hu B, Hu C, Zhu F, Liu X, Zhang J, et al. Clinical Characteristics of 138 Hospitalized Patients With 2019 Novel Coronavirus-Infected Pneumonia in Wuhan, China. JAMA. 2020;323(11):1061-9. [PubMed ID: 32031570]. [PubMed Central ID: PMC7042881]. https://doi.org/10.1001/jama.2020.1585.
-
12.
Wu J, Liu J, Zhao X, Liu C, Wang W, Wang D, et al. Clinical characteristics of imported cases of coronavirus disease 2019 (covid-19) in jiangsu province: A multicenter descriptive study. Clin Infect Dis. 2020;71(15):706-12. [PubMed ID: 32109279]. [PubMed Central ID: PMC7108195]. https://doi.org/10.1093/cid/ciaa199.
-
13.
Shrestha N. Detecting multicollinearity in regression analysis. American J Applied Mathematics Statistics. 2020;8(2):39-42. https://doi.org/10.12691/ajams-8-2-1.
-
14.
Sellers KF, Shmueli G. A flexible regression model for count data. Annals Applied Statistics. 2010;4(2). https://doi.org/10.1214/09-aoas306.
-
15.
Zaninotto P, Falaschetti E. Comparison of methods for modelling a count outcome with excess zeros: Application to Activities of Daily Living (ADL-s). J Epidemiol Community Health. 2011;65(3):205-10. [PubMed ID: 20675703]. https://doi.org/10.1136/jech.2008.079640.
-
16.
Famoye F, Singh KP. Zero-inflated generalized poisson regression model with an application to domestic violence data. J Data Sci. 2021;4(1):117-30. https://doi.org/10.6339/jds.2006.04(1).257.
-
17.
Karazsia BT, van Dulmen MH. Regression models for count data: Illustrations using longitudinal predictors of childhood injury. J Pediatr Psychol. 2008;33(10):1076-84. [PubMed ID: 18522994]. https://doi.org/10.1093/jpepsy/jsn055.
-
18.
YESILOVA A, Yılmaz KAYA, Barıs KAKI, Kasap İ. Analysis of plant protection studies with excess zeros using zero-inflated and negative binomial hurdle models. Gazi University J Sci. 2010;23(2):131-6.
-
19.
Jalali M, Nikfarjam A, Haghdoost AA, Memaryan N, Tarjoman T, Baneshi MR. Social hidden groups size analyzing: Application of count regression models for excess zeros. J Res in Health Sci. 2013;13(2):143-50.
-
20.
Greene W. Functional forms for the negative binomial model for count data. Economics Letters. 2008;99(3):585-90. https://doi.org/10.1016/j.econlet.2007.10.015.
-
21.
Gardner W, Mulvey EP, Shaw EC. Regression analyses of counts and rates: Poisson, overdispersed Poisson, and negative binomial models. Psychol Bull. 1995;118(3):392-404. [PubMed ID: 7501743]. https://doi.org/10.1037/0033-2909.118.3.392.
-
22.
Dean CB. Testing for overdispersion in poisson and binomial regression models. J American Statistical Association. 1992;87(418):451-7. https://doi.org/10.1080/01621459.1992.10475225.
-
23.
Hutchinson MK, Holtman MC. Analysis of count data using poisson regression. Res Nurs Health. 2005;28(5):408-18. [PubMed ID: 16163676]. https://doi.org/10.1002/nur.20093.
-
24.
Pregibon D. Goodness of link tests for generalized linear models. Applied Statistics. 1980;29(1). https://doi.org/10.2307/2346405.
-
25.
Alwafi H, Naser AY, Qanash S, Brinji AS, Ghazawi MA, Alotaibi B, et al. Predictors of length of hospital stay, mortality, and outcomes among hospitalised covid-19 patients in saudi arabia: A cross-sectional study. J Multidiscip Healthc. 2021;14:839-52. [PubMed ID: 33883900]. [PubMed Central ID: PMC8055273]. https://doi.org/10.2147/JMDH.S304788.
-
26.
Grasselli G, Zangrillo A, Zanella A, Antonelli M, Cabrini L, Castelli A, et al. Baseline characteristics and outcomes of 1591 patients infected with SARS-COV-2 admitted to icus of the lombardy region, italy. JAMA. 2020;323(16):1574-81. [PubMed ID: 32250385]. [PubMed Central ID: PMC7136855]. https://doi.org/10.1001/jama.2020.5394.
-
27.
Faes C, Abrams S, Van Beckhoven D, Meyfroidt G, Vlieghe E, Hens N, et al. Time between Symptom Onset, Hospitalisation and Recovery or Death: Statistical Analysis of Belgian COVID-19 Patients. Int J Environ Res Public Health. 2020;17(20). [PubMed ID: 33080869]. [PubMed Central ID: PMC7589278]. https://doi.org/10.3390/ijerph17207560.
-
28.
Wei C, Liu Y, Liu Y, Zhang K, Su D, Zhong M, et al. Clinical characteristics and manifestations in older patients with COVID-19. BMC Geriatrics. 2020;20(1). https://doi.org/10.1186/s12877-020-01811-5.
-
29.
Khajuria A, Bobdey S, Kumar S, Sahu R, Vashisht R, Bhaskar V, et al. An analysis of length of hospital stay of COVID-19 patients admitted in a dedicated COVID-19 hospital. J Marine Med Society. 2021;0(0). https://doi.org/10.4103/jmms.jmms_156_20.
-
30.
Nguyen NT, Chinn J, De Ferrante M, Kirby KA, Hohmann SF, Amin A. Male gender is a predictor of higher mortality in hospitalized adults with COVID-19. PLoS One. 2021;16(7). e0254066. [PubMed ID: 34242273]. [PubMed Central ID: PMC8270145]. https://doi.org/10.1371/journal.pone.0254066.
-
31.
Barboza JJ, Chambergo-Michilot D, Velasquez-Sotomayor M, Silva-Rengifo C, Diaz-Arocutipa C, Caballero-Alvarado J, et al. Assessment and management of asymptomatic COVID-19 infection: A systematic review. Travel Medicine Infectious Disease. 2021;41:102058.
-
32.
Verma HK. Radiological and clinical spectrum of COVID-19: A major concern for public health. World J Radiol. 2021;13(3):53-63. [PubMed ID: 33815683]. [PubMed Central ID: PMC8006056]. https://doi.org/10.4329/wjr.v13.i3.53.
-
33.
Bernheim A, Mei X, Huang M, Yang Y, Fayad ZA, Zhang N, et al. Chest CT Findings in Coronavirus Disease-19 (COVID-19): Relationship to duration of infection. Radiology. 2020;295(3):200463. [PubMed ID: 32077789]. [PubMed Central ID: PMC7233369]. https://doi.org/10.1148/radiol.2020200463.
-
34.
Shi H, Han X, Jiang N, Cao Y, Alwalid O, Gu J, et al. Radiological findings from 81 patients with COVID-19 pneumonia in Wuhan, China: A descriptive study. Lancet Infect Dis. 2020;20(4):425-34. [PubMed ID: 32105637]. [PubMed Central ID: PMC7159053]. https://doi.org/10.1016/S1473-3099(20)30086-4.
-
35.
Lim AYH, Goh JL, Chua MCW, Heng BH, Abisheganaden JA, George PP. Temporal changes of haematological and radiological findings of the COVID-19 infection-a review of literature. BMC Pulm Med. 2021;21(1):37. [PubMed ID: 33482780]. [PubMed Central ID: PMC7820529]. https://doi.org/10.1186/s12890-020-01389-z.
-
36.
Hasan SS, Capstick T, Ahmed R, Kow CS, Mazhar F, Merchant HA, et al. Mortality in COVID-19 patients with acute respiratory distress syndrome and corticosteroids use: A systematic review and meta-analysis. Expert Rev Respir Med. 2020;14(11):1149-63. [PubMed ID: 32734777]. [PubMed Central ID: PMC7544968]. https://doi.org/10.1080/17476348.2020.1804365.
-
37.
Shin E, Jin J, Park SY, Yoo YS, Lee JH, An J, et al. Impact of asthma, chronic obstructive pulmonary disease (COPD), and asthma-COPD overlap on the prognosis of coronavirus disease 2019. Asia Pac Allergy. 2022;12(2). e21. [PubMed ID: 35571550]. [PubMed Central ID: PMC9066077]. https://doi.org/10.5415/apallergy.2022.12.e21.
-
38.
Huang C, Wang Y, Li X, Ren L, Zhao J, Hu Y, et al. Clinical features of patients infected with 2019 novel coronavirus in Wuhan, China. Lancet. 2020;395(10223):497-506. [PubMed ID: 31986264]. [PubMed Central ID: PMC7159299]. https://doi.org/10.1016/S0140-6736(20)30183-5.
-
39.
Chen N, Zhou M, Dong X, Qu J, Gong F, Han Y, et al. Epidemiological and clinical characteristics of 99 cases of 2019 novel coronavirus pneumonia in Wuhan, China: A descriptive study. Lancet. 2020;395(10223):507-13. [PubMed ID: 32007143]. [PubMed Central ID: PMC7135076]. https://doi.org/10.1016/S0140-6736(20)30211-7.
-
40.
Fu L, Wang B, Yuan T, Chen X, Ao Y, Fitzpatrick T, et al. Clinical characteristics of coronavirus disease 2019 (COVID-19) in China: A systematic review and meta-analysis. J Infect. 2020;80(6):656-65. [PubMed ID: 32283155]. [PubMed Central ID: PMC7151416]. https://doi.org/10.1016/j.jinf.2020.03.041.
-
41.
Ji W, Zhang J, Bishnu G, Du X, Chen X, Xu H, et al. Comparison of severe and non-severe COVID-19 pneumonia: Review and meta-analysis. MedRxiv. 2020. https://doi.org/10.1101/2020.03.04.20030965.
-
42.
Yang J, Zheng Y, Gou X, Pu K, Chen Z, Guo Q, et al. Prevalence of comorbidities and its effects in patients infected with SARS-CoV-2: A systematic review and meta-analysis. Int J Infect Dis. 2020;94:91-5. [PubMed ID: 32173574]. [PubMed Central ID: PMC7194638]. https://doi.org/10.1016/j.ijid.2020.03.017.
-
43.
Wu S, Xue L, Legido-Quigley H, Khan M, Wu H, Peng X, et al. Understanding factors influencing the length of hospital stay among non-severe COVID-19 patients: A retrospective cohort study in a Fangcang shelter hospital. PLoS One. 2020;15(10). e0240959. [PubMed ID: 33085709]. [PubMed Central ID: PMC7577449]. https://doi.org/10.1371/journal.pone.0240959.
-
44.
Terlecki M, Wojciechowska W, Klocek M, Olszanecka A, Stolarz-Skrzypek K, Grodzicki T, et al. Association between cardiovascular disease, cardiovascular drug therapy, and in-hospital outcomes in patients with COVID-19: data from a large single-center registry in Poland. Kardiol Pol. 2021;79(7-8):773-80. [PubMed ID: 33926173]. https://doi.org/10.33963/KP.15990.
-
45.
Arrieta O, Angulo LP, Nunez-Valencia C, Dorantes-Gallareta Y, Macedo EO, Martinez-Lopez D, et al. Association of depression and anxiety on quality of life, treatment adherence, and prognosis in patients with advanced non-small cell lung cancer. Ann Surg Oncol. 2013;20(6):1941-8. [PubMed ID: 23263699]. https://doi.org/10.1245/s10434-012-2793-5.
-
46.
Dai M, Liu D, Liu M, Zhou F, Li G, Chen Z, et al. Patients with Cancer Appear More Vulnerable to SARS-CoV-2: A Multicenter Study during the COVID-19 Outbreak. Cancer Discov. 2020;10(6):783-91. [PubMed ID: 32345594]. [PubMed Central ID: PMC7309152]. https://doi.org/10.1158/2159-8290.CD-20-0422.