Abstract
Background:
Human fascioliasis, a growing health concern, has increased significantly over the last few decades and serves as a notable example of emerging and re-emerging disease foci in numerous countries.Objectives:
This study aimed to evaluate the incidence, spatial distribution, and hotspot regions of Fasciola infection in Iran using geographic information system (GIS) analyses from 2013 to 2022.Methods:
Data on Fasciola cases and populations at risk across various provinces were obtained from the Ministry of Health and Medical Education, Tehran, Iran, and other relevant organizations for the years 2013 to 2022. A map illustrating the geographical distribution of fascioliasis was generated. Spatial analyses were conducted using ArcGIS 10.5 software to identify hotspot regions of fascioliasis in Iran. The correlation between temperature, relative humidity, the normalized difference vegetation index (NDVI), and the incidence of fascioliasis (variables influencing the disease) was assessed using geographically weighted regression (GWR) in ArcGIS 10.5. Data analysis was performed using linear regression and SPSS version 21 software.Results:
Hotspot provinces for fascioliasis were identified in Gilan, Kermanshah, and Khorramabad. Geographically weighted regression analysis revealed a high correlation between humidity, temperature, vegetation density, and the incidence of Fasciola infection in the provinces of Gilan, Kermanshah, Khorramabad, Kurdistan, Semnan, and South Khorasan (P-value = 0.025).Conclusions:
This study demonstrated a significant relationship between relative humidity, mean annual temperature, NDVI, and the incidence of Fasciola infection in Iran. Geographic information system analysis proved to be an effective tool for identifying risk factors and assessing endemic areas of fascioliasis within specific regions.Keywords
Geographical Information System (GIS) Risk Mapping Spatial Modeling Fascioliasis Fasciola Infection
1. Background
Fasciola hepatica and Fasciola gigantica are major foodborne parasites responsible for fascioliasis, a serious and emerging infection affecting both humans and animals worldwide (1). Approximately 180 million people are at risk of Fasciola infection, with 2.4 to 17 million people currently infected globally (2, 3). Fascioliasis is recognized as a significant health concern in many countries, with its epidemiological patterns shifting as it emerges or re-emerges in various regions around the world (4). The global prevalence of fascioliasis among humans is increasing (1). Climate change has the potential to alter rainfall patterns and temperatures in various regions, creating new habitats suitable for Fasciola parasites and their hosts. Environmental modifications, such as irrigation projects, can also contribute to the availability of these habitats. These factors may lead to the expansion of endemic areas and an increase in the prevalence of human fascioliasis (5, 6). Iran, identified by the WHO as a significant endemic area for human Fasciola infection in Asia, is one of six countries facing serious challenges with fascioliasis. The infection is widespread among domestic animals in many parts of Iran, reaching up to 50% prevalence in some areas, with human cases reported in certain provinces, particularly in the north. Cases of human Fasciola infection have also been documented in other regions of the country (4). Zoonotic fascioliasis in Iran is influenced by factors such as the biology of the vector and parasite, livestock management practices, climatic conditions, drug resistance, food habits, ecological aspects of Fasciola transmission, the presence of an intermediate host, and free grazing by ruminants (7). Food habits, in particular, play a crucial role in determining the risk of fascioliasis. The consumption of wild watercress, which grows near livestock pasture areas, is implicated in most cases of sporadic transmission (5). The majority of sporadic Fasciola transmissions occur due to the consumption of wild watercress or other plants that grow near livestock pastures. Fascioliasis control strategies in endemic regions require fundamental information about various aspects of the disease, particularly its epidemiological patterns and transmission dynamics (8). Recent technological advances in Geographic Information Systems (GISs) have provided researchers with the ability to develop spatial and spatio-temporal risk maps for diseases (9). Geographic information system is invaluable for designing geographic distribution maps of disease prevalence, understanding factors that influence disease control and transmission, and identifying environmental variables that impact disease incidence (10).
2. Objectives
The primary objective of this research is to determine the incidence, spatial distribution, and hotspot regions of fascioliasis in Iran using GIS analysis for the period from 2013 to 2022.
3. Methods
3.1. Data Collection
Fascioliasis data from all provinces of Iran between 2013 and 2022 were obtained from the Ministry of Health (Disease Management Center), Tehran, Iran. This study was approved by the Research Ethics Committee of Kermanshah University of Medical Sciences (approval number: IR.KUMS.REC.1402.063). Meteorological data for the same 10-year period, including annual temperature and relative humidity for climatological terminals in 31 provinces, were acquired from the National Meteorological Organization.
The mean annual temperature and relative humidity for each province were calculated as follows: First, the average values for all weather stations within a province were determined, and then the mean of these averages across all stations within the province was computed. This process resulted in a single temperature value and a single humidity value for each province, which were used for subsequent analyses.
The normalized difference vegetation index (NDVI) layers for the month of May for the years 2013 to 2022 were calculated using Google Earth Engine software at a spatial resolution of one square kilometer per pixel. These layers were clipped in ArcMap based on provincial borders, and the mean NDVI value for each year and province was calculated. These derived NDVI values were utilized for the geographical weighted regression analysis in ArcMap 10.5.
The following formula was applied to calculate the incidence rate (per 100,000 population) for each province:
3.2. Spatial Analysis
The disease spatial database was constructed using ArcMap and included data on cases, patient age groups, locations, gender, and mortality across different age groups. Spatial distribution maps of the disease were generated for the period 2013 - 2022. Spatial analysis using ArcGIS 10.5 software, combined with the Hot Spot Analysis tool, was employed to identify disease hotspots across all provinces.
Hot Spot Analysis in ArcGIS is a suitable method for identifying provinces with significantly different disease incidences compared to others. This analysis provides Z-scores (both positive and negative) for each province, enabling the identification of significant disease hotspots and cold spots. A large positive Z-score indicates more critical points, whereas a higher negative Z-score indicates less critical points. The data points are classified into hot and cold spots at three confidence levels: 90%, 95%, and 99%, each associated with a specific Z-value (11).
Additionally, spatial analysis using geographically weighted regression (GWR) in ArcGIS 10.5 was conducted to explore the relationship between environmental variables (temperature, relative humidity, and NDVI density) and the incidence of fascioliasis from 2013 to 2022. Geographically weighted regression analysis allows for the modeling of spatially varying associations between the incidence of fascioliasis (dependent variable) and the average annual temperature, relative humidity, and NDVI (independent variables).
Statistical data analysis was performed using linear regression analysis and SPSS 21 software (Chicago, IL, USA), incorporating descriptive statistics tests.
4. Results
During the study period, 589 cases of fascioliasis were recorded in Iran. The incidence rate of human Fasciola infection is presented in Figure 1. The results revealed that the hotspots of the disease (areas with the highest incidence rates) were located in Gilan, Kermanshah, and Khorramabad provinces (Figures 2 and 3). Over the 10 years, the provinces of Gilan, Kermanshah, Khorramabad, Kurdistan, Semnan, and South Khorasan reported the highest rates of fascioliasis (Figures 4, 5, and 6).
The trend of human Fascioliasis during the years 2013 - 2022 in Iran
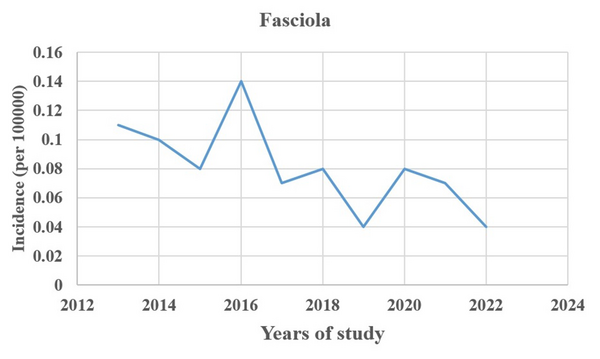
The Hotspot of fascioliasis in different provinces of Iran, 2013 to 2022
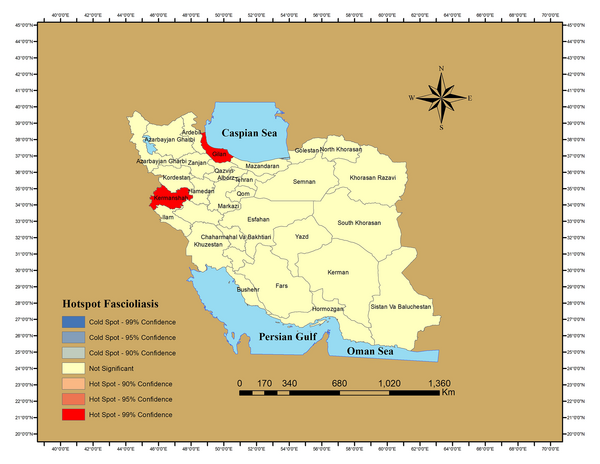
The fascioliasis Hotspot in different provinces of Iran, 2013 to 2022
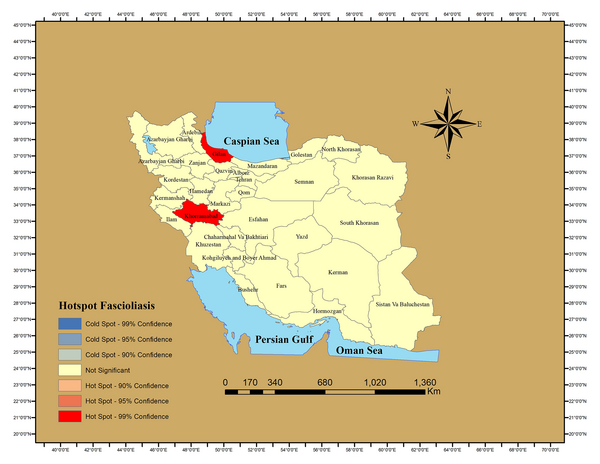
The results of geographically weighted regression analysis of the correlation between the relative humidity and the incidence of human fascioliasis in Iran, 2013 - 2022
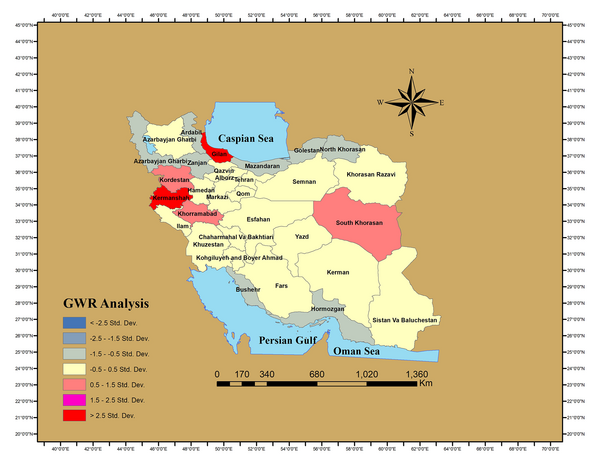
The results of geographically weighted regression analysis of the correlation between temperature and the incidence of human Fascioliasis in Iran, 2013 - 2022
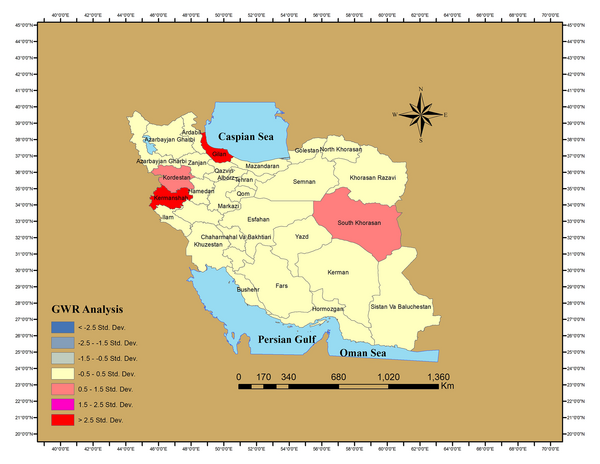
The results of geographically weighted regression analysis of the correlation between normalized difference vegetation index (NDVI) and the incidence of fascioliasis in Iran, 2013 to 2022
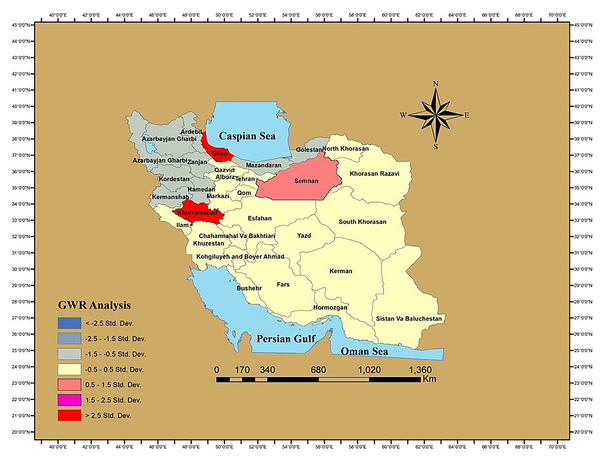
The GWR analysis results for the period 2013 to 2021 demonstrated a strong correlation between humidity (Figure 4), temperature (Figure 5), NDVI (Figure 6), and the incidence of Fasciola infection in the provinces of Gilan, Kermanshah, Khorramabad, Kurdistan, Semnan, and South Khorasan. Data analysis showed that in 2022, there were 28 new cases of fascioliasis (including both males and females), with an infection incidence rate of 0.04 per 100,000 individuals.
The relationship between the incidence of fascioliasis (per 100,000 individuals), NDVI, relative humidity (RH), and mean temperature (TM) was analyzed using linear regression analysis (Table 1). The results demonstrated a significant relationship between humidity and NDVI with the incidence of fascioliasis.
Relationship Between Environmental Variables and the Incidence of Human Fascioliasis in Iran, 2013 - 2022
Province | TM | RH | NDVI | Incidence/100000 |
---|---|---|---|---|
Alborz | 16.40039214 | 35.1 | 0.226 | 0.015 |
Ardabil | 9.268608143 | 69.3 | 0.386 | 0.024 |
West Azerbaijan | 13.45255068 | 58 | 0.351 | 0 |
East Azerbaijan | 12.74801269 | 49.95 | 0.325 | 0 |
Bushehr | 25.58515797 | 61.65 | 0.107 | 0 |
Chaharmahal and Bakhtiari | 12.47099015 | 44 | 0.266 | 0 |
Esfahan | 14.02026325 | 38.85 | 0.115 | 0.006 |
Fars | 17.68660851 | 41.45 | 0.154 | 0 |
Gilan | 15.83153861 | 76.05 | 0.564 | 1.688 |
Golestan | 18.53809255 | 67.9 | 0.404 | 0.006 |
Hamedan | 10.10541655 | 48.55 | 0.31 | 0 |
Hormozgan | 24.68627044 | 59.85 | 0.083 | 0 |
Ilam | 20.31200242 | 41.05 | 0.16 | 0 |
Kerman | 24.0960181 | 36.5 | 0.099 | 0 |
Kermanshah | 15.59402365 | 40.15 | 0.335 | 0.106 |
Khorasan Razavi | 15.07798998 | 47.1 | 0.145 | 0.008 |
Khuzestan | 25.06462137 | 47.3 | 0.139 | 0.006 |
Kordestan | 12.84783848 | 46.75 | 0.374 | 0.04 |
Markazi | 12.21402871 | 43.8 | 0.223 | 0.021 |
Mazandaran | 16.21690237 | 72.9 | 0.472 | 0.06 |
North Khorasan | 14.71020272 | 56.3 | 0.215 | 0 |
Qazvin | 15.22403958 | 52.55 | 0.282 | 0.008 |
Qom | 16.56578786 | 47.85 | 0.104 | 0 |
Semnan | 15.29186281 | 37.65 | 0.08 | 0.042 |
Sistan Va Baluchestan | 23.32389757 | 31.75 | 0.071 | 0.008 |
South Khorasan | 18.50197443 | 33.3 | 0.08 | 0.013 |
Tehran | 15.81778573 | 36.6 | 0.161 | 0.04 |
Yazd | 17.43841887 | 33.1 | 0.082 | 0 |
Zanjan | 12.75088759 | 53.85 | 0.278 | 0 |
Kohgiluyeh and Boyer Ahmad | 23. 07798998 | 40.65 | 0.218 | 0.03 |
Khorramabad | 19. 53809255 | 43.6 | 0.312 | 0.2 |
According to the findings, an increase of one unit in relative humidity caused an increase of 0.009 in the incidence of Fasciola infection (P-value = 0.027). Similarly, an increase of one NDVI unit resulted in a 1.186 increase in the incidence of fascioliasis (P-value = 0.025) (Table 2).
Results of the Univariate and Multiple Linear Regression Model for Human Fasciola Infection
Variables | Univariate | Multiple | ||
---|---|---|---|---|
Beta (SE) | P-Value | Beta (SE) | P-Value | |
TM | -0.003 (0.014) | 0.803 | 0.022 (0.015) | 0.152 |
RH | 0.009 (0.004) | 0.027 | -0.001 (0.006) | 0.888 |
NDVI | 1.186 (0.387) | 0.005 | 1.618 (0.677) | 0.025 |
5. Discussion
Geographic information system software plays a crucial role in epidemiological models for identifying risk factors involved in the transmission of fascioliasis and determining high-risk zones in previously unstudied areas (12). In this study, spatial analysis was utilized to estimate areas with high disease prevalence of fascioliasis. Interestingly, during 2013 - 2022, cold foci of the disease (provinces with significantly low disease incidence) were identified. The findings of this study align with the work of Malone et al. in the USA (13), which demonstrated that environmental factors such as air temperature, rainfall, and potential evapotranspiration are significantly related to the annual incidence of Fasciola infection in hosts. These environmental variables influence the free-living larval stages of eggs and metacercariae as well as snail (intermediate host) and parasite populations (14).
Moreover, Fuentes et al. applied GIS software to create a spatial and epidemiological model for mapping fascioliasis in the South American Andes. They categorized transmission risk into low, moderate, and high-risk areas, allowing them to pinpoint regions requiring targeted disease control measures (12). Notably, they employed modified climatic forecast indices (the MT and Wb-bs model) based on meteorological data from high-altitude and low-latitude endemic areas.
In our study, the GWR analysis showed a significant correlation between vegetation density, temperature, humidity, and the incidence of fascioliasis in Gilan, Kermanshah, Khorramabad, Kurdistan, Semnan, and South Khorasan (P-value = 0.025). Consequently, these provinces were identified as areas where fascioliasis infection is prevalent.
Regions with temperate climates, adequate rainfall, and favorable humidity provide optimal conditions for the parasite’s life cycle and the subsequent incidence of disease. Specifically, the provinces of Gilan, Kermanshah, Khorramabad, Kurdistan, Semnan, and South Khorasan exhibit relative humidity, average annual temperatures, and robust vegetation density, as confirmed through geographical weight regression analysis. These factors create an environment conducive to the breeding of the intermediate host, the survival of Fasciola larval stages, an effective parasite transmission cycle, and the contamination of both intermediate and final hosts (8, 15, 16). Consequently, fascioliasis incidence is notably higher in these areas.
The higher prevalence of Fasciola infection in these regions may also be attributed to the nomadic lifestyle of some populations, close contact with natural environments, the consumption of raw vegetables (particularly aquatic ones), and limited access to treated tap water (17). Infected ruminants act as major reservoirs for human infection due to their proximity to freshwater plants cultivated for human consumption. Furthermore, livestock breeding practices prevalent in these regions significantly influence the disease's transmission dynamics (16, 17).
Dietary habits play a significant role in the development of human Fasciola infection. Numerous wild aquatic and semi-aquatic plants have been identified as significant risk factors associated with human fascioliasis in Iran (18). These findings strongly suggest that while humidity and temperature are critical variables for the Fasciola parasite to complete its life cycle, other factors, such as vegetation density, also significantly affect fascioliasis prevalence and must be considered. In areas with varying environmental characteristics, diverse climate conditions significantly impact the development of snails as intermediate hosts for fascioliasis (19). Variables such as temperature, humidity, and vegetation density critically influence the survival of both the snail intermediate host and the larval stages of Fasciola trematodes.
Environmental temperatures above 10°C are essential for the development of the Fasciola parasite (miracidia and cercariae) and for snail reproduction. These stages are halted at temperatures below 5°C (19, 20). Optimal humidity conditions occur when rainfall exceeds transpiration, reaching saturation levels. These conditions are essential for miracidia to infect snails and for the dispersal of cercariae released from snails (20).
In our study, GWR analysis was employed to examine the correlation between temperature, relative humidity, and NDVI with the incidence of human fascioliasis. The results revealed a significant correlation between these variables and the incidence of fascioliasis in Gilan, Kermanshah, Khorramabad, Kurdistan, Semnan, and South Khorasan provinces (8, 15, 21).
The findings of Danson et al. further emphasized the value of GIS in studying human parasitic infections, providing enhanced insights into parasite transmission dynamics. Their research highlighted the impact of environmental factors such as temperature, humidity, and altitude on the distribution of worm infections (22), aligning with the findings of our study.
5.1. Conclusions
Geographic information system analysis can provide reliable predictions of Fasciola cases in endemic regions of Iran. Our study demonstrated a significant correlation between mean annual temperature, relative humidity, NDVI, and the incidence of fascioliasis in Iran. The data and results from this study can assist health policymakers in developing and sustaining prevention programs to address new cases. Accordingly, initiatives such as educating the population to avoid infection, screening participants who may act as reservoirs of fascioliasis, treating patients in endemic areas, and addressing environmental factors are crucial components of an effective control program.
Geographic information system, as an effective tool, is highly suitable for investigating and mapping the distribution of influencing factors in the health system. However, a limitation of our study was the presence of missing data during the data collection process.
References
-
1.
Davoodi L, Mizani A, Najafi-Vosough R, Hosseini Teshnizi S, Amouei A, Motavallihaghi S, et al. A Descriptive Study of Human Fascioliasis in Qaemshahr, Mazandaran Province, Iran: Its Prevalence and Risk Factors. Arch Clin Infect Dis. 2022;17(4). e123682. https://doi.org/10.5812/archcid-123682.
-
2.
Chetri PB, Shukla R, Tripathi T. Identification and characterization of cytosolic malate dehydrogenase from the liver fluke Fasciola gigantica. Sci Rep. 2020;10(1):13372. [PubMed ID: 32770017]. [PubMed Central ID: PMC7415141]. https://doi.org/10.1038/s41598-020-70202-y.
-
3.
Mirzadeh A, Jafarihaghighi F, Kazemirad E, Sabzevar SS, Tanipour MH, Ardjmand M. Recent Developments in Recombinant Proteins for Diagnosis of Human Fascioliasis. Acta Parasitol. 2021;66(1):13-25. [PubMed ID: 32974849]. https://doi.org/10.1007/s11686-020-00280-5.
-
4.
Sarkari B, Ghobakhloo N, Moshfea A, Eilami O. Seroprevalence of human fasciolosis in a new-emerging focus of fasciolosis in Yasuj district, southwest of Iran. Iran J Parasitol. 2012;7(2):15-20. [PubMed ID: 23109941]. [PubMed Central ID: PMC3469183].
-
5.
Caravedo MA, Cabada MM. Human Fascioliasis: Current Epidemiological Status and Strategies for Diagnosis, Treatment, and Control. Res Rep Trop Med. 2020;11:149-58. [PubMed ID: 33273878]. [PubMed Central ID: PMC7705270]. https://doi.org/10.2147/RRTM.S237461.
-
6.
Pozio E. How globalization and climate change could affect foodborne parasites. Exp Parasitol. 2020;208:107807. [PubMed ID: 31751558]. https://doi.org/10.1016/j.exppara.2019.107807.
-
7.
Khoramian H, Arbabi M, Mahami Osqoi M, Delavari M, Hooshyar H, Asgari M. Prevalence of ruminants fascioliasis and their economic effects in Kashan, center of Iran. Asian Pac J Trop Biomed. 2014;4(11):918-22. https://doi.org/10.12980/apjtb.4.2014apjtb-2014-0157.
-
8.
Ashrafi K. The Status of Human and Animal Fascioliasis in Iran: A Narrative Review Article. Iran J Parasitol. 2015;10(3):306-28. [PubMed ID: 26622287]. [PubMed Central ID: PMC4662732].
-
9.
Tsegaw T, Gadisa E, Seid A, Abera A, Teshome A, Mulugeta A, et al. Identification of environmental parameters and risk mapping of visceral leishmaniasis in Ethiopia by using geographical information systems and a statistical approach. Geospat Health. 2013;7(2):299-308. [PubMed ID: 23733292]. https://doi.org/10.4081/gh.2013.88.
-
10.
Parandin F, Bizhani N, Rahimi Esboei B, Feizi F, Soleymani E, Motavallihaghi SM, et al. Spatial modeling of visceral leishmaniasis in Iran from 2010 to 2018. Int J Health Sci. 2022;6(27):48780-95. https://doi.org/10.53730/ijhs.v6nS7.13658.
-
11.
Hanafi-Bojd AA, Chaharrahi Z. [Health Geography: Using GIS in Public Health Studies]. Vol. 1. Tehran: Entekhab; 2020. FA.
-
12.
Fuentes MV, Sainz-Elipe S, Nieto P, Malone JB, Mas-Coma S. Geographical Information Systems risk assessment models for zoonotic fascioliasis in the South American Andes region. Parassitologia. 2005;47(1):151-6. [PubMed ID: 16044684].
-
13.
Malone JB, Fehler DP, Loyacano AF, Zukowski SH. Use of LANDSAT MSS imagery and soil type in a geographic information system to assess site-specific risk of fascioliasis on Red River Basin farms in Louisiana. Ann N Y Acad Sci. 1992;653:389-97. [PubMed ID: 1626889]. https://doi.org/10.1111/j.1749-6632.1992.tb19667.x.
-
14.
Mas-Coma S, Valero MA, Bargues MD. Climate change effects on trematodiases, with emphasis on zoonotic fascioliasis and schistosomiasis. Vet Parasitol. 2009;163(4):264-80. [PubMed ID: 19375233]. https://doi.org/10.1016/j.vetpar.2009.03.024.
-
15.
Salahi-Moghaddam A, Arfaa F. Epidemiology of Human Fascioliasis Outbreaks in Iran. J Arch Mil Med. 2013;1(1):6-12. https://doi.org/10.5812/jamm.13890.
-
16.
Bozorgomid A, Nazari N, Kia EB, Mohebali M, Hajaran H, Hydarian P, et al. Epidemiology of Fascioliasis in Kermanshah Province, Western Iran. Iran J Public Health. 2018;47(7):967-72. [PubMed ID: 30181994]. [PubMed Central ID: PMC6119572].
-
17.
Zoghi S, Emami M, Shahriarirad S, Vahedi R, Cheraghi MR, Zamiri B, et al. Human fascioliasis in nomads: A population-based serosurvey in southwest Iran. Infez Med. 2019;27(1):68-72. [PubMed ID: 30882381].
-
18.
Meshgi B, Majidi-Rad M, Hanafi-Bojd AA, Fathi S. Ecological niche modeling for predicting the habitat suitability of fascioliasis based on maximum entropy model in southern Caspian Sea littoral, Iran. Acta Trop. 2019;198:105079. [PubMed ID: 31299282]. https://doi.org/10.1016/j.actatropica.2019.105079.
-
19.
Abdi J, Naserifar R, Rostami Nejad M, Mansouri V. New features of fascioliasis in human and animal infections in Ilam province, Western Iran. Gastroenterol Hepatol Bed Bench. 2013;6(3):152-5. [PubMed ID: 24834263]. [PubMed Central ID: PMC4017511].
-
20.
Khosravi A, Babaahmady E. Epidemiology of Fasciola Hepatica in Iran. Int J Biol. 2012;4(4):86-90. https://doi.org/10.5539/ijb.v4n4p86.
-
21.
Ashrafi K, Valero MA, Massoud J, Sobhani A, Solaymani-Mohammadi S, Conde P, et al. Plant-borne human contamination by fascioliasis. Am J Trop Med Hyg. 2006;75(2):295-302. [PubMed ID: 16896136].
-
22.
Danson FM, Bowyer P, Pleydell D, Craig P. Echinococcus multilocularis: The role of satellite remote sensing, GIS and spatial modelling. Southeast Asian J Trop Med Public Health. 2004;35(Suppl 1):189-93.