Abstract
Keywords
1. Background
The pacing strategy during an endurance event can have a significant impact on performance. Six different pacing strategies, such as negative, all-out, positive, even, parabolic-shaped and variable pacing, have been described (1). Generally, during endurance events, well trained athletes tend to adopt a positive pacing strategy, where the athlete progressively slows after peak speed is reached (1).
For four decades, pacing has been well studied in the other major endurance modes of exercise, such as running (2), cycling (3) and swimming (4). Aspects of pacing have preferably been investigated in running events of different distances in track-running from 800 m to 10,000 m (5-7) and in road running races of different distances such as 5 km (8), 10 km (9), half-marathon (10), marathon (11-15), 100-km ultra-marathon (16-19), 161-km ultra-marathon (20-22), running events of different durations such as 6-hour run (23), 24-hour run (24), and cross-country running (25, 26). However, in contrast to running, very little is known about pacing in cross-country skiing (27).
Different variables such as age, sex, performance level and experience seem to influence the pacing strategy of athletes in cross-country skiing (27, 28). In 10 km and 15 km cross-country skiing races held in World Cup, World Championships, and Olympic events, women and men demonstrated a positive pacing pattern with a decline in speed from the first to the last lap (28). In longer cross-country skiing races such as the ‘Vasaloppet’ covering a total distance of 90 km, women showed a more even pacing profile than men with the same finish time, start group, age, and race experience. Men were faster in the first half whereas women were faster in the second half of the race (27). Also, performance level has an influence on pacing in cross-country ski races. During 10 km and 15 km cross-country skiing races held in World Cup, World Championships, and Olympic Games, slower male skiers were characterized by a quick start relative to their average velocity, with a greater decrease in race speed during the race compared to the faster skiers (28).
Performance in cross-country skiing has been shown to be influenced by a series of physiological, psychological, technical and tactical characteristics, including pacing. In addition, pacing of performance groups has usually been examined by considering quartiles of performance (19, 29). Although the use of quartiles allowed the assignment of an equal number of participants in each performance group, it might be of limited practical value for coaches and athletes, because the cut-off points of groups did make sense from a statistical point of view but not from a sport perspective. Coaches and athletes might be more interested to know the pacing of a specific ‘race time’ group, i.e. what was the pacing strategy of athletes achieving a specific race time.
However, we have no knowledge whether differences between younger and older cross-country skiers in pacing exist. For 100-km ultra-marathoners, it has been shown that younger athletes (i.e. younger than 24 years) were slower than older athletes (i.e. older than 24 years) and older athletes did not slow down more than younger athletes (18). Therefore, the aim of this study was to investigate whether differences in pacing strategies do exist between younger and older cross-country skiers competing in a cross-country skiing race covering the distance of a marathon. We expected to find differences in pacing among skiers with similar performance and different ages due to the effect of aging on performance.
2. Methods
2.1. Ethical Approval
All procedures used in the study were approved by the institutional review board of Kanton St. Gallen, Switzerland, with a waiver of the requirement for informed consent of the participants since the study involved the analysis of publicly available data.
2.2. The Race
The ‘Engadin Ski Marathon’ is an annually held cross-country ski race in Switzerland, Europe that takes place on the second Sunday of March in the upper Engadin valley between Maloja and S-chanf. The race started in 1969, has been a part of the Worldloppet, and is one of the major cross-country skiing events in the Alps in Europe. Between 11,000 and 13,000 skiers participate annually in ‘Engadin Ski Marathon’. Since 1998, the total distance covered is 42 km. In that year, the race was extended by 2 km to match the distance of a full marathon. The ‘Engadin Ski Marathon’ is a freestyle race, but there are separate tracks for skiers practicing classic style for all but the narrowest parts of the race. Participation is open to anyone from the age of 16 years. In Silvaplana (10 km, 1,790 m above sea level), Pontresina (20 km, 1,805 m above sea level) and La Punt-Chamues-ch (35 km, 1,687 m above sea level), split times are taken at the time stations.
The race course of the ‘Engadin Ski Marathon’ is hilly in the first part (Figure 1). The race starts in Maloja at 1,820 m above sea level. The track leads over the frozen lakes in Lake Sils (1,796 m above sea level) and Lake Silvaplana (1,790 m above sea level). After passing St. Moritz (1,822 m above sea level) there is a forested climb in the Stazerwald. The proceeding descent to Pontresina (1,805 m above sea level) then leads to the runway of Samedan Airport (1,707 m above sea level) and afterwards on the right side of the Engadin valley, passing several small communities such as La Punt-Chamues-ch (1,687 m above sea level) and Zuoz (1,716 m above sea level) before reaching the finish in S-chanf at 1,670 m above sea level.
Course profile (30)
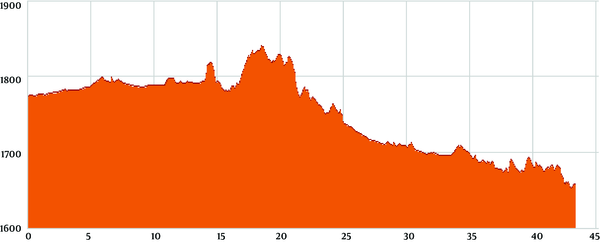
2.3. Data Sampling
All athletes who finished the ‘Engadin Ski Marathon’ between 1998 and 2016 were considered. Data with name, age and sex of the athletes were obtained from the publicly available race website of the ‘Engadin Skin Marathon’ at www.engadin-skimarathon.ch. Before 1998, data were not recorded electronically. The initial sample was 105,587 participants, classified in 5-year age groups. Twenty-two participants with missing split times were excluded from further analysis, resulting in a final sample of 105,565 participants.
2.4. Statistical Analysis
The statistical package IBM SPSS v.20.0 (SPSS, Chicago, USA) was used to perform all statistical analyses and the software GraphPad Prism v. 7.0 (GraphPad Software, San Diego, USA) to create figures. Descriptive statistics (mean ± standard deviation) were used for all variables. We created six performance groups according to race time (< 2 hours, 2 - 2.5 hours, 2.5 - 3, 3 - 3.5, 3.5 - 4.0, and > 4 hours) to allow an ‘absolute’ comparison among age groups, e.g. the athletes in age group 50 - 55 years with a race time 3 - 3.5 hours were compared with athletes in age group 20 - 25 years with the same race time. A two-way ANOVA examined the main effects of sex, age group, performance group and split, and the performance group × split and performance group × age group interaction on race speed, followed by a Bonferroni post-hoc analysis. The magnitude of differences in the ANOVA was evaluated using eta squared (η2) as trivial (η2 < 0.01), small (0.01 ≤ η2 < 0.06), moderate (0.06 ≤ η2 < 0.14) and large (η2 ≥ 0.14). We calculated three percentage changes of speed, change A (100 × (split2 - split1)/split1), change B (100 × (split3 - split2)/split2) and change C (100 × (split4 - split3)/split3). These changes refer to the time stations (i.e. Silvaplana = Change A, Pontresina = Change B and La Punt = Change C) during the race.
In addition, to study differences in speed and change of speed by sex, age group, performance group and split, we used a mixed-effects regression model with participants as random variables, whereas sex, performance group and age group were assigned as fixed variables. In addition, we examined interaction effects between these fixed variables. Akaike information criterion (AIC) was used to select the final model. Alpha level was set at 0.05.
3. Results
A sex × performance group association was observed (χ2 = 4147.22, P < 0.001, Cramer’s φ = 0.198) with the men-to-women ratio decreasing with increasing race time (Figure 2). For instance, the men-to-women ratio was 14.1 in the < 2 hours group and 2.5 in the > 4 hours group. An age group × performance group association was shown in women (χ2 = 1302.15, P < 0.001, Cramer’s φ = 0.259) and in men (χ2 = 8306.92, P < 0.001, Cramer’s φ = 0.310) with the faster performance groups being more prevalent in young age groups and the slower performance groups in older age groups (Figure 3). For instance, the < 2 hours group had 296 (out of 1,513, i.e. 19.6%) men in age group -20 years, but only 21 (out of 4,107, i.e. 0.5%) in the age group 65 - 70 years.
Distribution of performance groups by sex and men-to-women ratio (Δ)
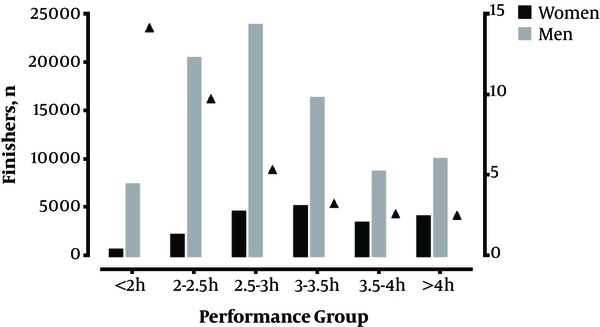
Distribution of performance groups by age group in women (A) and men (B)
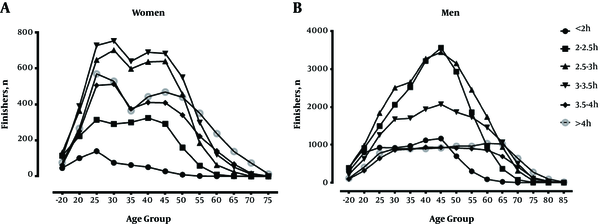
A moderate main effect of performance group on change A was observed (P < 0.001, η2 = 0.074) (Figure 4 and Table 1), where athletes in the < 2 hours group increased speed and athletes in the other groups decreased speed in a smaller (2 - 2.5 hours) or larger extent. A trivial main effect of sex on change A was shown (P < 0.001, η2 = 0.002) with women decreasing speed more than men. A trivial sex × performance group interaction on change A was found (P = 0.002, η2 < 0.001). Similarly, a moderate main effect of performance group on change B was observed, too (P < 0.001, η2 = 0.072), with change B presenting an inverse U shape across performance groups, i.e. 2.5 - 3 hours and 3 - 3.5 hours performance groups increased more their speed than the faster and slower performance groups. A small main effect of sex on change B was shown (P < 0.001, η2 = 0.010) with men presenting larger increase of speed than women. A trivial sex × performance group interaction on change B was found (P < 0.001, η2 = 0.001). In addition, a small main effect of performance group on change C was observed (P < 0.001, η2 = 0.034), with change C presenting a U shape across performance groups, i.e. athletes in the 2.5 - 3 hours and 3 - 3.5 hours performance groups decreased their speed more than athletes in the faster and slower performance groups. A trivial main effect of sex on change B was shown (P < 0.001, η2 = 0.008) with women presenting a larger decrease in speed than men. A trivial sex × performance group interaction on Change C was found (P < 0.001, η2 = 0.002).
Change of speed from split 1 to 2 (change A), from split 2 to 3 (change B) and from split 3 to 4 (change C) by sex and performance group. Error bars represented standard deviations.
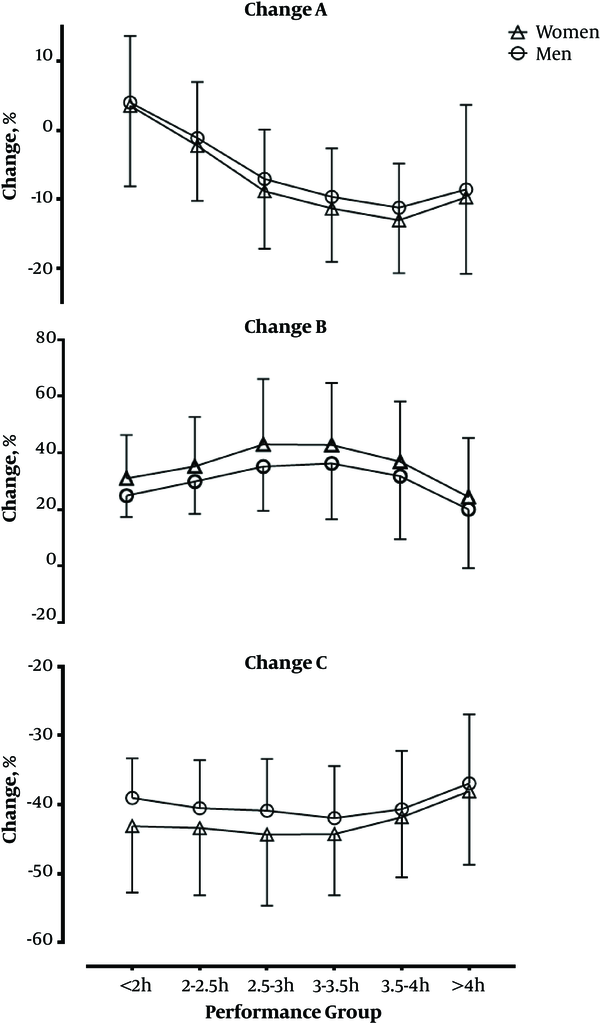
Mixed-Effects Regression Analysis
Parameter | C | SEE | P Value | Parameter | C | SEE | P Value |
---|---|---|---|---|---|---|---|
Total race time, s | Split 4 | ||||||
Intercept | 16675 | 30 | < 0.001 | Intercept | 6.73 | 0.04 | < 0.001 |
Performance group = < 2 h | -10014 | 87 | < 0.001 | Performance group = < 2 h | 9.11 | 0.11 | < 0.001 |
Performance group = 2 - 2.5 h | -8392 | 51 | < 0.001 | Performance group = 2 - 2.5 h | 6.17 | 0.06 | < 0.001 |
Performance group = 2.5 - 3 h | -6575 | 41 | < 0.001 | Performance group = 2.5 - 3 h | 3.76 | 0.05 | < 0.001 |
Performance group = 3 - 3.5 h | -4985 | 41 | < 0.001 | Performance group = 3 - 3.5 h | 2.48 | 0.05 | < 0.001 |
Performance group = 3.5 - 4 h | -3240 | 45 | < 0.001 | Performance group = 3.5 - 4 h | 1.48 | 0.06 | < 0.001 |
Performance group = > 4 h | 0b | 0 | Performance group = > 4 h | 0b | 0 | ||
Sex | 143 | 17 | < 0.001 | Sex | -0.05 | 0.02 | 0.037 |
[Performance group = < 2 h] × sex | -185 | 45 | < 0.001 | [Performance group = < 2 h] × sex | 0.84 | 0.06 | < 0.001 |
[Performance group = 2 - 2.5 h] × sex | -204 | 27 | < 0.001 | [Performance group = 2 - 2.5 h] × sex | 0.52 | 0.03 | < 0.001 |
[Performance group = 2.5 - 3 h] × sex | -248 | 23 | < 0.001 | [Performance group = 2.5 - 3 h] × sex | 0.50 | 0.03 | < 0.001 |
[Performance group = 3 - 3.5 h] × sex | -186 | 23 | < 0.001 | [Performance group = 3-3.5h] × sex | 0.21 | 0.03 | < 0.001 |
[Performance group = 3.5 - 4 h] × sex | -154 | 25 | < 0.001 | [Performance group = 3.5-4h] × sex | 0.05 | 0.03 | 0.117 |
[Performance group = > 4 h] × sex | 0b | 0 | [Performance group = > 4 h] × sex | 0b | 0 | ||
Split 1 | Change A | ||||||
Intercept | 10.04 | 0.04 | < 0.001 | Intercept | -10.86 | 0.33 | < 0.001 |
Performance group = < 2 h | 12.00 | 0.12 | < 0.001 | Performance group = < 2 h | 13.94 | 0.94 | < 0.001 |
Performance group = 2 - 2.5 h | 8.23 | 0.07 | < 0.001 | Performance group = 2 - 2.5 h | 7.50 | 0.55 | < 0.001 |
Performance group = 2.5 - 3 h | 5.47 | 0.06 | < 0.001 | Performance group = 2.5 - 3 h | 0.22 | 0.45 | 0.621 |
Performance group = 3 - 3.5 h | 3.59 | 0.06 | < 0.001 | Performance group = 3 - 3.5 h | -2.16 | 0.44 | < 0.001 |
Performance group = 3.5 - 4 h | 2.1 | 0.06 | < 0.001 | Performance group = 3.5 - 4 h | -4.01 | 0.49 | < 0.001 |
Performance group = > 4 h | 0b | 0 | Performance group = > 4 h | 0b | 0 | ||
Sex | -0.04 | 0.02 | 0.110 | Sex | 1.14 | 0.19 | < 0.001 |
[Performance group = < 2 h] × sex | 0.12 | 0.06 | 0.064 | [Performance group = < 2 h] × sex | -0.67 | 0.49 | 0.173 |
[Performance group = 2 - 2.5 h] × sex | 0.08 | 0.04 | 0.026 | [Performance group = 2 - 2.5 h] × sex | -0.02 | 0.30 | 0.936 |
[Performance group = 2.5 - 3 h] × sex | 0.11 | 0.03 | < 0.001 | [Performance group = 2.5 - 3 h] × sex | 0.65 | 0.49 | 0.009 |
[Performance group = 3 - 3.5 h] × sex | 0.06 | 0.03 | 0.056 | [Performance group = 3 - 3.5 h] × sex | 0.54 | 0.25 | 0.028 |
[Performance group = 3.5 - 4 h] × sex | 0.05 | 0.04 | 0.185 | [Performance group = 3.5 - 4 h] × sex | 0.68 | 0.28 | 0.013 |
[Performance group = > 4 h] × sex | 0b | 0 | [Performance group = > 4 h] × sex | 0b | 0 | ||
Split 2 | Change B | ||||||
Intercept | 8.84 | 0.04 | < 0.001 | Intercept | 28.84 | 0.58 | < 0.001 |
Performance group = < 2 h | 13.73 | 0.12 | < 0.001 | Performance group = < 2 h | 8.20 | 1.67 | < 0.001 |
Performance group = 2 - 2.5h | 8.76 | 0.07 | < 0.001 | Performance group = 2 - 2.5 h | 11.75 | 0.97 | < 0.001 |
Performance group = 2.5 - 3h | 4.98 | 0.06 | < 0.001 | Performance group = 2.5 - 3 h | 22.08 | 0.79 | < 0.001 |
Performance group = 3 - 3.5h | 2.97 | 0.06 | < 0.001 | Performance group = 3 - 3.5 h | 20.57 | 0.78 | < 0.001 |
Performance group = 3.5 - 4h | 1.49 | 0.06 | < 0.001 | Performance group = 3.5 - 4 h | 13.23 | 0.87 | < 0.001 |
Performance group = > 4 h | 0b | 0 | Performance group = > 4 h | 0b | 0 | ||
Sex | 0.07 | 0.02 | 0.003 | Sex | -4.41 | 0.33 | < 0.001 |
[Performance group = < 2 h] × sex | 0.15 | 0.06 | 0.021 | [Performance group = < 2 h] × sex | -1.65 | 0.87 | 0.056 |
[Performance group = 2 - 2.5h] × sex | 0.18 | 0.04 | < 0.001 | [Performance group = 2 - 2.5h] × sex | -0.96 | 0.52 | 0.066 |
[Performance group = 2.5 - 3 h] × sex | 0.26 | 0.03 | < 0.001 | [Performance group = 2.5 - 3 h] × sex | -3.47 | 0.44 | < 0.001 |
[Performance group = 3 - 3.5 h] × sex | 0.17 | 0.03 | < 0.001 | [Performance group = 3 - 3.5 h] × sex | -2.15 | 0.43 | < 0.001 |
[Performance group = 3.5 - 4 h] × sex | 0.14 | 0.04 | < 0.001 | [Performance group = 3.5 - 4 h] × sex | -0.75 | 0.49 | 0.122 |
[Performance group = > 4 h] × sex | 0b | 0 | < 0.001 | [Performance group = > 4 h] × sex | 0b | 0 | |
Split 3 | Change C | ||||||
Intercept | 11.32 | 0.07 | < 0.001 | Intercept | -39.23 | 0.27 | < 0.001 |
Performance group = < 2 h | 19.54 | 0.19 | < 0.001 | Performance group = < 2 h | -7.96 | 0.77 | < 0.001 |
Performance group = 2- 2.5 h | 13.31 | 0.11 | < 0.001 | Performance group = 2 - 2.5 h | -7.07 | 0.45 | < 0.001 |
Performance group = 2.5 - 3 h | 9.41 | 0.09 | < 0.001 | Performance group = 2.5 - 3 h | -8.55 | 0.37 | < 0.001 |
Performance group = 3 - 3.5 h | 6.24 | 0.09 | < 0.001 | Performance group = 3 - 3.5 h | -7.34 | 0.36 | < 0.001 |
Performance group = 3.5 - 4 h | 3.28 | 0.10 | < 0.001 | Performance group = 3.5 - 4 h | -3.76 | 0.40 | < 0.001 |
Performance group = > 4 h | 0b | 0.00 | Performance group = > 4 h | 0b | 0 | ||
Sex | -0.31 | 0.04 | < 0.001 | Sex | 1.15 | 0.15 | < 0.001 |
[Performance group = < 2 h] × sex | -0.79 | 0.10 | < 0.001 | [Performance group = < 2 h] × sex | 2.92 | 0.40 | < 0.001 |
[Performance group = 2 - 2.5 h] × sex | -0.29 | 0.06 | < 0.001 | [Performance group = 2 - 2.5 h] × sex | 1.74 | 0.24 | < 0.001 |
[Performance group = 2.5 - 3 h] × sex | -0.33 | 0.05 | < 0.001 | [Performance group = 2.5 - 3 h] × sex | 2.29 | 0.20 | < 0.001 |
[Performance group = 3 - 3.5 h] × sex | -0.16 | 0.05 | 0.001 | [Performance group = 3-3.5 h] × sex | 1.15 | 0.20 | < 0.001 |
[Performance group = 3.5 - 4 h] × sex | 0.02 | 0.06 | 0.656 | [Performance group = 3.5 - 4 h] × sex | 0.01 | 0.22 | 0.954 |
[Performance group = > 4 h] × sex | 0b | 0.00 | [Performance group = > 4 h] × sex | 0b | 0 |
In women, no main effect of age group on Change A (P = 0.150, η2 = 0.001), Change B (P = 0.109, η2 = 0.001) and Change C (P = 0.206, η2 = 0.001) was observed. However, a trivial performance group × age group interaction on these changes was shown (P < 0.001, η2 = 0.009; P < 0.001, η2 = 0.004; P = 0.002, η2 = 0.004, respectively) (Figure 5 and Table 2). In men, a trivial main effect of age group on Change A (P < 0.001, η2 = 0.002), Change B (P < 0.001, η2 = 0.001) and Change C (P < 0.001, η2 = 0.001) was found (Table 3). A trivial performance group × age group interaction on Change A (P < 0.001, η2 = 0.007), on Change B (P < 0.001, η2 = 0.003) and Change C (P < 0.001, η2 = 0.004) was observed.
Change of speed from split 1 to 2 (change A), from split 2 to 3 (change B) and from split 3 to 4 (change C) by sex, age group and performance group. Error bars represented standard error.
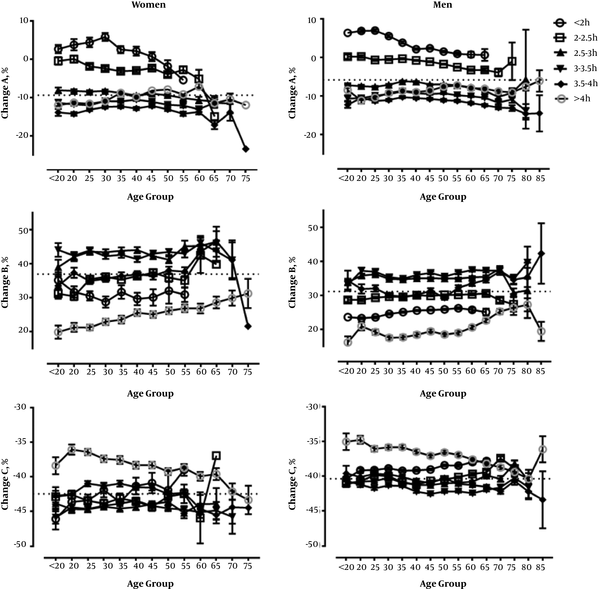
Mixed-Effects Regression Analysis in Women
Parameter | C | SEE | P Value | Parameter | C | SEE | P Value |
---|---|---|---|---|---|---|---|
Total race time, s | Split 4 | ||||||
Intercept | 16336 | 40 | < 0.001 | Intercept | 6.91 | 0.04 | < 0.001 |
Performance group = < 2 h | -9804 | 113 | < 0.001 | Performance group = < 2 h | 9.57 | 0.12 | < 0.001 |
Performance group = 2 - 2.5 h | -8221 | 69 | < 0.001 | Performance group = 2 - 2.5 h | 6.59 | 0.07 | < 0.001 |
Performance group = 2.5 - 3 h | -6368 | 57 | < 0.001 | Performance group = 2.5 - 3 h | 4.01 | 0.06 | < 0.001 |
Performance group = 3 - 3.5 h | -4701 | 55 | < 0.001 | Performance group = 3-3.5 h | 2.46 | 0.06 | < 0.001 |
Performance group = 3.5 - 4 h | -2945 | 60 | < 0.001 | Performance group = 3.5 - 4 h | 1.37 | 0.06 | < 0.001 |
Performance group = > 4 h | 0b | 0 | Performance group = > 4 h | 0b | 0 | ||
Age group | 81 | 6 | < 0.001 | Age group | -0.04 | 0.01 | < 0.001 |
[Performance group = < 2 h] × age group | -56 | 27 | 0.036 | [Performance group = < 2 h] × age group | 0.08 | 0.03 | 0.005 |
[Performance group = 2 - 2.5 h] × age group | -58 | 12 | < 0.001 | [Performance group = 2 - 2.5 h] × age group | 0.01 | 0.01 | 0.478 |
[Performance group = 2.5 - 3 h] × age group | -75 | 9 | < 0.001 | [Performance group = 2.5 - 3 h] × age group | 0.04 | 0.01 | < 0.001 |
[Performance group = 3 - 3.5 h] × age group | -78 | 9 | < 0.001 | [Performance group = 3 - 3.5 h] × age group | 0.04 | 0.01 | < 0.001 |
[Performance group = 3.5 - 4h] × Age group | -74 | 10 | < 0.001 | [Performance group = 3.5 - 4 h] × age group | 0.03 | 0.01 | 0.011 |
[Performance group = > 4 h] × age group | 0b | 0 | [Performance group = > 4 h] × age group | 0b | 0 | ||
Split 1 | Change A | ||||||
Intercept | 10.55 | 0.05 | < 0.001 | Intercept | -11.83 | 0.38 | < 0.001 |
Performance group = < 2 h | 11.75 | 0.13 | < 0.001 | Performance group = < 2 h | 17.15 | 1.07 | < 0.001 |
Performance group = 2 - 2.5 h | 7.96 | 0.08 | < 0.001 | Performance group = 2-2.5h | 11.61 | 0.66 | < 0.001 |
Performance group = 2.5 - 3h | 5.01 | 0.06 | < 0.001 | Performance group = 2.5 - 3 h | 4.21 | 0.54 | < 0.001 |
Performance group = 3 - 3.5 h | 3.12 | 0.06 | < 0.001 | Performance group = 3 - 3.5 h | 0.63 | 0.52 | 0.227 |
Performance group = 3.5 - 4h | 1.70 | 0.07 | < 0.001 | Performance group = 3.5 - 4 h | -0.77 | 0.57 | 0.175 |
Performance group = > 4 h | 0b | 0 | Performance group = > 4 h | 0b | 0 | ||
Age group | -0.09 | 0.01 | < 0.001 | Age group | 0.35 | 0.06 | < 0.001 |
[Performance group = < 2 h] × age group | 0.04 | 0.03 | 0.181 | [Performance group = < 2 h] × age group | -0.84 | 0.25 | 0.001 |
[Performance group = 2 - 2.5 h] × age group | 0.05 | 0.01 | < 0.001 | [Performance group = 2-2.5h] × age group | -0.77 | 0.12 | < 0.001 |
[Performance group = 2.5 - 3 h] × Age group | 0.10 | 0.01 | < 0.001 | [Performance group = 2.5 - 3 h] × age group | -0.59 | 0.09 | < 0.001 |
[Performance group = 3 - 3.5 h] × age group | 0.09 | 0.01 | < 0.001 | [Performance group = 3 - 3.5 h] × age group | -0.38 | 0.08 | < 0.001 |
[Performance group = 3.5 - 4 h] × age group | 0.08 | 0.01 | < 0.001 | [Performance group = 3.5 - 4 h] × age group | -0.43 | 0.09 | < 0.001 |
[Performance group = > 4 h] × age group | 0b | 0 | [Performance group = > 4 h] × age group | 0b | 0 | ||
Split 2 | Change B | ||||||
Intercept | 9.19 | 0.04 | < 0.001 | Intercept | 19.36 | 0.81 | < 0.001 |
Performance group = < 2 h | 14.10 | 0.12 | < 0.001 | Performance group = < 2 h | 13.40 | 2.29 | < 0.001 |
Performance group = 2 - 2.5 h | 9.19 | 0.07 | < 0.001 | Performance group = 2 - 2.5 h | 12.31 | 1.41 | < 0.001 |
Performance group = 2.5 - 3 h | 5.12 | 0.06 | < 0.001 | Performance group = 2.5 – 3 h | 23.15 | 1.15 | < 0.001 |
Performance group = 3 - 3.5 h | 2.90 | 0.06 | < 0.001 | Performance group = 3 - 3.5 h | 23.21 | 1.12 | < 0.001 |
Performance group = 3.5 – 4 h | 1.48 | 0.06 | < 0.001 | Performance group = 3.5 – 4 h | 13.83 | 1.22 | < 0.001 |
Performance group = > 4 h | 0b | 0 | Performance group = > 4 h | 0b | 0 | ||
Age group | -0.05 | 0.01 | < 0.001 | Age group | 0.84 | 0.12 | < 0.001 |
[Performance group = < 2 h] × age group | -0.09 | 0.03 | 0.001 | [Performance group = < 2 h] × age group | -1.34 | 0.54 | 0.014 |
[Performance group = 2 - 2.5 h] × age group | -0.06 | 0.01 | < 0.001 | [Performance group = 2 - 2.5 h] × age group | -0.11 | 0.25 | 0.665 |
[Performance group = 2.5 - 3 h] × age group | 0.02 | 0.01 | 0.115 | [Performance group = 2.5 – 3 h] × age group | -0.74 | 0.19 | < 0.001 |
[Performance group = 3 - 3.5 h] × age group | 0.04 | 0.01 | < 0.001 | [Performance group = 3 - 3.5 h] × age group | -0.80 | 0.18 | < 0.001 |
[Performance group = 3.5 - 4 h] × age group | 0.02 | 0.01 | 0.020 | [Performance group = 3.5 - 4 h] × age group | -0.18 | 0.19 | 0.364 |
[Performance group = > 4 h] × age group | 0b | 0 | [Performance group = > 4 h] × age group | 0b | 0 | ||
Split 3 | Change C | ||||||
Intercept | 10.93 | 0.09 | < 0.001 | Intercept | -35.52 | 0.37 | < 0.001 |
Performance group = < 2 h | 19.86 | 0.25 | < 0.001 | Performance group = < 2 h | -9.80 | 1.04 | < 0.001 |
Performance group = 2 - 2.5 h | 13.11 | 0.15 | < 0.001 | Performance group = 2 - 2.5 h | -7.46 | 0.64 | < 0.001 |
Performance group = 2.5 - 3 h | 9.28 | 0.12 | < 0.001 | Performance group = 2.5 - 3 h | -9.19 | 0.52 | < 0.001 |
Performance group = 3 - 3.5 h | 6.18 | 0.12 | < 0.001 | Performance group = 3 - 3.5 h | -8.77 | 0.51 | < 0.001 |
Performance group = 3.5 - 4 h | 3.17 | 0.13 | < 0.001 | Performance group = 3.5 - 4 h | -4.97 | 0.55 | < 0.001 |
Performance group = > 4 h | 0b | 0 | Performance group = > 4 h | 0b | 0 | ||
Age group | 0.01 | 0.01 | 0.311 | Age group | -0.43 | 0.06 | < 0.001 |
[Performance group = < 2 h] × age group | -0.30 | 0.06 | < 0.001 | [Performance group = < 2 h] × age group | 1.03 | 0.25 | < 0.001 |
[Performance group = 2 - 2.5 h] × age group | -0.02 | 0.03 | 0.558 | [Performance group = 2 - 2.5 h] × age group | 0.34 | 0.11 | 0.003 |
[Performance group = 2.5 - 3 h] × age group | -0.04 | 0.02 | 0.080 | [Performance group = 2.5 - 3 h] × age group | 0.50 | 0.09 | < 0.001 |
[Performance group = 3 - 3.5 h] × age group | -0.02 | 0.02 | 0.360 | [Performance group = 3 - 3.5 h] × age group | 0.43 | 0.08 | < 0.001 |
[Performance group = 3.5 - 4 h] × age group | 0.03 | 0.02 | 0.209 | [Performance group = 3.5 - 4 h] × age group | 0.19 | 0.09 | 0.035 |
[Performance group = > 4 h] × age group | 0b | 0 | [Performance group = > 4 h] × age group | 0b | 0 |
Mixed-Effects Regression Analysis in Men
Parameter | C | SEE | P Value | Parameter | C | SEE | P Value |
---|---|---|---|---|---|---|---|
Total race time, s | Split 4 | ||||||
Intercept | 16605 | 23 | < 0.001 | Intercept | 6.83 | 0.03 | < 0.001 |
Performance group = < 2 h | -10324 | 34 | < 0.001 | Performance group = < 2 h | 11.34 | 0.05 | < 0.001 |
Performance group = 2 - 2.5 h | -8572 | 29 | < 0.001 | Performance group = 2 - 2.5 h | 7.18 | 0.04 | < 0.001 |
Performance group = 2.5 - 3 h | -6747 | 27 | < 0.001 | Performance group = 2.5 - 3h | 4.62 | 0.04 | < 0.001 |
Performance group = 3 - 3.5 h | -5037 | 29 | < 0.001 | Performance group = 3 - 3.5 h | 2.79 | 0.04 | < 0.001 |
Performance group = 3.5 - 4 h | -3232 | 34 | < 0.001 | Performance group = 3.5 - 4 h | 1.48 | 0.05 | < 0.001 |
Performance group = > 4 h | 0b | 0 | Performance group = > 4 h | 0b | 0 | ||
Age group | 48 | 3 | < 0.001 | Age group | -0.03 | < 0.01 | < 0.001 |
[Performance group = < 2 h] × age group | 10 | 5 | < 0.001 | [Performance group = < 2 h] × age group | -0.12 | 0.01 | < 0.001 |
[Performance group = 2 - 2.5 h] × age group | -27 | 4 | 0.070 | [Performance group = 2 - 2.5 h] × age group | < 0.01 | 0.01 | 0.741 |
[Performance group = 2.5 - 3 h] × age group | -43 | 4 | < 0.001 | [Performance group = 2.5 - 3 h] × age group | 0.02 | < 0.01 | < 0.001 |
[Performance group = 3 - 3.5 h] × age group | -43 | 4 | < 0.001 | [Performance group = 3 - 3.5 h] × age group | 0.01 | 0.01 | 0.004 |
[Performance group = 3.5 - 4 h] × age group | -42 | 4 | < 0.001 | [Performance group = 3.5 - 4 h] × age group | 0.01 | 0.01 | 0.030 |
[Performance group = > 4 h] × age group | 0b | 0 | [Performance group = > 4 h] × age group | 0b | 0 | ||
Split 1 | Change A | ||||||
Intercept | 10.43 | 0.03 | < 0.001 | Intercept | -10.08 | 0.25 | < 0.001 |
Performance group = < 2 h | 12.42 | 0.05 | < 0.001 | Performance group = < 2 h | 18.80 | 0.39 | < 0.001 |
Performance group = 2 - 2.5 h | 8.07 | 0.04 | < 0.001 | Performance group = 2 - 2.5 h | 11.19 | 0.32 | < 0.001 |
Performance group = 2.5 - 3 h | 5.25 | 0.04 | < 0.001 | Performance group = 2.5 - 3 h | 3.72 | 0.31 | < 0.001 |
Performance group = 3 - 3.5 h | 3.27 | 0.04 | < 0.001 | Performance group = 3 - 3.5 h | 1.01 | 0.33 | 0.002 |
Performance group = 3.5 - 4 h | 1.77 | 0.05 | < 0.001 | Performance group = 3.5 - 4 h | 0.20 | 0.38 | 0.593 |
Performance group = > 4 h | 0b | 0 | Performance group = > 4 h | 0b | 0 | ||
Age group | -0.06 | < 0.01 | < 0.001 | Age group | 0.20 | 0.03 | < 0.001 |
[Performance group = < 2 h] × age group | -0.07 | 0.01 | < 0.001 | [Performance group = < 2 h] × age group | -1.12 | 0.06 | < 0.001 |
[Performance group = 2 - 2.5 h] × age group | 0.04 | 0.01 | < 0.001 | [Performance group = 2 - 2.5 h] × age group | -0.56 | 0.04 | < 0.001 |
[Performance group = 2.5 - 3h] × age group | 0.06 | 0.01 | < 0.001 | [Performance group = 2.5 - 3 h] × age group | -0.31 | 0.04 | < 0.001 |
[Performance group = 3 - 3.5 h] × age group | 0.06 | 0.01 | < 0.001 | [Performance group = 3 - 3.5 h] × age group | -0.29 | 0.04 | < 0.001 |
[Performance group = 3.5 - 4 h] × age group | 0.06 | 0.01 | < 0.001 | [Performance group = 3.5 - 4 h] × age group | -0.39 | 0.05 | < 0.001 |
[Performance group = > 4 h] × age group | 0b | 0 | [Performance group = > 4 h] × age group | 0b | 0 | ||
Split 2 | Change B | ||||||
Intercept | 9.24 | 0.03 | < 0.001 | Intercept | 15.76 | 0.43 | < 0.001 |
Performance group = < 2 h | 15.44 | 0.05 | < 0.001 | Performance group = < 2 h | 7.05 | 0.65 | < 0.001 |
Performance group = 2 - 2.5 h | 9.39 | 0.04 | < 0.001 | Performance group = 2 - 2.5 h | 13.12 | 0.54 | < 0.001 |
Performance group = 2.5 - 3 h | 5.35 | 0.04 | < 0.001 | Performance group = 2.5-3 h | 19.39 | 0.52 | < 0.001 |
Performance group = 3 - 3.5h | 3.15 | 0.04 | < 0.001 | Performance group = 3 - 3.5 h | 19.45 | 0.55 | < 0.001 |
Performance group = 3.5 - 4 h | 1.69 | 0.05 | < 0.001 | Performance group = 3.5 - 4 h | 12.83 | 0.64 | < 0.001 |
Performance group = > 4 h | 0b | 0 | Performance group = > 4 h | 0b | 0 | ||
Age group | -0.04 | < 0.01 | < 0.001 | Age group | 0.57 | 0.05 | < 0.001 |
[Performance group = < 2 h] × age group | -0.29 | 0.01 | < 0.001 | [Performance group = < 2 h] × age group | -0.16 | 0.10 | 0.109 |
[Performance group = 2 - 2.5 h] × age group | -0.05 | 0.01 | < 0.001 | [Performance group = 2 - 2.5 h] × age group | -0.41 | 0.07 | < 0.001 |
[Performance group = 2.5 - 3 h] × age group | 0.02 | 0.01 | < 0.001 | [Performance group = 2.5 - 3 h] × age group | -0.57 | 0.07 | < 0.001 |
[Performance group = 3 - 3.5h] × age group | 0.02 | 0.01 | < 0.001 | [Performance group = 3 - 3.5 h] × age group | -0.42 | 0.07 | < 0.001 |
[Performance group = 3.5 - 4 h] × age group | 0.01 | 0.01 | 0.094 | [Performance group = 3.5 – 4 h] × age group | -0.13 | 0.08 | 0.107 |
[Performance group = > 4 h] × age group | 0b | 0 | [Performance group = > 4 h] × age group | 0b | 0 | ||
Split 3 | Change C | ||||||
Intercept | 10.61 | 0.05 | < 0.001 | Intercept | -34.47 | 0.20 | < 0.001 |
Performance group = < 2 h | 19.59 | 0.08 | < 0.001 | Performance group = < 2 h | -5.17 | 0.30 | < 0.001 |
Performance group = 2 - 2.5 h | 13.27 | 0.06 | < 0.001 | Performance group = 2 - 2.5 h | -6.38 | 0.25 | < 0.001 |
Performance group = 2.5 – 3 h | 8.95 | 0.06 | < 0.001 | Performance group = 2.5 - 3 h | -6.44 | 0.24 | < 0.001 |
Performance group = 3 - 3.5 h | 5.99 | 0.06 | < 0.001 | Performance group = 3 - 3.5 h | -6.88 | 0.25 | < 0.001 |
Performance group = 3.5 - 4 h | 3.29 | 0.07 | < 0.001 | Performance group = 3.5 - 4 h | -4.70 | 0.29 | < 0.001 |
Performance group = > 4 h | 0b | 0 | Performance group = > 4 h | 0b | 0 | ||
Age group | 0.01 | 0.01 | 0.052 | Age group | -0.33 | 0.02 | < 0.001 |
[Performance group = < 2 h] × age group | -0.31 | 0.01 | < 0.001 | [Performance group = < 2 h] × age group | 0.45 | 0.05 | < 0.001 |
[Performance group = 2 - 2.5 h] × age group | -0.08 | 0.01 | < 0.001 | [Performance group = 2 - 2.5 h] × age group | 0.38 | 0.03 | < 0.001 |
[Performance group = 2.5 - 3 h] × age group | -0.03 | 0.01 | < 0.001 | [Performance group = 2.5-3h] × age group | 0.33 | 0.03 | < 0.001 |
[Performance group = 3 - 3.5 h] × age group | -0.01 | 0.01 | 0.273 | [Performance group = 3-3.5 h] × age group | 0.24 | 0.03 | < 0.001 |
[Performance group = 3.5 – 4 h] × age group | 0.01 | 0.01 | 0.489 | [Performance group = 3.5 - 4 h] × age group | 0.12 | 0.04 | 0.001 |
[Performance group = > 4 h] × age group | 0b | 0 | [Performance group = > 4 h] × age group | 0b | 0 |
4. Discussion
The main findings of the present study were that (i) the men-to-women ratio was lower in slower performance groups, (ii) in both women and men, the faster performance groups were more prevalent in younger age groups and the slower performance groups in older age groups, (iii) only athletes in the < 2 hours performance group increased speed in change A, (iv) a non-linear variation of change of speed was observed across performance groups in change B and C, and (v) the age group × performance group interaction on pacing was trivial.
Younger athletes and men were relatively faster than older athletes and women.
The first main finding was that there was an imbalance in the distribution of sex among performance groups, e.g. the men-to-women ratio was close to 14 in the < 2 hours performance group and close to 2.5 in the > 4 hours performance group. This observation should be attributed to the sex difference in performance in cross-country skiing, according to which men were faster than women. In turn, the faster race time in men might reflect physiological and anthropometric differences compared to women, such as their higher maximal oxygen uptake (VO2max) (31, 32), muscle power and body mass (33). It should be highlighted that VO2max was a parameter that could discriminate cross-country skiers into medallists and non-medallists in Olympic games and world championships (34). Also, lean mass was related to performance of cross-country skiers (35).
In addition, we observed a performance group × age group association in both women and men, with a larger magnitude in the latter, where the faster performance groups were more prevalent in younger age groups and the slower performance groups more in the older age groups. This remark might reflect age-related differences in race time, according to which athletes in younger age groups were faster than athletes in older age groups (36). The decline in performance with aging could be attributed to the drop in performance-related parameters, such as VO2max, which has been shown to be ~10% per decade (37).
With regards to the larger magnitude of performance group × age group association in men than in women, this observation should be attributed partially to sex differences in the correlation of performance to their changes with aging. For instance, in male adolescent skiers, skiing performance was related to strength and power parameters, whereas in female skiers it was related to aerobic capacity (38). Also, it has been shown that women relied less on anaerobic metabolism than men (39).
4.1. The Fastest Increased Speed in the First Part of the Race
The overall pattern of pacing (i.e. ~6% decrease of speed in Change A, ~32% increase in Change B and ~41% decrease in change C) denoted an inverse U shape. The most striking pattern was that of athletes in the < 2 hours performance group in change A which was the only group increasing speed in this change. The pattern of pacing in the present study disagreed with a previous study in cross-country skiing which showed a positive pacing (28, 40). This discrepancy might be due to different race distance and statistical approaches in the abovementioned studies, which examined 15 km and 10 km races in men and women, respectively, and considered only the 40 fastest skiers. Another study on a small sample in skiers examined pacing during a 10 km race and found a decrease of speed during the second and third quarter of the race, which was maintained in the last quarter (41).
4.2. Variation of Change of Speed Across Performance Groups in the Last Part of the Race
Moreover, a non-linear variation of change of speed was observed across performance groups in change B and C. For instance, the fastest group seemed to have similar patterns of change of speed as the two slowest groups. However, their similarity should be due to different reasons. In the case of the fastest group this should be attributed to their relatively fast beginning and the accompanying fatigue that did not allow increasing speed in change B as much as slower groups. On the other hand, the slowest groups did not increase speed in change B as much as faster groups due their slow speed. Additionally, it might be contributed to the course profile with more ascents in the first part of the race (change A and B) but more descents in the second part of the race (change C).
4.3. Differences in Variation of Pacing by Age Groups
The last major finding was that the differences in variation of pacing by performance groups among age groups were trivial. This finding practically meant that skiers with a similar performance differing for age adopted similar pacing strategies. We would expect to find differences in pacing among skiers with similar performance and different age due to the effect of aging on performance-related anthropometric characteristics and physiological parameters. On the other hand, what we did not know was the distribution of performance groups by age groups which might influence our findings. For instance, most men of the age group 25 - 29 years were classified in the 2.5 - 3 hours performance group, whereas relatively very few athletes belonged to this performance group in the age group of 70 - 74 years. Thus, it is reasonable to assume that those athletes belonging to this performance group have an average performance and should have average physiological characteristics in age group 25 - 29 years. However, they have a high performance and should have ‘exceptional’ physiological characteristics in age group 70 - 74 years. Although previous research showed a decline in VO2max of skiers with aging, this decline could be attenuated by increasing exercise stimulus (37). Moreover, older skiers might possess superior skiing economy and gross efficiency than younger skiers (42) which might offset any physiological disadvantage.
4.4. Limitations, Strengths and Practical Applications
The findings of this study should be limited by the specific characteristics of ‘Engadin Ski Marathon’ (e.g. race distance, change of altitude), environmental conditions (temperature, humidity and wind) and our statistical approach that used all skiers with no limitation to the top athletes in age or performance group. Thus, the results should be interpreted with caution when comparing with studies on other cross-country skiing races and using a different statistical design. On the other hand, the strength was the large number (n > 100,000) of finishers examined which allowed the analysis of performance groups by age groups and the identification even of trivial differences. Moreover, profiling pacing strategies by sex, performance group and age group, had important practical applications to coaches and athletes, who could optimize their pacing according to the data corresponding to their sex-, age- and performance-specific characteristics. Based on our findings, future research should examine the differences in anthropometric and physiological characteristics in finishers with similar race time differing in age.
4.5. Conclusions
In summary, the findings did not confirm our hypothesis that skiers of different age groups with similar race time would pace differently. In addition, we confirmed previous findings about the effects of sex and performance on pacing. Therefore, sex and performance should be the main criteria for coaches and skiers in order to optimize their pacing strategy.
References
-
1.
Abbiss CR, Laursen PB. Describing and understanding pacing strategies during athletic competition. Sports Med. 2008;38(3):239-52. [PubMed ID: 18278984].
-
2.
Leger LA, Ferguson RJ. Effect of pacing on oxygen uptake and peak lactate for a mile run. Eur J Appl Physiol Occup Physiol. 1974;32(3):251-7. [PubMed ID: 4836732].
-
3.
Katch V, Weltman A, Traeger L. All-out versus a steady-paced cycling strategy for maximal work output of short duration. Res Q. 1976;47(2):164-8. [PubMed ID: 1067630].
-
4.
Robertson E, Pyne D, Hopkins W, Anson J. Analysis of lap times in international swimming competitions. J Sports Sci. 2009;27(4):387-95. [PubMed ID: 19214862]. https://doi.org/10.1080/02640410802641400.
-
5.
Aragon S, Lapresa D, Arana J, Anguera MT, Garzon B. Tactical behaviour of winning athletes in major championship 1500-m and 5000-m track finals. Eur J Sport Sci. 2016;16(3):279-86. [PubMed ID: 25665767]. https://doi.org/10.1080/17461391.2015.1009494.
-
6.
Thiel C, Foster C, Banzer W, De Koning J. Pacing in Olympic track races: competitive tactics versus best performance strategy. J Sports Sci. 2012;30(11):1107-15. [PubMed ID: 22738897]. https://doi.org/10.1080/02640414.2012.701759.
-
7.
Tucker R, Lambert MI, Noakes TD. An analysis of pacing strategies during men's world-record performances in track athletics. Int J Sports Physiol Perform. 2006;1(3):233-45. [PubMed ID: 19116437].
-
8.
Deaner RO, Lowen A. Males and Females Pace Differently in High School Cross-Country Races. J Strength Cond Res. 2016;30(11):2991-7. [PubMed ID: 26950352]. https://doi.org/10.1519/JSC.0000000000001407.
-
9.
Lima-Silva AE, Bertuzzi RC, Pires FO, Barros RV, Gagliardi JF, Hammond J, et al. Effect of performance level on pacing strategy during a 10-km running race. Eur J Appl Physiol. 2010;108(5):1045-53. [PubMed ID: 20012450]. https://doi.org/10.1007/s00421-009-1300-6.
-
10.
Hanley B. Pacing profiles and pack running at the IAAF World Half Marathon Championships. J Sports Sci. 2015;33(11):1189-95. [PubMed ID: 25483017]. https://doi.org/10.1080/02640414.2014.988742.
-
11.
Deaner RO, Addona V, Carter RE, Joyner MJ, Hunter SK. Fast men slow more than fast women in a 10 kilometer road race. PeerJ. 2016;4. e2235. [PubMed ID: 27547544]. [PubMed Central ID: PMC4963220]. https://doi.org/10.7717/peerj.2235.
-
12.
Haney TJ, Mercer JA. A Description of Variability of Pacing in Marathon Distance Running. Int J Exerc Sci. 2011;4(2):133-40. [PubMed ID: 27182360]. [PubMed Central ID: PMC4738997].
-
13.
Hanley B. Pacing, packing and sex-based differences in Olympic and IAAF World Championship marathons. J Sports Sci. 2016;34(17):1675-81. [PubMed ID: 26736042]. https://doi.org/10.1080/02640414.2015.1132841.
-
14.
March DS, Vanderburgh PM, Titlebaum PJ, Hoops ML. Age, sex, and finish time as determinants of pacing in the marathon. J Strength Cond Res. 2011;25(2):386-91. [PubMed ID: 20224445]. https://doi.org/10.1519/JSC.0b013e3181bffd0f.
-
15.
Santos-Lozano A, Collado PS, Foster C, Lucia A, Garatachea N. Influence of sex and level on marathon pacing strategy. Insights from the New York City race. Int J Sports Med. 2014;35(11):933-8. [PubMed ID: 24886929]. https://doi.org/10.1055/s-0034-1367048.
-
16.
Knechtle B, Rosemann T, Zingg MA, Stiefel M, Rust CA. Pacing strategy in male elite and age group 100 km ultra-marathoners. Open Access J Sports Med. 2015;6:71-80. [PubMed ID: 25848325]. [PubMed Central ID: PMC4376307]. https://doi.org/10.2147/OAJSM.S79568.
-
17.
Lambert MI, Dugas JP, Kirkman MC, Mokone GG, Waldeck MR. Changes in Running Speeds in a 100 KM Ultra-Marathon Race. J Sports Sci Med. 2004;3(3):167-73. [PubMed ID: 24482594]. [PubMed Central ID: PMC3905299].
-
18.
Rust CA, Rosemann T, Zingg MA, Knechtle B. Do non-elite older runners slow down more than younger runners in a 100 km ultra-marathon? BMC Sports Sci Med Rehabil. 2015;7:1. [PubMed ID: 25973205]. [PubMed Central ID: PMC4430021]. https://doi.org/10.1186/2052-1847-7-1.
-
19.
Renfree A, Crivoi do Carmo E, Martin L. The influence of performance level, age and gender on pacing strategy during a 100-km ultramarathon. Eur J Sport Sci. 2016;16(4):409-15. [PubMed ID: 26034882]. https://doi.org/10.1080/17461391.2015.1041061.
-
20.
Hoffman MD. Pacing by winners of a 161-km mountain ultramarathon. Int J Sports Physiol Perform. 2014;9(6):1054-6. [PubMed ID: 24664982]. https://doi.org/10.1123/ijspp.2013-0556.
-
21.
Parise CA, Hoffman MD. Influence of temperature and performance level on pacing a 161 km trail ultramarathon. Int J Sports Physiol Perform. 2011;6(2):243-51. [PubMed ID: 21725109].
-
22.
Tan PL, Tan FH, Bosch AN. Similarities and Differences in Pacing Patterns in a 161-km and 101-km Ultra-Distance Road Race. J Strength Cond Res. 2016;30(8):2145-55. [PubMed ID: 26808845]. https://doi.org/10.1519/JSC.0000000000001326.
-
23.
Giovanelli N, Taboga P, Lazzer S. Changes in Running Mechanics During a 6-Hour Running Race. Int J Sports Physiol Perform. 2017;12(5):642-7. [PubMed ID: 27768506]. https://doi.org/10.1123/ijspp.2016-0135.
-
24.
Bossi AH, Matta GG, Millet GY, Lima P, Pertence LC, de Lima JP, et al. Pacing Strategy During 24-Hour Ultramarathon-Distance Running. Int J Sports Physiol Perform. 2017;12(5):590-6. [PubMed ID: 27618658]. https://doi.org/10.1123/ijspp.2016-0237.
-
25.
Esteve-Lanao J, Larumbe-Zabala E, Dabab A, Alcocer-Gamboa A, Ahumada F. Running world cross-country championships: a unique model for pacing. Int J Sports Physiol Perform. 2014;9(6):1000-5. [PubMed ID: 24664894]. https://doi.org/10.1123/ijspp.2013-0457.
-
26.
Hanley B. Senior men's pacing profiles at the IAAF World Cross Country Championships. J Sports Sci. 2014;32(11):1060-5. [PubMed ID: 24506144]. https://doi.org/10.1080/02640414.2013.878807.
-
27.
Carlsson M, Assarsson H, Carlsson T. The influence of sex, age, and race experience on pacing profiles during the 90 km Vasaloppet ski race. Open Access J Sports Med. 2016;7:11-9. [PubMed ID: 26937207]. [PubMed Central ID: PMC4762471]. https://doi.org/10.2147/OAJSM.S101995.
-
28.
Losnegard T, Kjeldsen K, Skattebo O. An Analysis of the Pacing Strategies Adopted by Elite Cross-Country Skiers. J Strength Cond Res. 2016;30(11):3256-60. [PubMed ID: 26982973]. https://doi.org/10.1519/JSC.0000000000001424.
-
29.
Knechtle B, Nikolaidis PT. The Age of Peak Marathon Performance in Cross-Country Skiing-The "Engadin Ski Marathon". J Strength Cond Res. 2018;32(4):1131-6. [PubMed ID: 29570600]. https://doi.org/10.1519/JSC.0000000000001931.
-
30.
Touren. Originalstrecke des bekannten Engadin Skimarathon von Maloja nach S-chanf. 2015. Available from: http://www.gps-touren.ch/01_touren/einzel.php?tourId=980.
-
31.
Haymes EM, Dickinson AL. Characteristics of elite male and female ski racers. Med Sci Sports Exerc. 1980;12(3):153-8. [PubMed ID: 7402049].
-
32.
Schafer D, Gjerdalen GF, Solberg EE, Khokhlova M, Badtieva V, Herzig D, et al. Sex differences in heart rate variability: a longitudinal study in international elite cross-country skiers. Eur J Appl Physiol. 2015;115(10):2107-14. [PubMed ID: 26002403]. https://doi.org/10.1007/s00421-015-3190-0.
-
33.
Hegge AM, Bucher E, Ettema G, Faude O, Holmberg HC, Sandbakk O. Gender differences in power production, energetic capacity and efficiency of elite crosscountry skiers during wholebody, upperbody, and arm poling. Eur J Appl Physiol. 2016;116(2):291-300. [PubMed ID: 26476546]. https://doi.org/10.1007/s00421-015-3281-y.
-
34.
Tonnessen E, Haugen TA, Hem E, Leirstein S, Seiler S. Maximal aerobic capacity in the winter-Olympics endurance disciplines: Olympic-medal benchmarks for the time period 1990-2013. Int J Sports Physiol Perform. 2015;10(7):835-9. [PubMed ID: 25611016]. https://doi.org/10.1123/ijspp.2014-0431.
-
35.
Carlsson M, Carlsson T, HammarstrOm D, Malm C, Tonkonogi M. Prediction of race performance of elite cross-country skiers by lean mass. Int J Sports Physiol Perform. 2014;9(6):1040-5. [PubMed ID: 24700141]. https://doi.org/10.1123/ijspp.2013-0509.
-
36.
Knechtle B, Nikolaidis PT. Sex differences in pacing during 'Ultraman Hawaii'. PeerJ. 2016;4. e2509. [PubMed ID: 27703854]. [PubMed Central ID: PMC5045888]. https://doi.org/10.7717/peerj.2509.
-
37.
Grimsmo J, Arnesen H, Maehlum S. Changes in cardiorespiratory function in different groups of former and still active male cross-country skiers: a 28-30-year follow-up study. Scand J Med Sci Sports. 2010;20(1):e151-61. [PubMed ID: 19422661]. https://doi.org/10.1111/j.1600-0838.2009.00931.x.
-
38.
Stoggl R, Muller E, Stoggl T. Motor abilities and anthropometrics in youth cross-country skiing. Scand J Med Sci Sports. 2015;25(1):e70-81. [PubMed ID: 24894129]. https://doi.org/10.1111/sms.12254.
-
39.
McGawley K, Holmberg HC. Aerobic and anaerobic contributions to energy production among junior male and female cross-country skiers during diagonal skiing. Int J Sports Physiol Perform. 2014;9(1):32-40. [PubMed ID: 24088732]. https://doi.org/10.1123/ijspp.2013-0239.
-
40.
Skattebo O, Hallen J, Ronnestad BR, Losnegard T. Upper body heavy strength training does not affect performance in junior female cross-country skiers. Scand J Med Sci Sports. 2016;26(9):1007-16. [PubMed ID: 26146761]. https://doi.org/10.1111/sms.12517.
-
41.
Formenti D, Rossi A, Calogiuri G, Thomassen TO, Scurati R, Weydahl A. Exercise Intensity and Pacing Strategy of Cross-country Skiers during a 10 km Skating Simulated Race. Res Sports Med. 2015;23(2):126-39. [PubMed ID: 25670160]. https://doi.org/10.1080/15438627.2015.1005298.
-
42.
Ainegren M, Carlsson P, Tinnsten M, Laaksonen MS. Skiing economy and efficiency in recreational and elite cross-country skiers. J Strength Cond Res. 2013;27(5):1239-52. [PubMed ID: 22344058].