Abstract
Background:
Welding fumes consist of a wide range of complex metal component. Metals induced chronic obstructive pulmonary disease, bronchitis, metal fume fever, cancer, and functional changes in the lung. Since oxidative stress plays a role in this pathogenesis, it is characterized by airflow limitation.Objectives:
This study focused on the anticipation of the oxidative stress biomarker in welders by assessing the amount of urinary metals and spirometry airflow index.Materials and Methods:
We measured malondialdehyde (MDA), as a biomarker of oxidative stress, in urine from20 manual metal arc welders of a petroleum tank making plant. For controls, we recruited 20 ministerial workers who were matched with welders. Urine content of chromium, cadmium, and lead as well as spirometry airflow parameters such as expiratory volumes were applied to partial least square regression (PLS) model for predicting oxidative stress biomarker.Results:
The Results revealed that metal urine concentration in welders was higher than controls but only the difference in chromium concentration was significant (P < 0.002). In the range of metals exposure, induction of oxidative stress for exposed group was observed by increase in urine MDA (11.17 ± 4.23 and 4.83 ± 1.82 mM in welders and controls, respectively; P < 0.01). Information of the metals urine concentration and FEV1/FVC, FEF25%-75% of spirometry index were subjected to PLS analysis to predict oxidative stress biomarker. This model was capable of predicting the concentration of MDA with the regression of R2 = 0.91.Conclusions:
PLS predicts the oxidative stress biomarker with an acceptable sensitivity. According to our research, we can assess the level of oxidative stress as the sign of multi-metal toxicity by following the common biomonitoring assessment. This method could be useful for further engineering control procedures.Keywords
1. Background
Exposure to mixture of metals is a serious health problem. Occupational exposure to multi-metals occurs in welding, melting, and mining employees (1-3). Manual metal arc welding (MMAW) is one of the world's most popular processes to weld iron, stainless steel, nickel, and aluminum alloys (4, 5). Depending on the joining metals, component of electrodes, welding technique and condition of welding process, welding fumes consist of a wide range of complex metal component. Cadmium, chromium, lead, nickel, magnesium, and other metals are commonly detectable in welding exposure; stainless steel welding mostly contain chromium fume (6, 7). Metals can induce different diseases such as chronic obstructive pulmonary disease (8), bronchitis (9), metal fume fever (10), cancer (11), and dermatitis (12). Exposure to fumes increases the risk of lung cancer. The International Agency for Research on Cancer (IARC) classifies welding fumes as “possibly carcinogenic to humans” (13). Based on experimental studies, DNA interaction, DNA damage, and generation of reactive oxygen species (ROS) in metal exposure could promote the carcinogenicity effect (14). Oxidative stress is a toxicity mechanism that results from the imbalance between free radical production (e.g. ROS) and antioxidant defense in cells. Oxidative stress target wide range of macromolecules including nucleic acids (15) and proteins (16), which mediates the induction of several pathogeneses. It has been documented that metals-induced damage can be related to oxidative stress generation (17-19). Some studies have confirmed that occurrence of oxidative stress in metal exposure is relevant for human health assessment (20-22). Moreover, recent studies have confirmed a correlation between spirometry indexes and oxidative stress damage (23). Partial least square regression (PLS) is a common multivariate data modeling in the field of chemometrics method, which is based on other methods including principal components analysis (24).
2. Objectives
In the present study, we evaluated urine concentration of cadmium, chromium, and lead as well as spirometry index in MMAW employee of a petroleum tank making (PTM) factory. These parameters were presented to PLS model for anticipating malondialdehyde (MDA) as oxidative stress biomarker of multi-metal toxicity. The aim of this challenge was to predict oxidative damage in welders as an early effect of multi-metal exposure using routine hygiene biomonitoring.
3. Materials and Methods
3.1. Chemicals
We used 1,1,3,3-tetraethoxypropane, 2-thiobarbituric acid (TBA) (Sigma), ammonium pyrrolidine dithiocarbamate (APDC), triton X-100, nitric acid, methyl isobutyl ketone, n-butanol, chromium, cadmium, and lead standards (Merck, Germany).
3.2. Human Subjects
We recruited 20 MMAW workers from a PTM factory with the mean age of 38.6 ± 7.4 years and at least one year in the profession. We obtained 10 mL urine from each of them. Age-and socioeconomically-matched controls (P values < 0.01, n = 20 was recruited from ministerial employee who were not occupationally exposed to physical or chemical compounds. Two subject of welder with over five year history of cigarette smoking were matched with control. In all subjects, pulmonary function parameters (i.e. FEV1/FVC, FEF25%-75%) were tested by spirometry.
3.3. Assessment Methods
3.3.1. Chromium, Cadmium, and Lead in Urine
Metals in urine samples were extracted by methyl isobutyl ketone solutions of metal-APDC complex (25). Chromium, cadmium, and lead concentrations were assayed with an AL2200 Aurora flameless atomic absorption spectrophotometer.
3.3.2. Malondialdehyde in Urine
For this assay, a mixture of 1% TBA and urine was heated in a boiling water bath. Briefly, 100 μL of concentrated HCl was added to 10 mL urine samples containing 1% TBA. This mixture was heated at 120℃ for 40 minutes to hydrolyze the MDA of urine samples. The resulting color complex was extracted with liquid-phase extraction by n-butyl alcohol and the absorbance of the organic phase was measured at the wavelength of 530 nm (26).
3.4. Data Analysis
3.4.1. Statistical Analysis
Statistical analysis was applied using the JMP-7 software. The results were expressed as means ± standard deviation. The difference between subject and control groups was assessed with independent-samples student t-test. P values < 0.05 were considered as statistically significant.
3.4.2. Partial Least Square Regression Analysis
PLS is a common multivariable linear regression in chemometrics modeling. Y is defined as independent objects by m variable output matrix and X is defined as n dependent objects by p variable predictor matrix. Hereby, PLS is based on the simultaneous decomposition of X and Y into latent variables (T) and associated loading vectors (Q). Regression is performed on these components; thus, Y = TQ + E, where Q is a matrix of regression coefficients (loadings) for T (27).
In this study, we considered MDA concentration in urine as Y, and the urine concentration of chromium, cadmium, and lead as well as FEV1/FVC, FEF25%-75% of spirometry index as X for the PLS analysis using MATLAB software.
4. Results
4.1. Parameters Assessment
Biological monitoring of metals in fume exposure is shown in Figure 1. MMAW workers had significantly higher levels of urine chromium concentration than controls (P < 0.002). The mean values of chromium in urine samples of welders and control were 2.32 ± 0.77 and 1.16 ± 0.435 µg/L, respectively (Figure 1). The Results showed that cadmium and lead concentration in welders' urine was higher than controls; however, the difference was not significant (Figure 2).
Metal Concentration in Urine of Welders and Controls
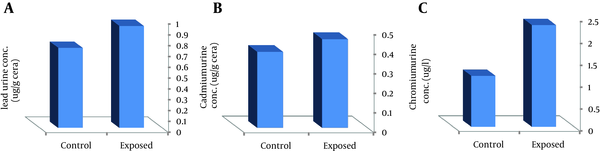
Oxidative Damage in Welders and Controls
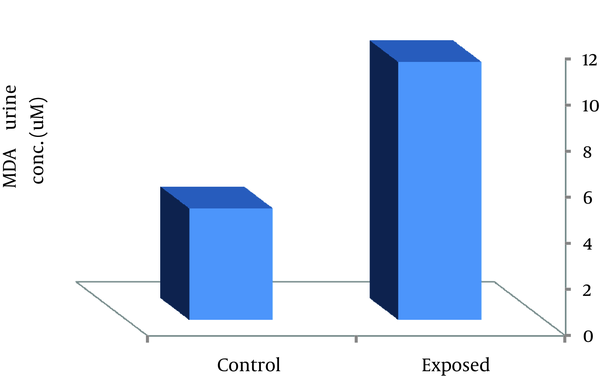
Although the concentrations of carcinogen metals in the urine of welders were higher than those of controls, both of them were much lower than BEIs (Table 1). MDA level as lipid peroxidation marker for workers were also significantly (P < 0.01) higher than that of controls (mean ± SD, 11.17 ± 4.23 and 4.83 ± 1.182 mM, respectively; P < 0.01).
Metal | Urine Concentration | BEI |
---|---|---|
Chromium, µg/L | 2.32 ± 0.77 | 10 |
Cadmium, µg/g creatinine | 0.94 ± 0.04 | 5 |
4.2. Partial Least Square Analysis
Input matrix (40 × 5) sorted in data sets composed of 30 training variables and ten testing variables. Data were modeled with PLS analyzing to predict pattern for oxidative damage. To choose an optimized number of principal components (PCs), we examined the mean squared prediction errors between the measured concentration of MDA and the predicted oxidative damage with increasing numbers of PCs. Figure 3 shows that the mean squared prediction was minimized with just three PCs for the PLS model. In order to evaluate the performance of the models, correlation coefficient (R2) values for observation and predicted oxidative damage were used. When the model was performed for the training dataset, MDA concentration in testing dataset was predicted using the rules of PLS model. There were suitable correlation with the regression of R2 = 0.91 for anticipating oxidative damage in testing dataset.
Estimated Means Squared Prediction Errors of Cross-Validation for Oxidative Damage Using Partial Least Square Regression Analysis
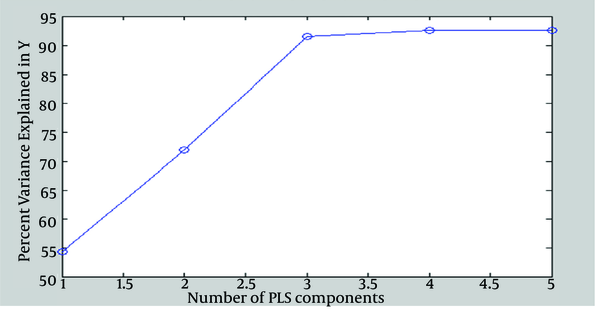
5. Discussion
The results of this study showed that urine chromium concentration and MDA, as oxidative damage indicator, were significantly higher in welders in comparison to the controls. Mean concentrations of chromium, cadmium, and lead in urine of MMAW workers were 2.32 ± 0.77 µg/L, 0.45 ± 0.011 µg/g creatinine, and 0.94 ± 0.04 µg/g creatinine, respectively, which were lower than other reports (7). Exposure to multi-metals such as lead, cadmium, chromium, molybdenum, and magnesium is reported in welders (28). Whereas steel, iron, and stainless steel have to be added to this study; exposure to chromium is highlighted in biomonitoring of MMAW workers. Urinary concentration of chromium among subjects was twice as great as controls. Previous investigations have demonstrated that in addition to the air monitoring in occupational exposure, metal assessment in biological samples is suitable for hygiene engineering control (29); however, researchers have obtained weak association between airborne and urinary concentrations of metals in welder (7). Moreover, our results showed, urine concentrations of metals were lower than BEIs (30) whereas oxidative stress was occurred in exposed group. It seems that in multi-metal exposure, metal estimation in biological samples could not characterize the toxicity and synergism effect. Similar trace effect of metals toxicity such as oxidative damage is more suitable for multi-metal damage estimation. Exposure to metals in welders has been reported frequently (5, 31). The base of this study was oxidative damage and MDA production in metals-exposed population (15, 16). Since lipid peroxidation produces MDA (32), we measured the excretion of MDA in urine of MMAW worker from a PTM factory for oxidative damage estimation. Metal exposure was assessed by measuring chromium, cadmium, and lead in urine sample. Approximation of oxidative stress is not a routine experiment in usual laboratories. In this work, we suggest prediction of oxidative damage using common biomonitoring assessment. Estimations of oxidative stress among the multi-metal exposure helps to distinguish people with higher priority for monitoring. With regards to identifying damaged group based on the oxidative toxicity, management control and engineering control measures could be justified.
References
-
1.
Hearing VJ, Ekel TM, Montague PM, Nicholson JM. Mammalin tyrosinase. Stoichiometry and measurement of reaction products. Biochim Biophys Acta. 1980;611(2):251-68. [PubMed ID: 6766744].
-
2.
Mayer AM. Polyphenol oxidases in plants and fungi: going places? A review. Phytochemistry. 2006;67(21):2318-31. [PubMed ID: 16973188]. https://doi.org/10.1016/j.phytochem.2006.08.006.
-
3.
Theos AC, Tenza D, Martina JA, Hurbain I, Peden AA, Sviderskaya EV, et al. Functions of adaptor protein (AP)-3 and AP-1 in tyrosinase sorting from endosomes to melanosomes. Mol Biol Cell. 2005;16(11):5356-72. [PubMed ID: 16162817]. https://doi.org/10.1091/mbc.E05-07-0626.
-
4.
Lehnert M, Pesch B, Lotz A, Pelzer J, Kendzia B, Gawrych K, et al. Exposure to inhalable, respirable, and ultrafine particles in welding fume. Ann Occup Hyg. 2012;56(5):557-67. [PubMed ID: 22539559]. https://doi.org/10.1093/annhyg/mes025.
-
5.
Laohaudomchok W, Lin X, Herrick RF, Fang SC, Cavallari JM, Shrairman R, et al. Neuropsychological effects of low-level manganese exposure in welders. Neurotoxicology. 2011;32(2):171-9. [PubMed ID: 21192973]. https://doi.org/10.1016/j.neuro.2010.12.014.
-
6.
Matczak W, Chmielnicka J. Relation between various chromium compounds and some other elements in fumes from manual metal arc stainless steel welding. Br J Ind Med. 1993;50(3):244-51. [PubMed ID: 8457491].
-
7.
Golbabaei F, Seyedsomea M, Ghahri A, Shirkhanloo H, Khadem M, Hassani H, et al. Assessment of welders exposure to carcinogen metals from manual metal arc welding in gas transmission pipelines, iran. Iran J Public Health. 2012;41(8):61-70. [PubMed ID: 23113226].
-
8.
Saini Y, Greenwood KK, Merrill C, Kim KY, Patial S, Parameswaran N, et al. Acute cobalt-induced lung injury and the role of hypoxia-inducible factor 1alpha in modulating inflammation. Toxicol Sci. 2010;116(2):673-81. [PubMed ID: 20511350]. https://doi.org/10.1093/toxsci/kfq155.
-
9.
Kusaka Y, Iki M, Kumagai S, Goto S. Epidemiological study of hard metal asthma. Occup Environ Med. 1996;53(3):188-93. [PubMed ID: 8704860].
-
10.
Kelleher P, Pacheco K, Newman LS. Inorganic dust pneumonias: the metal-related parenchymal disorders. Environ Health Perspect. 2000;108 Suppl 4:685-96. [PubMed ID: 10931787].
-
11.
Cohen MD, Zelikoff JT, Chen LC, Schlesinger RB. Immunotoxicologic effects of inhaled chromium: role of particle solubility and co-exposure to ozone. Toxicol Appl Pharmacol. 1998;152(1):30-40. [PubMed ID: 9772197]. https://doi.org/10.1006/taap.1998.8502.
-
12.
Bordignon V, Palamara F, Cordiali-Fei P, Vento A, Aiello A, Picardo M, et al. Nickel, palladium and rhodium induced IFN-gamma and IL-10 production as assessed by in vitro ELISpot-analysis in contact dermatitis patients. BMC Immunol. 2008;9:19. [PubMed ID: 18482439]. https://doi.org/10.1186/1471-2172-9-19.
-
13.
Iarmarcovai G, Sari-Minodier I, Chaspoul F, Botta C, De Meo M, Orsiere T, et al. Risk assessment of welders using analysis of eight metals by ICP-MS in blood and urine and DNA damage evaluation by the comet and micronucleus assays; influence of XRCC1 and XRCC3 polymorphisms. Mutagenesis. 2005;20(6):425-32. [PubMed ID: 16234265]. https://doi.org/10.1093/mutage/gei058.
-
14.
Snow ET. Metal carcinogenesis: mechanistic implications. Pharmacol Ther. 1992;53(1):31-65. [PubMed ID: 1641401].
-
15.
Hofer T, Marzetti E, Xu J, Seo AY, Gulec S, Knutson MD, et al. Increased iron content and RNA oxidative damage in skeletal muscle with aging and disuse atrophy. Exp Gerontol. 2008;43(6):563-70. [PubMed ID: 18395385]. https://doi.org/10.1016/j.exger.2008.02.007.
-
16.
Yuzefovych LV, Musiyenko SI, Wilson GL, Rachek LI. Mitochondrial DNA damage and dysfunction, and oxidative stress are associated with endoplasmic reticulum stress, protein degradation and apoptosis in high fat diet-induced insulin resistance mice. PLoS One. 2013;8(1). ee54059. [PubMed ID: 23342074]. https://doi.org/10.1371/journal.pone.0054059.
-
17.
Barton JK, Olmon ED, Sontz PA. Metal Complexes for DNA-Mediated Charge Transport. Coord Chem Rev. 2011;255(7-8):619-34. [PubMed ID: 21643528]. https://doi.org/10.1016/j.ccr.2010.09.002.
-
18.
Lodovici M, Bigagli E. Oxidative stress and air pollution exposure. J Toxicol. 2011;2011:487074. [PubMed ID: 21860622]. https://doi.org/10.1155/2011/487074.
-
19.
Al Zabadi H, Ferrari L, Sari-Minodier I, Kerautret MA, Tiberguent A, Paris C, et al. Integrated exposure assessment of sewage workers to genotoxicants: an urinary biomarker approach and oxidative stress evaluation. Environ Health. 2011;10:23. [PubMed ID: 21435260]. https://doi.org/10.1186/1476-069X-10-23.
-
20.
Ellis JK, Athersuch TJ, Thomas LD, Teichert F, Perez-Trujillo M, Svendsen C, et al. Metabolic profiling detects early effects of environmental and lifestyle exposure to cadmium in a human population. BMC Med. 2012;10:61. [PubMed ID: 22713677]. https://doi.org/10.1186/1741-7015-10-61.
-
21.
Goodrich JM, Wang Y, Gillespie B, Werner R, Franzblau A, Basu N. Glutathione enzyme and selenoprotein polymorphisms associate with mercury biomarker levels in Michigan dental professionals. Toxicol Appl Pharmacol. 2011;257(2):301-8. [PubMed ID: 21967774]. https://doi.org/10.1016/j.taap.2011.09.014.
-
22.
Martinez-Zamudio R, Ha HC. Environmental epigenetics in metal exposure. Epigenetics. 2011;6(7):820-7. [PubMed ID: 21610324].
-
23.
Bartoli ML, Novelli F, Costa F, Malagrino L, Melosini L, Bacci E, et al. Malondialdehyde in exhaled breath condensate as a marker of oxidative stress in different pulmonary diseases. Mediators Inflamm. 2011;2011:891752. [PubMed ID: 21772668]. https://doi.org/10.1155/2011/891752.
-
24.
McDonald JD, Eide I, Seagrave J, Zielinska B, Whitney K, Lawson DR, et al. Relationship between composition and toxicity of motor vehicle emission samples. Environ Health Perspect. 2004;112(15):1527-38. [PubMed ID: 15531438].
-
25.
Manual of analytical methods, method 8003. NIOSH; 1994.
-
26.
Nacitarhan S, Ozben T, Tuncer N. Serum and urine malondialdehyde levels in NIDDM patients with and without hyperlipidemia. Free Radic Biol Med. 1995;19(6):893-6. [PubMed ID: 8582665].
-
27.
Le Cao KA, Martin PG, Robert-Granie C, Besse P. Sparse canonical methods for biological data integration: application to a cross-platform study. BMC Bioinformatics. 2009;10:34. [PubMed ID: 19171069]. https://doi.org/10.1186/1471-2105-10-34.
-
28.
Imamoglu N, Yerer MB, Donmez-Altuntas H, Saraymen R. Erythrocyte antioxidant enzyme activities and lipid peroxidation in the erythrocyte membrane of stainless-steel welders exposed to welding fumes and gases. Int J Hyg Environ Health. 2008;211(1-2):63-8. [PubMed ID: 17400508]. https://doi.org/10.1016/j.ijheh.2007.02.003.
-
29.
Quandt SA, Jones BT, Talton JW, Whalley LE, Galvan L, Vallejos QM, et al. Heavy metals exposures among Mexican farmworkers in eastern North Carolina. Environ Res. 2010;110(1):83-8. [PubMed ID: 19818439]. https://doi.org/10.1016/j.envres.2009.09.007.
-
30.
2010.
-
31.
Laohaudomchok W, Cavallari JM, Fang SC, Lin X, Herrick RF, Christiani DC, et al. Assessment of occupational exposure to manganese and other metals in welding fumes by portable X-ray fluorescence spectrometer. J Occup Environ Hyg. 2010;7(8):456-65. [PubMed ID: 20526948]. https://doi.org/10.1080/15459624.2010.485262.
-
32.
Moller P, Loft S. Oxidative damage to DNA and lipids as biomarkers of exposure to air pollution. Environ Health Perspect. 2010;118(8):1126-36. [PubMed ID: 20423813]. https://doi.org/10.1289/ehp.0901725.