Abstract
Background:
There is a great need for further study on the mechanism of HCV infection or its pathopoiesis mechanism. Therefore, an HCV infection model was used to analyze the mechanisms of transcriptional and post-transcriptional regulation of gene expression.Methods:
The detections of transcriptome and microRNAs expressions in Huh7.5.1 cells infected with JFH-1 were conducted with next-generation sequencing. Moreover, bioinformatics data were obtained.Results:
There were 21,827,299, and 42,588,251 reads qualified Illumina read pairs obtained from JFH-1-infected (HCV) and non-infected (blank) Huh7.5.1 cells, respectively. Moreover, 678 and 1,041 mRNAs data with a length of 101 bp from HCV and blank Huh7.5.1 cells cDNA sequence were generated, respectively. The results of comparative transcriptome sequencing analysis declared 460 differentially expressed mRNAs in HCV-infected cells, including 152 upregulated mRNAs and 308 downregulated mRNAs (HCV vs. blank). Gene Ontology (GO) and KEGG pathway enrichment analyses indicated the involved pathways, such as MAPK, p53, and PI3K/Akt signaling pathways, as well as oocyte meiosis and pathways in cancer.Conclusions:
Our work confirmed the transcriptome and microRNA data profiling from the cell model of HCV infection with JFH-1 using next-generation sequencing (NGS). Furthermore, the gene expression and regulation information or signaling pathways associated with the pathopoiesis mechanism of HCV infection were identified.Keywords
Transcriptome microRNA Hepatitis C Virus (HCV) JFH-1 Next-generation Sequencing
1. Background
Worldwide, more than 184 million people have chronic HCV infection, which is associated with a high risk of liver inflammation, fibrosis, cirrhosis, steatosis, and even hepatocellular carcinoma (HCC) (1, 2). As known, IFN-a is the standard therapy for chronic hepatitis C (CHC), but only 50 - 60% of the patients obtain a sustained virologic response (SVR) (3). Newer HCV-specific therapeutics, namely direct-acting antivirals (DAA), have brought a new and highly successful approach to the treatment of hepatitis C (4). Therefore, there is a great need for further study on the pathogenesis mechanism of HCV infection.
The replication and protein expression of HCV can induce differential gene expressions in the host (5, 6). The breakthrough about HCV was the discovery of JFH1, a strain that is able to replicate efficiently and produce particles of HCV in cultured cells (7-9). An in vitro model was used to analyze the transcriptome characteristics of HCV infection, which identified that the regulated expression of genes was related to apoptosis and cell cycle (10-13). Moreover, the studies on the proteome revealed that the profuse confusions were associated with the metabolism of sphingomyelins and phospholipids that play substantial roles in replication, assembly, and viral secretions (14). These suggested that there were crucial differences between the transcriptome and proteome of HCV-infected cells, which may depend on the stability of proteins and the expression regulation at the translational level. Actually, the translational regulation of mRNA has been shown to distinguish between the amounts of mRNA and their commensurate proteins (15, 16).
The gene expression regulation of miRNAs is vital in the differentiation, proliferation, and apoptosis of cells (17-19). Moreover, miRNAs can regulate a great deal of crucial biological processes (20-22). However, how miRNAs mediate mRNA expression regulation at the translational level in hepatoma cells infected with HCV remains unclear. Thus, our study analyzed the differences in the transcriptome and miRNAs profiling between HCV-infected and non-infected cells.
2. Methods
2.1. Cell Line
Huh7.5.1 cell line was purchased from the American type culture collection (ATCC), which came from the tissues of human hepatoma. The cultured cells with DMEM and FBS (37°C, 5% CO2) were infected by JFH1 (HCV genotype 2a) (8, 23). After the purified pJFH-1 plasmid was used to perform RNA synthesis, HCV RNA underwent transfection to pulse the cells with the electroporation method using a Gene Pulser IITM apparatus at 260 V and 950 μF. Furthermore, the northern blot analysis and the HCV core protein quantification were conducted to confirm the expression and function of JFH-1 in the cells.
2.2. Next-generation Sequencing
The isolated total RNA was assessed with agarose gel electrophoresis. Degraded RNA and contaminations were removed, and then the purified RNA was extracted with the Thermo Scientific KingFisher Pure RNA Tissue Kit. A Bioanalyzer 2100 system and a Nano 6000 RNA Assay Kit were used to assess the integrity of the RNA. The RIN values of all samples were above eight. Then, the separate Illumina sequencing libraries were generated and purified from pooling samples with the TruSeq™ RNA Sample Preparation Kit. The first and second synthesized strand cDNAs were ligated to adapter oligonucleotides. Moreover, an AMPure XP system was used to purify and quantify the library fragments and obtain 0 - 100 bp paired-end reads (24).
2.3. Analysis of Bioinformatics Data
2.3.1. Control for Data Quality
The FASTQ clean data were obtained from raw reads, and then sequences and content duplication levels of GC, Q20, and Q30 were calculated (24).
2.3.2. Alternative Analysis and Read Alignment with TopHat
The workflow for complete RNA-seq was provided by Cufflinks and TopHat (25). Its core step was the alignment of sequencing data with a reference genome (25). The economical FM index Bowtie was usually utilized for alignment ‘engine’; it stored the sequence of the reference genome and was the extreme data structure (26). The transcripts expressions were accurately quantified by alignments, and the gene discovery and transcript assembly were addressed to analyze the dif¬ferential expression.
2.3.3. Calculation of Expression Levels
A linear statistical model was implemented to evaluate the assignment abundance by Cuffdiff and Cufflinks. Each transcript could explain the maximum likelihood of the observed reads (25).
2.3.4. Transcript Assembly Based on Cufflinks
The individual transcripts were assembled from the RNA-seq reads with Cufflinks, which reported the transcriptome assembly of data aligned to the genome. Moreover, the expression level was quantified in each transfrag of the sample with Cufflinks after the assembly phase (27).
2.3.5. Differential Analysis According to Cuffdiff
The numerous output files were reported by Cuffdiff, and contained differential analysis results. Besides, simple tabular output files reported the changes in transcript and gene expression levels, which described the attribution of gene and transcript expressions and included the common locations and names in the genome. Moreover, the differential analysis results about gene expression were identified by Cuffdiff, which also confirmed differential regulation through promoter switching and differential spliced genes (25).
2.3.6. CummeRbund Visualization
The differential regulation expression was assessed with Cuffdiff at the genome-wide and transcriptional levels. CummeRbund was used to integrate, visualize, and manage all data that came from Cuffdiff analysis (25).
2.3.7. Processing Time and Memory Requirements
The --mask/-M option was used to exclude genes during Cufflinks. During differential analysis, these genes were added to the final transcriptome file by Cuffmerge based on the available reference transcriptome annotation (25).
2.4. Differential miRNAs Analysis
After miRNAs extraction from the above total RNAs using the miRNeasy Mini Kit, the next-generation sequencing (NGS) was conducted with the Illumina HiSeq 2000 platform. Then, miRBase (version 19.0) was matched to the mature sequences of miRNAs based on the reference sequences (28, 29) and the reference library of Bowtie (version 0.12.7) (26). Finally, these data were normalized according to Reads per Million (RPM) (30).
2.5. Statistical Analysis
All data are shown as mean ± standard deviation (SD). Statistical analysis was performed using GraphPad Prism (version 8.0). The differences between the two groups were analyzed with Student’s t-test. The ANOVA with Kruskal-Wallis post hoc test was utilized to compare differences between multiple groups. A P < 0.05 was statistically significant.
3. Results
3.1. Differential Expression Analysis of Transcriptome Profiling
The original raw data of transcriptome sequencing were first obtained (Appendix 1). Then the data preprocessing of quality was performed. There were three steps in quality pre-processing: 1) The proportional threshold was 50%, and the quality threshold was 20 (error rate = 1%); thus, the low-quality reads were removed, 2) The N parts of reads were cut, and 3) The low-quality data at the end of the sequences were eliminated. The results after processing are shown in Appendix 2. Then, the gene mapping for transcriptome data was performed (Appendix 3). The mapped data of the transcriptome come from the matching genes are shown in Appendices 4 and 5, respectively.
Moreover, a random analysis of the read length was conducted. Each gene sequence was extracted to check the distribution of read lengths. The random distribution of read lengths in the transcripts of different samples was analyzed. The positional relationships of read lengths in transcripts over each transcript were counted. The areas were normalized to 0-100. Moreover, the random test of Kolmogorov-Smirnov test was used to check whether the position of read lengths was uniformly distributed. The p value was greater than 0.05, indicating that the data did not show a significant difference from the uniform distribution (Appendix 6). From the above results, it was demonstrated that no significant difference existed between each read length and the uniform distribution; so the uniformity of randomness was very fine, which met the expectations of data analysis (Figure 1).
The distribution of each read length and the uniform distribution. From the above results, we could see that there was no significant difference between the distribution of each read length and the uniform distribution, so the uniformity of randomness was very good, which met the expectations of data analysis. (A) Blank; (B) HCV.
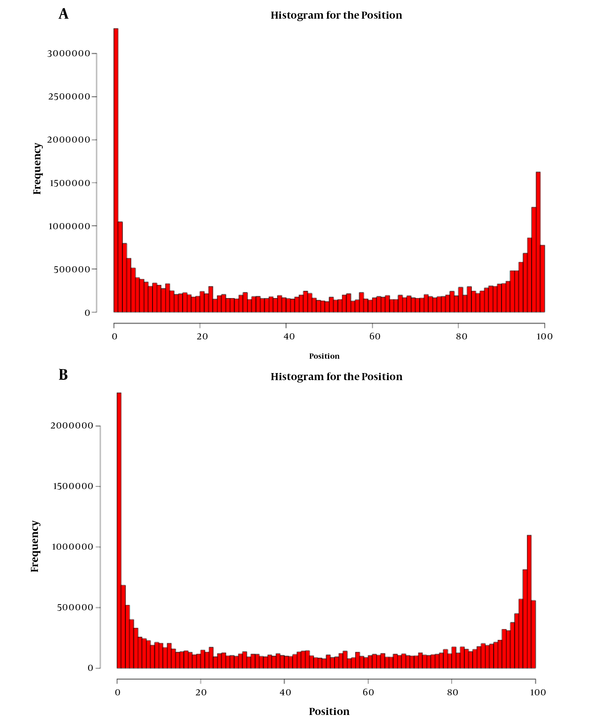
Furthermore, the differential gene expressions were analyzed to take the gene as the unit and count the expression abundance in different samples. Then, the differentially expressed genes (DEGs) in the two groups were detected. The following information was given separately as follows: Test_id: gene ID number; Locus: the location of the gene in the chromosome; A: the expression level of sample A (FPKM); B: the expression level of sample B (FPKM); logFC: log multiple ratio; logCPM: the logarithmic sum of the expressed values; P-value: the t-test significance level; and FDR: the significance level of the FDR test gave the log odds ratio. We selected genes with P value < 0.05 and log2 (fold_change) > 1 as the screening conditions for conducting A-B comparative analysis of expression differences. Moreover, the GO classification analysis was performed. The results had to meet two criteria: (1) The fold change was more than two times, which meant logFC > 1 and (2) P < 0.05.
Besides, the Kyoto Encyclopedia of Genes and Genomes (KEGG) analysis of metabolic pathways was conducted. The upregulation pathways of axon guidance (Appendix 7A), oocyte meiosis (Appendix 7B), regulation of actin cytoskeleton (Appendix 7C), and viral myocarditis (Appendix 7D) were identified.
Axon guidance pathways could regulate axon guidance, synaptogenesis, progenitor dynamics, and cell migration using a variety of mechanisms. Originally found to control local cytoskeletal rearrangements, axon guidance pathways might also regulate gene expression to control these complex developmental processes. In most mammals, oocytes were arrested at the diplotene stage, also called the germinal vesicle (GV) stage of the first meiotic prophase until a surge of gonadotrophin (particularly luteinizing hormone (LH)) from the pituitary stimulated the immature oocyte to resume the first meiosis and ovulate. Moreover, the actomyosin cytoskeleton generated force through the association with myosin motors and anchorage at subcellular structures, such as the plasma membrane. Key signaling pathways affected actin filament growth, bundling, branching, crosslinking, and severing. Besides, viral myocarditis was a rare cardiac disease associated with inflammation and injury of the myocardium. The downstream effects were a product of cooperation between viral processes and the innate immune response of host.
Meanwhile, the downregulation of focal adhesion (Appendix 8A), hepatitis B (Appendix 8B), HTLV-1 infection (Appendix 8C), MAPK signaling pathway (Appendix 8D), osteoclast differentiation (Appendix 8E), p53 signaling pathway (Appendix 8F), pathways in cancer (Appendix 8G), PI3K-Akt signaling (Appendix 8H), proteoglycans in cancer (Appendix 8I), and salmonella infection (Appendix 8J) were obtained.
Focal adhesion kinase (FAK) was a cytoplasmic tyrosine kinase that played critical roles in integrin-mediated signal transductions and also participated in signaling by other cell surface receptors. Additionally, HBV proteins targeted host proteins, involved in a variety of functions, thus regulating transcription, cellular signaling cascades, proliferation, differentiation, and apoptosis. The HTLV-1-infected cells presenting in breast milk (T cells, macrophages, and/or epithelial cells) could transmit the virus across the oral or gastrointestinal mucosa in several ways. The JNK and p38 MAPK signaling pathways were activated by various types of cellular stress. The JNK pathway consisted of JNK, a MAP2K such as MKK4 (SEK1) or MKK7, and a MAP3K such as ASK1, TAK1, MEKK1, or MLK3. In the p38 pathway, p38 was activated by MKK3 or MKK6, and these MAP2Ks were activated by the same MAP3Ks that function in the JNK pathway. The osteoclasts, multinucleated cells originating from the hematopoietic monocyte-macrophage lineage, were responsible for bone resorption. Osteoclastogenesis was mainly regulated by signaling pathways activated by RANK and immune receptors, whose ligands were expressed on the surface of osteoblasts. Signaling from RANK changed gene expression patterns through transcription factors like NFATc1 and characterized the active osteoclast. The P53-mediated cell signal transduction pathway played an important role in regulating the normal life activities of cells, and it was involved in the regulation of 160 genes. In addition, we knew of many situations where altered signaling pathways produced dramatic changes in cell survival, cell proliferation, morphology, angiogenesis, longevity, or other properties that characterized cancer cells. The PI3K-Akt and Ras-ERK pathways were just examples of oncogenic signaling pathways. Many of the genes commonly mutated in cancer encode the components or targets of PI3K-Akt and Ras-ERK pathways. Many proteoglycans (PGs) in the tumor microenvironment had been shown to be key macromolecules that contributed to the biology of various types of cancer including proliferation, adhesion, angiogenesis, and metastasis, affecting tumor progress. Salmonella infection usually presented as self-limiting gastroenteritis or the more severe typhoid fever and bacteremia. The common disease-causing Salmonella species in humans was a single species, Salmonella enterica, which had numerous serovars.
3.2. Differential Expression Analysis of microRNAs Profiling
The original raw data of sequencing microRNAs profiling are shown in Appendix 9. As indicated, Q20 (%) = Q > 20 read/Total read × 100, and Q10 was similar to Q20. Based on Illumina's technical documentation, when the quality value was 10, the error rate was 10%, and when the quality was 29, the error was 1%. Next, the preprocessing for the quality of data was performed, and low-quality reads were removed based on the proportional threshold of 40% and the quality threshold of 20 (error rate = 1%). The read sequences contained a large proportion of N parts, t then these sequences were removed, and the threshold was 4%. After the joint sequences were removed, the effective sequences in length interval were cut out (18 - 35 bp). The length distribution for clean sequences was assessed. The results after processing are shown in Appendix 10. A summary of miRNA data is shown in Figure 2A. The differences in reads coming from sequencing miRNAs are exhibited including FASTQ data, clean data, mapped genome data, mapped sRNA data, the known miRNAs, and novel miRNAs between the two groups (HCV vs. blank control). The length distribution diagram of clean sequences is shown in Figure 2B and 2C. The differences in length distribution coming from sequencing miRNAs are exhibited only for clean sequences (HCV vs. blank control). It could be seen from the above figure that the effective data retention rate was high (average 77.70%), and the effective data length was mainly distributed around 19 - 24 bp, which met the requirements of miRNA subsequent analysis.
Summary of miRNA data and the length distribution diagram of clean sequences. A summary of miRNA data is shown in the above figure. The differences in reads came from sequencing miRNAs are exhibited including Fastq data, clean data, mapped genome data, mapped sRNA data, know miRNAs, and novel miRNAs between the two groups (HCV vs. blank control). The differences in length distribution came from sequencing miRNAs are exhibited only for clean sequences (HCV vs. blank control). (B) Blank; (C) HCV.
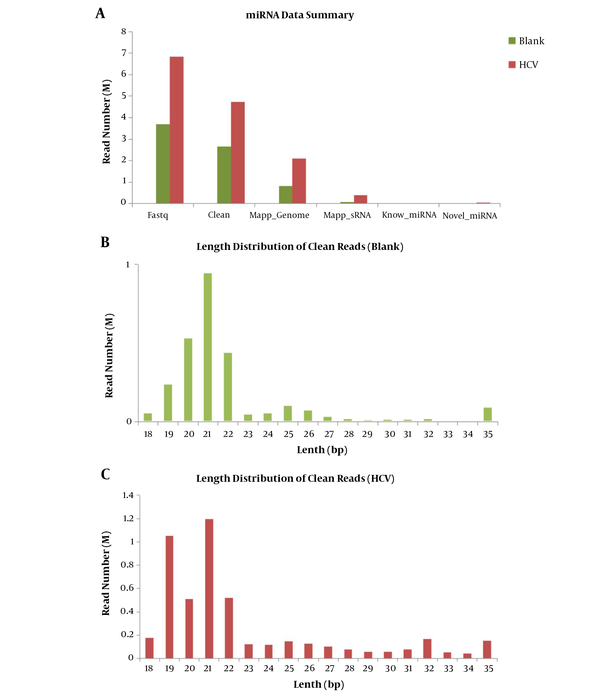
Furthermore, the classification annotation of location comparison was performed for these data. The comparison and annotation between all clean reads and the various types of RNA were summarized. In each comment information, there might be a read that compares the two different bodies of comment information at the same time. In order to make each read have a unique annotation, the RNA was matched according to the priority order of rRNA > Rfam > Repeat > Known miRNA > Exon > Intron, and the reads that had no annotation information were represented by Un-annotated. Then, the comparison statistical results of clean reads of each database (statistics of RNA sequences from individual libraries) were obtained (Appendix 11).
Furthermore, the known microRNA analysis was conducted. The obtained clean sequences were compared with the known miRNA by miRBase (version 20.0) to identify the known miRNA sequence. In this database, the sequence of miRNA hairpin precursors had risen to 24,521, with more than 3,000 new additions. Meanwhile, the mature miRNAs had risen to 30,424, with more than 5,000 new additions. Among the newly added mature miRNAs, human mature miRNAs increased to 2,578, mouse mature miRNAs enhanced to 1,908, and rat miRNAs accelerated to 728. The revised database had systematically revised miRNA sequence annotation and naming rule. The miR* naming rule has been discontinued and replaced by -5p and -3p nomenclature. The sequence information is shown in http://www.mirbase.org/cgi-bin/browse.pl. Besides, the differential expression analysis of microRNA was conducted with software DEGseq. The results of differential expression analysis between the two groups (blank vs. HCV) are presented in the boxplot for known miRNAs (Figure 3A). The boxplot reflected the overall uniformity of data distribution between the two groups, so our data were suitable for subsequent analysis. The numbers of miRNAs in the samples were detected and shown with a Venn chart (Figure 3B). The Venn chart reflected the overlap of the detected miRNA species between the two groups. Moreover, the known miRNA differential expression map (DEGseq) is shown in Figure 3C. This graph was an MA-Plot graph showing the data distribution, and the relationship between differential expression and signal value. The X-axis represented the logarithmic sum of the average number of gene expression levels under the two conditions. Meanwhile, the Y-axis represented the differential expression of these multiple genes; it took log as the base, and the plus and minus signs demonstrated up and downregulation, which was generally less than -1 and more than 1. It confirmed that the different was significant,, and the red dots in the figure indicated the significant differential expression of the FDR test.
Differential expression analysis of microRNA conducted with DEGseq software. The results of differential expression analysis between the two groups (blank vs. HCV) are presented in the boxplot for known miRNAs (A). The boxplot reflected the overall uniformity of data distribution between the two groups, so our data were suitable for subsequent analysis. The numbers of miRNAs between samples were detected and shown with Venn chart (B). The Venn chart reflected the overlap of the detected miRNA species between the two groups. Moreover, the known miRNA differential expression map (DEGseq) is shown in (C).
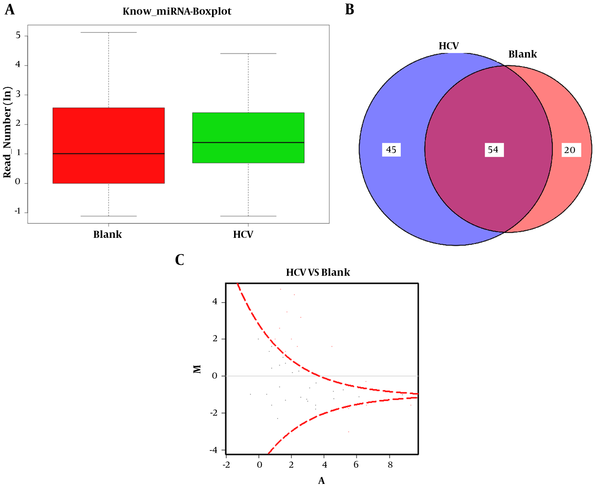
4. Discussion
The development of sophisticated strategies promotes the life cycle of viruses, which further causes evasion from antiviral immune systems, thus inducing diseases. The HCV-RNA consists of approximately 9,500 - 10,000 bp, including 5' and 3' non-coding regions (NCR) with 319 - 341 bp and 27 - 55 bp, respectively, containing several forward and reverse repeat sequences, which may be related to gene replication. An open reading frame (ORF) is located immediately downstream of the 5′ non-coding region, in which the genome sequence is 5′-C-E1-E2-p7-NS2-NS3-NS4-NS5-3′. It can encode a length of approximately 3,014 amino acids, and is a polyprotein precursor. The latter can be cleaved into 10 types of viral proteins by the host cell and the virus's own protease, including three structural proteins, namely nucleocapsid protein with a molecular weight of 19 KD (or core protein, Core) and two glycoproteins (E1 protein with a molecular weight of 33 KD and E2 protein with a molecular weight of 72 KD). Besides, p7 encodes a membrane-intrinsic protein, and its function may be an ion channel. Non-structural proteins include NS2, NS3, NS4A, NS5A, and NS5B. Non-structural proteins are very important to the life cycle of viruses. As known, NS2 and NS3 have protease activity and participate in the cleavage of viral polyprotein precursors. In addition, the NS3 protein has helicase activity and participates in unwinding HCV-RNA molecules to assist in RNA replication, and the function of NS4 is unclear. Besides, NS5A is a phosphoprotein that can interact with a variety of host cell proteins and plays an important role in virus replication. Also, NS5B has RNA-dependent RNA polymerase activity and participates in HCV genome replication (31).
However, the pathogenesis of hepatitis C is still not very clear. When HCV replicates in hepatocytes, it causes changes in the structure and function of liver cells or interferes with protein synthesis in liver cells, then causing degeneration and necrosis of liver cells. It indicates that HCV directly damages the liver and plays a role in pathogenesis. However, most scholars believe that cellular immune pathological reactions might play an important role (32).
As known, HCV is one of the major health concerns globally, and there is a lack of animal models for executing efficient HCV replicons and complete life cycle of HCV. The first discovery of hepatitis C was done by NIH researchers in the United States 40 years ago, and there are currently about 200 million people worldwide infected with or carrying HCV, including more than 40 million patients in my country. Hepatitis C can cause terrible consequences such as cirrhosis and liver cancer, and it also puts tremendous pressure on the health care systems of countries around the world. Because HCV is highly variable, it is easy to develop drug resistance. For a long time, many scientists have been exploring a suitable animal model for in-depth study of HCV. However, HCV only infects humans and chimpanzees, so developing an experimental mouse model encounters great difficulties in practice. The success of previous research provided the most advanced materials to reveal the pathogenic mechanism of hepatitis C, and it can fundamentally promote hepatitis C research on vaccines and drugs for prevention and treatment. The researchers expressed the two receptor molecules for HCV entry into liver cells, i.e., CD81 and OCLN, by transgenic technology on mouse liver cells. Hepatitis C virus can recognize and invade the liver cells of mice and replicate highly. At the same time, 80% of the infected mice subsequently showed typical acute HCV infection and chronic pathological progress, including fatty liver, liver fibrosis, and cirrhosis. Researchers in the laboratory have used this model to observe how HCV escapes the immune system and how antiviral drugs reduce viral titers (7, 9, 33).
A network of mutual effects interacts between the hepatitis C virus and live human cell proteins. The results of the research will help to better understand the mechanism behind the hepatitis C virus causing inflammatory liver disease and open up new treatment avenues. Viruses use human cells to reproduce and spread. This process involves cell host factors and virus interactions. Hepatitis C virus forms a precursor protein, which is processed into 10 viral proteins. Now, scientists have discovered how these proteins interact with each other. The disclosure of this interaction network will help to better understand viral replication and pathogenesis, and pave the way for the design of new antiviral treatments. The results show how viral proteins interact with human cells, which provides a basis for finding new antiviral substances (34-36).
There is thus a great need for further study on the mechanism of HCV infection or its pathopoiesis. To better understand the host aspect for HCV infection, the transcriptome and miRNA sequencing analyses were conducted in Huh7.5.1 cell model infected with JFH-1 according to the technology of Illumina deep sequencing. Transcriptome analysis has been widely used in basic scientific research, as well as medical and drug research or development. Its technical advantages are mainly five points: (1) digitized signals, sequences obtained directly by sequencing, no background noise, no cross-hybridization, and ability to identify a base difference between sequences; (2) high throughput, tens of millions generated by one sequence, and sequencing detection of 100,000 to 300,000 sequence tags; (3) high repeatability, the correlation between two independent experiments in the same laboratory exceeds 99%, and the correlation between two independent experiments in different laboratories is as high as 98%; (4) a wide detection threshold, which spans six orders of magnitude, making it detect rare transcripts as low as two copies and accurately quantify hundreds of thousands of copies of high-expression transcripts; and (5) compatibility of analysis, the data format is the same as the chip, and compatible with chip analysis software (37).
MicroRNA sequencing refers to the collection of all transcribed microRNA products in a certain species or specific cell under a certain physiological function state, including the limitation of time and space. The microRNA transcription regulation level is the most current investigation, and it is also the most important regulation method of organisms. High-throughput technology for transcriptome sequencing is a fast and reliable method to obtain transcriptome information. MicroRNA transcript expression analysis and its accurate counting method can also carry out precise quantitative analysis of genes.
In this work, an HCV-infected cell model was used to conduct the combination of transcriptome and microRNA sequencing analyses. We found 21, 827,299, and 42, 588,251 Illumina read pairs from cDNA libraries of JFH-1-infected (HCV) and non-infected (blank) Huh7.5.1 cells, respectively. Moreover, 678 and 1,041 mRNAs with a length of 101 bp from cDNA libraries of HCV and blank Huh7.5.1 cells were generated, respectively. Comparative transcriptome analysis confirmed 460 differentially expressed mRNAs in the HCV-infected cell model, including 152 upregulated and 308 downregulated unigenes in HCV vs. blank, respectively. The results of GO analysis revealed that the differentially expressed genes were involved in MAPK, p53, and PI3K/Akt signaling pathways, oocyte meiosis, and pathways in cancer. Our work acquired transcriptome data came from a cell model of HCV infection with JFH-1 by next-generation sequencing (NGS) techniques. Therefore, valuable information associated with gene expression and regulation or signaling pathways was obtained in the mechanism and pathopoiesis of HCV infection.
Besides, the key pathways involved in HCV infection or the interaction between HCV and host liver cells were confirmed. Therefore, we thought that they were associated with the key signaling pathways affecting actin filament growth, bundling, branching, crosslinking, and severing. Moreover, the results from analysis confirmed that focal adhesion kinase (FAK), HTLV-1-infected, JNK, and p38 MAPK, P53-mediated, proteoglycans, PI3K-Akt and Ras-ERK cell signal transduction pathways might all play an important role in the process of HCV infection. The inhibitors of integrin-linked kinase (ILK) or FAK restored the IFN-stimulated response element (ISRE) luciferase activity and the expression of IFN-stimulated Genes (ISG) proteins. It suggested that β1-integrin-mediated signals affected the IFN signaling and promoted HCV replication. Therefore, the accumulation of extracellular matrix (ECM) in liver fibrosis may impair IFN signaling through β1-integrin-mediated signaling involving ILK and FAK (38). In addition, the facilitation of HCV replication by Tax protein may partially account for more severe clinical consequences of HCV-related disease in HCV/HTLV co-infected individuals (39). Furthermore, cellular responses to oxidative stress in HCV subgenomic replicon-expressing and Ad-NS5A-transduced cells are regulated by two distinct signaling pathways involving p38 MAPK and JNK via AP-1 that are linked to increased oxidative stress and therefore to an increased antioxidant MnSOD response (40). Besides, the Pro variant of P53 rs1042522 may be used as a genetic predictor for non-responsiveness, while the A/A variant of CD95 rs1800682 may be used as a sensitive biomarker for responsiveness to antiviral therapy of HCV genotype-4a infection (41). Additionally, infection with HCV leads to the activation of nuclear factor-κB, resulting in increased expression of SMAD6 and SMAD7. The upregulation of SMAD6 and SMAD7 induces the expression of heparan sulfate proteoglycans (HSPGs), such as syndecan 1 (SDC1), as well as LDLR, very LDLR, and the scavenger receptor class B member 1, which promote HCV entry and propagation, as well as cellular uptake of cholesterol and lipoprotein (42). Besides, the PI3K-Akt signaling pathway positively regulates HCV translation through SREBPs (43). The effects of GBV-B and HCV NS5A on the PI3K and Ras-Erk pathways were confirmed in cells harboring subgenomic replicons derived from the two viruses (44). Furthermore, deep research will carry out the interaction roles among HCV infection and mRNA regulation medicated by miRNAs involved in the pathways of host cells.
4.1. Conclusions
Our work confirmed the transcriptome and microRNA data profiling from the cell model of HCV infection with JFH-1 using Next-generation Sequencing (NGS). Furthermore, the gene expression and regulation information or signaling pathways associated with the pathopoiesis mechanism of HCV infection were initially identified.
References
-
1.
Bostan N, Mahmood T. An overview about hepatitis C: a devastating virus. Crit Rev Microbiol. 2010;36(2):91-133. [PubMed ID: 20345213]. https://doi.org/10.3109/10408410903357455.
-
2.
Thrift AP, El-Serag HB, Kanwal F. Global epidemiology and burden of HCV infection and HCV-related disease. Nat Rev Gastroenterol Hepatol. 2017;14(2):122-32. [PubMed ID: 27924080]. https://doi.org/10.1038/nrgastro.2016.176.
-
3.
McHutchison JG, Lawitz EJ, Shiffman ML, Muir AJ, Galler GW, McCone J, et al. Peginterferon alfa-2b or alfa-2a with ribavirin for treatment of hepatitis C infection. N Engl J Med. 2009;361(6):580-93. [PubMed ID: 19625712]. https://doi.org/10.1056/NEJMoa0808010.
-
4.
Rogers ME, Balistreri WF. Cascade of care for children and adolescents with chronic hepatitis C. World J Gastroenterol. 2021;27(12):1117-31. [PubMed ID: 33828389]. [PubMed Central ID: PMC8006101]. https://doi.org/10.3748/wjg.v27.i12.1117.
-
5.
Aizaki H, Harada T, Otsuka M, Seki N, Matsuda M, Li YW, et al. Expression profiling of liver cell lines expressing entire or parts of hepatitis C virus open reading frame. Hepatology. 2002;36(6):1431-8. [PubMed ID: 12447869]. https://doi.org/10.1053/jhep.2002.36937.
-
6.
Ciccaglione AR, Marcantonio C, Tritarelli E, Tataseo P, Ferraris A, Bruni R, et al. Microarray analysis identifies a common set of cellular genes modulated by different HCV replicon clones. BMC Genomics. 2008;9:309. [PubMed ID: 18590516]. [PubMed Central ID: PMC2474623]. https://doi.org/10.1186/1471-2164-9-309.
-
7.
Lindenbach BD, Evans MJ, Syder AJ, Wolk B, Tellinghuisen TL, Liu CC, et al. Complete replication of hepatitis C virus in cell culture. Science. 2005;309(5734):623-6. [PubMed ID: 15947137]. https://doi.org/10.1126/science.1114016.
-
8.
Wakita T, Pietschmann T, Kato T, Date T, Miyamoto M, Zhao Z, et al. Production of infectious hepatitis C virus in tissue culture from a cloned viral genome. Nat Med. 2005;11(7):791-6. [PubMed ID: 15951748]. [PubMed Central ID: PMC2918402]. https://doi.org/10.1038/nm1268.
-
9.
Zhong J, Gastaminza P, Cheng G, Kapadia S, Kato T, Burton DR, et al. Robust hepatitis C virus infection in vitro. Proc Natl Acad Sci U S A. 2005;102(26):9294-9. [PubMed ID: 15939869]. [PubMed Central ID: PMC1166622]. https://doi.org/10.1073/pnas.0503596102.
-
10.
Blackham S, Baillie A, Al-Hababi F, Remlinger K, You S, Hamatake R, et al. Gene expression profiling indicates the roles of host oxidative stress, apoptosis, lipid metabolism, and intracellular transport genes in the replication of hepatitis C virus. J Virol. 2010;84(10):5404-14. [PubMed ID: 20200238]. [PubMed Central ID: PMC2863852]. https://doi.org/10.1128/JVI.02529-09.
-
11.
Liu X, Wang T, Wakita T, Yang W. Systematic identification of microRNA and messenger RNA profiles in hepatitis C virus-infected human hepatoma cells. Virology. 2010;398(1):57-67. [PubMed ID: 20006370]. https://doi.org/10.1016/j.virol.2009.11.036.
-
12.
Walters KA, Syder AJ, Lederer SL, Diamond DL, Paeper B, Rice CM, et al. Genomic analysis reveals a potential role for cell cycle perturbation in HCV-mediated apoptosis of cultured hepatocytes. PLoS Pathog. 2009;5(1). e1000269. [PubMed ID: 19148281]. [PubMed Central ID: PMC2613535]. https://doi.org/10.1371/journal.ppat.1000269.
-
13.
Woodhouse SD, Narayan R, Latham S, Lee S, Antrobus R, Gangadharan B, et al. Transcriptome sequencing, microarray, and proteomic analyses reveal cellular and metabolic impact of hepatitis C virus infection in vitro. Hepatology. 2010;52(2):443-53. [PubMed ID: 20683944]. [PubMed Central ID: PMC3427885]. https://doi.org/10.1002/hep.23733.
-
14.
Jacobs JM, Diamond DL, Chan EY, Gritsenko MA, Qian W, Stastna M, et al. Proteome analysis of liver cells expressing a full-length hepatitis C virus (HCV) replicon and biopsy specimens of posttransplantation liver from HCV-infected patients. J Virol. 2005;79(12):7558-69. [PubMed ID: 15919910]. [PubMed Central ID: PMC1143647]. https://doi.org/10.1128/JVI.79.12.7558-7569.2005.
-
15.
Ideker T, Thorsson V, Ranish JA, Christmas R, Buhler J, Eng JK, et al. Integrated genomic and proteomic analyses of a systematically perturbed metabolic network. Science. 2001;292(5518):929-34. [PubMed ID: 11340206]. https://doi.org/10.1126/science.292.5518.929.
-
16.
Shenton D, Smirnova JB, Selley JN, Carroll K, Hubbard SJ, Pavitt GD, et al. Global translational responses to oxidative stress impact upon multiple levels of protein synthesis. J Biol Chem. 2006;281(39):29011-21. [PubMed ID: 16849329]. https://doi.org/10.1074/jbc.M601545200.
-
17.
Reinhart BJ, Slack FJ, Basson M, Pasquinelli AE, Bettinger JC, Rougvie AE, et al. The 21-nucleotide let-7 RNA regulates developmental timing in Caenorhabditis elegans. Nature. 2000;403(6772):901-6. [PubMed ID: 10706289]. https://doi.org/10.1038/35002607.
-
18.
Brennecke J, Hipfner DR, Stark A, Russell RB, Cohen SM. bantam encodes a developmentally regulated microRNA that controls cell proliferation and regulates the proapoptotic gene hid in Drosophila. Cell. 2003;113(1):25-36. [PubMed ID: 12679032]. https://doi.org/10.1016/s0092-8674(03)00231-9.
-
19.
Hatfield SD, Shcherbata HR, Fischer KA, Nakahara K, Carthew RW, Ruohola-Baker H. Stem cell division is regulated by the microRNA pathway. Nature. 2005;435(7044):974-8. [PubMed ID: 15944714]. https://doi.org/10.1038/nature03816.
-
20.
Garzon R, Marcucci G, Croce CM. Targeting microRNAs in cancer: rationale, strategies and challenges. Nat Rev Drug Discov. 2010;9(10):775-89. [PubMed ID: 20885409]. [PubMed Central ID: PMC3904431]. https://doi.org/10.1038/nrd3179.
-
21.
Bader AG, Brown D, Winkler M. The promise of microRNA replacement therapy. Cancer Res. 2010;70(18):7027-30. [PubMed ID: 20807816]. [PubMed Central ID: PMC2940943]. https://doi.org/10.1158/0008-5472.CAN-10-2010.
-
22.
Lee J, Kemper JK. Controlling SIRT1 expression by microRNAs in health and metabolic disease. Aging (Albany NY). 2010;2(8):527-34. [PubMed ID: 20689156]. [PubMed Central ID: PMC2954045]. https://doi.org/10.18632/aging.100184.
-
23.
Tai AW, Benita Y, Peng LF, Kim SS, Sakamoto N, Xavier RJ, et al. A functional genomic screen identifies cellular cofactors of hepatitis C virus replication. Cell Host Microbe. 2009;5(3):298-307. [PubMed ID: 19286138]. [PubMed Central ID: PMC2756022]. https://doi.org/10.1016/j.chom.2009.02.001.
-
24.
Lv J, Liu P, Wang Y, Gao B, Chen P, Li J. Transcriptome analysis of Portunus trituberculatus in response to salinity stress provides insights into the molecular basis of osmoregulation. PLoS One. 2013;8(12). e82155. [PubMed ID: 24312639]. [PubMed Central ID: PMC3849447]. https://doi.org/10.1371/journal.pone.0082155.
-
25.
Trapnell C, Roberts A, Goff L, Pertea G, Kim D, Kelley DR, et al. Differential gene and transcript expression analysis of RNA-seq experiments with TopHat and Cufflinks. Nat Protoc. 2012;7(3):562-78. [PubMed ID: 22383036]. [PubMed Central ID: PMC3334321]. https://doi.org/10.1038/nprot.2012.016.
-
26.
Langmead B, Trapnell C, Pop M, Salzberg SL. Ultrafast and memory-efficient alignment of short DNA sequences to the human genome. Genome Biol. 2009;10(3):R25. [PubMed ID: 19261174]. [PubMed Central ID: PMC2690996]. https://doi.org/10.1186/gb-2009-10-3-r25.
-
27.
Trapnell C, Williams BA, Pertea G, Mortazavi A, Kwan G, van Baren MJ, et al. Transcript assembly and quantification by RNA-Seq reveals unannotated transcripts and isoform switching during cell differentiation. Nat Biotechnol. 2010;28(5):511-5. [PubMed ID: 20436464]. [PubMed Central ID: PMC3146043]. https://doi.org/10.1038/nbt.1621.
-
28.
Kozomara A, Griffiths-Jones S. miRBase: integrating microRNA annotation and deep-sequencing data. Nucleic Acids Res. 2011;39(Database issue):D152-7. [PubMed ID: 21037258]. [PubMed Central ID: PMC3013655]. https://doi.org/10.1093/nar/gkq1027.
-
29.
Martin M. Cutadapt removes adapter sequences from high-throughput sequencing reads. EMBnet J. 2011;17(1):10. https://doi.org/10.14806/ej.17.1.200.
-
30.
Wojcicka A, Swierniak M, Kornasiewicz O, Gierlikowski W, Maciag M, Kolanowska M, et al. Next generation sequencing reveals microRNA isoforms in liver cirrhosis and hepatocellular carcinoma. Int J Biochem Cell Biol. 2014;53:208-17. [PubMed ID: 24875649]. https://doi.org/10.1016/j.biocel.2014.05.020.
-
31.
Sainz BJ, Chisari FV. Production of infectious hepatitis C virus by well-differentiated, growth-arrested human hepatoma-derived cells. J Virol. 2006;80(20):10253-7. [PubMed ID: 17005703]. [PubMed Central ID: PMC1617281]. https://doi.org/10.1128/JVI.01059-06.
-
32.
Lupberger J, Croonenborghs T, Roca Suarez AA, Van Renne N, Juhling F, Oudot MA, et al. Combined Analysis of Metabolomes, Proteomes, and Transcriptomes of Hepatitis C Virus-Infected Cells and Liver to Identify Pathways Associated With Disease Development. Gastroenterology. 2019;157(2):537-551 e9. [PubMed ID: 30978357]. [PubMed Central ID: PMC8318381]. https://doi.org/10.1053/j.gastro.2019.04.003.
-
33.
Bartosch B, Dubuisson J, Cosset FL. Infectious hepatitis C virus pseudo-particles containing functional E1-E2 envelope protein complexes. J Exp Med. 2003;197(5):633-42. [PubMed ID: 12615904]. [PubMed Central ID: PMC2193821]. https://doi.org/10.1084/jem.20021756.
-
34.
Miyamoto M, Kato T, Date T, Mizokami M, Wakita T. Comparison between subgenomic replicons of hepatitis C virus genotypes 2a (JFH-1) and 1b (Con1 NK5.1). Intervirology. 2006;49(1-2):37-43. [PubMed ID: 16166787]. https://doi.org/10.1159/000087261.
-
35.
El-Awady MK. Antibody to E1 peptide of hepatitis C virus genotype 4 inhibits virus binding and entry to HepG2 cells in vitro. World J Gastroenterol. 2006;12(16):2530. https://doi.org/10.3748/wjg.v12.i16.2530.
-
36.
Lazaro CA, Chang M, Tang W, Campbell J, Sullivan DG, Gretch DR, et al. Hepatitis C virus replication in transfected and serum-infected cultured human fetal hepatocytes. Am J Pathol. 2007;170(2):478-89. [PubMed ID: 17255316]. [PubMed Central ID: PMC1851861]. https://doi.org/10.2353/ajpath.2007.060789.
-
37.
Hrdlickova R, Toloue M, Tian B. RNA-Seq methods for transcriptome analysis. Wiley Interdiscip Rev RNA. 2017;8(1). [PubMed ID: 27198714]. [PubMed Central ID: PMC5717752]. https://doi.org/10.1002/wrna.1364.
-
38.
Kuwashiro T, Iwane S, Jinghe X, Matsuhashi S, Eguchi Y, Anzai K, et al. Regulation of interferon signaling and HCVRNA replication by extracellular matrix. Int J Mol Med. 2018;42(2):957-65. [PubMed ID: 29786754]. [PubMed Central ID: PMC6034922]. https://doi.org/10.3892/ijmm.2018.3693.
-
39.
Zhang J, Yamada O, Kawagishi K, Yoshida H, Araki H, Yamaoka S, et al. Up-regulation of hepatitis C virus replication by human T cell leukemia virus type I-encoded Tax protein. Virology. 2007;369(1):198-205. [PubMed ID: 17765943]. https://doi.org/10.1016/j.virol.2007.07.032.
-
40.
Qadri I, Iwahashi M, Capasso JM, Hopken MW, Flores S, Schaack J, et al. Induced oxidative stress and activated expression of manganese superoxide dismutase during hepatitis C virus replication: role of JNK, p38 MAPK and AP-1. Biochem J. 2004;378(Pt 3):919-28. [PubMed ID: 14670077]. [PubMed Central ID: PMC1224028]. https://doi.org/10.1042/BJ20031587.
-
41.
Abd-Rabou AA, Eskander EF, Mohamed MS, Yahya SMM, Sherbini AE, Shaker OG. P53 rs1042522 and CD95 rs1800682 genetic variations in HCV-4a response to antiviral therapy. Genes Dis. 2015;2(2):197-210. [PubMed ID: 30258864]. [PubMed Central ID: PMC6150111]. https://doi.org/10.1016/j.gendis.2015.02.004.
-
42.
Zhang F, Sodroski C, Cha H, Li Q, Liang TJ. Infection of Hepatocytes With HCV Increases Cell Surface Levels of Heparan Sulfate Proteoglycans, Uptake of Cholesterol and Lipoprotein, and Virus Entry by Up-regulating SMAD6 and SMAD7. Gastroenterology. 2017;152(1):257-270 e7. [PubMed ID: 27693511]. [PubMed Central ID: PMC5547743]. https://doi.org/10.1053/j.gastro.2016.09.033.
-
43.
Shi Q, Hoffman B, Liu Q. PI3K-Akt signaling pathway upregulates hepatitis C virus RNA translation through the activation of SREBPs. Virology. 2016;490:99-108. [PubMed ID: 26855332]. https://doi.org/10.1016/j.virol.2016.01.012.
-
44.
Mankouri J, Milward A, Pryde KR, Warter L, Martin A, Harris M. A comparative cell biological analysis reveals only limited functional homology between the NS5A proteins of hepatitis C virus and GB virus B. J Gen Virol. 2008;89(Pt 8):1911-20. [PubMed ID: 18632962]. https://doi.org/10.1099/vir.0.2008/001131-0.