Abstract
Background:
Following menopause, the risk of many diseases is increased, and this situation may be due to changes in anthropometric indices (AI), while the association between adiposity and age at natural menopause (ANM) is not clear yet.Objectives:
This longitudinal study was conducted to investigate the ability of AI in predicting ANM.Methods:
For this purpose, a total of 3,876 women aged > 20 years old from participants of the Tehran lipid and glucose study (TLGS) met our eligibility criteria. The association between ANM and various AIs was assessed using the Accelerated Failure Time (AFT) model, and time ratio (TR) with 95% confidence intervals was reported in this longitudinal study.Results:
The median [interquartile range (IQR) 25 - 75] of the survival time was 12.5 (7.9 - 15.8) years. At the end of the follow-up, 1,479 (38.2%) of the participants reached menopause. The median time to natural menopause was decreased by about 2% with one standard deviation (SD) increase of both a body shape index (ABSI) (time ratio (TR): 0.98, 95% CI: 0.97, 0.99) and lipid accumulation product (LAP) (TR: 0.98, 95% CI: 0.98, 0.99) z-scores; and this time was increased by about 1% (TR: 1.01, 95% CI: 1.00, 1.02) with one SD increase in body mass index (BMI) z-score.Conclusions:
The ABSI, LAP, and BMI were the most useful AIs for identification of the time to menopause onset, and ABSI and LAP were inversely associated with the ANM. Also, the BMI was directly associated with the ANM.Keywords
1. Background
Menopause is considered one of the most important milestones during the reproductive age of women. There is a considerable individual variation in the age of menopause onset; its median is equal to 52.5 years old, but it varies from 40 to 60 years of age, and about 10% of women reach menopause by 45 years of age (1, 2). It has been shown that the level of general health is higher among the women with older age at natural menopause (ANM) versus ones with early menopause, experiencing the menopause before 40 years of age; early menopause is associated with a higher risk of cardiovascular disorders and premature mortality; however, late menopause is also associated with some cancers such as ovarian cancer and melanoma (3, 4).
Studies have shown that the menopause transition is related to the reorganization of the subcutaneous fat from the gluteal/femoral reservoir to the abdominal one (5), which is appropriate for the menopause condition, including the end of ovarian function and reduced activity, energy spending, and fat oxidation (6). Therefore, Cardio-Metabolic (CM) risk factors increase in women reaching menopause (7-9). Several indicators have been introduced for assessment of obesity. Body Mass Index (BMI) is the most common one; however, this index does not differentiate between the weight caused by fat accumulation and muscle weight (10, 11), nor does it differentiate the peripheral fat from abdominal one (12) and importantly, abdominal fat is a factor, which is more strongly associated with the development of non-communicable diseases (NCDs). Besides, the waist circumference (WC) and waist-to-hip ratio (WHR), as indicators of central adiposity, have also been recommended owing to their relevance with the fat distribution (13, 14), which are more precise than the BMI in predicting the mortality and cardiovascular disease (CVD) (15, 16).
Currently, several composite indicators have been introduced body shape index (ABSI) (17), which can precisely predict premature death more than that predicted by BMI and WC (18). The body roundness index (BRI) is another indicator (2013) (19). It has been shown that BRI can predict CVD (20). Recently, more indicators have been introduced, including the visceral adiposity index (VAI) and lipid accumulation product (LAP) (21). Since the relationship between anthropometric indices and menopausal age is not clear, the present study was carried out to investigate the association between the ANM and various anthropometric indices (AIs) using the data collected by the Tehran lipid and glucose study (TLGS).
2. Methods
2.1. Subjects
For the aim of this longitudinal cohort community-based study, data of the women participating in the TLGS were used. In fact, TLGS is an ongoing prospective study initiated in 1998 to specify the prevalence of NCD risk factors where 15,005 people aged ≥ 3 years old are followed up every three years, and the data on any changes in the demographic, anthropometric, reproductive, and metabolic characteristics and laboratory assessments are documented (22).
A standard questionnaire of the TLGS was completed for all of the subjects. Our study population included all the women aged > 20 years who participated in the TLGS (n = 5,405). The exclusion criteria were menopausal women at baseline (n = 125), those with missing data (322), those who met the eligibility criteria, including lack of a history of surgical menopause or any other ovarian surgery at all of the follow-ups (n = 183), and lost to follow up (n = 899). Finally, 3,876 women completed the follow-up (Figure 1).
Study flowchart
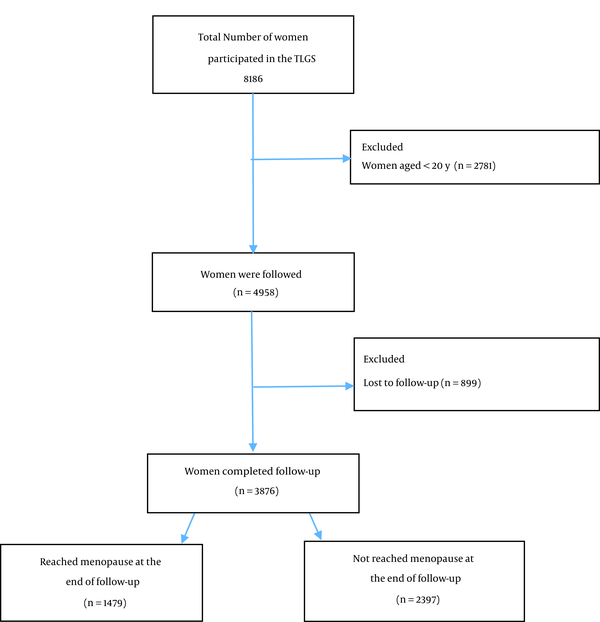
2.2. Definition
Menopause was defined as the absence of spontaneous menstrual bleeding for more than 12 months, for which no other pathologic or physiologic cause could be determined according to the World Health Organization (WHO) classification (23). Age at menopause was calculated based on their last reported menstrual cycle. AIs were calculated as follows:
VAI (for women) = WC/36.58 + (1.89 × BMI) × (TG/0.81) × (1.52/High-density lipoprotein cholesterol (HDL-C)
2.3. Sample Size
We calculated our sample size using the formula provided in Hsieh’s, Philip’s, and Lavori’s.
The formula was as below:
α = 0.05; β = 0.2; σ = 5;
Where σ2 is the variance of BMI and Δ is the log hazards ratio associated with a one-unit change in BMI obtained from previous studies. D is the required number of menopause events, ρ is the overall menopause rate in this study, and N is the needed sample size.
2.4. Statistical Analysis
According to the one-sample Kolmogorov–Smirnov test, continuous variables were checked for normality based on. The accelerated failure time (AFT) model was used to determine which of the AIs is associated with the ANM. Although the Cox proportional hazards model is the most popular model for the analysis of survival data and is used by most researchers in medical fields, results of accelerated failure time models have often been more valid and have had minor bias since these models have better fitness in similar conditions due to a specific statistical distribution for the survival time and their no need for PH (proportional hazard) assumption Anthropometric indices, including the ABSI, BRI, VAI, LAP, WHR, waist-to-height ratio (WHtR), BMI, and WC, were separately included in the AFT model as a time-dependent covariate. The AIs were converted to z-scores. In the AFT models, coefficients are most intuitively defined in the exponential form [time ratio (TR)]. A TR > 1 is related to prolonged survival time, whereas TR < 1 is associated with a decrease in survival time. AFT models with different parametric distributions have been applied in this analysis, and the Weibull distribution has been found as the best distribution fitted to the time to menopause onset based on Akaike information criterion (AIC). Moreover, the predictive power of the fitted models was measured by Harrell’s C and Somers’ D method.
Survival time was considered the interval between the age at the time of enrollment into the study and attained age at menopause or last follow-up. Women who did not reach menopause at the end of the follow-up period were censored. Statistical analysis was performed using the STATA software. Before doing the study, written informed consent was obtained from all participants before the study, and all procedures were approved by the Ethics Committee of the Research Institute for Endocrine sciences.
3. Results
The required number of menopause events would be 517, and a sample size of 1361 was needed. So, our sample size of 3,876 was considered to be sufficient for this study. The characteristics of the participants according to baseline, “reached menopause at the end of the follow-up” and “not reached menopause (or censored) at the end of the follow-up”. The baseline characteristics of the participants are shown in Table 1. The mean (SD) age of 3,876 subjects included in the study was 33.9 (8.9) years old. At the end of the follow-up, 1,479 (38.2%) of the participants reached menopause, and the mean (SD) age was 51.0 (4.0) years. The median (interquartile range) of the survival time (years) was 12.5 (7.9 - 15.8) years (phase 1 to 6 of TLGS study). The mean (SD) of BMI, WHR, WHtR, and ABSI were 27.0 (5.1) kg/m2, 0.8 (0.1), 0.5 (0.1), and 0.75 (0.05), respectively.
Baseline Characteristics of the Participants
Characteristics | Baseline (N = 3876) | Reached Menopause at the End of Follow-up (N = 1479) | Not reached Menopause or Censored at the End of Follow-up (N = 2397) |
---|---|---|---|
Age (y) a | 33.9 (8.9) | 51.0 (4.0) | 42.2 (6.3) |
Total cholesterol (mmol/L) a | 5.0 (1.1) | 5.2 (1.1) | 4.8 (0.9) |
High-density lipoprotein (mmol/L) a | 1.1 (0.3) | 1.3 (0.3) | 1.3 (0.3) |
Low-density lipoprotein (mmol/L) a | 3.2 (0.9) | 2.7 (6.6) | 2.0 (9.3) |
Triglyceride (mmol/L) b | 1.3 (0.9 - 1.9) | 1.6 (1.2 - 2.1) | 1.3 (0.9 - 1.8) |
Weight (kg) a | 66.5 (12.6) | 73.4 (12.5) | 71.8 (13.5) |
BMI (kg/m2) a | 27.0 (5.1) | 30.5 (5.0) | 28.7 (5.2) |
WC (cm) a | 84.3 (12.3) | 99.5 (11.6) | 91.6 (12.0) |
WHR a | 0.8 (0.1) | 0.9 (0.1) | 0.9 (0.1) |
WHtR a | 0.5 (0.1) | 0.6 (0.1) | 0.6 (0.1) |
ABSI a | 0.75 (0.05) | 0.82 (0.05) | 0.78 (0.05) |
BRI a | 4.23 (1.72) | 6.5 (2.0) | 5.1 (1.8) |
VAI b | 2.07 (1.31 - 3.43) | 2.4 (1.6 - 3.6) | 1.9 (1.2 - 2.9) |
LAP b | 32.02 (16.86 - 59.35) | 64.2 (43.4 - 94.0) | 41.0 (24.5 - 66.8) |
Parity a | 3 (1.7) | ||
Education (y) | |||
< 6 | 759 (19.6) | 355 (24) | 316 (13.2) |
6 - 12 | 2571 (66.3) | 630 (42.6) | 1950 (81.3) |
> 12 | 546 (14.1) | 494 (33.4) | 131 (5.5) |
Ever smoker (yes) | 139 (3.6) | 80 (5.4) | 131 (5.5) |
Physical activity (yes) | 1076 (27.8) | 447 (30.2) | 569 (23.7) |
Table 2 shows the TR and corresponding 95% CI for AIs z-score estimated using Weibull AFT models regarding the ANM-related data. After adjusting, it was revealed that the TR (95% CI) for ANM associated with ABSI z-score and LAP z-score were 0.98 (0.97, 0.99) and 0.98 (0.98, 0.99), respectively; indicating that per one SD increment of ABSI and LAP z-scores, the median time to natural menopause decreases by about 2%. BMI z-score was positively associated with ANM [TR: 1.01, 95% CI: (1.00, 1.02)], which showed per one SD increase of BMI, the median time to natural menopause increases by 1% times.
Time Ratio and Corresponding 95% Confidence Interval for Anthropometric Indices z-Scores Estimated Using Weibull AFT models a
Anthropometric Indices z-Scores | Model 1 b | Model 2 c | ||||||||
---|---|---|---|---|---|---|---|---|---|---|
TR | 95% CI | P-Value | AIC | TR | 95% CI | P-Value | AIC | Harrell’s C Index Difference | P-Value for Harrell’s C Index Difference | |
ABSI z-score | 0.98 | (0.97, 0.99) | < 0.001 | 1631.9 | 0.98 | (0.97, 0.99) | < 0.001 | 1419.5 | 0.003 | 0.5 |
BRI z-score | 1.00 | (0.99, 1.01) | 0.9 | 1633.1 | 1.00 | (0.99, 1.01) | 0.6 | 1420.2 | 0.001 | 0.6 |
VAI z-score | 0.99 | (0.98, 1.0) | 0.5 | 1624.7 | .99 | (0.98, 1.00) | 0.4 | 1413.3 | 0.004 | 0.6 |
LAP z-score | 0.99 | (0.98, 0.99) | 0.03 | 1624.1 | 0.98 | (0.98, 0.99) | 0.01 | 1413.5 | 0.003 | 0.8 |
WHR z-score | 0.99 | (0.98, 1.00) | 0.2 | 1633.6 | 0.99 | (0.98, 1.00) | 0.2 | 1420.1 | 0.004 | 0.8 |
WHtR z-score | 1.00 | (0.99, 1.01) | 0.9 | 1633.1 | 1.00 | (0.99, 1.01) | 0.5 | 1421.1 | 0.002 | 0.6 |
BMI z-score | 1.01 | (1.00, 1.02) | 0.02 | 1634.9 | 1.01 | (1.00, 1.03) | 0.01 | 1421.4 | 0.004 | 0.7 |
WC z-score | 1.00 | (0.99, 1.01) | 0.9 | 1633.1 | 1.00 | (0.99, 1.01) | 0.5 | 1420.9 | 0.001 | 0.9 |
4. Discussion
The results of this large cohort study on the Iranian population conducted over 15 years of follow-up showed that LAP and ABSI are inversely associated with the ANM, while it is positively associated with BMI.
Our results showed that the ABSI z-score is inversely associated with the ANM so that, 1 SD increase in the ABSI z-score leads to a 2% decrease in a median time of natural menopause. Recently, ABSI has been introduced as a strong AI that can better show the health status and predict early atherosclerosis (24). Several studies have investigated the association between the ABSI and NCDs, but they are mainly observational (25, 26). ABSI provides a valuable description regarding visceral fat (26). Besides, it can show information about the fat-free mass in people with similar BMI (27). Furthermore, ABSI is inversely associated with the fat-free mass in obese men and women such that higher ABSI is associated with lower fat-free mass (27). Remarkably, this AI is supposed as a better predictor than the BMI for all-cause mortality and diabetes (17, 25). A relationship has also been reported between the ABSI and CM risk factors, serum vitamin D, and HDL-C (28, 29).
The LAP is an advanced index of the visceral lipid that can be used to assess the CM risk factors (30). Our results showed that the LAP z-score is inversely associated with the ANM. So, one SD increase in the LAP leads to an almost 2% decrease in the median time to natural menopause. The LAP is considered a reliable predictor for the assessment of metabolic profiles and CVD risks among the healthy population (31-33). LAP has also been introduced as a novel AI to predict the risk of metabolic syndrome (34). It has been found that the LAP could be a better parameter for the assessment of the association between lipid accumulation and diabetes (as a CVD risk factor) compared to other AIs (35).
The findings of a systematic review have shown an unclear association between BMI and ANM (36). Our findings showed that the BMI, as the most common AI indicator, is directly associated with the ANM such that one SD increase in the BMI z-score leads to a 1% increase in a median time of natural menopause. Zhu et al., in a study conducted on 24,196 women with natural menopause, reported that the risk of early menopause increases by two times in underweight women, whereas overweight and obesity are associated with more than 50% higher risk of undergoing late menopause (ANM ≥ 56 years) (37).
In fact, BMI is the most commonly used adiposity indicator. Nevertheless, it has several limitations, including not providing comprehensive information on the adiposity, distribution of the body fat, body shape, and the factors associated with the risk of obesity-related NCDs (38-40). The association between BMI and ANM has been investigated previously, however, the results of a systematic review have revealed an unclear association in this regard (36).
4.1. Strengths and Limitation
The strength of our study was using an AFT model in a sample of women selected from an ongoing population-based cohort with an average follow-up of 12.5 years and adjusting the results for the main known confounders. AFT regression gives more valid results and minor bias than PH cox models due to a specific statistical distribution for the survival time (41-43). The ANM was determined retrospectively so that the women were asked to remember the time of their last menstrual cycle within three years since their last interview. However, the recordings regarding the time of menopause onset were believed to be sufficiently valid as it has been shown that 70% of women can accurately recall the time of their menopause onset within a year after the event (44). Although the results of this study showed a statistically significant relationship between some AIs and ANM; however, due to the small values of this association, it may not be clinically valuable. It should be noted about 2,397 (61.8%) participants did not reach menopause at the end of the follow-up; however, the ongoing characteristic of TLGS enables us to upgrade our results in the future.
4.2. Conclusion
The results of the present longitudinal cohort community-based study revealed that the ABSI and LAP might be considered new AIs for the assessment of ANM. These new AIs were inversely associated with the ANM. On the contrary, BMI, as a traditional AI, was directly associated with the ANM. However, since the risk of some diseases, especially NCDs, is increased following menopause, it is recommended that clinicians pay more attention to the association between AI and ANM for detecting age at menopause, also doing screening and special caring after menopause. We recommend other studies should be carried out among other populations for a longer time, and the results should be compared with the current results.
Acknowledgements
References
-
1.
Farahmand M, Tehrani FR, Pourrajabi L, Najafi M, Azizi F. Factors associated with menopausal age in Iranian women: Tehran lipid and glucose study. J Obstet Gynaecol Res. 2013;39(4):836-41. [PubMed ID: 23279558]. https://doi.org/10.1111/j.1447-0756.2012.02050.x.
-
2.
Gold EB. The timing of the age at which natural menopause occurs. Obstet Gynecol Clin North Am. 2011;38(3):425-40. [PubMed ID: 21961711]. [PubMed Central ID: PMC3285482]. https://doi.org/10.1016/j.ogc.2011.05.002.
-
3.
Schottenfeld D, Fraumeni JF. Cancer epidemiology and prevention. Oxford, UK: Oxford University Press; 2006.
-
4.
Donley GM, Liu WT, Pfeiffer RM, McDonald EC, Peters KO, Tucker MA, et al. Reproductive factors, exogenous hormone use and incidence of melanoma among women in the United States. Br J Cancer. 2019;120(7):754-60. [PubMed ID: 30814688]. [PubMed Central ID: PMC6461881]. https://doi.org/10.1038/s41416-019-0411-z.
-
5.
Abdulnour J, Doucet E, Brochu M, Lavoie JM, Strychar I, Rabasa-Lhoret R, et al. The effect of the menopausal transition on body composition and cardiometabolic risk factors: A Montreal-Ottawa new emerging team group study. Menopause. 2012;19(7):760-7. [PubMed ID: 22395454]. https://doi.org/10.1097/gme.0b013e318240f6f3.
-
6.
Lovejoy JC, Champagne CM, de Jonge L, Xie H, Smith SR. Increased visceral fat and decreased energy expenditure during the menopausal transition. Int J Obes (Lond). 2008;32(6):949-58. [PubMed ID: 18332882]. [PubMed Central ID: PMC2748330]. https://doi.org/10.1038/ijo.2008.25.
-
7.
Goodpaster BH, Krishnaswami S, Harris TB, Katsiaras A, Kritchevsky SB, Simonsick EM, et al. Obesity, regional body fat distribution, and the metabolic syndrome in older men and women. Arch Intern Med. 2005;165(7):777-83. [PubMed ID: 15824297]. https://doi.org/10.1001/archinte.165.7.777.
-
8.
Goodpaster BH, Thaete FL, Simoneau JA, Kelley DE. Subcutaneous abdominal fat and thigh muscle composition predict insulin sensitivity independently of visceral fat. Diabetes. 1997;46(10):1579-85. [PubMed ID: 9313753]. https://doi.org/10.2337/diacare.46.10.1579.
-
9.
Carey DG, Jenkins AB, Campbell LV, Freund J, Chisholm DJ. Abdominal fat and insulin resistance in normal and overweight women: Direct measurements reveal a strong relationship in subjects at both low and high risk of NIDDM. Diabetes. 1996;45(5):633-8. [PubMed ID: 8621015]. https://doi.org/10.2337/diab.45.5.633.
-
10.
Gomez-Ambrosi J, Silva C, Galofre JC, Escalada J, Santos S, Millan D, et al. Body mass index classification misses subjects with increased cardiometabolic risk factors related to elevated adiposity. Int J Obes (Lond). 2012;36(2):286-94. [PubMed ID: 21587201]. https://doi.org/10.1038/ijo.2011.100.
-
11.
Nevill AM, Stewart AD, Olds T, Holder R. Relationship between adiposity and body size reveals limitations of BMI. Am J Phys Anthropol. 2006;129(1):151-6. [PubMed ID: 16270304]. https://doi.org/10.1002/ajpa.20262.
-
12.
Ruhl CE, Everhart JE. Trunk fat is associated with increased serum levels of alanine aminotransferase in the United States. Gastroenterology. 2010;138(4):1346-56. 1356 e1-3. [PubMed ID: 20060831]. [PubMed Central ID: PMC2847039]. https://doi.org/10.1053/j.gastro.2009.12.053.
-
13.
Taylor RW, Jones IE, Williams SM, Goulding A. Evaluation of waist circumference, waist-to-hip ratio, and the conicity index as screening tools for high trunk fat mass, as measured by dual-energy X-ray absorptiometry, in children aged 3-19 y. Am J Clin Nutr. 2000;72(2):490-5. [PubMed ID: 10919946]. https://doi.org/10.1093/ajcn/72.2.490.
-
14.
Klein S, Allison DB, Heymsfield SB, Kelley DE, Leibel RL, Nonas C, et al. Waist circumference and cardiometabolic risk: A consensus statement from shaping America's health: Association for Weight Management and Obesity Prevention; NAASO, the Obesity Society; the American Society for Nutrition; and the American Diabetes Association. Obesity (Silver Spring). 2007;15(5):1061-7. [PubMed ID: 17495180]. https://doi.org/10.1038/oby.2007.632.
-
15.
Jacobs EJ, Newton CC, Wang Y, Patel AV, McCullough ML, Campbell PT, et al. Waist circumference and all-cause mortality in a large US cohort. Arch Intern Med. 2010;170(15):1293-301. [PubMed ID: 20696950]. https://doi.org/10.1001/archinternmed.2010.201.
-
16.
Czernichow S, Kengne AP, Stamatakis E, Hamer M, Batty GD. Body mass index, waist circumference and waist-hip ratio: which is the better discriminator of cardiovascular disease mortality risk?: evidence from an individual-participant meta-analysis of 82 864 participants from nine cohort studies. Obes Rev. 2011;12(9):680-7. [PubMed ID: 21521449]. [PubMed Central ID: PMC4170776]. https://doi.org/10.1111/j.1467-789X.2011.00879.x.
-
17.
Krakauer NY, Krakauer JC. A new body shape index predicts mortality hazard independently of body mass index. PLoS One. 2012;7(7). e39504. [PubMed ID: 22815707]. [PubMed Central ID: PMC3399847]. https://doi.org/10.1371/journal.pone.0039504.
-
18.
Krakauer NY, Krakauer JC. Dynamic association of mortality hazard with body shape. PLoS One. 2014;9(2). e88793. [PubMed ID: 24586394]. [PubMed Central ID: PMC3930607]. https://doi.org/10.1371/journal.pone.0088793.
-
19.
Thomas DM, Bredlau C, Bosy-Westphal A, Mueller M, Shen W, Gallagher D, et al. Relationships between body roundness with body fat and visceral adipose tissue emerging from a new geometrical model. Obesity (Silver Spring). 2013;21(11):2264-71. [PubMed ID: 23519954]. [PubMed Central ID: PMC3692604]. https://doi.org/10.1002/oby.20408.
-
20.
Maessen MF, Eijsvogels TM, Verheggen RJ, Hopman MT, Verbeek AL, de Vegt F. Entering a new era of body indices: The feasibility of a body shape index and body roundness index to identify cardiovascular health status. PLoS One. 2014;9(9). e107212. [PubMed ID: 25229394]. [PubMed Central ID: PMC4167703]. https://doi.org/10.1371/journal.pone.0107212.
-
21.
Ramezani Tehrani F, Minooee S, Azizi F. Comparison of various adiposity indexes in women with polycystic ovary syndrome and normo-ovulatory non-hirsute women: A population-based study. Eur J Endocrinol. 2014;171(2):199-207. [PubMed ID: 24816947]. https://doi.org/10.1530/EJE-14-0094.
-
22.
Farahmand M, Ramezani Tehrani F, Simbar M, Mehrabi Y, Khalili D, Azizi F. Does metabolic syndrome or its components differ in naturally and surgically menopausal women? Climacteric. 2014;17(4):348-55. [PubMed ID: 24188285]. https://doi.org/10.3109/13697137.2013.856400.
-
23.
Word Health Organization. Research on the menopause. Geneva, Switzerland: Word Health Organization; 1999.
-
24.
Nimkuntod P, Tongdee P. A body shape index versus traditional anthropometric parameters to identify subclinical atherosclerosis in perimenopausal/menopausal women. J Med Assoc Thai. 2016;99 Suppl 7:S81-6. [PubMed ID: 29901934].
-
25.
Bawadi H, Abouwatfa M, Alsaeed S, Kerkadi A, Shi Z. Body shape index is a stronger predictor of diabetes. Nutrients. 2019;11(5). [PubMed ID: 31067681]. [PubMed Central ID: PMC6566958]. https://doi.org/10.3390/nu11051018.
-
26.
Gomez-Peralta F, Abreu C, Cruz-Bravo M, Alcarria E, Gutierrez-Buey G, Krakauer NY, et al. Relationship between "a body shape index (ABSI)" and body composition in obese patients with type 2 diabetes. Diabetol Metab Syndr. 2018;10:21. [PubMed ID: 29568333]. [PubMed Central ID: PMC5859756]. https://doi.org/10.1186/s13098-018-0323-8.
-
27.
Biolo G, Di Girolamo FG, Breglia A, Chiuc M, Baglio V, Vinci P, et al. Inverse relationship between "a body shape index" (ABSI) and fat-free mass in women and men: Insights into mechanisms of sarcopenic obesity. Clin Nutr. 2015;34(2):323-7. [PubMed ID: 24814384]. https://doi.org/10.1016/j.clnu.2014.03.015.
-
28.
Zamaninour N, Ansar H, Pazouki A, Kabir A. Relationship between modified body adiposity index and a body shape index with biochemical parameters in bariatric surgery candidates. Obes Surg. 2020;30(3):901-9. [PubMed ID: 31898041]. https://doi.org/10.1007/s11695-019-04256-x.
-
29.
Sousa-Santos AR, Afonso C, Santos A, Borges N, Moreira P, Padrao P, et al. The association between 25(OH)D levels, frailty status and obesity indices in older adults. PLoS One. 2018;13(8). e0198650. [PubMed ID: 30153256]. [PubMed Central ID: PMC6112621]. https://doi.org/10.1371/journal.pone.0198650.
-
30.
Kahn HS. The "lipid accumulation product" performs better than the body mass index for recognizing cardiovascular risk: A population-based comparison. BMC Cardiovasc Disord. 2005;5:26. [PubMed ID: 16150143]. [PubMed Central ID: PMC1236917]. https://doi.org/10.1186/1471-2261-5-26.
-
31.
Ioachimescu AG, Brennan DM, Hoar BM, Hoogwerf BJ. The lipid accumulation product and all-cause mortality in patients at high cardiovascular risk: A PreCIS database study. Obesity (Silver Spring). 2010;18(9):1836-44. [PubMed ID: 20035284]. https://doi.org/10.1038/oby.2009.453.
-
32.
Wakabayashi I, Daimon T. A strong association between lipid accumulation product and diabetes mellitus in Japanese women and men. J Atheroscler Thromb. 2014;21(3):282-8. [PubMed ID: 24304961]. https://doi.org/10.5551/jat.20628.
-
33.
Chiang JK, Koo M. Lipid accumulation product: A simple and accurate index for predicting metabolic syndrome in Taiwanese people aged 50 and over. BMC Cardiovasc Disord. 2012;12:78. [PubMed ID: 23006530]. [PubMed Central ID: PMC3506496]. https://doi.org/10.1186/1471-2261-12-78.
-
34.
Kahn HS, Valdez R. Metabolic risks identified by the combination of enlarged waist and elevated triacylglycerol concentration. Am J Clin Nutr. 2003;78(5):928-34. [PubMed ID: 14594778]. https://doi.org/10.1093/ajcn/78.5.928.
-
35.
Sun K, Lin D, Feng Q, Li F, Qi Y, Feng W, et al. Assessment of adiposity distribution and its association with diabetes and insulin resistance: A population-based study. Diabetol Metab Syndr. 2019;11:51. [PubMed ID: 31297161]. [PubMed Central ID: PMC6598265]. https://doi.org/10.1186/s13098-019-0450-x.
-
36.
Schoenaker DA, Jackson CA, Rowlands JV, Mishra GD. Socioeconomic position, lifestyle factors and age at natural menopause: A systematic review and meta-analyses of studies across six continents. Int J Epidemiol. 2014;43(5):1542-62. [PubMed ID: 24771324]. [PubMed Central ID: PMC4190515]. https://doi.org/10.1093/ije/dyu094.
-
37.
Zhu D, Chung HF, Pandeya N, Dobson AJ, Kuh D, Crawford SL, et al. Body mass index and age at natural menopause: an international pooled analysis of 11 prospective studies. Eur J Epidemiol. 2018;33(8):699-710. [PubMed ID: 29460096]. https://doi.org/10.1007/s10654-018-0367-y.
-
38.
Field AE, Coakley EH, Must A, Spadano JL, Laird N, Dietz WH, et al. Impact of overweight on the risk of developing common chronic diseases during a 10-year period. Arch Intern Med. 2001;161(13):1581-6. [PubMed ID: 11434789]. https://doi.org/10.1001/archinte.161.13.1581.
-
39.
Janssen I, Katzmarzyk PT, Ross R. Body mass index is inversely related to mortality in older people after adjustment for waist circumference. J Am Geriatr Soc. 2005;53(12):2112-8. [PubMed ID: 16398895]. https://doi.org/10.1111/j.1532-5415.2005.00505.x.
-
40.
Ross R, Berentzen T, Bradshaw AJ, Janssen I, Kahn HS, Katzmarzyk PT, et al. Does the relationship between waist circumference, morbidity and mortality depend on measurement protocol for waist circumference? Obes Rev. 2008;9(4):312-25. [PubMed ID: 17956544]. https://doi.org/10.1111/j.1467-789X.2007.00411.x.
-
41.
Knaus WA, Harrell FE, Fisher CJ, Wagner DP, Opal SM, Sadoff JC, et al. The clinical evaluation of new drugs for sepsis. A prospective study design based on survival analysis. JAMA. 1993;270(10):1233-41. [PubMed ID: 8355388].
-
42.
George B, Seals S, Aban I. Survival analysis and regression models. J Nucl Cardiol. 2014;21(4):686-94. [PubMed ID: 24810431]. [PubMed Central ID: PMC4111957]. https://doi.org/10.1007/s12350-014-9908-2.
-
43.
Moran JL, Bersten AD, Solomon PJ, Edibam C, Hunt T; Australian, et al. Modelling survival in acute severe illness: Cox versus accelerated failure time models. J Eval Clin Pract. 2008;14(1):83-93. [PubMed ID: 18211649]. https://doi.org/10.1111/j.1365-2753.2007.00806.x.
-
44.
Rodstrom K, Bengtsson C, Lissner L, Bjorkelund C. Reproducibility of self-reported menopause age at the 24-year follow-up of a population study of women in Goteborg, Sweden. Menopause. 2005;12(3):275-80. [PubMed ID: 15879916]. https://doi.org/10.1097/01.gme.0000135247.11972.b3.