Abstract
Background:
Type 2 diabetes, common and serious global health concern, had an estimated worldwide prevalence of 366 million in 2011, which is expected to rise to 552 million people, by 2030, unless urgent action is taken.Objectives:
The aim of this study was to identify risk patterns for type 2 diabetes incidence using association rule mining (ARM).Patients and Methods:
A population of 6647 individuals without diabetes, aged ≥ 20 years at inclusion, was followed for 10-12 years, to analyze risk patterns for diabetes occurrence. Study variables included demographic and anthropometric characteristics, smoking status, medical and drug history and laboratory measures.Results:
In the case of women, the results showed that impaired fasting glucose (IFG) and impaired glucose tolerance (IGT), in combination with body mass index (BMI) ≥ 30 kg/m2, family history of diabetes, wrist circumference > 16.5 cm and waist to height ≥ 0.5 can increase the risk for developing diabetes. For men, a combination of IGT, IFG, length of stay in the city (> 40 years), central obesity, total cholesterol to high density lipoprotein ratio ≥ 5.3, low physical activity, chronic kidney disease and wrist circumference > 18.5 cm were identified as risk patterns for diabetes occurrence.Conclusions:
Our study showed that ARM is a useful approach in determining which combinations of variables or predictors occur together frequently, in people who will develop diabetes. The ARM focuses on joint exposure to different combinations of risk factors, and not the predictors alone.Keywords
1. Background
Type 2 diabetes, a common and serious global health concern, had an estimated worldwide diabetes prevalence of 366 million in 2011, which is expected to rise to about 552 million people by 2030, unless urgent action is taken (1, 2). Diabetes leads to significant medical complications, including retinopathy, nephropathy, neuropathy, stroke, and myocardial infarction (3). In type 2 diabetes, cardiovascular events are responsible for 80% of all deaths (4). Several possible risk factors have been known to contribute to the development of type 2 diabetes, such as ethnicity, obesity, unhealthy diet, inactivity, insulin resistance and family history of diabetes (5). Epidemiological studies have shown that lifestyle modifications can prevent or delay development of type 2 diabetes, making the early identification of populations at high risk a major healthcare necessity (6, 7). During the past 2 decades, epidemiologists and statisticians have attempted to develop simple, reliable, affordable, and widely implementable weighted models for the prediction of future type 2 diabetes risk (7). Although firm scientific evidences for the prevention of type 2 diabetes are available, the researchers continue to look for the causes of diabetes and ways to manage, prevent, or cure the disorder (8, 9). In current medical studies, the identification of risk factors for diabetes and designing a diagnostic or prediction model are generally based on multivariate statistical analysis utilizing logistic regression (6). Huge amounts of data generated by health care systems and epidemiological studies, on the other hand, contain hidden knowledge, which is impossible to uncover by using traditional methods; using data mining, therefore, is more adapted for medical studies (10-12). Data mining, a part of the Knowledge Discovering from Databases (KDD), is an interdisciplinary active research area that is used to extract high-level knowledge from low-level data, in the context of large data sets (13). Decision trees, clustering and neural network are the most common data mining methods that have been used for pattern extraction and to develop prediction models in the medical domain. However, association rules mining (ARM), one of the most popular methods in data mining, is rarely used (14-17). The ARM is a technique used to discover associations between variables (14). Several applications of ARM in the medical domain include discovering disease co-occurrences (18), identifying adverse effects of drugs (19), public health surveillance (20), detecting risk factors for heart disease and diabetes (21, 22) and determining relations among complications or the various diseases that accompany type 2 diabetes (23).
2. Objectives
In this study, we applied ARM for extracting risk patterns associated with type 2 diabetes occurrence, using data from the Tehran Lipid and Glucose Study (TLGS).
3. Patients and Methods
3.1. Study Population
Data was obtained from the TLGS, a prospective population based study performed in district 13 of Tehran, the capital of Iran. In a baseline survey performed from 1999 to 2001 (phase 1), 15000 residents, aged ≥ 3 years, were investigated and followed during three consecutive phases with 3-year intervals [2002 to 2005 (phase 2), 2005 to 2008 (phase 3), and the last, 2009-2012 (phase 4)] (24). Another 3500 people were added in the second phase and followed in the next two phases. In our study, subjects aged ≥ 20 years, from the first and second phases, were selected. We then excluded subjects with prevalent diabetes at baseline and, also, those with missing data on fasting and 2-hour post-prandial glucose, leaving 10310 non-diabetic subjects in the first and second phases, which were followed in the next phases. Overall, 3663 (35%) subjects missed their follow-ups. A total of 729 individuals developed type 2 diabetes by the end of a 12-year follow-up (phase 4), and 5918 subjects remained diabetes-free. The final data source, hence, included 6647 people (Figure 1). The incidence of type 2 diabetes was defined based on fasting plasma glucose (FPG) ≥ 126 mg/dL or 2 h post-challenge plasma glucose (2 h PCPG) ≥ 200 mg/dL, or taking anti-diabetic medication (25).
Follow up Status of the Subjects in the Tehran Lipid and Glucose Study
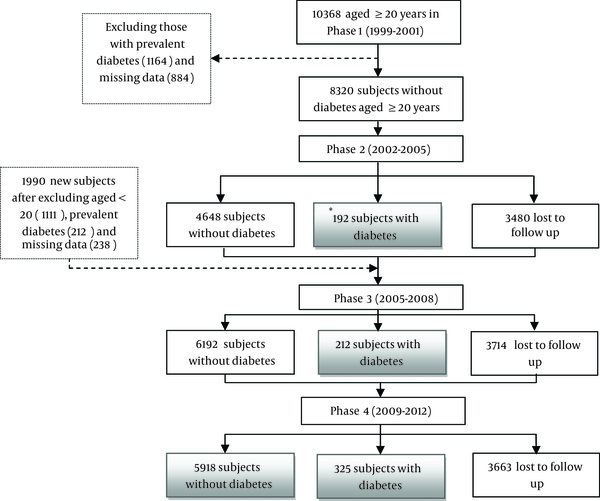
3.2. General Description of Data
The data set included demographic characteristics, like age, gender, marital status, education, and information on smoking status, physical activity, and medical and drug history, which were collected during interviews, using a pretested questionnaire. Anthropometric measures, including weight, height, waist, hip and wrist circumference, were obtained according to a standard protocol (26). Systolic and diastolic blood pressures were measured twice, on the right arm, using a standardized mercury sphygmomanometer, and the mean of the two measurements was considered as the subject's blood pressure. All blood parameters, except for 2h plasma glucose, were based on fasting blood samples (after 12-14 hours overnight fasting). Blood samples obtained were centrifuged within 30-45 minutes of collection and kept cool until analysis at the TLGS research laboratory. Fasting and 2h plasma glucose were determined, based on an enzymatic colorimetric method, using oxidase kits (Pars Azmoon Inc., Tehran, Iran) with inter- and intra-assay coefficients of variation (CV), both < 2.2%. Triglycerides (TGs), total and high density lipoprotein (HDL) cholesterol were measured, using methods described elsewhere (27, 28).
3.3. Pre-Processing and Transformation of Data
In order to mine the dataset for association rules, all the variables should be transformed to the binary form. We had two types of variables for analysis, numeric and categorical. Numeric variables were categorized using the well-known medical cutoffs into intervals and then each interval was mapped to an item (binary variable). Age and total length of stay in the city were categorized into four groups (Table 1). Chronic kidney disease (CKD) was considered as an estimated glomerular filtration fate (GFR) below 60 mL/min/1.73 m2 (29). For categorization of lipid profiles (triglycerides, HDL, total cholesterol, cholesterol to HDL ratio, and triglyceride to HDL ratio) we used the cutoff value for prediction of diabetes in Iranian populations (30). Waist circumference was categorized using the cutoff value for Iranian men and women to predict incident cardiovascular diseases (31). Body mass index (BMI), waist-to-hip ratio (WHR), waist-to-height ratio (WHtR), blood pressure (BP), fasting plasma glucose (FPG) and 2 h plasma glucose were categorized using the World Health Organization (WHO) cutoffs for definition of metabolic syndrome, overweight and obesity (32). As there is no definite cutoff point for wrist circumferences, we categorized it in men and women using the equal frequency binning method. In this method, individuals were divided into three groups, so that each group contains the same number of individuals (Table 1). For other categorical variables, each category was mapped to an item. In summary, each row of data is a set of items and each item corresponds to the presence or absence of one category or numerical interval value of the variables.
Variables | Cutoff Value | Values b |
---|---|---|
Age, y | ||
Group 1 | 20-34 | 2525 (37.98) |
Group 2 | 35-49 | 2444 (36.76) |
Group 3 | 50-64 | 1310 (19.70) |
Group 4 | ≥ 65 | 368 (5.53) |
Total length of stay in the city, y | ||
Group 1 | < 20 | 609 (9.2) |
Group 2 | 20-39 | 3884 (58.4) |
Group 3 | ≥ 40 | 2131 (32.1) |
Education | ||
Group 1 | ≥ 13 years | 990 (14.89) |
Group 2 | 6-12 years | 3877 (58.32) |
Group 3 | ≤ 5 years | 1780 (26.77) |
Gender | ||
Female | - | 3762 (56.59) |
Male | - | 2885 (43.40) |
Occupation | ||
Employed | - | 2718 (40.89) |
Housekeeping (for females) | - | 3041 (45.74) |
Student | - | 215 (3.23) |
Unemployed | - | 642 (9.65) |
Other | - | 31 (0.49) |
Marital status | ||
Divorced | - | 55 (0.82) |
Married | - | 5512 (82.92) |
Single (unmarried) | - | 869 (13.07) |
Widowed | - | 210 (3.15) |
3.4. Analysis Method
3.4.1. Association Rules Mining
The ARM is a fundamental data mining technique that exhaustively looks for hidden patterns, making them ideal for the discovery of predictive rules from medical databases (33, 34). An association rule (AR) is a pair (X, Y) of sets of attributes, denoted by X → Y, where X is the antecedent and Y is the consequent of the rule X → Y. Basically, the rule states that if X happens, then Y does happen. In general, a set of items, such as X or Y, which are disjoint, is called an item set (33). Applied to a medical condition, association rules can identify subpopulations at particularly high risk of a given disease. They are interpretable, and suggest interactions between risk factors (35). Association rules are generated in two steps. First, a set of frequent item sets, or patterns, are generated. Second, these patterns can be used for generation of association rules (33, 36). Support and confidence are two measures of statistical significance and strength of a rule, respectively (21, 33). The support of a rule (X → Y) is defined as the percentage of records (rows) in the dataset that contain both X and Y (XUY). Confidence of a rule (X → Y) is the percentage of records in a dataset containing X that also contain Y (33). The correlation between X and Y is measured by the lift value, which is given as follows: The lift value is simply the ratio of the posterior and the prior confidence of an association rule. Consider the number of samples (records) in our database is “θ”, if " θ → diabetes" has a confidence of 10% and "X → Y" has a confidence of 70%, then the lift value (of the second rule) is 70/10 = 7. Obviously, if the posterior confidence equals the prior confidence, the value of this measure is 1. If the posterior confidence is greater than the prior confidence, the lift value exceeds 1 (the presence of the antecedent items raises the confidence), and if the posterior confidence is less than the prior confidence, the lift value is less than 1 (the presence of the antecedent items lowers the confidence). More formally, the lift of the rule (X → Y) is (Equation 1):

Supp (θ) = the number of records in database (33, 37).
Typically, association rules are considered interesting if they satisfy both a minimum support threshold and a minimum confidence threshold. Such thresholds can be set by users or domain experts, while the lift value cannot be set by user (16). For the current study, we used the Apriori algorithm, the best-known and standard approach for discovering association rules in a cohort dataset (16). The algorithm includes two separate steps. In the first, minimum support is applied to identify all frequent item sets in a database. In the second step, these frequent item sets and the minimum confidence are used to generate rules (38). We considered support, confidence and lift as measures of interestingness and performance of the rules (39). As the goal of our study was to relate predictor variables to diabetes occurrence, we therefore, limited all predictor variables, to appear only in the antecedent (IF part), and diabetes occurrence (outcome variable) to appear only in the consequent (THEN part). To generate strong association rules, we started our analysis from initial support 2% (which encompasses about 20% of diabetic subjects), and confidence thresholds 75% (based on acceptable level of sensitivity for prediction models), for the generation of frequent item sets and rule induction. We also set the number of item sets to five, in the antecedents of rules; two types of rules were extracted for males and females, separately. We used SPSS modeler 14.2 (IBM, Armonk, NY, USA) to apply an association rule algorithm.
4. Results
A total of 6647 persons participated in the study, with more than 10 years follow up. The study included 42 input variables (Tables 1-5). The occurrence of diabetes during the follow-up period was 11% (427 cases) and 10% (302 cases) in females and males, respectively.
Variables | Cutoff Value | Values b |
---|---|---|
Waist circumference, cm | ||
Normal | < 90 | 3554 (53.47) |
Abnormal | ≥ 90 | 2914 (43.84) |
Wrist circumference, cm | ||
Women | ||
Group 1 | < 15.5 | 1058 (28.12) |
Group 2 | 15.5-16.5 | 1357 (36.07) |
Group 3 | > 16.5 | 1252 (33.28) |
Men | ||
Group 1 | < 17.2 | 934 (32.37) |
Group 2 | 17.2-18 | 806 (27.94) |
Group 3 | > 18 | 1109 (38.44) |
Waist to height ratio | ||
Normal | < 0.5 | 785 (11.80) |
Abnormal | ≥ 0.5 | 5683 (85.49) |
Waist to hip ratio | ||
Men | ||
Normal | < 0.9 | 476 (16.49) |
Abnormal | ≥ 0.9 | 2373 (82.25) |
Women | ||
Normal | < 0.85 | 2172 (57.73) |
Abnormal | ≥ 0.85 | 1447 (38.46) |
Body Mass Index, kg/ m2 | ||
Normal | < 25 | 2364 (35.56) |
Overweight | 25-30 | 2702 (40.64) |
Obese | ≥ 30 | 1424 (21.42) |
Variables | Cutoff Value | Values c |
---|---|---|
Fasting Plasma Glucose, mg/dL | ||
Normal | < 100 | 946 (14.23) |
Impaired Fasting Glucose | 100-126 | 5701 (85.76) |
Two hour postprandial plasma glucose, mg/dL | ||
Normal | < 140 | 5848 (87.97) |
Impaired Glucose Tolerance | 140-200 | 799 (12.02) |
Total cholesterol, mg/dL | ||
Normal | < 200 | 3382 (50.88) |
Hypercholesterolemia | ≥ 200 | 3265 (49.11) |
Triglyceride Levels, mg/dL | ||
Normal | < 150 | 3690 (55.51) |
Hypertriglyceridemia | ≥150 | 2957 (44.48) |
Cholesterol to High Density lipoprotein Ratio | ||
Normal | < 5.3 | 3841 (57.78) |
Abnormal | ≥ 5.3 | 2801 (42.13) |
High Density Lipoprotein, mg/dL | ||
Men | ||
Low | < 40 | 1893 (65.61) |
Normal | ≥ 40 | 990 (34.31) |
Women | ||
Low | < 50 | 2747 (73.01) |
Normal | ≥ 50 | 1012 (26.90) |
Triglyceride to High Density Lipoprotein Ratio | ||
Men | ||
Normal | < 4.7 | 1647 (57.08) |
Abnormal | ≥ 4.7 | 1236 (42.84) |
Women | ||
Normal | < 3.7 | 2358 (62.67) |
Abnormal | ≥ 3.7 | 1401 (37.24) |
Chronic Kidney Disease | ||
CKD | GFR < 60 | 2262 (34.03) |
Non CKD | GFR ≥ 60 | 4382 (65.92) |
Systolic Blood Pressure, mm Hg | ||
Normal | < 140 | 5946 (89.45) |
Hypertension | ≥ 140 | 613 (9.22) |
Diastolic Blood Pressure, mm Hg | ||
Normal | < 90 | 5852 (88.04) |
Hypertension | ≥ 90 | 707 (10.64) |
Variables | Values b |
---|---|
History of hospitalization until now | |
Yes | 4566 (68.69) |
No | 2081 (31.30) |
History of ischemic heart disease | |
Yes | 173 (2.60) |
No | 6474 (97.40) |
History of non-ischemic heart disease | |
Yes | 295 (4.43) |
No | 6352 (95.57) |
History of hypertension | |
Yes | 725 (10.90) |
No | 5922 (89.10) |
History of hyperlipidemia | |
Yes | 1161 (17.46) |
No | 5486 (82.54) |
Family history of cardiovascular disease in male relatives (father, brother, son) aged under 55 | |
Yes | 566 (8.51) |
No | 6081 (91.49) |
Family history of cardiovascular disease in female relatives (mother, sister, daughter) aged under 65 | |
Yes | 523 (7.86) |
No | 6124 (92.14) |
Family history of diabetes in first-degree relatives | |
Yes | 1731 (26.04) |
No | 4916 (73.96) |
Goiter Status | |
Grade 1and 2 | 1764 (26.53) |
No goiter | 4883 (73.47) |
Thyroid nodules | |
Yes | 393 (5.91) |
No | 6254 (94.09) |
Variables | Values b |
---|---|
Current cigarette smoking | |
Yes (daily / occasionally) | 830 (12.48) |
No | 5817 (87.52) |
Former cigarette smoking | |
Yes (daily / occasionally) | 489 (7.35) |
No | 6158 (92.65) |
Exposed to second hand smoke at home or at work | |
Yes | 1690 (25.42) |
No | 4957 (74.57) |
Physical activity levels | |
Low (doing exercise or labor less than three times a week) | 4426 (66.58) |
Normal (doing exercise or labor more than three times in a week) | 2221 (33.42) |
Use of diet or exercise for the management of hyperlipidemia | |
Yes | 559 (8.40) |
No | 6088 (91.59) |
Use of diet or exercise for the management of hypertension | |
Yes | 319 (4.79) |
No | 6328 (95.20) |
Use of antihypertensive drugs in the past month | |
Yes | 324 (4.87) |
No | 6323 (95.12) |
Use of lipid lowering drugs in the past month | |
Yes | 150 (2.25) |
No | 6497 (97.74) |
Use of diuretic drugs in the past month | |
Yes | 112 (1.68) |
No | 6535 (98.32) |
Use of thyroid drugs in the past month | |
Yes (ordered / unordered) | 166 (2.49) |
No | 6481 (97.51) |
Use of aspirin in the past month | |
Yes (ordered / unordered) | 178 (2.67) |
No | 6469 (97.33) |
4.1. Results of Association Rules Mining Using the Apriori Algorithm
With initial support and confidence thresholds of 2% and 75%, respectively, we could not find any rule for men. Hence, we reduced the thresholds for support and confidence by 0.2 on each run. Finally, with a threshold value of 1.8% and 65% for support and confidence, respectively, the Apriori discovered a set of rules for men. Since such thresholds were acceptable by experts, we set the support and confidence to the mentioned values. A total of 480 rules were discovered for females. Due to the finding of a large number of rules in females, we had to report a number of the most interesting rules. As both support and confidence were important in our study, we could not select the rules based on only one of them. Therefore, considering a tradeoff between two measures, we reported rules above a threshold 2% and 75% for support and confidence, respectively. For several of the rules that were subsets of other rules, and with the same confidence and support, we reported the rule with more item sets in the IF Part. After that, we were left with a collection of seven association rules for females, shown in Table 6. For males, a total of four rules were found (Table 7).
Rule Number | Antecedent | Consequent | Support b | Confidence c | Lift d |
---|---|---|---|---|---|
1 | IFG = yes, IGT = yes, BMI ≥ 30, waist to height ≥ 0.5 | Type 2 DM | 2.8 | 75.0 | 6.6 |
2 | IFG = yes, IGT = yes, BMI ≥ 30, Marital status = Married, waist to height ≥ 0.5 | Type 2 DM | 2.4 | 75.0 | 6.6 |
3 | IFG = yes, IGT = yes, BMI ≥ 30, HDL < 50, waist to height ≥ 0.5 | Type 2 DM | 2.3 | 75.6 | 6.7 |
4 | IFG = yes, IGT = yes, BMI ≥ 30, wrist circumference ≥ 16.5, waist to height ≥ 0.5 | Type 2 DM | 2.2 | 76.5 | 6.7 |
5 | IFG = yes, IGT = yes, , BMI ≥ 30, waist to hip ≥ 0.85, waist to height ≥ 0.5 | Type 2 DM | 2.2 | 76.5 | 6.7 |
6 | IFG = yes, IGT = yes, Family history of diabetes = yes, waist to height ≥ 0.5 | Type 2 DM | 2.1 | 78.2 | 6.9 |
7 | IFG = yes, IGT = yes , BMI ≥ 30, HDL< 50, Marital status = Married | Type 2 DM | 2.1 | 75.6 | 6.7 |
Rule Number | Antecedent | Consequent | Support b | Confidence c | Lift d |
---|---|---|---|---|---|
1 | IGT= yes, IFG= yes, CHO to HDL ≥ 5.3, occupation status= employed, waist to hip ≥ 0.9 | Type 2 DM | 2.2 | 65.1 | 6.2 |
2 | IGT= yes, IFG= yes, length of stay in the city ≥ 40, wrist circumference ≥ 18, waist to hip ≥ 0.9 | Type 2 DM | 1.9 | 66.1 | 6.3 |
3 | IGT= yes, IFG= yes, and CKD= yes, Physical activity levels = low, waist to hip ≥ 0.9 | Type 2 DM | 1.8 | 65.4 | 6.2 |
4 | IGT= yes, IFG= yes, wrist circumference ≥ 18, occupation status= employed , waist to height ≥ 0.5 | Type 2 DM | 1.8 | 69.2 | 6.6 |
5. Discussion
This study describes a rule extraction experiment on a cohort database, using ARM. We analyzed the association of baseline characteristics and diabetes occurrence in the study population. As we limited diabetes occurrence to appear only in the consequent part, all variables, which appear in the antecedent, are the predictor and observed pattern, or a combination of predictors, known as the risk pattern. Generated rules can be interpreted as the conditional probability of diabetes incidence within the special subpopulation. For example, in Table 6, rule 1 (IFG = yes, IGT = yes, BMI ≥ 30 kg/m2, waist to height ≥ 0.5) can be interpreted as: The probability of diabetes in women who are obese and have IFG, IGT and waist to height ratio ≥ 0.5 is 75%. Lift value of this rule can be interpreted as ratio of this probability (75%) to probability of diabetes in all the population of the database. Since the prior probability of diabetes in the female database is 11%, the lift value will be 6.6 (75% / 11.3%) for this rule. Association rule mining revealed several interesting patterns or relations between variables. The results showed that for women, IFG and IGT repeated in six rules (Table 6), whereas no rule was found containing only these two items in the antecedent part by the defined threshold. This shows that in women, several predisposing factors simultaneously affect the development of diabetes, besides IFG and IGT. Obesity in women is the most important risk factor, and combined with IFG and IGT, it can progress to type 2 diabetes, because it has appeared in six of the seven rules. Family history of diabetes and wrist circumference greater than 16.5 cm appeared in two rules (Table 6, Rules 6 and 4). No study has yet prospectively examined wrist circumference, as a predictor for diabetes occurrence, in an adult population. There is just one study conducted on TLGS data that has shown that wrist circumference is a significant predictor for diabetes, in both genders of adult population (40). The overall cutoff for wrist circumference in that study was found to be 15.7 cm in women. In our study, we categorized wrist circumference in three equal count groups. The related rule (Table 6, Rule 4) showed that wrist circumference >16.5 cm in a group with IFG, IGT, BMI ≥ 30 kg/m2 and waist to height ≥ 0.5 associate with diabetes occurrence. For men (Table 7), results show that IGT and IFG are also two frequent items, which are repeated in all the rules. Comparison of these results with the risk patterns in women showed that items, such as length of stay > 40 years in the city, central obesity, cholesterol to HDL ratio, low physical activity, CKD and wrist circumference > 18.5 cm are potential risk patterns for men. Previous studies in adults have shown the predictability of most of these factors in diabetes occurrence (7, 41). However, only one study in men has shown predictability of wrist circumference in diabetes occurrence (40). Unlike women, among men, high wrist circumference was observed in combinations with central obesity (Table 7). Generally, the rules discovered in the present study showed different risk patterns for males and females. Previous studies have identified several of these variables as risk factors for the development of type 2 diabetes (6). Our study uncovered novel data into diabetes research. Most of the discovered rules had lift values greater than 6, and confidence levels above 75%. These association rules are easily interpretable, and could be designed to provide support to the healthcare professional (35, 42). When choosing ARM for extracting rules, several points should be considered. For instance, changing support and confidence level will produce different rules or patterns. Therefore, obtaining the desired supports and confidence level for extracting interesting rules requires a close relationship between the medical researcher and data miners. Also, it should be considered that selecting cutoffs for categorizing variables is the most important step in data mining method, specially extracting rules by association rules mining (43). The strength of this study is that we used a prospective large data set for extraction of predictive rules. We also used well known cut points for categorizing of continuous variables, which can be interpreted easily by users. Also, we entered a large number of variables in the study, which would be impossible in the case of traditional methods. There are several limitations in the current study over not considering all possible predictors, such as sociological factors (44), or several nutritional factors (45). It should be mentioned that several risk factors could be changed, treated, or modified, during the study period. Therefore, as our next step in this context, we intend to identify the temporal or sequential pattern for predicting type 2 diabetes. The results of this study showed the usefulness of ARM for extracting risk patterns from a large prospective database. Our study showed that ARM is a useful approach in determining which combinations of variables or predictors occur together frequently, in people who will develop diabetes. Although most of the variables in the extracted patterns had been known in previous studies, as a predictors of diabetes, the ARM focuses on joint exposure to different combinations of risk factors, and not the predictors alone.
Acknowledgements
References
-
1.
Shaw JE, Sicree RA, Zimmet PZ. Global estimates of the prevalence of diabetes for 2010 and 2030. Diabetes Res Clin Pract. 2010;87(1):4-14. [PubMed ID: 19896746]. https://doi.org/10.1016/j.diabres.2009.10.007.
-
2.
Whiting DR, Guariguata L, Weil C, Shaw J. IDF diabetes atlas: global estimates of the prevalence of diabetes for 2011 and 2030. Diabetes Res Clin Pract. 2011;94(3):311-21. [PubMed ID: 22079683]. https://doi.org/10.1016/j.diabres.2011.10.029.
-
3.
Emerging Risk Factors C, Sarwar N, Gao P, Seshasai SR, Gobin R, Kaptoge S, et al. Diabetes mellitus, fasting blood glucose concentration, and risk of vascular disease: a collaborative meta-analysis of 102 prospective studies. Lancet. 2010;375(9733):2215-22. [PubMed ID: 20609967]. https://doi.org/10.1016/S0140-6736(10)60484-9.
-
4.
Booth GL, Kapral MK, Fung K, Tu JV. Relation between age and cardiovascular disease in men and women with diabetes compared with non-diabetic people: a population-based retrospective cohort study. Lancet. 2006;368(9529):29-36. [PubMed ID: 16815377]. https://doi.org/10.1016/S0140-6736(06)68967-8.
-
5.
Diabetes atlas. 3th ed. Brussels: International Diabetes Federation; 2006.
-
6.
Abbasi A, Peelen LM, Corpeleijn E, van der Schouw YT, Stolk RP, Spijkerman AM, et al. Prediction models for risk of developing type 2 diabetes: systematic literature search and independent external validation study. BMJ. 2012;345. ee5900. [PubMed ID: 22990994]. https://doi.org/10.1136/bmj.e5900.
-
7.
Noble D, Mathur R, Dent T, Meads C, Greenhalgh T. Risk models and scores for type 2 diabetes: systematic review. BMJ. 2011;343:d7163. [PubMed ID: 22123912]. https://doi.org/10.1136/bmj.d7163.
-
8.
Uusitupa M, Tuomilehto J, Puska P. Are we really active in the prevention of obesity and type 2 diabetes at the community level? Nutr Metab Cardiovasc Dis. 2011;21(5):380-9. [PubMed ID: 21470836]. https://doi.org/10.1016/j.numecd.2010.12.007.
-
9.
U.S. Department of Health and Human Services. National Diabetes Information Clearinghouse (NDIC) National Diabetes Statistics. National Diabetes Statistics; 2011.
-
10.
Koh HC, Tan G. Data mining applications in healthcare. J Healthc Inf Manag. 2011;19(2):65.
-
11.
Francisci D, Brisson L, Collard M. A scalar evolutionnary approach to rule extraction. Rapport de Recherche; 2003.
-
12.
Mao R, Yin Y, Pei P. Data Mining and Knowledge Discovery. Kluwer Academic Publishers; 2004.
-
13.
Fayyad U, Piatetsky SG, Smyth P. From data mining to knowledge discovery in databases. AI magazine. 1996;17(3):37.
-
14.
Ordonez C, Omiecinski E, de Braal L, Santana CA, Ezquerra N, Taboada JA, editors. Mining constrained association rules to predict heart disease. 2013 IEEE 13th International Conference on Data Mining. 2001. IEEE Computer Society; 433 p.
-
15.
Agrawal R, Imielinski T, Swami A, editors. Mining association rules between sets of items in large databases. ACM SIGMOD Record. 1993. Association for Computing Machinery; p. 207-16.
-
16.
Agrawal R, Srikant R, editors. Fast algorithms for mining association rules. Proc. 20th int. conf. very large data bases, VLDB. 1994. p. 487-99.
-
17.
Ramezankhani A, Pournik O, Shahrabi J, Khalili D, Azizi F, Hadaegh F. Applying decision tree for identification of a low risk population for type 2 diabetes. Tehran Lipid and Glucose Study. Diabetes Res Clin Pract. 2014;105(3):391-8. [PubMed ID: 25085758]. https://doi.org/10.1016/j.diabres.2014.07.003.
-
18.
Cao H, Markatou M, Melton GB, Chiang MF, Hripcsak G, editors. Mining a clinical data warehouse to discover disease-finding associations using co-occurrence statistics. AMIA Annual Symposium Proceedings. 2005. American Medical Informatics Association; 106 p.
-
19.
Wang C, Guo XJ, Xu JF, Wu C, Sun YL, Ye XF, et al. Exploration of the association rules mining technique for the signal detection of adverse drug events in spontaneous reporting systems. PLoS One. 2012;7(7). ee40561. [PubMed ID: 22815764]. https://doi.org/10.1371/journal.pone.0040561.
-
20.
Mullins IM, Siadaty MS, Lyman J, Scully K, Garrett CT, Miller WG, et al. Data mining and clinical data repositories: Insights from a 667,000 patient data set. Comput Biol Med. 2006;36(12):1351-77. [PubMed ID: 16375883]. https://doi.org/10.1016/j.compbiomed.2005.08.003.
-
21.
Nahar J, Imam T, Tickle KS, Chen YPP. Association rule mining to detect factors which contribute to heart disease in males and females. Expert Syst Appl. 2013;40(4):1086-93. https://doi.org/10.1016/j.eswa.2012.08.028.
-
22.
Simon GJ, Schrom J, Castro MR, Li PW, Caraballo PJ. Survival association rule mining towards type 2 diabetes risk assessment. AMIA Annu Symp Proc. 2013;2013:1293-302. [PubMed ID: 24551408].
-
23.
Kim HS, Shin AM, Kim MK, Kim YN. Comorbidity study on type 2 diabetes mellitus using data mining. Korean J Intern Med. 2012;27(2):197-202. [PubMed ID: 22707892]. https://doi.org/10.3904/kjim.2012.27.2.197.
-
24.
Azizi F, Ghanbarian A, Momenan AA, Hadaegh F, Mirmiran P, Hedayati M, et al. Prevention of non-communicable disease in a population in nutrition transition: Tehran Lipid and Glucose Study phase II. Trials. 2009;10:5. [PubMed ID: 19166627]. https://doi.org/10.1186/1745-6215-10-5.
-
25.
Report of the Expert Committee on the Diagnosis and Classification of Diabetes Mellitus. Diabetes Care. 1997;20(7):1183-97. [PubMed ID: 9203460].
-
26.
Azizi F, Rahmani M, Emami H, Mirmiran P, Hajipour R, Madjid M, et al. Cardiovascular risk factors in an Iranian urban population: Tehran lipid and glucose study (phase 1). Soz Praventivmed. 2002;47(6):408-26. [PubMed ID: 12643001].
-
27.
Harati H, Hadaegh F, Saadat N, Azizi F. Population-based incidence of Type 2 diabetes and its associated risk factors: results from a six-year cohort study in Iran. BMC Public Health. 2009;9:186. [PubMed ID: 19531260]. https://doi.org/10.1186/1471-2458-9-186.
-
28.
Hadaegh F, Bozorgmanesh MR, Ghasemi A, Harati H, Saadat N, Azizi F. High prevalence of undiagnosed diabetes and abnormal glucose tolerance in the Iranian urban population: Tehran Lipid and Glucose Study. BMC Public Health. 2008;8:176. [PubMed ID: 18501007]. https://doi.org/10.1186/1471-2458-8-176.
-
29.
National Kidney F. K/DOQI clinical practice guidelines for chronic kidney disease: evaluation, classification, and stratification. Am J Kidney Dis. 2002;39(2 Suppl 1):S1-266. [PubMed ID: 11904577].
-
30.
Hadaegh F, Hatami M, Tohidi M, Sarbakhsh P, Saadat N, Azizi F. Lipid ratios and appropriate cut off values for prediction of diabetes: a cohort of Iranian men and women. Lipids Health Dis. 2010;9:85. [PubMed ID: 20712907]. https://doi.org/10.1186/1476-511X-9-85.
-
31.
Azizi F, Khalili D, Aghajani H, Esteghamati A, Hosseinpanah F, Delavari A, et al. Appropriate waist circumference cut-off points among Iranian adults: the first report of the Iranian National Committee of Obesity. Arch Iran Med. 2010;13(3):243-4. [PubMed ID: 20433230].
-
32.
Global database on Body Mass Index: BMI Classification. WHO; 2006.
-
33.
Han J, Kamber. M, Pei J. Data mining: concepts and techniques. Morgan kaufmann; 2006.
-
34.
Ordonez C, Ezquerra N, Santana CA. Constraining and summarizing association rules in medical data. Knowl Inf Syst. 2006;9(3):1-2. https://doi.org/10.1007/s10115-005-0226-5.
-
35.
Schrom JR, Caraballo PJ, Castro MR, Simon GJ. Quantifying the effect of statin use in pre-diabetic phenotypes discovered through association rule mining. AMIA Annu Symp Proc. 2013;2013:1249-57. [PubMed ID: 24551405].
-
36.
Kwasnicka H, Switalski K. Discovery of association rules from medical data-classical and evolutionary approaches. Annales UMCS Sectio AI Informatica. 2006;4(1):204-17.
-
37.
Hahsler M, Grun B, Hornik K. Introduction to arules–mining association rules and frequent item sets. SIGKDD Explor. 2007.
-
38.
Ordonez C, Zhao K. Evaluating association rules and decision trees to predict multiple target attributes. Intell Data Anal. 2011;15(2):173-92.
-
39.
Sheikh LM, Tanveer B, Hamdani M, editors. Interesting measures for mining association rules. Multitopic Conference, 2004. Proceedings of INMIC 2004. 8th International. 2004. IEEE; p. 641-4.
-
40.
Jahangiri Noudeh Y, Hadaegh F, Vatankhah N, Momenan AA, Saadat N, Khalili D, et al. Wrist circumference as a novel predictor of diabetes and prediabetes: results of cross-sectional and 8.8-year follow-up studies. J Clin Endocrinol Metab. 2013;98(2):777-84. [PubMed ID: 23341488]. https://doi.org/10.1210/jc.2012-2416.
-
41.
Haffner SM. Abdominal obesity, insulin resistance, and cardiovascular risk in pre-diabetes and type 2 diabetes. Eur Heart J Suppl. 2006;8(suppl B):B20-5.
-
42.
Cheng CW, Chanani N, Venugopalan J, Maher K, Wang MD. icuARM-An ICU Clinical Decision Support System Using Association Rule Mining. Trans Eng Health Med. 2013;1:4400110.
-
43.
Muhlenbach F, Rakotomalala R. Encyclopedia of Data Warehousing and Mining. In: Discretization of continuous attributes, editor. . 1. 2005. p. 397-402.
-
44.
Palizgir M, Bakhtiari M, Esteghamati A. Association of depression and anxiety with diabetes mellitus type 2 concerning some sociological factors. Iran Red Crescent Med J. 2013;15(8):644-8. [PubMed ID: 24578829]. https://doi.org/10.5812/ircmj.12107.
-
45.
Ghasemi A, Zahediasl S. Potential therapeutic effects of nitrate/nitrite and type 2 diabetes mellitus. Int J Endocrinol Metab. 2013;11(2):63-4. [PubMed ID: 23825974]. https://doi.org/10.5812/ijem.9103.