Abstract
Background:
For addressing the burden of non-communicable diseases and policymaking, the world health organization uses World Bank income group to classify countries. This calcification method might not be optimal. This study aimed to investigate the role of World Bank income group, health expenditure, and cardiometabolic risk factors of countries in explaining the gap between their cardiometabolic mortality.Methods:
In total, 190 countries were categorized into four income groups according to the World Bank definition. The energy consumption, health expenditure, and data of sex-specified age-standardized prevalence of obesity, hypercholesterolemia, hypertension, diabetes, smoking, and physical inactivity in 2008 and cardiometabolic mortality in 2012 were used. Multivariable-adjusted mixed-effect linear regression models were applied to relate country-level predictors to their mortality outcomes.Results:
While the lowest cardiometabolic mortality was recorded in high-income countries in both genders, the highest rates were recorded in the low-income category for women and in low and middle-income for men. Countries had lower cardiometabolic mortality for women compared to men; however, such a difference was not shown in low-income countries. World Bank income group of countries, per se, explained one-third of the variation in their mortality outcomes while adding health expenditure, energy consumption, and cardiometabolic risk factors increased the explanatory power of the model considerably. Moreover, the more the health expenditure, the weaker the association of prevalence of hypertension with cardiometabolic mortality.Conclusions:
Adding countries’ health expenditure and/or the prevalence of risk factors to their World Bank income group may contribute to the better explanation of the gap between them in cardiometabolic mortality.Keywords
Cardiometabolic Mortality Cardiovascular Risk Factors Income Health Expenditure Ecological Study
1. Background
Non-communicable diseases are the main cause of death worldwide, the majority of which is due to cardiometabolic mortalities including deaths related to coronary heart disease, stroke, and diabetes (1-4). Meanwhile, it is estimated that there will be a dramatic upward trend in cardiometabolic mortality, which occurs mostly in low and middle-income countries (2, 4-6). Meanwhile, developed countries have enjoyed substantial falls in cardiometabolic mortality (2, 4-6). Reasonably, for addressing the burden of non-communicable diseases and policymaking, the world health organization (WHO) uses different definitions to classify countries mostly based on their income and level of development (7, 8). The most well-known and widely used categorization is based on the World Bank income group (7, 8). However, it has remained unclear whether the gap in cardiometabolic mortality between countries with different incomes is mostly explained by their differences in their World Bank income group status or it can be explained better by other determinants such as their total health-expenditure-per-capita or prevalence of cardiometabolic risk factors. A country-level analysis can contribute a great deal to public health policymakers to interpret the current health status among countries.
2. Objectives
The present study investigated to what extent the variance in cardiometabolic mortality of member states of the WHO in 2012 could be explained by their World Bank income category in 2008. We also aimed to determine the contribution of health-expenditure and cardiometabolic risk factors to optimizing the explanation of the current gap.
3. Methods
Out of 193 member states of the WHO in 2008, 190 were included in the current study. There were no available data for Monaco, San Marino, and Niue. The selected countries were categorized in one of the following income groups according to their gross national-income-per-capita in 2008, using the World Bank Atlas method (7, 8):
1- Low-income countries: (975 United States (US) dollars or less)
2- Lower-middle-income countries: (976 to 3855 US dollars)
3- Upper-middle-income countries: (3856 to 11905 US dollars)
4- High-income countries: (11906 US dollars or more)
Total health-expenditure-per-capita for each country in 2008 was also derived from the World Bank database (9). In the current study, the term “health-expenditure” refers to “the total expenditure on health per-capita converted using purchasing-power-parity”. Moreover, the energy-consumption-per-capita for the period of 2006 - 2008 was extracted from each country from reports of the food and agriculture organization of the United Nations (10).
Data of sex-specified and age-standardized prevalence of conventional cardiometabolic risk factors (i.e. obesity, hypercholesterolemia, hypertension, diabetes, smoking, and physical inactivity) in 2008 and sex-specific age-standardized mortality in 2012 for cardiovascular disease and diabetes for each country were extracted using global health observatory data repository (11). All data represent the vital statistics of adult populations as reported from the related ministries of each individual country using a standard format. To remove the effects of the population size and age structure, age-standardized mortality and prevalence data were used, enabling us to compare different countries with each other. Detailed definition of the above-mentioned variables is provided in Table 1. The design of the study was approved by our institution’s review board. The study followed the RECORD statement (12) and designed for observational studies using routinely collected health data. Moreover, the work was approved by the ethics committee of the research institute for endocrine Sciences.
Detailed Definition of Variables Used
Variable | Definitiona |
---|---|
Hypertension | Systolic blood pressure ≥ 140 mmHg or diastolic blood pressure ≥ 90 mmHg or consumption of medication for raised blood pressure |
Diabetes | Fasting plasma glucose ≥ 126 mg/dL (7.0 mmol/L) or consumption of medication for raised blood glucose |
Hypercholesterolemia | Total cholesterol ≥ 240 mg/dL (6.2 mmol/L) |
Obesity | Body mass index (BMI) ≥ 30 kg/m2 |
Smoking | Consuming any form of tobacco, including cigarettes, cigars, pipes, etc. (excluding smokeless tobacco) at the time of the survey, including daily and non-daily smoking |
Physical inactivity | Not being engaged in at least 150 minutes of moderate intensity physical activity per week or equivalent |
3.1. Statistical Analysis
Associations of World Bank income group, health-expenditure, and prevalence of conventional cardiometabolic risk factors of countries with their cardiometabolic mortality were analyzed using Spearman or Pearson correlation in each gender after checking the normality assumption and then presented in scatter plots. Moreover, the mean (95% confidence interval (CI)) of the aforementioned variables was reported in each income group.
Furthermore, multivariable-adjusted regression models were applied to relate country level pre-specified predictors to cardiometabolic mortality. The prevalence of conventional cardiometabolic risk factors and cardiometabolic mortality was recorded for each gender in each country separately (2 × 172 records).
Multivariable-adjusted mixed-effect linear regression models were used to assess the relationship of gender, World Bank income group, health-expenditure, and prevalence of conventional cardiometabolic risk factors with cardiometabolic mortality of countries in different multivariable models. Random-effect was specified for countries to determine the correlation between the two repeated measurements on the same country for each gender. Prior to building multivariable regression models, linear and quadratic models were checked visually via likelihood ratio test and with fractional-polynomial analysis. Independent covariates were entered into the multivariable models in a hierarchical manner as follows: model 1: Gender and World Bank income group; model 2: model 1 plus health-expenditure and; model 3: model 2 plus energy-consumption and prevalence of conventional cardiometabolic risk factors. All possible first-order interactions between independent variables (except interactions between cardiometabolic risk factors themselves) were checked in multivariable analysis at each hierarchical level and all interactions with P values < 0.05 in univariate models were included in the final analyses. To deal with missing values, all independent variables in different countries were imputed by recoding them into their mean value in their income group and the main analyses were conducted using imputed centered independent variables in those countries with available data of outcome (172 countries). As sensitivity analyses, the abovementioned hierarchy models were repeated in only those countries with all available data (complete case analyses (106 pairs)) and multiple imputed data for both independent and outcome variables (193 pairs). The analyses were performed using STATA ver. 14 (the USA, 2016).
4. Results
Of 190 countries, 42 were categorized in the low-income group, 54 in the lower-middle-income group, 46 in the upper-middle-income group, and 48 in the high-income group. Figure 1 shows the correlations of health-expenditure, energy-consumption, and prevalence of cardiometabolic risk factors in countries with their cardiometabolic mortality in each gender. According to Pearson/Spearman tests, in both genders, there were positive associations between hypertension and diabetes prevalence and cardiometabolic mortality among countries; however, the associations were negative for hypercholesterolemia, obesity, physical inactivity, health-expenditure, and energy consumption. Interestingly, smoking was in men positively related and in women negatively related to cardiometabolic mortality.
Correlation of health expenditure, energy consumption, and age-standardized prevalence of cardio-metabolic risk factors in countries in 2008 with their cardio-metabolic mortality rates in 2012 in each gender. Observed mortality rates in men, for each country, is illustrated with black-filled dots and solid line (dark grey shadow) representing the fitted line (95% confidence interval). Observed mortality rates in women, for each country, is illustrated with grey-filled dots and dashed line (light grey shadow) representing the fitted line (95% confidence interval). Health expenditure was defined as the sum of public and private health expenditures as a ratio of total population in 2008 (data are in current U.S. dollars converted using purchasing power parity rates. Energy consumption refers to the amount of food available for human consumption per capita for 2006 - 2008 period (data are in calories). Cardio-metabolic mortality was defined as number of death due to cardiovascular diseases or diabetes in agestandardized population in 100,000 in 2012.
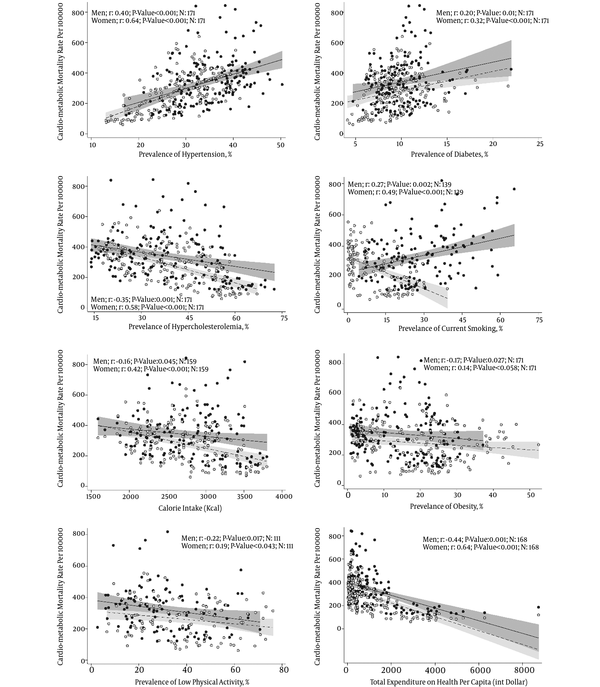
Table 2 shows the mean (95% CI) of health-expenditure, energy-consumption, the prevalence of cardiometabolic risk factors, and cardiometabolic mortality in each income group. Cardiometabolic mortality had a declining trend from 350.2 per 100000 in the low-income group to 153.9 per 100000 in the high-income group in women; while in men, the highest and the lowest cardiometabolic mortality was recorded in the lower-middle-income and high-income categories, respectively.
Mean (95% Confidence Interval) of Health Expenditure, Energy Consumption, and Sex-Specific Age-Standardized Prevalence of Cardiometabolic Risk Factors in 2008 and Cardiometabolic Mortality in 2012 in Each Income Groupa
Variables | Low-Income | Lower-Middle Income | Upper-Middle Income | High-Income |
---|---|---|---|---|
Health expenditureb | 92 (76 - 108) | 323 (269 - 376) | 826 (729 - 923) | 2859 (2425 - 3294) |
Energy consumptionc (calories) | 2274 (2129 - 2420) | 2598 (2468 - 2729) | 2921 (2772 - 3070) | 3337 (3236 - 3439) |
Cardiometabolic risk factors | ||||
Men, % | ||||
Hypertension | 35.4 (32.8 - 38.1) | 35.1 (31.9 - 38.3) | 38.0 (35.9 - 40.1) | 31.5 (28.8 - 34.2) |
Diabetes | 8.1 (7.6 - 8.7) | 11.1 (9.3 - 12.8) | 10.9 (10.2 - 11.7) | 10.4 (9.3 - 11.5) |
Hypercholesterolemia | 22.9 (19.7 - 26.0) | 32.0 (28.0 - 36.0) | 42.1 (38.6 - 45.6) | 57.6 (55.0 - 60.2) |
Obesity | 2.4 (2.0 - 2.9) | 11.3 (6.8 - 15.9) | 17.6 (14.6 - 20.5) | 23.2 (21.2 - 25.2) |
Smoking | 22.7 (17.6 - 27.9) | 30.7 (22.4 - 39.1) | 29.3 (23.8 - 34.7) | 26.8 (23.1 - 30.4) |
Physical inactivity | 15.4 (11.9 - 18.9) | 26.8 (22.5 - 31.1) | 38.8 (33.1 - 44.4) | 38.8 (33.8 - 43.9) |
Women, % | ||||
Hypertension | 32.4 (29.8 - 34.9) | 29.2 (26.6 - 31.8) | 31.3 (29.3 - 33.3) | 22.2 (19.9 - 24.5) |
Diabetes | 8.4 (7.8 - 9.1) | 11.5 (9.6 - 13.4) | 11.0 (10.0 - 12.1) | 8.3 (6.9 - 9.6) |
Hypercholesterolemia | 24.4 (22.2 - 26.5) | 36.3 (32.1 - 40.5) | 44.6 (41.9 - 47.2) | 55.4 (53.6 - 57.3) |
Obesity | 7.1 (4.8 - 9.4) | 20.6 (14.1 - 27.0) | 28.8 (25.5 - 32.2) | 23.7 (20.2 - 27.2) |
Smoking | 3.3 (1.4 - 5.3) | 6.9 (1.8 - 12.1) | 11.4 (7.5 - 15.2) | 16.9 (14.0 - 19.8) |
Physical inactivity | 21.1 (16.2 - 26.0) | 35.6 (29.8 - 41.4) | 47.1 (41.3 - 52.9) | 43.2 (36.9 - 49.6) |
Cardiometabolic mortalityd | ||||
Men | 340.2 (327.4 - 393.0) | 409.4 (362.1 - 456.6) | 374.3 (318.4 - 430.3) | 221.6 (190.1 - 253.1) |
Women | 350.2 (317.9 - 382.5) | 336.7 (304.8 - 368.6) | 271.9 (237.6 - 306.2) | 153.9 (129.0 - 178.8) |
Table 3 shows the association of gender, the World Bank income group, health-expenditure, energy-consumption, and age-standardized prevalence of cardiometabolic risk factors with age-standardized cardiometabolic mortality. According to model 1, low or middle-income countries had higher mortality risk, compared to countries with high income. The biggest difference in cardiometabolic mortality was detected between lower-middle-income and high-income countries in men and low-income and high-income in women. Women had less cardiometabolic mortality compared to men in country level in all income groups although there was not enough evidence against null hypothesis in the low-income group (women in the low-income group had 9.97 less cardiometabolic mortality compared to men in low and middle-income countries; 95% CI: -34.14; 14.19). Moreover, according to the overall R2 of model 1, one-third of the variance of cardiometabolic mortality in each gender among countries could be explained only by their income categories. Regarding model 2, adding health-expenditure to the model caused beta-coefficients of middle-income categories to decrease dramatically. Health-expenditure, itself, showed a strong negative association with cardiometabolic rate (a 3.8-per-100000 decrease for each 100 dollars increase in health-expenditure-per-capita (95% CI: -5.8; -2.0). In model 3, after more adjustment with the prevalence of cardiometabolic risk factors, the difference between high- and upper-middle-income and low-income countries in men and the difference between high- and upper-middle-income in women did not show enough evidence against the null hypothesis that there is no association between the mentioned income-group and cardiometabolic mortality. The association of health-expenditure with cardiometabolic mortality remained almost unchanged. Among cardiometabolic risk factors, prevalence rates of smoking, diabetes, and hypertension were associated with higher cardiometabolic mortality in men whereas, in women, the risk of the prevalence of diabetes and smoking was lower. Energy consumption also had a positive association with cardiometabolic mortality in both genders. Last but not least, there was an effect modification between hypertension and health-expenditure for the outcome of cardiometabolic mortality in a way that by increasing health-expenditure, the risk of the prevalence of hypertension for cardiometabolic mortality decreased (β: -0.001, 95% CI: -0.003; -0.00005). According to R2 of model 3, more than half of variance of cardiometabolic mortality of countries could be explained by their health-expenditure, income-group, and prevalence of conventional cardiometabolic risk factors.
The Association of Gender, the World Bank Income Groups, Health Expenditure, Energy Consumption, and Age-Standardized Prevalence of Cardiometabolic Risk Factors in 2008 with Age-standardized Cardiometabolic Mortality in 2012 Driven from Multivariable-Adjusted Mixed-Effect Linear Regression
Variables | Model 1 | Model 2 | Model 3 | |||
---|---|---|---|---|---|---|
Coefficient | 95% CI | Coefficient | 95% CI | Coefficient | 95% CI | |
Overall R2 | 0.33 | 0.37 | 0.54 | |||
Gender | ||||||
Men | Reference | Reference | Reference | |||
Women | -67.75 | -91.92; -43.57 | -67.75 | -91.92; -43.57 | -10.33 | -50.59; 29.92 |
World Bank income groupa | ||||||
High-income | Reference | Reference | Reference | |||
Upper-middle-income | 152.70 | 100.58; 204.82 | 76.49 | 11.81; 141.17 | 44.04 | -17.08; 105.16 |
Lower-middle-income | 187.74 | 138.50; 236.99 | 91.16 | 21.15; 161.16 | 97.42 | 23.74; 171.10 |
Low-income | 138.57 | 88.77; 188.36 | 32.63 | -41.43; 106.69 | 81.11 | -12.57; 174.80 |
Health expenditureb, $ | -0.038 | -0.058; -0.02 | -0.04 | -0.07; -0.02 | ||
Prevalence of cardiometabolic risk factors, % | ||||||
Smoking | 2.59 | 1.68; 3.51 | ||||
Obesity | -0.87 | -2.65; 0.91 | ||||
Hypercholesterolemia | 0.47 | -1.50; 2.44 | ||||
Diabetes | 15.09 | 8.09; 22.10 | ||||
Hypertension | 6.69 | 4.44; 8.95 | ||||
Physical inactivity (%) | -0.59 | -1.95; 0.78 | ||||
Energy consumptionc | 0.07 | 0.02; 0.12 | ||||
Interactions | ||||||
Gender- high-income | Reference | Reference | Reference | |||
Gender- upper-middle-income | -34.70 | -70.69; 1.29 | -34.70 | -70.69; 1.29 | -10.23 | -49.76; 29.29 |
Gender- lower-middle-income | -4.90 | -38.90; 29.10 | -4.90 | -38.90; 29.10 | 13.94 | -28.65; 56.53 |
Gender- low-income | 57.78 | 23.39; 92.16 | 57.78 | 23.39; 92.16 | 52.08 | 0.71; 103.46 |
Gender- hypertension | -0.80 | -2.72; 1.13 | ||||
Gender- diabetes | -7.77 | -12.71; -2.84 | ||||
Gender- physical inactivity | 1.27 | 0.19; 2.35 | ||||
Gender- smoking | -2.19 | -3.81; -0.56 | ||||
Health expenditure- hypertension | -0.001 | -0.003; -0.00005 |
5. Discussion
In this worldwide ecological study, we assessed the association of countries’ World Bank income group, health-expenditure, and prevalence of conventional cardiometabolic risk factors with their cardiometabolic mortality. While the lowest cardiometabolic mortality was recorded in high-income countries in both genders, the highest rates were recorded in the low-income category for women and in lower-middle income ones for men. Countries had lower cardiometabolic mortality for women compared to men; however, such a difference was not shown in low-income countries. Moreover, income category of countries played a significant role in explaining their cardiometabolic mortality only in lower-middle-income countries for men and low-income and lower-middle-income countries for women. Health-expenditure had a protective association with cardiometabolic mortality in different countries. Furthermore, the prevalence of hypertension, diabetes, and smoking, as well as energy-consumption among countries, was associated with higher cardiometabolic mortality in men whereas, in women, the risk of the prevalence of diabetes and smoking was lower. Last but not least, according to the models, the more the health-expenditure, the weaker the association between the prevalence of hypertension and cardiometabolic mortality.
We showed that in both genders, low- and middle-income countries had higher cardiometabolic mortality compared to high-income countries and one-third of the gap between countries can be explained by their income-group. Therefore, one can interpret that World Bank categories can be relatively a useful tool for explaining the gap between countries in their cardiometabolic mortality. However, the strong association between income-groups and cardiac mortality reduced noticeably when we adjusted our model for health-expenditure. This may be explained by the fact that wealthier countries are more likely to expend more on their health section, effecting more-expensive medications, interventions, and surgeries (13). Furthermore, wealthier countries spend more on health education and health policies, which have been shown to have significant effects on health outcomes (14-16).
After controlling for countries’ health-expenditure, women in low- and middle-income countries and men in middle-income countries had higher cardiometabolic mortality compared to high-income countries. This finding indicated that although funding of health-care systems is quite important, by no means is the entire problem. In other words, even if the countries spend equally on health, low and middle-income countries would have higher cardiometabolic mortality in comparison with their high-income counterparts. Similarly, in a systematic review of 30 population-based studies, Luciano et al. found that lower gross domestic product per capita adjusted for purchasing-power-parity and lower total health-expenditures-per-capita at purchasing-power-parity were associated with higher incidence risk of stroke, higher case-fatality, and lower age at stroke onset (17). Gwatkin et al. (18) made the point that the health of the poor populations has more to do with their social and environmental circumstances than with their lack of healthcare.
In line with several studies, we found that except for hypertension, the prevalence of risk factors was the lowest in low-income countries. This may be because an increase in wealth influences the population’s lifestyle, leading to increased consumption of tobacco, fat, prepared foods, and decreased physical activity (13). However, the higher prevalence of cardiometabolic risk factors in the current study was not coupled with higher cardiometabolic mortality in high- or upper-middle-income countries, whereas the opposite correlation was expected (13). Such a discrepancy can be explained by the important role of income groups and health-expenditure as important confounders. Not surprisingly, after adjustment for gender, income groups, and health-expenditure, we found positive associations between the prevalence of smoking, diabetes, and hypertension as well as energy consumption and cardiometabolic mortality. The prominent role of risk factors such as smoking, diabetes, hypertension, and overeating in cardiometabolic mortality has been reported previously at global scales (19). However, it should be noted that our results are in the level of countries. After adjustment for cardiometabolic risk factors, we observed a noticeable rise in the proportion of variability in cardiometabolic mortality that could be explained by our model from 37% to 54%. We also found that the more nations spend on the health, the weaker the association between the prevalence of hypertension and cardiometabolic mortality, which may highlight the importance of secondary prevention of cardiometabolic risk factors such as hypertension in controlling the burden of cardiometabolic mortality by reducing their case-fatality. Likewise, the result of IMPACT, a study conducted to explore the contributions of risk factors and treatments in over 15 countries where mortality rates have been declining, showed that in different populations, about 40% to 75% of the coronary heart disease mortality decline could be attributed to risk factor changes and the remaining 25% to 55% to treatments (20). Similarly, in the MONICA analysis, approximately three-quarters of the fall observed could be attributed to the decline in event rates (mainly reflecting risk factor improvements) while approximately one-quarter could be attributed to a decrease in case of death (mainly reflecting medical treatments) (21).
According to our models, generally, women had lower cardiometabolic mortality compared to men. However, the prominent difference was disappeared in low-income countries. Moreover, according to the data, 33 countries had higher mortality rates for their women than for men, mostly in low- (18 countries) and lower-middle- (8 countries) income groups (only Qatar and Kuwait from the high-income group). Higher cardiovascular mortality rates for women, which cannot be explained by conventional risk factors, were also reported in previous studies in low-income countries (22). This finding might be explained by a widespread neglect of health, nutrition, and other interests of women that could affect their survival (22-24).
5.1. Strength and Limitations of This Study
Several limitations should be considered when assessing the results of the current study. First, every ecological study is apt to suffer from inherent biases. However, our study aimed to investigate the relationships and associations between countries rather than individuals; therefore, some limitations of ecological studies such as ecological fallacy do not count for the current study. Second, regarding cardiometabolic mortality among countries, there might be differences in reporting patterns between countries as they were collected in distinct settings, despite the existence of the statistical classification of diseases and related health problems (ICD), which try to standardize the cause of death coding. To minimize such biases, we used cardiometabolic mortality as the sum of death rates related to cardiovascular disease and diabetes instead of using only one of them. Third, the reliability and validity of the prevalence of risk factors may vary from a country to another. Fourth, the relatively low number of subjects in our models (172 countries) might result in low statistical power. By using data for both genders in each country and running mixed-effect analyses, we included 172 × 2 records in the analysis and improved the power. Some might see the gross national income (GNI) per capita at purchasing power parity (PPP) a better indicator of the economic status of a country; however, it should be considered that GNI per capita at PPP is highly skewed and cannot be used in models without advanced transformations. Interpretation of transformed GNI per capita at PPP is very hard if not impossible for readers. Moreover, to the best of our knowledge, the WHO has not yet used GNI per capita at PPP for classification of countries. The main strengths of our study are using age-standardized mortality rates and prevalence of cardiometabolic risk factors in each gender, which allowed us to compare countries, regardless of their age structure and population size. We assessed the association of independent variables of income, health-expenditure, and risk factors of countries derived in 2008 with their cardiometabolic mortality of 2012, which minimized biases related to cross-sectional analyses.
5.2. Conclusion
In this study, the World Bank income group, health-expenditure, and prevalence of cardiometabolic risk factors all had remarkable power to explain the gap in cardiometabolic mortality between countries. Therefore, classification of countries by adding their health-expenditure and/or prevalence of cardiometabolic risk factors to their income might lead to better classification. In addition, as a post hoc finding, we detected a higher cardiovascular mortality for women in low-income countries, which cannot be explained by income groups, health-expenditure, and prevalence of cardiometabolic risk factors. Further research is needed to investigate the potential explanations for such a finding.
Acknowledgements
References
-
1.
Alwan A. Global status report on noncommunicable disaeses 2010: World Health Organization. 2011. 3 p.
-
2.
Whiting DR, Guariguata L, Weil C, Shaw J. IDF diabetes atlas: global estimates of the prevalence of diabetes for 2011 and 2030. Diabetes Res Clin Pract. 2011;94(3):311-21. [PubMed ID: 22079683]. https://doi.org/10.1016/j.diabres.2011.10.029.
-
3.
Murray CJ, Vos T, Lozano R, Naghavi M, Flaxman AD, Michaud C, et al. Disability-adjusted life years (DALYs) for 291 diseases and injuries in 21 regions, 1990-2010: a systematic analysis for the Global Burden of Disease Study 2010. Lancet. 2012;380(9859):2197-223. [PubMed ID: 23245608]. https://doi.org/10.1016/S0140-6736(12)61689-4.
-
4.
IDF Diabetes Atlas. Brussels, Belgium: International Diabetes Federation; 2014. Available from: http://www.diabetesatlas.org.
-
5.
Fact sheet cardiovascular disease:. World Health Organization; 2013. Available from: http://www.who.int/countries/en/.
-
6.
Mathers CD, Loncar D. Projections of global mortality and burden of disease from 2002 to 2030. PLoS Med. 2006;3(11). e442. [PubMed ID: 17132052]. [PubMed Central ID: PMC1664601]. https://doi.org/10.1371/journal.pmed.0030442.
-
7.
Ramachandran A, Snehalatha C, Yamuna A, Mary S, Ping Z. Cost-effectiveness of the interventions in the primary prevention of diabetes among Asian Indians: within-trial results of the Indian Diabetes Prevention Programme (IDPP). Diabetes Care. 2007;30(10):2548-52. [PubMed ID: 17670917]. https://doi.org/10.2337/dc07-0150.
-
8.
Diabetes Prevention Program Research G. Within-trial cost-effectiveness of lifestyle intervention or metformin for the primary prevention of type 2 diabetes. Diabetes Care. 2003;26(9):2518-23. [PubMed ID: 12941712]. [PubMed Central ID: PMC1360736]. https://doi.org/10.2337/diacare.26.9.2518.
-
9.
Bandura A. Health promotion by social cognitive means. Health Educ Behav. 2004;31(2):143-64. [PubMed ID: 15090118]. https://doi.org/10.1177/1090198104263660.
-
10.
Carver CS, Scheier MF. Self-regulation of action and affect. Handbook of self-regulation: Research, theory, and applications. 2004. p. 13-39.
-
11.
Herman WH, Hoerger TJ, Brandle M, Hicks K, Sorensen S, Zhang P, et al. The cost-effectiveness of lifestyle modification or metformin in preventing type 2 diabetes in adults with impaired glucose tolerance. Ann Intern Med. 2005;142(5):323-32. [PubMed ID: 15738451]. [PubMed Central ID: PMC2701392]. https://doi.org/10.7326/0003-4819-142-5-200503010-00007.
-
12.
Benchimol EI, Smeeth L, Guttmann A, Harron K, Moher D, Petersen I, et al. The REporting of studies Conducted using Observational Routinely-collected health Data (RECORD) statement. PLoS Med. 2015;12(10). e1001885. [PubMed ID: 26440803]. [PubMed Central ID: PMC4595218]. https://doi.org/10.1371/journal.pmed.1001885.
-
13.
Singh R. The Adverse Effects of Wealth on Cardiovascular Health: A Scientific Statement of the International College of Cardiology. Cardiol Angiol. 2013;1(1):9-22. https://doi.org/10.9734/ca/2013/3375.
-
14.
Sidney S, Rosamond WD, Howard VJ, Luepker RV, National Forum for Heart D, Stroke P. The "heart disease and stroke statistics--2013 update" and the need for a national cardiovascular surveillance system. Circulation. 2013;127(1):21-3. [PubMed ID: 23239838]. https://doi.org/10.1161/CIRCULATIONAHA.112.155911.
-
15.
Singh RB, Anjum B, Takahashi T, Martirosyan DM, Pella D, De Meester F. Poverty is not the absolute cause of deaths due to non-communicable diseases. World Heart J. 2012;4(2/3):221.
-
16.
Woolf SH, Aron L. US Health in International Perspective:: Shorter Lives, Poorer Health. National Academies Press; 2013.
-
17.
Sposato LA, Saposnik G. Gross domestic product and health expenditure associated with incidence, 30-day fatality, and age at stroke onset: a systematic review. Stroke. 2012;43(1):170-7. [PubMed ID: 22033985]. https://doi.org/10.1161/STROKEAHA.111.632158.
-
18.
Gwatkin DR, Wagstaff A, Yazbeck AS. Reaching the poor with health, nutrition, and population services: what works, what doesn't, and why. World Bank Publications; 2005. https://doi.org/10.1596/978-0-8213-5961-7.
-
19.
Yusuf S, Hawken S, Ounpuu S, Dans T, Avezum A, Lanas F, et al. Effect of potentially modifiable risk factors associated with myocardial infarction in 52 countries (the INTERHEART study): case-control study. Lancet. 2004;364(9438):937-52. [PubMed ID: 15364185]. https://doi.org/10.1016/S0140-6736(04)17018-9.
-
20.
Hunink MG, Goldman L, Tosteson AN, Mittleman MA, Goldman PA, Williams LW, et al. The recent decline in mortality from coronary heart disease, 1980-1990. The effect of secular trends in risk factors and treatment. JAMA. 1997;277(7):535-42. [PubMed ID: 9032159].
-
21.
Tunstall-Pedoe H, Kuulasmaa K, Mahonen M, Tolonen H, Ruokokoski E, Amouyel P. Contribution of trends in survival and coronary-event rates to changes in coronary heart disease mortality: 10-year results from 37 WHO MONICA project populations. Monitoring trends and determinants in cardiovascular disease. Lancet. 1999;353(9164):1547-57. [PubMed ID: 10334252]. https://doi.org/10.1016/S0140-6736(99)04021-0.
-
22.
Osmani S, Sen A. The hidden penalties of gender inequality: fetal origins of ill-health. Econ Hum Biol. 2003;1(1):105-21. [PubMed ID: 15463967]. https://doi.org/10.1016/S1570-677X(02)00006-0.
-
23.
Dollar D, Gatti R. Gender inequality, income, and growth: are good times good for women?: Development Research Group. Washington, DC: The World Bank; 1999.
-
24.
Sen A. The many faces of gender inequality. New republic; 2001. p. 35-9.