Abstract
Background:
Early prediction of disease progression in coronavirus disease 2019 (COVID-19) patients can be helpful for personalized therapy, as well as the optimal allocation of public health resources.Objectives:
This study aimed to present predictive models for identifying potential high-risk COVID-19 patients upon hospital admission, based on the examination of clinical and radiological features by radiologists and artificial intelligence (AI).Patients and Methods:
A total of 786 initially non-severe COVID-19 patients were retrospectively enrolled in this study between January 2 and May 28, 2020. The patients were randomly divided into training (n = 628, 80%) and test (n = 158, 20%) groups. Clinical factors, laboratory indicators, and radiologist- and AI-extracted radiological features of pneumonia lesions were determined using a convolution neural network. The features were selected based on the Boruta algorithm with five-fold cross-validation. Four models, including a model based on clinical findings (model C), a model based on the physician’s examination of radiological features (R-Doc model), a model based on AI-derived radiological features (R-AI model), and an AI-based model mimicking the physician’s examinations (AI-Mimic-Doc model), were constructed for predicting COVID-19 progression upon admission, using a logistic regression analysis. The predictive performance of the four models was evaluated by calculating the area under the receiver operating characteristic (AUC) curve with a 95% confidence interval (95% CI) and then compared using the DeLong test.Results:
Overall, 238 out of 786 patients (30.3%) progressed into severe or critical pneumonia during the 14-day follow-up. Nine clinical findings, 17 laboratory indicators, 48 physician-extracted radiological features of pneumonia lesions, and 126 AI-driven radiological features were collected. The urea, albumin level, and lesion size in the basal segment of the right lower lobe of the lung or the proportion of CT values in the range of -200 - 60 in the left lung were the representative features for constructing the R-Doc and R-AI models, respectively. Comparison of the R-Doc model (AUC: 0.840, 95% CI: 0.747 - 0.933 for the training set and 0.731, 95% CI: 0.606 - 0.857 for the test set) with the R-AI model (AUC: 0.803, 95% CI: 0.701 - 0.906 for the training set and AUC: 0.731, 95% CI: 0.606 - 0.857 for the validation set) indicated a marginal difference in identifying patients at risk of progression to pneumonia upon admission (P < 0.1). The R-AI model was superior to model C, with an AUC of 0.770 for the training set (95% CI: 0.657 - 0.882) and 0.666 for the validation set to identify high-risk non-severe cases upon admission.Conclusion:
By using radiological features along with blood tests, early identification of COVID-19 patients, who are at risk of disease progression, can be achieved on admission (rapidly by using AI); therefore, the use of these features can contribute to the clinical management of COVID-19.Keywords
COVID-19 SARS-CoV-2 Disease Progression Early Identification Risk Prediction
1. Background
The coronavirus disease 2019 (COVID-19) pandemic, caused by SARS-CoV-2 as a newly emergent coronavirus, was first reported in Wuhan, China, in December 2019. Until July 10, 2020, more than 250,000 people died because of COVID-19, and the total number of expired cases was over 10,000,000 globally. According to the clinical management guidelines for COVID-19 issued by the World Health Organization (WHO), COVID-19 pneumonia has a wide clinical spectrum, ranging from a mild or moderate infection to severe or critical forms (1).
Approximately 80% of COVID-19 patients have a non-severe disease form, including mild (40%) and moderate (40%) infections. On the other hand, almost 15% of these patients may progress into a severe disease form, requiring oxygen support. The remaining patients (5%) have a critical condition, as well as complications, such as respiratory failure, acute respiratory distress syndrome (ARDS), sepsis or septic shock, thromboembolism, and/or multiorgan failure (eg, acute renal and cardiac injuries) (1). Besides, some non-severe cases may develop severe pneumonia, and some may even progress into the critical form of the disease and experience complications, such as respiratory failure and septic shock. According to a recent report by the Chinese Center for Disease Control and Prevention, the overall case-fatality rate of COVID-19 was 2.3%, while the mortality rate was 49.0% in critical cases (2). Therefore, early differentiation of high-risk patients with a poor prognosis from non-severe cases of pneumonia is essential.
Computed tomography (CT) is an effective, non-invasive method for pneumonia diagnosis and identification (or exclusion) of pulmonary complications in clinical practice (3-5). Zhang et al. (5) reported that CT scan can be used by radiologists and physicians to rapidly diagnose COVID-19 and differentiate it from other common types of pneumonia. However, it is still unclear whether radiological features can be helpful in differentiating severe pneumonia from non-severe pneumonia.
With the advent of artificial intelligence (AI), AI-based systems have been widely used in medicine (6, 7). Previous studies have suggested the great potential of AI to improve the diagnosis of COVID-19 (8) and stratify disease severity (9, 10) with high efficiency. However, to the best of our knowledge, no research has yet investigated the early detection of COVID-19 patients, who are at risk of progression into a severe disease form upon admission, based on AI-based radiological features. Besides, potential risk factors, including age (11), D-dimer level (12), lactate dehydrogenase, consolidation volume (13), and direct bilirubin level (14), have been reported in recent research.
Unlike manual readings by radiologists, which are elaborate and time-consuming, AI can rapidly and automatically represent the radiological features within a few seconds, enabling clinicians to make a rapid prediction and save time. Therefore, it is important to determine whether clinical features, together with radiological features based on AI, can predict COVID-19 progression.
2. Objectives
An early identification of patients who are at risk of disease progression allows for a rapid and optimal initiation of individualized supportive treatments and appropriate allocation of public health resources. For a non-invasive and time-efficient prediction of severe or critical pneumonia and its differentiation from non-severe cases upon admission, this study aimed to explore whether the examination of radiological features in CT images (extracted by either radiologists or AI systems), together with clinical indicators, can increase the prediction performance.
3. Patients and Methods
3.1. Participants
This study was approved by the ethics committee (ethics code: V1.0), and the requirement to obtain a written informed consent form was waived. A total of 786 laboratory-confirmed patients with SARS-CoV-2, admitted to Renmin Hospital of Wuhan University in China, were enrolled in this study between January 2 and May 28, 2020. The diagnosis of COVID-19 was confirmed based on the positive results of reverse transcription-polymerase chain reaction (RT-PCR) assay of respiratory secretions, including bronchoalveolar lavage, tracheal aspirate, and nasopharyngeal or oropharyngeal swabs; the detailed RT-PCR procedures were based on a previous study (15).
Patients, who were diagnosed with COVID-19 and non-severe pneumonia via RT-PCR upon admission and had non-contrast chest CT scans three days after RT-PCR, were included in the study. The exclusion criteria were as follows: (1) age < 18 years; (2) pregnancy; (3) incomplete laboratory data; (4) follow-up < 14 days; 5) medication use for > 14 days before hospital admission; and (6) pulmonary thromboemboli. The clinical findings, chest scans, and laboratory data of 786 non-severe patients (out of 1,535 confirmed COVID-19 cases based on RT-PCR) were finally examined for data analysis.
The patients were divided into none-progressing and progressing groups according to the disease severity during 14 days of follow-up. The high-risk group included COVID-19 patients who progressed from non-severe to severe disease. On the other hand, the low-risk group included patients without disease progression. The patients were randomly divided into a training cohort (n = 628) and a test cohort (n = 158). The training cohort was used to determine the risk of disease progression via five-fold cross-validation. The test cohort was used to validate the risk of disease progression. The procedure of patient enrollment is presented in Figure 1.
The flowchart of patient enrollment
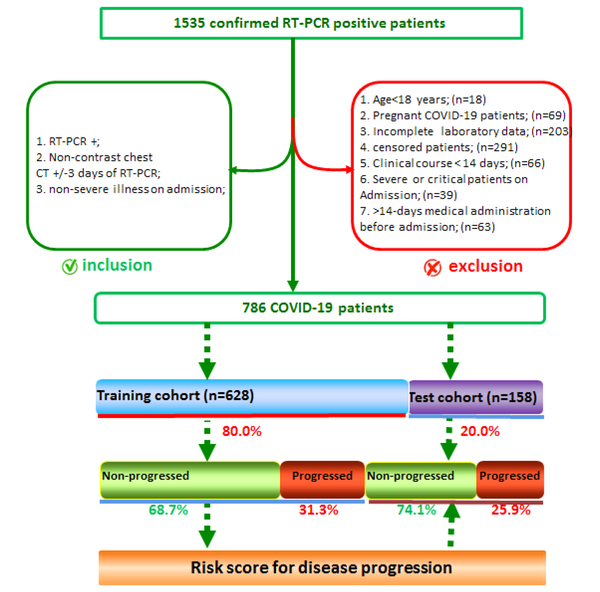
3.2. Definitions
The severity of COVID-19 was defined according to the clinical management of COVID-19 interim guidance, issued by the WHO on May 27, 2020 (1). A mild disease was defined as a symptomatic infection meeting the diagnostic criteria for COVID-19, without evidence of viral pneumonia or hypoxia. Patients with pneumonia signs (eg, fever, cough, dyspnea, and fast breathing), but no signs of severe pneumonia (SpO2 ≥ 90% on room air) were classified as moderate. Also, patients with pneumonia signs, together with a respiratory rate > 30 bpm, severe respiratory distress, or SpO2 < 90% on room air, were classified as severe pneumonia. Finally, critical pneumonia was characterized by one of the following symptoms: respiratory failure requiring mechanical ventilation, sepsis and septic shock, or other types of organ failure requiring intensive care unit (ICU) monitoring.
The disease severity was determined based on the clinical findings, along with chest imaging findings, including radiographs, CT scans, and ultrasounds. Moreover, in this study, COVID-19 cases that were considered moderate during the follow-up were defined as non-progressing, while moderate cases upon admission that progressed to severe or critical pneumonia during the follow-up were defined as progressing.
3.3. Data Collection
The electronic medical records of laboratory-confirmed COVID-19 cases were examined in this study. Clinical characteristics, including clinical signs, symptoms, underlying non-communicable diseases, and disease severity, were extracted by two experienced senior clinicians independently. Laboratory data, including the sample’s name, sampling time, quantitative factors, and reference medical ranges, were also collected. Besides, the CT scans of all patients were collected upon admission.
3.4. Physician-based and AI-based Quantization of CT Scans
The CT scans for the training set were reviewed by two radiologists in respiratory medicine (XF Lu and LH Hu with more than five years of experience) using a double-blind method; the results were combined by consensus. A senior radiologist (with over 15 years of experience) was invited to resolve any discrepancies. Next, radiological features (a total of 48), such as lesion number, lesion size, and lesion type, were extracted manually (an operator-based visual assessment of radiological features). The kappa coefficient was also calculated to determine the inter-observer and intra-observer variability between readers.
As shown in Figure 2, the images were automatically quantified by an AI-based system (YT-CT-Lung, YITU Healthcare Technology Co., Ltd., China), which combined a fully convolutional network with adaptive threshold and morphological operations for segmentation of the lungs and pneumonia lesions under the supervision of two board-certified radiologists with more than five years of experience (16, 17) to re-examine the lesions and improve the AI Software performance. External validation with 383 axial CT scans of 206 patients indicated a dice coefficient of 82.08% for COVID-19 pneumonia lesion segmentation, which is close to the segmentation performance for lung nodules in two datasets (82.15% and 80.02%, respectively), as reported in a study by Wang et al. (18). To be more time-efficient and economical, in the test set, only selected radiological indicators in the final models were extracted manually (using the aforementioned procedures) instead of all Manually extracted features; no significant difference was found in the AI-based quantization process.
The quantitative evaluation system for COVID-19. The first columns represent the original CT scans. The second column represents automatic quantization and visualization by the AI system. 2A-2B, Original CT scans and quantization and visualization by the AI system for a 38-year-old patient at the time of hospital admission. 2C-2D, CT scans for patient with non-progressing pneumonia 12 days after admission. 2E-2F, Original CT scans and quantization and visualization for a 28-year-old patient on admission. 2G-2H, CT scans for a 28-year-old patient with progressing pneumonia 12 days after admission (red and blue pseudo colors represent ground glass opacity and consolidation, respectively).
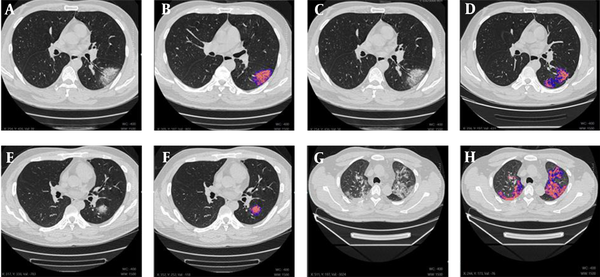
The AI system first segments the entire lung into pulmonary segments, as described by Ronneberger et al. (19). Next, a central focused convolutional neural network (CF-CNN) (18) was used to locate and analyze pneumonia lesions in each region. By using a threshold for the CT values of pneumonia lesions, the distribution of CT values in the lungs was calculated to obtain a histogram, which was subsequently used to calculate the pulmonary inflammation volume. Finally, radiological features, including the volume of lesion, ground glass opacity, and consolidation (and their proportions), as well as the volume percentages of CT values in ranges of -700 to -500 Hounsfield units (HU), -500 to -200 HU, and -200 to 60 HU, respectively, were reported using the AI technology. Overall, 126 AI-based quantitative features were extracted, as shown in Appendix 1 in Supplementary File.
3.5. Feature Selection and Model Construction
The Boruta algorithm (20), which is designed as a wrapper built around the random forest classification algorithm in the R package, was used to select the features after analyzing the correlations between features and omitting highly correlated features. To avoid randomness and obtain more consistent results, the five-fold cross-validation method was used with different numbers of seeds for (1) only clinical factors (including nine demographic and 17 laboratory indictors) (Table 1); (2) clinical features combined with 48 physician-extracted radiological features (Appendix 2 in Supplementary File); and (3) clinical features with 126 AI-derived radiological features (Appendix 1 in Supplementary File) in the training set to confirm the most important attributes for progression into COVID-19 pneumonia. Only features confirmed in all cross-validations were included to construct the predictive models.
The Basic Characteristics of the Training and Test Sets
Variables | Total | Training set | Test set | P-value |
---|---|---|---|---|
No. | 786 | 628 | 158 | |
Age (median [IQR]) | 56.00 [42.00, 65.00] | 50.50 [36.75, 58.75] | 61.50 [52.75, 69.00] | < 0.001 |
Sex, female (%) | 432 (55.0) | 322 (51.3) | 110 (69.6) | 0.433 |
Comorbidity, yes (%) | 382 (48.6) | 298 (47.4) | 84 (53.2) | 0.888 |
Hypertension | 146 (38.3) | 116 (38.9) | 32 (38.1) | 0.565 |
Diabetes | 101 (26.4) | 83 (27.8) | 21 (25.0) | 0.557 |
Cardiovascular disease | 67 (17.5) | 41(13.8) | 18 (21.5) | 0.268 |
Others | 68 (17.8) | 58 (19.5) | 13 (15.5) | 0.494 |
Primary symptoms (%) | ||||
Fever | 550 (70.0) | 438 (69.7) | 112 (70.8) | 0.545 |
Cough | 404 (51.4) | 330 (52.6) | 74 (48.6) | 0.444 |
Fatigue | 387 (49.3) | 256 (40.8) | 131 (82.9) | 0.021 |
Myalgia | 314 (39.9) | 257 (40.9) | 57 (36.1) | 0.352 |
Others | 180 (22.9) | 157 (25.0) | 23 (14.6) | 0.325 |
Outcomes, progressing (%) | 238 (30.3) | 197 (31.3) | 41 (25.9) | 0.506 |
Laboratory indicators | ||||
CK, U/L (ref: 40 - 200) | 70.00 [45.00, 101.00] | 77.00 [56.00, 100.50] | 62.00 [39.50, 105.00] | 0.224 |
LDH, U/L (ref: 120 - 250) | 211.00 [179.00, 277.00] | 218.00 [186.25, 277.00] | 201.00 [168.50, 257.50] | 0.084 |
ALT, U/L (ref: 7 - 40) | 20.00 [13.00, 30.50] | 19.00 [13.00, 30.00] | 22.00 [13.00, 36.00] | 0.28 |
AST, U/L (ref: 13 - 35) | 25.00 [17.50, 31.00] | 24.00 [18.00, 30.25] | 25.00 [17.00, 31.50] | 0.87 |
TBIL, μmol/L (ref: 0 - 23) | 9.15 [7.40, 12.55] | 8.50 [7.40, 12.20] | 9.50 [7.20, 13.55] | 0.494 |
Cr, μmol/L (ref: 41 - 73) | 60.00 [50.50, 73.50] | 61.00 [51.75, 78.00] | 58.00 [48.50, 71.00] | 0.054 |
Urea, mmol/L (ref: 2.6 - 7.5) | 4.15 [3.33, 5.16] | 4.22 [3.58, 5.39] | 4.11 [3.17, 5.09] | 0.159 |
PLT, 109/L (ref: 125 - 350) | 181.00 [143.50, 242.00] | 157.00 [131.50, 196.00] | 230.00 [160.00, 260.00] | < 0.001 |
Mono, 109/L (ref: 0.1 - 0.6) | 0.44 [0.33, 0.57] | 0.45 [0.33, 0.58] | 0.44 [0.34, 0.57] | 0.927 |
Lym, 109/L (ref: 1.1 - 3.2) | 1.24 [0.94, 1.62] | 1.19 [0.90, 1.57] | 1.31 [0.96, 1.71] | 0.185 |
WBC, 109/L (ref: 3.5 - 9.5) | 5.19 [3.81, 6.13] | 4.78 [3.66, 6.07] | 5.45 [3.96, 6.42] | 0.12 |
Neu, 109/L (ref: 1.8 - 6.3) | 3.14 [2.19, 4.20] | 3.09 [2.13, 4.05] | 3.14 [2.33, 4.45] | 0.236 |
Lym, % (ref: 20 - 50) | 26.80 [20.05, 34.50] | 27.05 [20.08, 34.52] | 26.80 [20.30, 34.10] | 0.99 |
CRP, mg/L (ref: 0 - 10) | 12.66 [4.14, 32.95] | 12.59 [4.03, 33.53] | 13.65 [4.60, 29.48] | 0.976 |
ALB, g/L (ref: 40 - 55) | 39.50 [36.66, 42.20] | 39.90 [36.65, 42.12] | 38.80 [36.66, 42.25] | 0.837 |
DBIL, μmol/L (ref: 0 - 8.0) | 3.25 [2.52, 4.30] | 3.20 [2.50, 4.20] | 3.30 [2.65, 4.45] | 0.686 |
GLB, g/L (ref: 20 - 40) | 23.30 [21.60, 25.40] | 23.35 [21.92, 25.50] | 22.85 [21.07, 24.30] | 0.146 |
The selected features were then used to develop three predictive models based on a logistic regression analysis for the training set, including a clinical feature-based model (model C), a model based on the physician-extracted radiological features (R-Doc model), and a model based on the AI-derived radiological features (R-AI model). To mimic how radiologists examine CT scans, an AI-based model mimicking the physician’s examination (AI-Mimic-Doc model) was developed by using the indicators of R-Doc model, with the original radiological features replaced by AI-derived values. The numerical values were reclassified into two categories, similar to the R-Doc model, to keep them as compatible as possible. Next, the coefficients for each model were measured to determine the risk scores for both the training and test sets. Moreover, the receiver operating characteristic (ROC) curves were drawn to evaluate the sensitivity and specificity of the models for predicting disease progression. The cutoff values were determined using the Youden’s Index, and high-risk and low-risk patients were dichotomized accordingly. The source codes obtained using the ROCR package (R version 3.6.2) are freely available in here.
3.6. Statistical Analysis
The clinical characteristics and radiological features, stratified by datasets (training vs. test), are described as mean and standard deviation for normally distributed variables, interquartile range (median [Q25-Q75]) for variables without a normal distribution, or frequency and percentage for categorical variables. Differences between variables were compared using t-test, Mann-Whitney U test, and chi-square test (or Fisher’s exact probability test) to ensure a balanced distribution of the patients. The DeLong test was also used to compare the AUCs of different models.
The high- and low-risk groups were predicted based on the cutoff values (median of risk scores for each model). A decision curve analysis was also performed to evaluate the models. Additionally, the Kendall’s rank coefficient was measured to evaluate correlations between clinical variables (eg, age with albumin level and comorbidities with urea) and explain why some of the common clinical indicators were not selected in the final models. A two-tailed P-value less than 0.05 was considered statistically significant. All analyses were conducted in R-3.6.2 Software (R Foundation for Statistical Computing, Vienna, Austria).
4. Results
4.1. Demographic Characteristics
A total of 876 COVID-19 patients were enrolled in this study, 30.3% (n = 71) of whom progressed into severe or critical pneumonia. The median age of the patients was 56.0 years, and 77 (55%) patients were female. Overall, 48.6% of the patients had comorbidities, including hypertension (38.2%), diabetes (26.5%), and cardiovascular disease (17.6%). Fever (70.0%), cough (51.4%), fatigue (49.3%), and myalgia (40.0%) were the most common primary symptoms in this retrospective cohort study. The patients’ laboratory findings are shown in Table 1.
4.2. Lung Lesion Quantization
Forty-eight handcrafted lung lesion features were delineated and extracted by radiologists (Appendix 2 in Supplementary File). The radiologists had adequate inter-observer (kappa coefficient = 0.86) and moderate intra-observer (kappa coefficient = 0.75) agreement. Overall, 126 AI-derived radiological features were quantified by the YT-CT-Lung Quantitative Evaluation System (Appendix 1 in Supplementary File). As shown in Figure 2, the lung lesions could be detected and visualized automatically by the AI system, with the quantified lesion volume and its proportion. The percentage of ground glass opacity and consolidation volume are represented by different pseudo colors (red and blue).
4.3. Representative Features and Model Construction
A total of 200 variables, including nine clinical features, 17 laboratory indicators, 126 AI-derived radiological features, and 48 radiologist-extracted radiological features, were analyzed in this study. After implementing the Boruta algorithm five times for feature selection, the confirmed attributes for each model were as follows: (1) urea and albumin for model C; (2) urea, albumin, and lesion size in the basal segment of the right lower lobe (RLL) lesions for the R-Doc model: and (3) urea, albumin level, and percentage of CT values of -200 to 60 in the left lung for model R-AI. The AI-Mimic-Doc model was identical to the R-Doc model, with the exception that lesion size in the RLL was derived from AI, as presented in Table 2.
Representative Features of 4 Predictive Models for Disease Progressiona
Variables | Model-C | Model-R-Doc | Model-AI-Mimic-Doc | Model-R-AI | ||||||||
---|---|---|---|---|---|---|---|---|---|---|---|---|
Beta | OR (95% CI) | P-value | Beta | OR (95% CI) | P-value | Beta | OR (95% CI) | P-value | Beta | OR (95% CI) | P-value | |
Urea | 0.57 | 1.77 (1.22 - 2.57) | 0.003 | 0.69 | 1.98 (1.30 - 3.03) | 0.001 | 0.55 | 1.73 (1.19 - 2.53) | 0.004 | 0.65 | 1.91 (1.26 - 2.89) | 0.002 |
Albumin | -0.17 | 0.84 (0.74 - 0.97) | 0.015 | - 0.16 | 0.85 (0.73 - 0.99) | 0.036 | - 0.14 | 0.87 (0.75 - 1.00) | 0.052 | - 0.14 | 0.87 (0.75 - 0.99) | 0.042 |
Lesion size in basal segment of RLL (ref: none) | ||||||||||||
< 3 cm | 0.26 | 1.30 (0.29 - 5.83) | 0.733 | |||||||||
≥ 3 cm | 2.09 | 8.11 (1.49 - 44.09) | 0.015 | |||||||||
Lesion volume in basal segment of RLL (AI-derived) | ||||||||||||
< (1.5 × 0.5 × 0.5) cm3 | 0.97 | 2.65 (0.83 - 8.40) | 0.099 | |||||||||
≥ (1.5 × 0.5 × 0.5) cm3 | 1.1 | 2.99 (0.52 - 17.08) | 0.218 | |||||||||
PCTL [-200~60) (ref: < 1.77) | 1.13 | 3.10 (1.00 - 9.58) | 0.050 |
4.4. Construction and Validation of Risk Scores
The risk scores for disease progression were measured in non-severe COVID-19 patients according to the coefficients (ie, beta coefficients in Table 2) in the four predictive models; their distribution is presented in Figure 3. As shown in Table 3, in model C, the AUC was 0.770 (95% CI: 0.657 - 0.0.882) for the training set, which reduced to 0.666 for the test set. Compared to model C, the AUC was marginally higher in the R-Doc model (0.840, 95% CI: 0.747 - 0.933) for the training set (P < 0.1), while there was no significant difference for the test set.
The area under the curve (AUC) of different models for the training (based on five-fold cross-validation) and test cohort sets. The five-fold CV cross-validation training set comprised 80% of the training set, and the five5-fold cross-validation set comprised 20% of the training set. Model-R-Doc, a model based on the physician’s examination of radiological features; Model-R-AI, a based on AI-derived radiological features; Model-C, a model based on clinical findings; Model-AI-Mimic-Doc, an AI-based model mimicking the physician’s examinations.
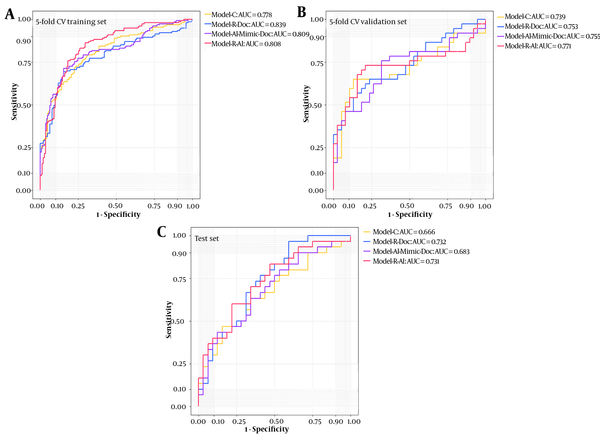
Models | Cutoff value | Training set | Test set | ||||||
---|---|---|---|---|---|---|---|---|---|
Sensitivity | Specificity | AUC (95% CI) | P-value | Sensitivity | Specificity | AUC (95% CI) | P- value | ||
Model C | -4.264 | 0.711 | 0.722 | 0.770 (0.657 - 0.882) | Ref | 0.600 | 0.656 | 0.666 (0.528 - 0.803) | Ref |
R-Doc model | -3.097 | 0.789 | 0.806 | 0.840 (0.747 - 0.933) | 0.099 | 0.833 | 0.469 | 0.732 (0.608 - 0.857) | 0.177 |
AI-Mimic-Doc model | -2.542 | 0.711 | 0.722 | 0.793 (0.689 - 0.897) | 0.357 | 0.500 | 0.688 | 0.683 (0.549 - 0.818) | 0.655 |
R-AI model | -2.240 | 0.763 | 0.778 | 0.803 (0.701 - 0.906) | 0.198 | 0.600 | 0.781 | 0.731 (0.606 - 0.857) | 0.086 |
Conversely, in the R-AI model, the AUCs were 0.803 and 0.731 for the training and test sets, respectively; there was only a marginal difference in the latter set (P = 0.086) by considering the results of model C as the reference. However, the AUCs of the AI-Mimic-Doc model were not significantly different from those of model C for both training and test sets. Considering the cutoff risk scores of -3.097 and -2.240, the R-Doc model showed high sensitivity (0.833) for predicting disease progression, while good specificity (0.781) was attributed to the R-AI model. The ROC curves for the training (based on five-fold cross-validation) and test cohorts are presented in Figure 3. The decision curves for the four models are also presented in Appendix 3 in Supplementary File.
5. Discussion
In this single-center retrospective study, different models were developed and examined based on clinical factors, along with radiological features, to predict COVID-19 patients at risk of progression into severe or critical pneumonia upon hospital admission. The integration of urea, albumin, and AI-derived features (percentage of CT values of -200 to 60 in the left lung) in the models yielded satisfactory results in this cohort. Therefore, clinicians could use these models to evaluate the risk of disease progression for every COVID-19 patient on admission and administer personalized treatments accurately. Based on the models, patients with a low risk of progression into pneumonia can be monitored and treated in routine clinical practice, while early emergency airway management/oxygen therapy or ICU admission is needed for high-risk patients.
Although several studies have investigated the influential factors for COVID-19, urea has been never considered as a risk factor. A recent study by Bonetti et al. found that urea was significantly higher in COVID-19 patients who expired during hospitalization (21). Based on their findings, COVID-19 patients with kidney damage, caused by the overexpression of angiotensin-converting enzyme 2 (ACE2) (22), are prone to progression into severe pneumonia. Therefore, early interventions to protect the kidney function after hospital admission are highly recommended. Besides, albumin is an important laboratory factor, representing liver injury to some extent; it may also indicate disease progression. As CT scans can clearly indicate a lung injury, and clinical factors can indicate other visceral damages, the combination of radiological features with clinical factors can help predict the COVID-19 progression effectively.
Radiological features, unlike clinical factors, have been rarely used in risk models for predicting COVID-19 progression. In this study, radiological features were extracted manually. We tried to select AI-derived features similar to the handcrafted ones to compare the prediction performance of these two models. Similar to previous research (5, 14), CT imaging features played an important role in identifying COVID-19 progression from non-severe to severe/critical pneumonia. However, unlike previous studies that primarily focused on the lesion size or features of the entire lung, lesion size in the basal segment of RLL was significantly related to the progression of COVID-19. This finding is mainly due to the fact that lesions in the basal segment of RLL are more difficult to be absorbed compared to lesions in other segments of the lung; therefore, particular attention should be paid to this region in the clinical management of COVID-19. To the best of our knowledge, this is the first report to consider lesion size in the basal segment of RLL for the prediction of COVID-19 prognosis; this model was found to be reliable and stable in this retrospective cohort.
Additionally, we found that the percentage of CT values of -200 to 60 in the left lung, derived from AI, significantly contributed to the COVID-19 progression. Interestingly, evaluation of the predictive performance of the AI-based radiological model indicated more stable and reliable AUCs for the cross-validation training, validation, and test sets, compared to measurements by radiologists; this difference might arise from differences in CT readings between computers and humans. Overall, the mechanically derived CT value is more objective and stable than the lesion volume and type determined by the human eyes. It also provided some helpful cues for the rapid detection of high-risk patients based on automatically derived imaging features rather than a laborious and time-consuming reading process by physicians.
The percentages of CT values of -200 to 60 in the left lung, mainly linked to pulmonary fibrosis, are consistent with previous studies, including volumetric measurements as prognostic factors for pulmonary disease (23-25). Nevertheless, radiological features selected in AI- and doctor-based models were different, which is probably due to the fact that imaging findings indicating pulmonary fibrosis were not measured by radiologists (it was not considered as a potential sign of disease progression at the beginning of the study). However, pulmonary fibrosis in the left lung, according to our findings, should be emphasized in the management of COVID-19 pneumonia, and it is our future research interest.
In the present study, age and comorbidities were not selected in the final predictive models. Both age and comorbidities were highly correlated with urea and albumin level, with Kendall’s tau coefficients of 0.158, 0.382, -0.360, and -0.246, respectively (Appendix 4 in Supplementary File). Patients with excessive urea and albumin levels were consistently older and had a coexisting disease. Additionally, renal and hepatic involvements have been observed in the early stage of pneumonia in several recent studies (15, 26), and aberrant urea and albumin levels have been detected; overall, these laboratory indicators could be earlier predictive factors for disease progression. We also acknowledge that some cases might have developed into a critical disease; however, it would not affect our model performance.
This study had some limitations. First, it only included patients from a single hospital with a limited sample size for model construction. Also, the sample size for validation was limited; therefore, a larger sample size should be recruited from areas outside Hubei Province and even China. Second, the applied treatments were not included as risk factors for model construction. However, no effective treatment has been proposed for COVID-19 so far. More importantly, in this retrospective cohort study, all the patients were treated using a similar therapeutic approach, such as oxygen therapy and antibiotic treatment; therefore, treatments do not seem to predict COVID-19 progression. The present results are not inclusive or generalizable to all COVID-19 patients; they are only generalizable to COVID-19 patients with both CT involvement and dyspnea upon admission.
In conclusion, predictive models were developed in the present study, combining clinical and radiological features for the early identification of COVID-19 patients at a high risk of disease progression upon hospital admission. By using both radiological features and handcrafted blood results, early identification of COVID-19 patients at risk of disease progression can be achieved upon hospital admission (rapidly if using AI). The present model could be generalizable to patients with mild pneumonia in the first stages of disease or at the time of assessment to guide the clinic management of COVID-19 and optimize public health resource allocation.
References
-
1.
World Health Organization. Clinical management of COVID-19: Interim guidance. World Health Organization; 2020.
-
2.
Wu Z, McGoogan JM. Characteristics of and Important Lessons From the Coronavirus Disease 2019 (COVID-19) Outbreak in China: Summary of a Report of 72314 Cases From the Chinese Center for Disease Control and Prevention. JAMA. 2020;323(13):1239-42. [PubMed ID: 32091533]. https://doi.org/10.1001/jama.2020.2648.
-
3.
Bai HX, Wang R, Xiong Z, Hsieh B, Chang K, Halsey K, et al. Artificial Intelligence Augmentation of Radiologist Performance in Distinguishing COVID-19 from Pneumonia of Other Origin at Chest CT. Radiology. 2020;296(3). [PubMed ID: 32339081]. [PubMed Central ID: PMC7233483]. https://doi.org/10.1148/radiol.2020201491.
-
4.
Li L, Qin L, Xu Z, Yin Y, Wang X, Kong B, et al. Artificial Intelligence Distinguishes COVID-19 from Community Acquired Pneumonia on Chest CT. Radiology. 2020. https://doi.org/10.1148/radiol.2020200905.
-
5.
Zhang K, Liu X, Shen J, Li Z, Sang Y, Wu X, et al. Clinically Applicable AI System for Accurate Diagnosis, Quantitative Measurements, and Prognosis of COVID-19 Pneumonia Using Computed Tomography. Cell. 2020;181(6):1423-1433 e11. [PubMed ID: 32416069]. [PubMed Central ID: PMC7196900]. https://doi.org/10.1016/j.cell.2020.04.045.
-
6.
Ji MY, Yuan L, Jiang XD, Zeng Z, Zhan N, Huang PX, et al. Nuclear shape, architecture and orientation features from H&E images are able to predict recurrence in node-negative gastric adenocarcinoma. J Transl Med. 2019;17(1):92. [PubMed ID: 30885234]. [PubMed Central ID: PMC6423755]. https://doi.org/10.1186/s12967-019-1839-x.
-
7.
Kather JN, Pearson AT, Halama N, Jager D, Krause J, Loosen SH, et al. Deep learning can predict microsatellite instability directly from histology in gastrointestinal cancer. Nat Med. 2019;25(7):1054-6. [PubMed ID: 31160815]. [PubMed Central ID: PMC7423299]. https://doi.org/10.1038/s41591-019-0462-y.
-
8.
Mei X, Lee HC, Diao KY, Huang M, Lin B, Liu C, et al. Artificial intelligence-enabled rapid diagnosis of patients with COVID-19. Nat Med. 2020;26(8). [PubMed ID: 32427924]. [PubMed Central ID: PMC7446729]. https://doi.org/10.1038/s41591-020-0931-3.
-
9.
Li K, Fang Y, Li W, Pan C, Qin P, Zhong Y, et al. CT image visual quantitative evaluation and clinical classification of coronavirus disease (COVID-19). Eur Radiol. 2020;30(8):4407-16. [PubMed ID: 32215691]. [PubMed Central ID: PMC7095246]. https://doi.org/10.1007/s00330-020-06817-6.
-
10.
Hashmi HAS, Asif HM. Early Detection and Assessment of Covid-19. Front Med (Lausanne). 2020;7:311. [PubMed ID: 32582748]. [PubMed Central ID: PMC7296153]. https://doi.org/10.3389/fmed.2020.00311.
-
11.
Yang X, Yu Y, Xu J, Shu H, Xia J, Liu H, et al. Clinical course and outcomes of critically ill patients with SARS-CoV-2 pneumonia in Wuhan, China: a single-centered, retrospective, observational study. Lancet Respir Med. 2020;8(5):475-81. https://doi.org/10.1016/s2213-2600(20)30079-5.
-
12.
Zhou F, Yu T, Du R, Fan G, Liu Y, Liu Z, et al. Clinical course and risk factors for mortality of adult inpatients with COVID-19 in Wuhan, China: a retrospective cohort study. Lancet. 2020;395(10229):1054-62. https://doi.org/10.1016/s0140-6736(20)30566-3.
-
13.
Li Y, Shang K, Bian W, He L, Fan Y, Ren T, et al. Prediction of disease progression in patients with COVID-19 by artificial intelligence assisted lesion quantification. Sci Rep. 2020;10(1):22083. [PubMed ID: 33328512]. [PubMed Central ID: PMC7745019]. https://doi.org/10.1038/s41598-020-79097-1.
-
14.
Liang W, Liang H, Ou L, Chen B, Chen A, Li C, et al. Development and Validation of a Clinical Risk Score to Predict the Occurrence of Critical Illness in Hospitalized Patients With COVID-19. JAMA Intern Med. 2020;180(8). [PubMed ID: 32396163]. [PubMed Central ID: PMC7218676]. https://doi.org/10.1001/jamainternmed.2020.2033.
-
15.
Ji M, Yuan L, Shen W, Lv J, Li Y, Li M, et al. Characteristics of disease progress in patients with coronavirus disease 2019 in Wuhan, China. Epidemiol Infect. 2020;148. e94. [PubMed ID: 32374248]. [PubMed Central ID: PMC7225215]. https://doi.org/10.1017/S0950268820000977.
-
16.
Liu F, Zhang Q, Huang C, Shi C, Wang L, Shi N, et al. CT quantification of pneumonia lesions in early days predicts progression to severe illness in a cohort of COVID-19 patients. Theranostics. 2020;10(12):5613-22. [PubMed ID: 32373235]. [PubMed Central ID: PMC7196293]. https://doi.org/10.7150/thno.45985.
-
17.
Wang C, Huang P, Wang L, Shen Z, Lin B, Wang Q, et al. Temporal changes of COVID-19 pneumonia by mass evaluation using CT: a retrospective multi-center study. Ann Transl Med. 2020;8(15):935. [PubMed ID: 32953735]. [PubMed Central ID: PMC7475384]. https://doi.org/10.21037/atm-20-4004.
-
18.
Wang S, Zhou M, Liu Z, Liu Z, Gu D, Zang Y, et al. Central focused convolutional neural networks: Developing a data-driven model for lung nodule segmentation. Med Image Anal. 2017;40:172-83. [PubMed ID: 28688283]. [PubMed Central ID: PMC5661888]. https://doi.org/10.1016/j.media.2017.06.014.
-
19.
Ronneberger O, Fischer P, Brox T. U-Net: Convolutional Networks for Biomedical Image Segmentation. . Medical Image Computing and Computer-Assisted Intervention; 2015. p. 234-41. https://doi.org/10.1007/978-3-319-24574-4_28.
-
20.
Kursa MB, Rudnicki WR. Feature Selection with theBorutaPackage. J Stat Softw. 2010;36(11). https://doi.org/10.18637/jss.v036.i11.
-
21.
Bonetti G, Manelli F, Patroni A, Bettinardi A, Borrelli G, Fiordalisi G, et al. Laboratory predictors of death from coronavirus disease 2019 (COVID-19) in the area of Valcamonica, Italy. Clin Chem Lab Med. 2020;58(7):1100-5. [PubMed ID: 32573995]. https://doi.org/10.1515/cclm-2020-0459.
-
22.
Li MY, Li L, Zhang Y, Wang XS. Expression of the SARS-CoV-2 cell receptor gene ACE2 in a wide variety of human tissues. Infect Dis Poverty. 2020;9(1):45. [PubMed ID: 32345362]. [PubMed Central ID: PMC7186534]. https://doi.org/10.1186/s40249-020-00662-x.
-
23.
Yanagawa M, Tanaka Y, Kusumoto M, Watanabe S, Tsuchiya R, Honda O, et al. Automated assessment of malignant degree of small peripheral adenocarcinomas using volumetric CT data: correlation with pathologic prognostic factors. Lung Cancer. 2010;70(3):286-94. [PubMed ID: 20392516]. https://doi.org/10.1016/j.lungcan.2010.03.009.
-
24.
Yanagawa M, Tanaka Y, Leung AN, Morii E, Kusumoto M, Watanabe S, et al. Prognostic importance of volumetric measurements in stage I lung adenocarcinoma. Radiology. 2014;272(2):557-67. [PubMed ID: 24708191]. https://doi.org/10.1148/radiol.14131903.
-
25.
Scholten ET, Jacobs C, van Ginneken B, van Riel S, Vliegenthart R, Oudkerk M, et al. Detection and quantification of the solid component in pulmonary subsolid nodules by semiautomatic segmentation. Eur Radiol. 2015;25(2):488-96. [PubMed ID: 25287262]. https://doi.org/10.1007/s00330-014-3427-z.
-
26.
Pei G, Zhang Z, Peng J, Liu L, Zhang C, Yu C, et al. Renal Involvement and Early Prognosis in Patients with COVID-19 Pneumonia. J Am Soc Nephrol. 2020;31(6):1157-65. [PubMed ID: 32345702]. [PubMed Central ID: PMC7269350]. https://doi.org/10.1681/ASN.2020030276.