Abstract
Background:
Radiographs, adjunct to clinical examination are always valuable complementary methods for dental caries detection. Recently, progressing in digital imaging system provides possibility of software designing for automatically dental caries detection.Objectives:
The aim of this study was to develop and assess the function of diagnostic computer software designed for evaluation of approximal caries in posterior teeth. This software should be able to indicate the depth and location of caries on digital radiographic images.Materials and Methods:
Digital radiographs were obtained of 93 teeth including 183 proximal surfaces. These images were used as a database for designing the software and training the software designer. In the design phase, considering the summed density of pixels in rows and columns of the images, the teeth were separated from each other and the unnecessary regions; for example, the root area in the alveolar bone was eliminated. Therefore, based on summed intensities, each image was segmented such that each segment contained only one tooth. Subsequently, based on the fuzzy logic, a well-known data-clustering algorithm named fuzzy c-means (FCM) was applied to the images to cluster or segment each tooth. This algorithm is referred to as a soft clustering method, which assigns data elements to one or more clusters with a specific membership function. Using the extracted clusters, the tooth border was determined and assessed for cavity. The results of histological analysis were used as the gold standard for comparison with the results obtained from the software. Depth of caries was measured, and finally Intraclass Correlation Coefficient (ICC) and Bland-Altman plot were used to show the agreement between the methods.Results:
The software diagnosed 60% of enamel caries. The ICC (for detection of enamel caries) between the computer software and histological analysis results was determined as 0.609 (95% confidence interval [CI] = 0.159-0.849) (P = 0.006). Also, the computer program diagnosed 97% of dentin caries and the ICC between the software and histological analysis results for dentin caries was determined as 0.937 (95% CI=0.906-0.958) (P < 0.001). Bland-Altman plot showed an acceptable agreement for measuring the depth of caries in enamel and dentin.Conclusions:
The designed software was able to detect a significant number of dentin caries and acceptable measuring of the depth of carious lesions in enamel and dentin. However, the software had limited ability in detecting enamel lesions.Keywords
1. Background
Radiography along with clinical examination is the most important diagnostic method for dental caries (1). Diagnosis of approximal caries and choosing the best treatment plan for them are among the most common problems encountered in clinical dentistry (2). Even experienced clinicians have moderate accuracy and expertise in diagnosing proximal caries on a dental radiograph (3, 4). Studies have reported a sensitivity of 0.4 - 0.6 for conventional radiographs in diagnosing dental caries (4). Also, some researchers in UCLA showed that dentists misdiagnosed the depth of lesions up to 40% by using conventional radiography and in 20% of cases they misdiagnosed sound teeth as carious (5). Thus, it is not unusual if different dentists have different judgments about the same radiographs (6, 7). This is not because of their different therapeutic viewpoints but due to different diagnoses of presence or extensiveness of the lesions on the radiographs (7); this difficulty in diagnosis is due to the fact that the eyes tend to smooth the gray shadows (8). Therefore, radiography has some limitations and is unable to reveal the first stage of caries. It also underestimates the size of demineralized area (9). NIH (National Institute of Health) has mentioned the necessity of improving the accuracy of radiographic methods for caries diagnosis (10, 11). During the recent years, advances in digital radiography have made it possible to use caries detector softwares for diagnosing dental caries. These softwares can measure the grey shadows more precisely in the scale of 0-250 (12). Logicon caries detector is new FDA-approved software for this purpose that helps dentists detect proximal caries on intra-oral digital radiographs (13). This software can separate dentin caries that need to be restored from enamel lesions. Logicon can analyze the changes in radiographic density, detect the demineralized parts of the tooth and also determine whether it is enamel or dentin caries (8), but reports on its accuracy are controversial (8, 13).
2. Objectives
In the present study, we decided to design a software in our country for the detection of proximal caries in the posterior teeth (molars and premolars) and determination of depth and position of carious lesions by analyzing digital radiographs.
3. Materials and Methods
A total of 93 extracted posterior teeth were used in the design phase and 128 in the assessment phase of the software with no restorative materials. None of the teeth had any fracture, or anomalies in shape or size and no gross caries. The teeth had to have intact enamel in the gingival wall of the proximal cavity. The teeth with occlusal, buccal or lingual caries were excluded from the study. Before designing the software, digital radiographs were obtained from proximal surfaces of 183 teeth using Dixi® digital radiographic system (ZV3, Planmeca, Helsinki, Finland). These images were used to train the software designer and to extract basic information. The teeth were stored in 10% formalin solution for more than 24 hours for disinfection and mounted in blocks made of plaster and sawdust in a way that three teeth were placed next to each other with no overlap in their interproximal surfaces. Soft tissue wax up was performed using one layer of 18mm diameter plexiglass sheet and CCD sensor (Dixi, Planmeca, Helsinki, Finland) was placed parallel to the teeth. In order to ensure the reproducibility of the radiographic positioning, the sensor and dental block were placed parallel in a custom-made device for guiding X ray beams, so that the distance of sensor from the source remained constant (25 cm) in all radiographies. Periapical radiographs were taken with Gendex intraoral X ray system (Dentsply, IL, USA, 65kVp, 7 mA), using parallel technique with an exposure time of 0.08. These images were stored in JPEG format. In this phase, the software designer needed some basic information about the shape and form of proximal caries. Routinely, in clinic, when dentists or oral radiologists evaluate dental radiographs in terms of existence and extension of caries, they determine the margin of caries regarding to all radiographic criteria and based on caries extension into the teeth, that appears as a radiolucent area with a density less than tooth structure, and then they decide about the treatment. In this study, for definition and instruction of caries margins to the software designer who was not familiar to definition of dental caries in digitized radiographs, caries margins and the extent of caries were determined manually by drawing a line using Photoshop software by an expert radiologist. We also shared a number of intact caries free teeth with the designer to let him know how the intact surfaces appear in a radiograph, thus reducing the false positive records as much as possible (Figure 1).
Caries margins and the extent of caries that are marked manually with a red line to define these areas to help the software engineer. The first tooth from the right shows one of these intact samples.
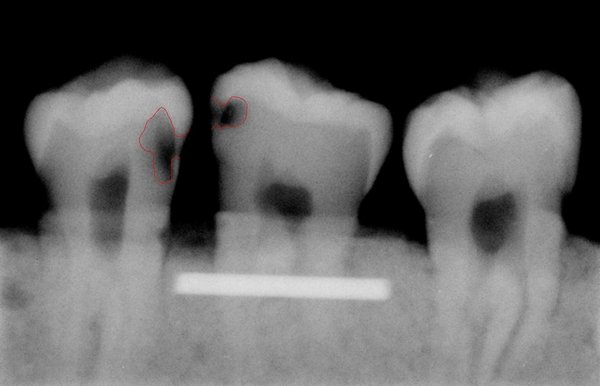
4. Results
Of 25 surfaces with enamel caries, the caries detector software was able to diagnose 15 surfaces. In other words, the software diagnosed 60% of enamel caries. The ICC between the software and histological analysis results for detection of enamel caries was determined as 0.609 (95% CI = 0.159-0.849) which was statistically significant (P = 0.006) (Table 1). Also, of 95 proximal surfaces with dentin caries, the software was able to successfully diagnose 93 of them. In other words, the software diagnosed 97% of dentin caries. The ICC between the software and histological analysis results for dentin caries was determined to be 0.937 (95% CI = 0.906-0.958), which was statistically significant (P < 0.001) (Table 1).
The agreement between the results of designed software and histopathology for measuring the depth of caries was investigated by the Bland-Altman plot (Figures 7 and 8). For enamel, only one measurement (4%) and for dentin, four measurements (4.2%) were beyond the acceptable area of the plot (95% CI for mean difference of measurements). This proposed a good and acceptable agreement.
Statistical Indices for Enamel and Dentin Lesions
Location | Number of Proximal Surfaces With Caries | Number of Surfaces Detected With Software | Percentage of Surfaces Detected With Software | ICC | 95% confidence interval for ICC |
---|---|---|---|---|---|
Enamel Lesions | 25 surfaces | 15 surfaces | 60% | 0.609 | 0.159-0.849 |
Dentin Lesions | 95 surfaces | 93 surfaces | 97% | 0.937 | 0.906-0.958 |
Bland-Altman plot shows an acceptable agreement for measuring the depth of caries in dentin between the software and histology results (n = 95).
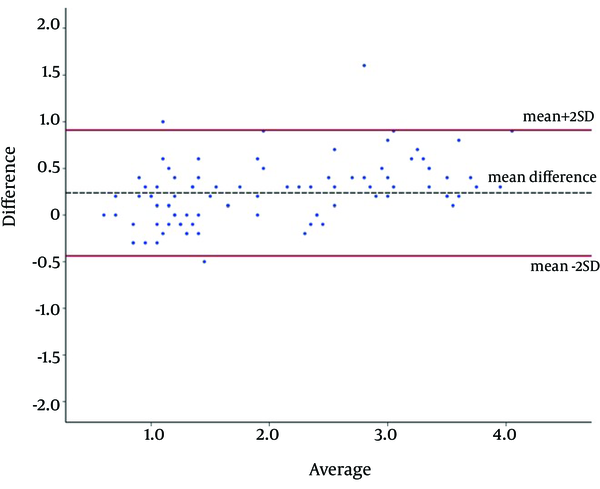
Bland-Altman plot shows an acceptable agreement for measuring the depth of caries in enamel between the software and histology results (n = 25).
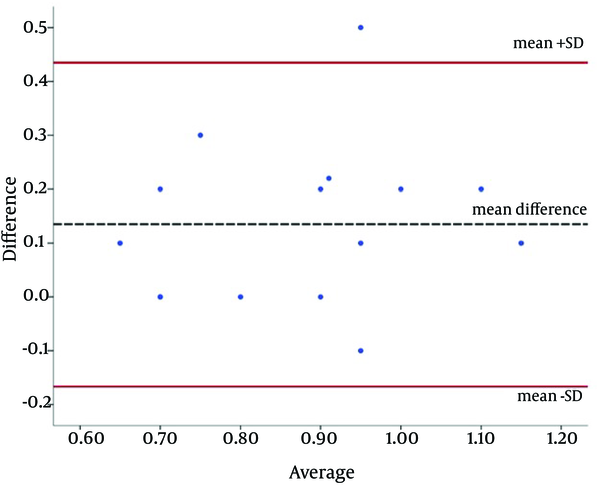
5. Discussion
Advances in digital radiography have made it possible to use automated caries detection tools for diagnosing dental caries (8). The previous studies only concentrated on the radiographic diagnostic accuracy of these softwares. However, the International Consensus Workshop in 2002 recommended the application of tools for quantitative assessment of the progression of carious lesions (14-16). Thus, in the present study, we tried to design a software with higher diagnostic accuracy to determine the depth of carious lesions. According to previous studies, the images obtained by Dixi digital radiographic system (Planmeca) are more accurate than those taken by other systems for determining the depth of caries (14). Therefore, Dixi system was used in this study. However, controversy still exists when comparing histological and radiographic depth of carious lesions (14). Since mechanically made carious lesions have greater chance to be diagnosed (14), we decided to establish our study on natural caries; so, histologic measurement of natural caries depth was used as the gold standard.
In the present study, natural caries and histologic measurement of depth was used as the gold standard because the lesions that were caused mechanically had a greater chance to be diagnosed compared to natural ones. In this study, we used teeth with natural caries and because of random sampling, distribution of samples in the two groups of enamel and dentin caries was not similar (95 samples with dentin caries and 25 samples with enamel caries). In the current study, we tried to design the software with the help of MATLAB software. After designing, its function was evaluated and it was revealed that the designed software was able to diagnose 60% of enamel and 97% of dentin caries.
The ICC between the software and histological analysis results was 0.609 for enamel and 0.937 for dentin caries. Thus, we may conclude that the software is more successful in detecting the dentin caries. Also, it is capable of successfully determining the depth of dentin and even enamel caries. These results were acceptable because the literature has shown that caries are not visible on radiographs until they penetrate more than one half the enamel thickness and enamel lesions are usually not visible until 30 - 40% of the lesion has become demineralized (4). That is why the actual depth of penetration of a carious lesion is actually deeper than it appears on the radiographs. Nevertheless, detecting early enamel caries may not be of clinical interest, since not all early enamel caries should be restored and many of these lesions are capable of being remineralized by proper preventive procedures(4). Sometimes, the overlapping in adjacent teeth in the radiographs is a serious problem in caries detection.
In our study, as explained before, the software gets an image with two or three teeth and uses a separation phase to separate the teeth from each other. This is done by summation on the intensities of the image in each column and columns with lower intensity sum are considered as candidates for the place of separation between two teeth. Therefore, in case of small to medium overlap between teeth, the summation remains small enough to indicate the place for separation between the two teeth and the software works properly, although the software accuracy in these cases may decrease. We should note that in usual condition, if a radiograph shows teeth overlapped (that is because of incorrect horizontal angle of X-ray in capturing phase of radiographs from patients), dentist or radiologist diagnosis for caries detection is not reliable and usually the radiographs need to be repeated. So our software is not able to omit the technical errors in taking radiographs.
The results in this study were in accordance with those of Wenzel et al. (13). They compared diagnostic accuracy of Logicon Caries Detector program with that of human observers in approximal caries. They showed that when deeper lesions are the main concern, Logicon can help in diagnosis. In a study by Behere and Lele, the overall accuracy of Logicon Caries Detector program for enamel and dentin caries were 79% and 96%, respectively. However, Logicon appears to be more reliable in ruling out carious lesions than in detecting them whether in the enamel or in dentin (17). In another study, Wenzel et al. (18) found mean kappa value for inter-observer agreement for caries scores to be 0.47 before and 0.48 after the use of LCD, which did not improve using the program. They also found that the program was not consistent and provided diverse opinions on the caries status.
Our findings were also in agreement with those of Gakenheimer et al. (8) They believe that Logicon helps in automatically diagnosis of dentin caries. Dentists’ accuracy improved from 75.6 percent before using the software to 88.3 percent afterward. Tracy et al. (19) showed that nowadays with upgraded CAD software in Logicon caries detectors, it is possible to find twice as much early dentine carious lesions requiring restoration than before, while not needlessly restoring healthy teeth. In their study, the sensitivity was 30% with the initial image and 69% with the density analysis tool. However, the specificity was found to be 97% initially and 94% when the density analysis tool was used (19).
Forner Navarro et al. (20) and Araki et al. (21) reported similar results as well. Forner Navarro et al. found that the Logicon program increased sensitivity, especially in lesions with dentinal caries (20). A similar report by Araki et al. indicates that when cases were restricted to caries in the inner half of the enamel or to dentinal caries, observations with Logicon Caries Detector performed significantly better than without it (21). As mentioned earlier, we used histological analysis results as the gold standard in our study, which is similar to what was done by some researchers in Khon Kean University in Thailand (22). However, they used different statistical indices, which makes it difficult to compare the results. The software designed by researchers in Thailand had a poor function in measuring the depth of lesions, which may be due to the limitations of their Neural Network method for designing.
The diagnostic accuracy of our new designed software is going to be compared with that of human observers and the results will be reported in the near future.The designed software was able to detect a significant number of dentin caries and acceptable measuring the depth of carious lesions in enamel and dentin when compared to the results of histological analysis. However, the software had limited ability in detecting enamel lesions.
References
-
1.
Roberson TM, Lundeen TF. Cariology: the lesion, etiology, prevention and Control. Art & Science of operative dentistry. 5th ed. St louis: Mosby; 2007. p. 65-131.
-
2.
Farman T, Gakenheimer D, Lempicki A, Miller S, Shafie A, editors. Computer- aided maxillofacial radiographic diagnosis impact of congress Series. 2003. p. 1212-8.
-
3.
Abesi F, Mirshekar A, Moudi E, Seyedmajidi M, Haghanifar S, Haghighat N, et al. Diagnostic accuracy of digital and conventional radiography in the detection of non-cavitated approximal dental caries. Iran J Radiol. 2012;9(1):17-21. [PubMed ID: 23329955]. https://doi.org/10.5812/iranjradiol.6747.
-
4.
Krick R, Muhe Y, Prick T, Bredschneider M, Bremer S, Wenzel D, et al. Piecemeal microautophagy of the nucleus: genetic and morphological traits. Autophagy. 2009;5(2):270-2. [PubMed ID: 19182523].
-
5.
White SC, Hollender L, Gratt BM. Comparison of xeroradiographs and film for detection of proximal surface caries. J Am Dent Assoc. 1984;108(5):755-9. [PubMed ID: 6588117].
-
6.
Lith A, Lindstrand C, Grondahl HG. Caries development in a young population managed by a restrictive attitude to radiography and operative intervention: I. A study at the patient level. Dentomaxillofac Radiol. 2002;31(4):224-31. [PubMed ID: 12087439]. https://doi.org/10.1038/sj.dmfr.4600710.
-
7.
Kay EJ, Nuttall NM, Knill-Jones R. Restorative treatment thresholds and agreement in treatment decision-making. Community Dent Oral Epidemiol. 1992;20(5):265-8. [PubMed ID: 1424545].
-
8.
Gakenheimer DC. The efficacy of a computerized caries detector in intraoral digital radiography. J Am Dent Assoc. 2002;133(7):883-90. [PubMed ID: 12148682].
-
9.
Langland OE, Langlais RP. Radiologic diagnosis of caries. In: langland OE, Langlais RP, editors. Principles of dental imaging. Baltimore: Williams & wilkins; 1997. p. 295-412.
-
10.
National Institutes of H. Diagnosis and management of dental caries throughout life. NIH Consens Statement. 2001;18(1):1-23. [PubMed ID: 11699634].
-
11.
Akgun OM, Guven Polat G, TuranIllca A, Yildirim C, Demir P, Basak F. Does Magnetic Resonance Imaging Affect the Microleakage of Amalgam Restorations? Iran J Radiol. 2014;11(3). ee15565. [PubMed ID: 11699634].
-
12.
Duncan RC, Heaven T, Weems RA, Firestone AR, Greer DF, Patel JR. Using computers to diagnose and plan treatment of approximal caries. Detected in radiographs. J Am Dent Assoc. 1995;126(7):873-82. [PubMed ID: 7629363].
-
13.
Wenzel A, Hintze H, Kold LM, Kold S. Accuracy of computer-automated caries detection in digital radiographs compared with human observers. Eur J Oral Sci. 2002;110(3):199-203. [PubMed ID: 12120704].
-
14.
Jacobsen JH, Hansen B, Wenzel A, Hintze H. Relationship between histological and radiographic caries lesion depth measured in images from four digital radiography systems. Caries Res. 2004;38(1):34-8. [PubMed ID: 14684975]. https://doi.org/10.1159/000073918.
-
15.
Wenzel A. Current trends in radiographic caries imaging. Oral Surg Oral Med Oral Pathol Oral Radiol Endod. 1995;80(5):527-39. [PubMed ID: 8556463].
-
16.
Wenzel A. Digital radiography and caries diagnosis. Dentomaxillofac Radiol. 1998;27(1):3-11. [PubMed ID: 9482015]. https://doi.org/10.1038/sj.dmfr.4600321.
-
17.
Behere RR, Lele SM. Reliability of Logicon caries detector in the detection and depth assessment of dental caries: an in-vitro study. Indian J Dent Res. 2011;22(2):362. [PubMed ID: 21891917]. https://doi.org/10.4103/0970-9290.84277.
-
18.
Wenzel A. Computer-automated caries detection in digital bitewings: consistency of a program and its influence on observer agreement. Caries Res. 2001;35(1):12-20. [PubMed ID: 11125191].
-
19.
Tracy KD, Dykstra BA, Gakenheimer DC, Scheetz JP, Lacina S, Scarfe WC, et al. Utility and effectiveness of computer-aided diagnosis of dental caries. Gen Dent. 2011;59(2):136-44. [PubMed ID: 21903524].
-
20.
Forner Navarro L, Llena Puy MC, Garcia Godoy F. Diagnostic performance of radiovisiography in combination with a diagnosis assisting program versus conventional radiography and radiovisiography in basic mode and with magnification. Med Oral Patol Oral Cir Bucal. 2008;13(4):E261-5. [PubMed ID: 18379453].
-
21.
Araki K, Matsuda Y, Seki K, Okano T. Effect of computer assistance on observer performance of approximal caries diagnosis using intraoral digital radiography. Clin Oral Investig. 2010;14(3):319-25.
-
22.
Suwadee K, Sanphet S, Plermkamon S, Bureerat S, Chetchotsak D. An Artificial Neural Network for Detection of Simulated Dental Caries. Int J Comput Assist Radiol Surg. 2006;1(2):91-6.