Abstract
Background:
Distinguishing low-grade from high-grade gliomas can aid in optimal treatment planning and prognostication. Diffusion-weighted imaging (DWI) and magnetic resonance spectroscopy (MRS) have been applied in several studies for non-invasive glioma grading. However, these studies focused on limited aspects of these imaging techniques and used different study setups, resulting in occasionally inconsistent and incomparable conclusions in the literature.Objectives:
This study was designed to introduce the optimal imaging setup and the most reliable and applicable imaging parameters in glioma grading, using DWI and MRS.Patients and Methods:
During this prospective study, using a 3T-MR scanner, 55 glioma patients underwent brain MRS with short, intermediate, and long echo times (TEs), as well as DWI using low, intermediate, and high b-values. Postoperatively, all of the specimens were graded pathologically using light microscopy.Results:
We found that Max (Chol/Cr)/ Min (NAA/Cr) ((maximum choline to creatine ratio)/(minimum N-acetyl aspartate to creatine ratio)), followed by Max (Chol/ Cr), both in long-TE, were the most reliable metabolite ratios on MRS for accurate glioma grading. These had values for area under the curve (AUC) of 0.92 (P < 0.05) and 0.89 (P = 0.001), respectively, compared to conventional MR imaging (cMRI), which had an AUC of 0.83 (P < 0.05). DWI at maximal accuracy showed an AUC of 0.80 (P < 0.05).Conclusion:
Max (Chol/Cr)/Min (NAA/Cr) in long-TE was the most reliable of all of the MRS parameters studied, while DWI showed no superiority over cMRI in glioma grading. No significant differences existed among the various b-values applied, or between the minimum and mean tumor apparent diffusion coefficient values used in DWI-based glioma grading.Keywords
Apparent Diffusion Coefficient Brain Tumor Diffusion-Weighted Imaging Glioma Grading Glioma Magnetic Resonance Spectroscopy
1. Background
In addition to the differences in prognostic issues between low- and high-grade gliomas, therapeutic approaches to high-grade gliomas include various combinations of surgery, radiotherapy, and chemotherapy, whereas generally more conservative approaches are considered for the treatment of low-grade gliomas (1, 2). Although conventional magnetic resonance imaging (cMRI) has a well-established role in the diagnosis and morphological assessment of brain gliomas, accurate tumor grading and the real infiltrative tumor boundaries in the surrounding brain parenchyma cannot be well-elucidated (3). Developments in magnetic resonance imaging (MRI) have led to the development of techniques to compensate for the low accuracy of cMRI. Of these techniques, diffusion-weighted imaging (DWI), magnetic resonance spectroscopy (MRS), and perfusion-weighted imaging are the most studied and promising in the literature (4-7).
DWI and MRS have been applied in several studies for non-invasive glioma grading. However, each study focused only on limited aspects of these imaging techniques in tumor grading, and they used different study setups, resulting in occasionally inconsistent conclusions in the literature.
2. Objectives
This research studies the efficacy of MRS- and DWI-based glioma grading in comparison to cMRI, and investigates all possible effective imaging parameters and various combinations thereof (true multimodal imaging) in an attempt to introduce more reliable parameters in glioma grading using MRS and DWI techniques. The study also evaluates the results of various echo times in MRS, and of various b-values in diffusion-based studies, in order to achieve the optimal imaging setup for non-invasive tumor grading.
3. Patients and Methods
3.1. Patient Population
Fifty-six patients with a diagnosis of brain glioma based on cMRI, who were candidates for craniotomy and tumor debulking or removal, were consecutively enrolled over the 36 months of this cross-sectional study from April 2010 to April 2013. The participants included 34 males and 22 females, with a mean age of 41 years (range 17 - 67 years). The study was approved by the ethics committee of our hospital and Tehran University of Medical Sciences, and informed consent was obtained from each patient. Considering the sample size formula and previous studies’ statistics on non-invasive grading of brain gliomas using MR techniques, a patient population of approximately 50 was needed. Infratentorial tumors, or supratentorial tumors in close vicinity to the bony skull base, were excluded from the study because of intolerable signal artifacts appearing on DWI and MRS images. Completely cystic lesions were also excluded. Children were not included because of relatively different brain MRS and DWI signal characteristics. Only patients who were candidates for tumor resection via craniotomy were included, in order to minimize the pathologic errors in tumor grade assessment that are generated by the heterogeneous nature of glial tumors. One patient with a postoperative pathology report of metastatic adenocarcinoma was retrospectively excluded from the study. A total of 55 cases of newly diagnosed brain gliomas that had never undergone any mode of brain tumor treatment were finally included in the study.
3.2. Conventional MRI
MR imaging was performed on a 3 Tesla MR scanner (Siemens MAGNETOM Tim Trio), using a phased-array head coil, flip angle = 90°, matrix size = 256 × 256 pixels, and field of view (FOV) = 140 × 140 cm2, which covered the tumorous region and the normal-appearing contralateral brain hemisphere, as described below.
1) Axial, coronal, and sagittal T2-weighted fast-spin echo, TR (repetition time)/TE (echo time) = 4500/90 msec.
2) Axial fluid-attenuated inversion recovery (FLAIR), TR/TE/TI (inversion time) = 9800/110/2200 msec.
3) Pre- and post-contrast axial T1-weighted spin echo (SE), TR/TE = 650/9 msec.
Tumor grading based on cMRI was accomplished by two experienced neuroradiologists. Features such as significant mass effect, substantial contrast enhancement or signal heterogeneity in the tumorous regions, irregular margins, lower solid-tumor T2-weighted image signal intensity, and the presence of necrotic portions or significant peritumoral edema all indicated a high-grade glioma.
3.3. MR Spectroscopy
3.3.1. Data Acquisition
MRS experiments were performed using the point-resolved spectroscopy (PRESS) pulse sequence, acquired in different TE values of 30, 140, and 270 msec, which represented short, intermediate, and long TEs, respectively. The parameters were FOV (field of view) = 176 × 176 mm2, voxel size = 11 × 11 × 15 mm3, TR = 1500 msec, flip angle = 90°, sampling interval = 0.833 msec, number of data points = 1024, and bandwidth = 1200 Hz. Multi-voxel two dimensional (2D) proton chemical-shift imaging (CSI) was obtained before contrast material administration to avoid the probable theoretical inaccuracies that would occur. The CSI grid was placed on the anatomical MR image (axial T2-weighted image) based on the morphological features of the lesion, and was extended to include the contralateral normal side of the brain. An extra single-voxel acquisition, located at the contralateral normal centrum semiovale, was performed to provide phase information with the eddy-current compensation (ECC) procedure. The chemical-shift selective (CHESS) technique was employed to suppress the water signal. Out-of-volume saturation bands were applied to suppress signals excited outside the FOV. No lipid suppression was applied. Manual shimming was performed.
3.3.2. Pre-Processing
Preliminary modifications in the time-domain and the employment of in-house quantification software (8) were applied to the raw data to achieve the best quantification performance as follows (9): 1) SNR (signal to noise ratio) enhancement; 2) ECC; and 3) residual water removal.
3.3.3. Quantification
The quantification procedure of the 1H-MRS imaging data was performed in the time-domain employing the subtract-QUEST algorithm (10), to simultaneously quantify the metabolites and to estimate the baseline signal generated with macromolecules (11). The quantification results for each metabolite were analyzed for the associated optimal TE, with metabolite peaks as follows: choline (Chol) at 3.25 ppm (parts per million); N-acetyl aspartate (NAA) at 2.02 ppm; and creatine (Cr) at 3.02 ppm.
3.4. Diffusion-Weighted Imaging
3.4.1. Data Acquisition
DW images were acquired with the gradient echo echo-planar imaging (GE-EPI) pulse sequence; TE/TR = 137/4300 msec; flip angle = 90°; number of averages = 2; image matrix = 192 × 192 pixels; FOV = 22 × 22 cm2; number of slices = 21; slice thickness = 5 mm; inter-slice gap = 0.5 mm; voxel size 1.15 × 1.15 × 5 mm3; and employing b-values of 50, 500, 1000, and 3000 sec/mm2 in the axial plane before the injection of the contrast agent. The images were acquired in three orthogonal directions and combined into a single image, resulting in three apparent diffusion coefficient (ADC) maps using three b-values of 500 (low), 1000 (intermediate), and 3000 (high) DW images, referred to as ADC500, ADC1000, and ADC3000, respectively.
3.4.2. Quantification
MR images for the ADC500, ADC1000, and ADC3000 maps (× 10-3 mm2/sec) were co-registered to compensate for rigid-body motion existing between the slices of each map and among the three maps, using an in-house software program developed in MATLAB 7.14 (MathWorks Inc., Natick, MA). Solid tumor and normal contralateral white and gray matter from one or more slices containing the largest cross-section of the brain tumor were sampled by two expert neuroradiologists on the ADC1000 map. The coordinates were transferred to the program to sample the corresponding regions on the ADC500 and ADC3000 maps, considering the voxel-by-voxel alignment of these maps. The intensity values for each region on each map were then exported for statistical analysis.
3.5. Histopathological Evaluation
Due to the heterogeneous nature of gliomas, tissue-sampling errors seem to be the main cause of occasional incorrect pathology reports of glial tumor grades postoperatively. To enhance our research reliability, we decided not to include patients whose brain tumor tissue specimens were derived from biopsies. After total or near-total resection of the tumors via craniotomy, the specimens were evaluated by an experienced neuropathologist under light microscopy, and tumor grading was performed according to the world health organization (WHO) criteria for brain tumor classification (12). Of the 55 patients in this study, one (2%) proved to have a pilocytic astrocytoma (grade 1), 22 (40%) had grade 2 gliomas, 10 (18%) had grade 3 gliomas, and 22 (40%) had grade 4 gliomas. Grades 1 and 2 were designated as low-grade tumors, while grades 3 and 4 were high-grade.
3.6. Statistical Analysis
After checking for normal distribution using the Kolmogorov-Smirnov test, the Mann-Whitney U test was used to evaluate differences between the WHO subgroups. P-values of less than 0.05 were considered statistically significant. Receiver operating characteristic (ROC) curve analyses were performed to define and compare the diagnostic values of various metabolite ratios. Using the ROC curve analyses, we defined the threshold values for imaging parameters of the present study that had met statistical significance in glioma grading. The corresponding sensitivity, specificity, positive predictive value (PPV), negative predictive value (NPV), and accuracy were also determined for each cut-off value. Statistical analyses were performed using SPSS software ver. 16 (SPSS Inc., Chicago, Il, USA) and STATA software ver. 11 (StataCorp. 2009. Stata Statistical Software: Release 11. College Station, TX: StataCorp LP.).
4. Results
4.1. cMRI
Pre-operative cMRI could correctly predict the pathologically proven tumor grade in only 69% of patients. Of the pathologically proven grade 2 tumors, cMRI falsely diagnosed 19% and 14% as grade 3 and grade 4, respectively, and of the grade 3 tumors, cMRI misdiagnosed 44% and 33% as grade 2 and grade 4, respectively. Only 5% of grade 4 tumors were falsely diagnosed as grade 2.
4.2. MR Spectroscopy
4.2.1. Normal Side of the Brain Metabolite Ratios
Table 1 presents the Chol/Cr, Chol/NAA, and NAA/Cr values, quantified in the contralateral normal-appearing white matter.
Tumor Mean Metabolite Ratios per Pathologic Tumor Grades and for Normal Contralateral White Matter
Metabolic Ratio | Tumor Grade | TE30 | TE140 | TE270 | |||
---|---|---|---|---|---|---|---|
Mean (SD) | Sig | Mean (SD) | Sig | Mean (SD) | Sig | ||
Chol/Cr | 1 | 0.42 (0.08) | < 0.001 | 0.37 (0.32) | < 0.001 | - | < 0.001 |
2 | 0.43 (0.27) | 0.86 (0.57) | 0.71 (0.33) | ||||
3 | 0.79 (0.63) | 1.04 (0.54) | 0.97 (0.43) | ||||
4 | 1.09 (0.48) | 1.52 (0.62) | 1.44 (0.60) | ||||
Normal | 0.24 (0.12) | 0.38 (0.15) | 0.45 (0.12) | ||||
Chol/NAA | 1 | - | < 0.001 | 0.43 (0.49) | < 0.05 | - | < 0.001 |
2 | 0.80 (0.65) | 1.04 (1.20) | 0.62 (0.51) | ||||
3 | 1.37 (1.80) | 1.47 (1.88) | 0.62 (0.63) | ||||
4 | 1.27 (0.74) | 1.78 (1.89) | 1.53 (1.10) | ||||
Normal | 0.25 (0.11) | 0.17 (0.05) | 0.12 (0.04) | ||||
NAA/Cr | 1 | - | < 0.001 | 1.23 (0.66) | < 0.001 | - | < 0.001 |
2 | 1.18 (0.46) | 1.68 (1.31) | 2.60 (1.33) | ||||
3 | 0.55 (0.43) | 1.52 (1.46) | 1.76 (0.60) | ||||
4 | 0.75 (0.83) | 0.74 (0.38) | 1.02 (0.53) | ||||
Normal | 1.36 (1.67) | 2.28 (0.91) | 4.13 (1.07) |
4.2.2. Metabolite Ratios in Various Glioma Grades Acquired With Different TEs
Table 1 presents the three ratios, Cho/Cr, Cho/NAA, and NAA/Cr, in three different TEs, showing a higher mean Chol/Cr ratio in the intermediate TE compared to the short and long TEs, and significant elevation of both the Chol/Cr and the Chol/NAA ratios with the tumor grade. In contrast, the NAA/Cr ratio had the highest values in long TE compared to short and intermediate TEs, showing an inverse relationship with tumor grade.
Table 2 presents maximum (max) (Chol/Cr), max (Chol/NAA), and minimum (min) (NAA/Cr) in various tumor grades, showing that max (Chol/Cr) was significantly elevated in higher grades in all three applied TEs. However, the min (NAA/Cr) differences between the different tumor grades were statistically significant only in long TE.
Solid-Tumor Mean Metabolite Ratios for Various Tumor Grades
Metabolic Ratio | Tumor Grade | TE30 | TE140 | TE270 | |||
---|---|---|---|---|---|---|---|
Mean (SD) | Sig | Mean (SD) | Sig | Mean (SD) | Sig | ||
Max (Chol/Cr) | 1 | 0.48 (-) | < 0.05 | 1.85 (-) | < 0.05 | - | < 0.001 |
2 | 0.76 (1.02) | 1.29 (0.58) | 0.77 (0.34) | ||||
3 | 1.10 (0.90) | 1.30 (0.69) | 1.16 (0.37) | ||||
4 | 1.65 (0.61) | 2.59 (1.10) | 1.97 (0.46) | ||||
Max (Chol/NAA) | 1 | - | < 0.001 | 0.79 (-) | 0.27 | - | < 0.05 |
2 | 0.91 (0.38) | 1.89 (2.13) | 0.82 (0.46) | ||||
3 | 1.10 (0.59) | 3.47 (3.74) | 1.29 (1.07) | ||||
4 | 2.18 (0.91) | 3.87 (3.41) | 2.79 (1.88) | ||||
Min (NAA/Cr) | 1 | - | 0.45 | 0.76 (-) | 0.1 | - | < 0.001 |
2 | 0.63 (0.55) | 1.22 (1.01) | 1.89 (0.46) | ||||
3 | 0.37 (0.35) | 0.84 (0.61) | 1.25 (0.56) | ||||
4 | 0.56 (0.36) | 0.53 (0.42) | 0.66 (0.39) |
4.2.3. Developing Metabolic Ratio Cut-Off Values for Clinical Applications
Due to the numerous metabolites under study, a long list of statistically significant variables for glioma grading was prepared for the ROC curve analysis. Table 3 presents the most reliable cut-off points derived from the analyses with an area under the curve (AUC) of ≥ 0.800.
The Most Reliable Single (Non-Combined) MRS and DWI Parameters and Their Respective Most Reliable Thresholds in Distinguishing High- From Low-Grade Gliomas
Ratio | Cut-off | Sensitivity | Specificity | PPV | NPV | AUC | sig |
---|---|---|---|---|---|---|---|
Max (Chol/Cr) at TE = 270 | 1.15 | 86 | 90 | 92 | 82 | 0.89 | 0.001 |
Min (NAA/Cr) at TE = 270 | 1.36 | 79 | 90 | 92 | 75 | 0.88 | < 0.05 |
Min (ADC) at b = 1000 | 0.83 | 72 | 86 | 88 | 69 | 0.80 | < 0.05 |
Mean (ADC) at b = 3000 | 0.85 | 69 | 81 | 82 | 68 | 0.80 | < 0.05 |
Min (ADC) at b = 500 | 0.95 | 73 | 75 | 79 | 68 | 0.80 | < 0.05 |
Highly reliable thresholds could not be determined to effectively separate grade 2 and 3 tumors. The best cut-off value to achieve this goal was 0.90 for Chol/Cr in long TE with PPV, NPV, and AUC of 61%, 72%, and 0.67, respectively.
4.3. DWI
4.3.1. ADC Values for the Normal side of Brain
Mean ADC values (× 10-3 mm2/sec) for normal brain gray and white matter are summarized in Table 4.
Mean and Minimum ADC Values of Solid Tumors for the Various Grades of Gliomas
Tumor Grade | b = 500 | b = 1000 | b = 3000 | ||||
---|---|---|---|---|---|---|---|
Mean (SD) | Sig | Mean (SD) | Sig | Mean (SD) | Sig | ||
Mean ADC value | 1 | 1.90 (0.99) | < 0.05 | 1.89 (1.05) | < 0.001 | 1.06 (0.26) | < 0.001 |
2 | 1.61 (0.40) | 1.56 (0.42) | 1.02 (0.22) | ||||
3 | 1.46 (0.48) | 1.35 (0.43) | 0.89 (0.17) | ||||
4 | 1.12 (0.34) | 1.04 (0.31) | 0.72 (0.14) | ||||
Normal GM | 0.72 (0.11) | 0.73 (0.15) | 0.53 (0.06) | ||||
Normal WM | 0.82 (0.11) | 0.75 (0.15) | 0.47 (0.07) | ||||
Min ADC value | 1 | 1.65 (0.91) | < 0.05 | 1.59 (0.89) | < 0.05 | 0.94 (0.23) | < 0.05 |
2 | 1.24 (0.45) | 1.20 (0.48) | 0.79 (0.27) | ||||
3 | 1.10 (0.53) | 1.02 (0.46) | 0.65 (0.20) | ||||
4 | 0.71 (0.23) | 0.68 (0.19) | 0.51 (0.13) |
4.3.2. ADC Values in Various Glioma Grades Using Different b Values
Table 4 presents the minimum and mean tumor ADC values for three different b-values, resulting in a statistically significant inverse relationship of the ADC values with tumor grading (P < 0.05).
4.3.3. Developing ADC Value Thresholds for Clinical Applications
The mean and minimum ADC values showed almost similar AUCs (around 0.800) for various b-values. Table 4 presents DWI thresholds with AUC ≥0.800 in grading of gliomas (high-grade versus low-grade). No DWI parameter could effectively distinguish grade 2 from 3 with high sensitivity and specificity.
4.4. Combining DWI and MRS Parameters: True Multimodal Parameters in Glioma Grading
In an attempt to achieve higher sensitivity, specificity, and accuracy, we combined the most reliable resulting parameters of MRS and DWI in glioma grading in different ways, which resulted in no significant improvements in sensitivity, specificity, and accuracy.
The most powerful of these new parameters are as follows:
-Max (Chol/Cr) at TE = 30/min (ADC) at b = 3000, with a cut-off point of 1.14, could distinguish high- from low-grade tumors with a sensitivity of 82% and specificity of 88% (AUC = 0.842, 95% confidence interval [CI] = 0.79 - 0.93, P < 0.001).
-Max (Chol/Cr) at TE = 270/mean(ADC) at b = 3000, with a cut-off point of 0.84, resulted in 92% sensitivity and 70% specificity for distinguishing high- from low-grade gliomas (AUC = 0.842, CI = 0.80 - 0.91, P = 0.007).
Combining certain relevant variables of MRS resulted in a more brilliant parameter. Max (Chol/Cr)/min (NAA/Cr) at TE = 270 had the best AUC of 0.92 (CI = 0.82 - 1), which showed a sensitivity of 100% and specificity of 50% for the threshold of 0.41. The sensitivity, specificity, PPV, and NPV were 86%, 91%, 93%, and 83%, respectively, for the threshold of 0.69. There was a sensitivity of 64% and specificity of 100% for the threshold of 1.10.
5. Discussion
A diagnostic accuracy of 55%–83.3% has been reported for glioma grading using cMRI (5, 7, 13). A diagnostic accuracy of 62%, sensitivity of 72%, and specificity of 48% has been reported in a recent study on contrast-enhanced MRI in differentiating between high- and low-grade gliomas (14, 15). Using the Spearman correlation analysis, a correlation coefficient of 0.72 was obtained between the grading with cMRI and the gold standard pathologic grading. A higher sensitivity of 83% and a specificity of 69% with cMRI in distinguishing high- from low-grade gliomas compared to most previous studies were observed (PPV=78% and NPV=76%), but grading using cMRI was still apparently less reliable than MRS (Figure 1).
This 22-year-old female was admitted due to recent seizure attacks and a relatively homogeneous non-enhancing intra-axial lesion in the right medial frontal lobe, with no obvious peritumoral edema and almost no mass effect, suggesting a low-grade glioma based on cMRI (A, T2WI; B, T1WI + contrast). However, a high-grade glioma with a high focal max(Chol/Cr) ratio (mean max[Chol/Cr] in the tumoral region of 2.11, compatible with a high-grade glioma) was observed on CSI-MRS (superimposed metabolite graphic map) with TE = 270 msec (C). The tumor was pathologically proven to be a glioblastoma multiforme.
![This 22-year-old female was admitted due to recent seizure attacks and a relatively homogeneous non-enhancing intra-axial lesion in the right medial frontal lobe, with no obvious peritumoral edema and almost no mass effect, suggesting a low-grade glioma based on cMRI (A, T2WI; B, T1WI + contrast). However, a high-grade glioma with a high focal max(Chol/Cr) ratio (mean max[Chol/Cr] in the tumoral region of 2.11, compatible with a high-grade glioma) was observed on CSI-MRS (superimposed metabolite graphic map) with TE = 270 msec (C). The tumor was pathologically proven to be a glioblastoma multiforme. This 22-year-old female was admitted due to recent seizure attacks and a relatively homogeneous non-enhancing intra-axial lesion in the right medial frontal lobe, with no obvious peritumoral edema and almost no mass effect, suggesting a low-grade glioma based on cMRI (A, T2WI; B, T1WI + contrast). However, a high-grade glioma with a high focal max(Chol/Cr) ratio (mean max[Chol/Cr] in the tumoral region of 2.11, compatible with a high-grade glioma) was observed on CSI-MRS (superimposed metabolite graphic map) with TE = 270 msec (C). The tumor was pathologically proven to be a glioblastoma multiforme.](http://services.brieflands.com/cdn/serve/313eb/93ec4e3ee23d4f9b91d86d2522317c20bb22e0e0/iranjradiol-In_Press-In_Press-17817-i001-preview.png)
Chol/Cr shows its highest values in intermediate TE and lowest values in short TE, whereas NAA/Cr is highest in long TE and lowest in short TE. These changes reflect the higher T2 relaxation time of Chol, and especially of NAA, relative to Cr. It is noteworthy that NAA has the longest T2 relaxation time among brain metabolites, as the T2 relaxation times for NAA, Chol, and Cr on 3T were 262 ± 37, 170 ± 18, and 151 ± 15 msec, respectively (15).
The signal contamination on NAA from the neighboring metabolites (9, 10), as well as the macromolecules’ baseline signal in short TE (16), causes underestimation of NAA and therefore higher NAA/Cr values in longer TEs, which are intensified with the high T2 relaxation time of NAA relative to Cr. Such a result in the quantification of NAA suggests relying on NAA/Cr quantification in long TEs, followed by intermediate TEs, where signals from the macromolecules’ baseline and other contaminating short-T2 metabolites have substantially subsided. This is also true when using NAA in combination with other metabolites in a ratio, such as Chol/NAA, which explains the statistically insignificant differences between high- and low-grade gliomas in a study from 2007 (17) that observed that the Chol/NAA ratio was quantified in short TE. The same concept holds for accurate Chol quantification that is hindered in short TE due to short-T2 metabolites and the macromolecules’ contaminating signals, resulting in reliable quantification of Chol/Cr in intermediate-to-long TEs.
Our findings confirm that Chol/Cr, particularly max (Chol/Cr), is the most viable metabolite ratio for accurate grading of gliomas, with a diagnostic accuracy of 70% for Chol/Cr in intermediate TE (and even higher for long TE), as well as 88% for max (Chol/Cr) in long TE. These results are in agreement with the results proposed by Senft et al. (14) in 2010 on 3T, with a diagnostic accuracy of 82.5% and 72.1% for max (Chol) and Chol/Cr, respectively, in intermediate TE, showing superior power for glioma grading based on the Chol content. However, our findings contrast with some other studies, such as one by Zonari et al. (18) in 2007 on 1.5T, emphasizing the role of the NAA/Cr ratio in glioma grading.
Figure 2 compares the ROC curves of cMRI and our most reliable metabolite ratio in glioma grading with the AUCs for cMRI and the MRS ratio of 0.83 and 0.92, respectively, proving that MRS (and particularly the new parameter) has a significant advantage over cMRI in glioma grading (P < 0.05).
Comparison of cMRI and our most reliable MRS metabolite ratio in glioma grading ROC curves
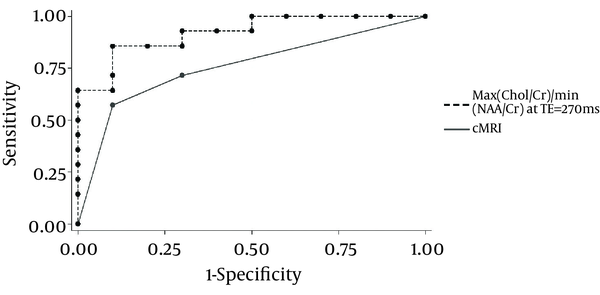
Comparisons of ADC values for normal brain gray and white matter in different b values (Table 3), which has been mostly ignored in the literature, revealed that imaging with lower diffusion sensitivities of b = 500 or 1000 provides relatively lower mean ADC values for gray matter. However, lower ADC values for white matter are observed by applying higher diffusion sensitivities of b = 3000. This difference is significant, both quantitatively and qualitatively.
Higher-grade gliomas showed consistently lower ADC values for all b-values, but using various b-values led to highly comparable results in glioma grading (P < 0.05). This is in contrast to the report of Alvarez et al. (19), who proposed that using a high b value (3000 in their study) is the most reliable for glioma grading.
It seems that using minimum ADC values in glioma grading (as reported by Lee et al. (20)) results in higher AUCs when compared to maximum or mean ADC values (although not statistically significant).
The parameter introduced by Murakami et al. (21) as the ADC difference value (the difference between minimum and maximum tumor ADC values) did not meet statistical significance in glioma grading at any of the applied b values.
Figure 3 compares the ROC curves for cMRI and DWI’s most reliable parameter in glioma grading. Considering the AUC of 0.83 (CI = 0.72 - 0.90) for cMRI (P = 0.08) and the AUC of 0.80 (CI = 0.70 - 0.86) for DWI (P = 0.035), despite the differences between these two AUCs not meeting statistical significance, it seems that DWI possesses the same power as cMRI in grading gliomas. Therefore, DWI does not show any advantage over cMRI in glioma grading.
Comparison of cMRI and DWI ROC curves in glioma grading
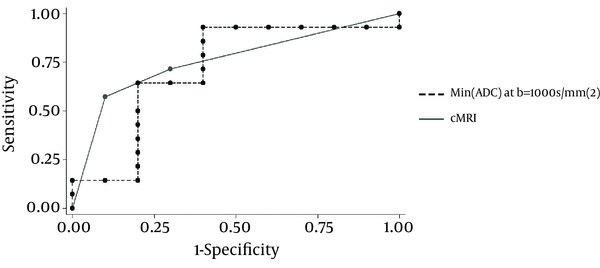
Acknowledgements
References
-
1.
Bauman G, Shaw EG. Low-grade glioma: current management and controversies. Clin Adv Hematol Oncol. 2003;1(9):546-53. [PubMed ID: 16258447].
-
2.
Nicholas MK. Glioblastoma multiforme: evidence-based approach to therapy. Expert Rev Anticancer Ther. 2007;7(12 Suppl):23-7. [PubMed ID: 18076314]. https://doi.org/10.1586/14737140.7.12s.S23.
-
3.
Di Costanzo A, Scarabino T, Trojsi F, Giannatempo GM, Popolizio T, Catapano D, et al. Multiparametric 3T MR approach to the assessment of cerebral gliomas: tumor extent and malignancy. Neuroradiology. 2006;48(9):622-31. [PubMed ID: 16752135]. https://doi.org/10.1007/s00234-006-0102-3.
-
4.
Sugahara T, Korogi Y, Kochi M, Ikushima I, Shigematu Y, Hirai T, et al. Usefulness of diffusion-weighted MRI with echo-planar technique in the evaluation of cellularity in gliomas. J Magn Reson Imaging. 1999;9(1):53-60. [PubMed ID: 10030650].
-
5.
Knopp EA, Cha S, Johnson G, Mazumdar A, Golfinos JG, Zagzag D, et al. Glial neoplasms: dynamic contrast-enhanced T2*-weighted MR imaging. Radiology. 1999;211(3):791-8. [PubMed ID: 10352608]. https://doi.org/10.1148/radiology.211.3.r99jn46791.
-
6.
Kaminogo M, Ishimaru H, Morikawa M, Ochi M, Ushijima R, Tani M, et al. Diagnostic potential of short echo time MR spectroscopy of gliomas with single-voxel and point-resolved spatially localised proton spectroscopy of brain. Neuroradiology. 2001;43(5):353-63. [PubMed ID: 11396738].
-
7.
Moller-Hartmann W, Herminghaus S, Krings T, Marquardt G, Lanfermann H, Pilatus U, et al. Clinical application of proton magnetic resonance spectroscopy in the diagnosis of intracranial mass lesions. Neuroradiology. 2002;44(5):371-81. [PubMed ID: 12012120]. https://doi.org/10.1007/s00234-001-0760-0.
-
8.
Madadi A, Mohseni M, Fathi Kazerooni A, Karimi Alavijeh S, Saligheh Rad H. Accurate Quantification of Metabolites’ Ratios in Glial Brain Tumors Employing 1H- MRSI at 3T. ESMRMB. Toulouse FR. 2013.
-
9.
Barker PB, Bizzi A, De Stefano N, Lin DDM. Clinical MR spectroscopy: techniques and applications. UK: Cambridge University Press; 2010.
-
10.
Ratiney H, Sdika M, Coenradie Y, Cavassila S, van Ormondt D, Graveron-Demilly D. Time-domain semi-parametric estimation based on a metabolite basis set. NMR Biomed. 2005;18(1):1-13. [PubMed ID: 15660450]. https://doi.org/10.1002/nbm.895.
-
11.
Sima DM, Rodriguez Diaz AM, Van Huffel S. Modeling the macromolecular background in Nuclear Magnetic Resonance spectroscopic signals. 4th European Conference of the International Federation for Medical and Biological Engineering. Springer; 2009. p. 372-6.
-
12.
Kleihues P, Louis DN, Scheithauer BW, Rorke LB, Reifenberger G, Burger PC, et al. The WHO Classification of Tumors of the Nervous System. J Neuropathol Experimental Neurol. 2002;61(3):215-25. https://doi.org/10.1093/jnen/61.3.215.
-
13.
Asari S, Makabe T, Katayama S, Itoh T, Tsuchida S, Ohmoto T. Assessment of the pathological grade of astrocytic gliomas using an MRI score. Neuroradiology. 1994;36(4):308-10. [PubMed ID: 8065577].
-
14.
Senft C, Hattingen E, Pilatus U, Franz K, Schanzer A, Lanfermann H, et al. Diagnostic value of proton magnetic resonance spectroscopy in the noninvasive grading of solid gliomas: comparison of maximum and mean choline values. Neurosurgery. 2009;65(5):908-13. [PubMed ID: 19834403]. https://doi.org/10.1227/01.NEU.0000356982.82378.BA.
-
15.
Xu D, Ozturk Isik E, Lupo JM, Chen AP, Vigneron DB, Nelson SJ, et al. T1 and T2 metabolite relaxation times in normal brain at 3T and 7T. J Molecular Imaging Dynamics. 2013;2012.
-
16.
Ratiney H, Coenradie Y, Cavassila S, van Ormondt D, Graveron-Demilly D. Time-domain quantitation of 1H short echo-time signals: background accommodation. MAGMA. 2004;16(6):284-96. [PubMed ID: 15168136]. https://doi.org/10.1007/s10334-004-0037-9.
-
17.
Aragao Mde F, Otaduy MC, Melo RV, Azevedo Filho HR, Victor EG, Silva JL, et al. [Multivoxel spectroscopy with short echo time: choline/N-acetyl-aspartate ratio and the grading of cerebral astrocytomas]. Arq Neuropsiquiatr. 2007;65(2A):286-94. [PubMed ID: 17607430].
-
18.
Zonari P, Baraldi P, Crisi G. Multimodal MRI in the characterization of glial neoplasms: the combined role of single-voxel MR spectroscopy, diffusion imaging and echo-planar perfusion imaging. Neuroradiology. 2007;49(10):795-803. [PubMed ID: 17619871]. https://doi.org/10.1007/s00234-007-0253-x.
-
19.
Alvarez-Linera J, Benito-Leon J, Escribano J, Rey G. Predicting the histopathological grade of cerebral gliomas using high b value MR DW imaging at 3-tesla. J Neuroimaging. 2008;18(3):276-81. [PubMed ID: 18482368]. https://doi.org/10.1111/j.1552-6569.2008.00263.x.
-
20.
Lee EJ, Lee SK, Agid R, Bae JM, Keller A, Terbrugge K. Preoperative grading of presumptive low-grade astrocytomas on MR imaging: diagnostic value of minimum apparent diffusion coefficient. AJNR Am J Neuroradiol. 2008;29(10):1872-7. [PubMed ID: 18719036]. https://doi.org/10.3174/ajnr.A1254.
-
21.
Murakami R, Hirai T, Sugahara T, Fukuoka H, Toya R, Nishimura S, et al. Grading astrocytic tumors by using apparent diffusion coefficient parameters: superiority of a one- versus two-parameter pilot method. Radiology. 2009;251(3):838-45. [PubMed ID: 19318585]. https://doi.org/10.1148/radiol.2513080899.