Abstract
Context:
Due to the chronic nature of cancer, screening programs were a set of sequential decisions taken over time. Markov decision process (MDP) and partially observable Markov decision process (POMDP) models were the mathematical tools applied in studies, including sequential decision-making such as screening protocols for medical decision-making.Objectives:
The main goal of this study was to investigate optimal policy for cancer screening using MDP and POMDP models.Methods:
We performed a review of articles published within July 2000 to November 2022 in PubMed, Web of Science, and Scopus databases. The stopping age, the type of optimal strategy, the benefits of the optimal policy, and the relationship between age and risk threshold were extracted. Studies that did not use MDPs and POMDPs as the mathematical maximization models in cancer screening, review articles, editorials or commentaries, non-English articles, and those that did not focus on optimization were excluded.Results:
From 532 articles, 6 studies met the study criteria. All studies suggested that the optimal policy was control-limit, and the cancer risk threshold was a non-decreasing function of age. Three studies specified a stopping age for cancer screening. In five studies, the optimal policies outperformed the guidelines or no screening strategy.Conclusions:
Two essential factors in screening decisions were cancer risk and age, which were individual variables. The control-limit policy included these factors in decision-making for cancer screening. These policies highlighted personalized screening and showed that this type of screening could outperform cancer screening guidelines regarding economic and clinical benefits.Keywords
Markov Decision Process Partially Observable Markov Decision Process Cancer Screening Diagnosis Early Detection
1. Context
Non-communicable diseases (NCDs) constitute a large part of global mortality in the 21st century (1). Cancer as an NCD is a leading cause of death that decreases life expectancy (2). Cancer cases and deaths should be 18 and 9.6 million in 2018, respectively (2). Researchers predict that 26 million new cancer cases and 17 million deaths annually will occur by 2030 (3). Growing older, population growth, and changes in factors related to socioeconomic development are some of the factors contributing to this growth (4, 5). Although cancer has significant mortality rates, if it is detected in a localized stage, it is likely to be curable (6). An example is that only 27% of women with distant breast cancer survive for 5 years, compared to 99% of women with localized breast cancer at an early stage (7).
Screening can help find cancers at an early stage when they are likely to be easier to treat. Therefore, researchers have done extensive work on technologies and tests that make cancer detection in asymptomatic patients better (8). There are different screening modalities, such as clinical breast exams, X-ray mammography, colonoscopy, and prostate-specific antigen (PSA), to detect prostate cancer (PC) (6). Screening using these tools has some harms and benefits. On the one hand, they have some potential risks, including radiation exposure, over-diagnosis, and false-positive that can lead to an unnecessary biopsy, which has a cost, pain, and the possibility of side effects. On the other hand, there are long-term benefits for cancer detection and treatment in the early stages (9, 10). Therefore, comparing the positive and negative effects of screening is crucial. A screening program is successful if it can attain maximum net benefits (11).
Recently, several guidelines have recommended individual-based screening for cancer detection (12, 13). They have considered some patient-level factors in screening (Table 1). Age and cancer risk are two important individual factors in these guidelines that change over time, and optimal screening decisions must be updated based on them to suggest a dynamic, personalized cancer screening policy (i.e., a policy that prescribes different screening intervals depending on the individuals' age and personalized risk of cancer). It might be preferable to a population-based screening strategy (14).
Cancer Screening Guidelines
Cancer | Ref | Guideline | Recommendations |
---|---|---|---|
Breast | (15, 16) | American Cancer Society (ACS) | (1) Every year for women aged 45 to 54; (2) women 55 years and older annually or biannually; (3) continuing screening mammography as long as overall health is good and life expectancy is 10 years or more. |
(17) | American College of Physicians (ACP) | (1) Women aged 40 to 49 should be screened based on the potential benefits and harms and a woman's preferences. The potential harms outweigh the benefits in most women aged 40 to 49 years; (2) women aged 50 to 74 years with biennial mammography; (3) screening for women aged 75 years or with a life expectancy of 10 years or less should be discontinued. | |
(18) | United States Preventive Services Task Force (USPSTF) | Biannual screening mammography for women aged 50 to 74 years | |
(19) | American College of Obstetricians and Gynecologists (ACOG) | (1) Starting from 40 and stopping at 75; (2) biannual screening mammograms after age 55 years; (3) age alone should not be the basis to continue or stop screening mammograms. For women older than age 75 years, the decision to discontinue screening mammograms should be made based on the woman's health status and life expectancy. | |
(20) | National Comprehensive Cancer Network (NCCN) | Annual for individuals 40 years and older | |
Prostate | (21, 22) | American Cancer Society (ACS) | The decisions should be made after getting information about the uncertainties, risks, and potential benefits of prostate cancer (PC) screening. |
(12) | American College of Physicians (ACP) | Starting from 50 and stopping at 69 or men with a life expectancy of less than 10 to 15 years. | |
(13) | United States Preventive Services Task Force (USPSTF) | (1) Individual-based prostate-specific antigen (PSA) screening for men aged 55 to 69 with respect to potential benefits and harms of screening; (2) stopping PSA screening for men aged 70 or older. | |
Colorectal | (22-24) | American Cancer Society (ACS) | (1) Screening should be done for average-risk individuals aged 45 to 75 years; (2) for individuals ages 76 through 85, the decision to be screened should be based on preferences, overall health, and prior screening history; (3) discontinuing for patients older than 85. |
(25) | American College of Physicians (ACP) | (1) Screening should be done for average-risk individuals aged 50 to 75; (2) colonoscopy every 10 years; (3) discontinuing screening in average-risk adults older than 75 years or in adults with a life expectancy of 10 years or less. | |
(11) | The United States Preventive Services Task Force (USPSTF) | (1) Starting at age 50 years; (2) colonoscopy screening every 10 years; (3) in adults ages 76 to 85 years, based on the patient's health status, harms and benefits of screening. |
When individuals grow older, life expectancy will decrease, and cancer detection will be less beneficial for older patients (26). Therefore, the cancer risk threshold at which a patient should be referred for biopsy or treatment increases (14, 27). It can be one of the essential issues that are not considered in many cancer screening guidelines and CEA studies, and screening decisions are made regardless of personal risk score (28).
Another issue is the chronic nature of cancer, which makes screening programs a set of sequential decisions that are taken over time. Clinicians are responsible for choosing the most beneficial decisions in cancer screening. These decisions are usually taken under uncertainty and over the lifetime horizon. Although they have the current screening test results, some uncertainties, including the unknown state of cancer in the future, the screening's side effects, and over-diagnosis, might arise. Cancer is a situation in a medical context in which a patient's physiological state is not directly observable, and the screening result can often be probabilistically related to the actual state. These problems have led to the need for more data in decision-making, and more complicated models are also required to consider these conditions (29).
There are some powerful tools in operations research (OR) that can be used to scrutinize these data to decide on screening options (30). Markov decision process (MDP) and partially observable Markov decision process (POMDP) models are mathematical tools that have been applied in sequential decision-making, such as screening protocols for medical decision-making (31). Markov decision process and POMDPs can use the outcomes of screening tests to find the type of optimal screening decisions (optimal policy), the relationship between cancer risk score, age, and screening decisions, and the maximum benefit of the optimal policy over no screening or guidelines (32).
2. Objectives
We performed a systematic review of articles published on MDPs and POMDPs in the context of cancer screening. The main objective of this study was to investigate optimal policy for cancer screening using MDPs and POMDPs to find the relationship between age and risk threshold and their effects on screening decisions.
3. Materials and Methods
A systematic search of literature databases was performed within July 2000 to November 2022. The search was limited to papers published in English, including PubMed, Web of Science, and Scopus. The search strategy was:
("Markov decision process" [Title/Abstract] OR "MDP" [Title/Abstract] OR "partially observable markov decision process" [Title/Abstract] OR "POMDP" [Title/Abstract]) AND ("screening" [Title/Abstract] OR "mass screening" [Title/Abstract] OR "early detection" [Title/Abstract] OR "diagnosis" [Title/Abstract] OR "biopsy" [Title/Abstract]) AND ("cancer" [Title/Abstract] OR "neoplasm" [Title/Abstract]) AND ((English [Filter]) AND ((English [Filter]) AND (2000:2022[pdat]))
3.1. Definitions
• States: The set of all situations that a system can occupy (30).
• Action: The set of all possibilities (i.e., a treatment, biopsy, or a screening test) that a decision-maker can take in a medical process (30).
• Policies: A policy denotes a sequence of decisions (taking action or another) over some time (30).
• Policy stationery: If the decision rule about taking action is constant for any epoch, it is called policy stationery (30).
• Control-limit type policy: It is a policy in which the decision rule is in the following form:
where
• Double control-limit type policy: It is a policy in which the decision rule is in the following form:
where
• Optimal policy: It is a policy that, through its system, can attain maximum total expected rewards (maximum quality-adjusted life-years [QALYs]) (30).
• Termination (optimal stopping): It is a decision epoch after which the system (screening) moves to state Ω (discontinuing), where it remains forever and receives reward zero (30).
• Risk threshold: At any age, there is a number such that if the probability of cancer is greater than the number, the patient should be referred for biopsy or treatment (14).
3.2. Inclusion and Exclusion Criteria
All English studies that investigated optimizing cancer screening benefits using MDP and POMDP models until November 30, 2022, were included. The present study did not include review articles, editorials, or commentaries. All inclusion and exclusion criteria were reported in Table 2.
Inclusion and Exclusion Criteria Applied for Selected Articles in the Study
Variables | Inclusion Criteria | Exclusion Criteria |
---|---|---|
Model type | MDP or POMDP | Not MDP and POMDP |
Approach type | Optimization | Not optimization |
Intervention type | Individual-based screening | Not individual-based screening |
Disease type | Cancer | Not cancer |
Time period | Articles published between July 2000 and November 2021 | Articles published before July 2000 or after November 20 |
3.3. Sifting Process
Sifting was conducted systematically in four different steps. After screening the papers' titles, abstracts were obtained and screened during the second sift. Subsequently, the full texts of the papers were studied, and papers that met the criteria were included in the final analyses.
3.4. Study Selection and Data Extraction
After the removal of duplicates, the remaining articles were screened based on title and abstract information by one author (NM). In the second step, the full text of the articles was given to two trained authors (MH and AN) to assess the inclusion. Each article was reviewed by two individuals independently. If authors had opposing opinions about an article, the article was judged by a third reviewer (NM). Three authors (MH, AN, and NM) extracted the data. The required parameters and characteristics of each study were described and extracted. These characteristics are summarized in Table 3.
Study Characteristics
Study (Y) | Country/ Perspective | Cancer Type | Model Type | Outcomes | Solving Approach | Strategies |
---|---|---|---|---|---|---|
Chhatwal et al. (2010) (27) | USA/ patient | Breast | MDP | Optimal age-dependent policy to perform biopsy or mammography; expected QALYs; true positive no; true negative no; false positive no; false negative no | Approximate dynamic programming | Mammography decision based on the optimal policy; mammography decision based on the radiologists’ interpretation |
Ayer et al. (2012) (32) | USA/ patient | Breast | POMDP | Optimal age-dependent policy to perform biopsy or mammography; expected QALYs; expected number of mammograms; expected false-positives; risk of developing undetected invasive cancer | Monahan’s algorithm with Eagle’s reduction | Mammography decision based on the optimal policy; no screening; annual mammography between 40 and 74 years; annual mammography between 40 and 79 years; annual mammography between 50 and 74 years; annual mammography between 50 and 79 years; biennial mammography between 40 and 74 years; biennial mammography between 40 and 79 years; biennial mammography between 50 and 74 years; biennial mammography between 50 and 79 years; biennial mammography between 50 and 69 years; biennial mammography between 45 and 69 years |
Zhang et al. (2012) (14) | USA/ patient | Prostate | POMDP | Optimal age-dependent policy to perform biopsy or PSA test; expected QALYs | Incremental pruning | PSA-based screening from the optimal policy using variable threshold; no screening; annual screening with a 4 ng/mL threshold |
Zhang et al. (2012) (33) | USA/ patient; societal | Prostate | POMDP | Optimal age-dependent policy to perform biopsy, PSA test, or do nothing; expected QALYs; costs of screening, biopsy, and treatment | Incremental pruning | PSA-based screening from the optimal policy using variable threshold; no screening; annual screening with a 4 ng/mL threshold |
Alagoz et al. (2013) (34) | USA/ patient | Breast | MDP | Optimal age-dependent policy to perform biopsy or mammography; expected QALYs; true positive rate; true negative rate; the number of annual mammograms; the number of follow-up mammograms; the number of biopsies | Approximate dynamic programming | Mammography decision based on the optimal policy; mammography decision based on the radiologists’ interpretation |
Erenay et al. (2014) (35) | USA/ patient | Colon | POMDP | Optimal age-dependent policy to perform colonoscopy or wait; expected TQALYs; expected number of colonoscopies per patient; lifetime CRC risk; CRC mortality; total costs | Monahan’s algorithm with Eagle’s reduction | Colonoscopy decisions based on the optimal policy; AGA screening recommendation (every 10 years); no screening |
As the purpose of the current review was to report the application of a mathematical approach in cancer screening optimization, two checklists were integrated to evaluate the quality of studies. The first was the ISPOR Constrained Optimization Task Force Good Practices Checklist (36). This checklist assessed the quality of the optimization approach in the studies. The checklist consisted of eight criteria, including problem structuring, mathematical formulation, model development, model validation, selection of optimization method, performing optimization/sensitivity analysis, reporting results, and decision making. Each criterion present in the articles took a score from 0 to 2. The second was a newly adapted tool for the assessment of modeling study. It included 14 criteria, including aims and objectives, setting and population, intervention/comparators, outcome measures, parameters, ranges and data sources, model structure and time horizon, modeling methods, parameters, assumptions explicit and justified, quality of data and uncertainty and/or sensitivity analyses, method of fitting, model validation, funding source and conflicts of interest, interpretation and discussion of results, and presentation of results and uncertainty. Several criteria were repeated in both checklists; therefore, the repeated criteria were deleted. Finally, the quality was assessed using 16 criteria after integration. The checklist contained the questions for each of the 16 criteria. If a criterion was not relevant for a particular paper, a score of one was assigned. The articles were scored 0 - 2 on each of the 16 criteria, giving a maximum score of 32 points. The checklist is available in the Appendix 1. This study considered articles with a total score of 16 to be of adequate quality.
3.5. Statistical Analysis
Outcomes from the different studies in the present systematic review cannot be combined. As a result, it was impossible to perform any meta-analysis, and all the results and characteristics of the individual studies are shown in a table or a descriptive form.
4. Results
4.1. Result of the Sifting Process
The present search in this systematic review retrieved 686 articles with 532 relevant titles after deleting duplicate articles. A total of 532 abstracts were read, and then, in the third step, 21 full texts were reviewed. Finally, in the fourth step of the sifting process, six full texts were of high quality and included in the review (Figure 1).
Sifting process of systematic review of studies published about optimal policies in cancer screening using MDP and POMDP models.
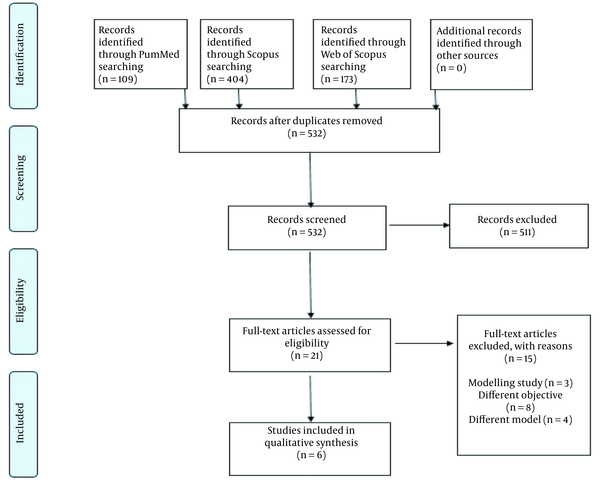
4.2. Articles Quality Assessment
The results of the quality assessment are shown in Appendix 1. This study assessed the quality of articles using the checklists. Quality assessment results showed that no article was given a perfect score. Two articles (Zhang et al. (14) and Erenay et al. (35)) obtained the highest score. Out of 32 possible points, they scored 29. Additionally, Chhatwal et al. (27) received the lowest quality score, obtaining only 22 points out of 32 from the quality assessment checklist.
All articles (n = 6, 100%) described the aims, objectives, mathematical formulation, and programming model in software and showed that the modeling method was appropriate for the study question. Three articles reported setting, perspective, and population characteristics completely. Half of the articles (n = 3) adequately discussed the intervention and comparators. None of the articles mentioned a conflict of interest among the authors. All articles (n = 6) described the model structure and time horizon clearly. Reporting all parameters, their sources, and the ranges was only done by Zhang et al. (14) and Erenay et al. (35). Half of the articles (n = 3) discussed the model fitting and calibration Method. All studies reported the results; however, only two articles (Zhang et al. (14) and Erenay et al. (35)) performed a comprehensive sensitivity analysis. More than half of the articles did not report the generalizability of their results to other settings (n = 4). Outcome measures were not completely described in one study (Chhatwal et al. (27)). In all articles, all assumptions were explicitly stated and justified. Half of the articles (n = 3) had an assessment of the validity of the results by comparing across one or more different model structures or against a validation data set.
4.3. Comparison of Optimal Screening Policy
Four studies (n = 4, 66%) suggested that the optimal screening policy is control-limit (Table 4). In these studies, decision alternatives (actions) were screening or referring to biopsy (14, 27, 32, 35). Two studies (n = 2, 34%) used screening, follow-up, and referral for biopsy as actions, and they showed a double control-limit optimal policy (6, 33).
Results of Optimal Policy for Different Cancer Screening Programs
Authors (Y) | Ref | Perspective | Optimal Policy | Termination | Risk Threshold | Benefit Over No Screening (QALY/Person) | Benefit Over Guidelines (QALY/Person) |
---|---|---|---|---|---|---|---|
Chhatwal et al. (2010) | (27) | Patient | Control-limit type. | - | The referral threshold on the probability of having cancer is non-decreasing as age increases. | - | - |
Ayer et al. (2012) | (32) | Patient | Control-limit type. | - | The referral threshold on the probability of having cancer is non-decreasing as age increases. | Average-risk (0.76); high-risk (1.97) | - |
Zhang et al. (2012) | (14) | Patient | Control-limit type. | 74: Patient perspective | The referral threshold on the probability of having cancer is non-decreasing as age increases. | 0.102 | 0.115 |
Zhang et al. (2012) | (33) | Patient | Double control-limit type. | 76: Patient perspective | The referral threshold on the probability of having cancer is non-decreasing as age increases. | 0.131 (Patient perspective) | 0.165 (Patient perspective) |
Societal | 71: Societal perspective | 0.110 (Societal perspective) | 0.161 (Societal perspective) | ||||
Alagoz et al. (2013) | (34) | Patient | Double control-limit type. | - | The referral threshold on the probability of having cancer is non-decreasing as age increases. | - | 1.987 |
Erenay et al. (2014) | (35) | Patient | Control-limit type. | 78: Low-risk male | The referral threshold on the probability of having cancer is non-decreasing as age increases. | Low-risk male (0.97) | Low-risk male (0.16) |
83: High-risk male | High-risk male (2.78) | High-risk male (0.19) | |||||
86: Post-CRC male | Post-CRC male (4.27) | Post-CRC male (0.90) | |||||
80: Low-risk female | Low-risk female (0.85) | Low-risk female (0.13) | |||||
83: High-risk female | High-risk female (2.36) | High-risk female (0.15) | |||||
91: Post-CRC female | Post-CRC female (8.19) | Post-CRC female (3.18) |
4.4. Relationship Between Age and Risk Threshold in Optimal Policy Based-Screening Decisions
All the reviewed studies (n = 6, 100%) expressed that risk threshold based on optimal policy is a non-decreasing function of age (Figure 2) (14, 27, 32-35).
Risk threshold- the line between the biopsy area and the wait (screening) area- as a non-decreasing function of age.

4.5. Termination (Optimal Stopping)
Most guidelines do not specify a stopping age for cancer screening; however, a few numbers of them, for example, the United States Preventive Services Task Force (USPSTF), suggest that BC screening for women should be stopped after the age of 74 years (18). In the present review, Zhang et al. (14), Zhang et al. (33), and Erenay et al. (35) (n = 3, 50%) demonstrated some optimal stopping age for cancer screening. Chatwal et al., Ayer et al., and Alagoz et al. (n = 3, 50%) did not mention any stopping age (27, 32, 34).
3.6. Benefits of Optimal Policy
Five studies (n = 5, 83%) only considered patient perspective. In the aforementioned studies, QALY was considered the benefit measure, and screening and treatment costs were not included. Four out of five studies have shown that the optimal biopsy attained more QALYs than the guidelines or no screening strategies (6, 14, 32, 35). Only one study (n = 1, 17%) has taken societal perspective into account. Zhang et al. expressed that the expected incremental benefit of optimal screening over the traditional guideline of annual PSA screening with a threshold of 4ng/mL for the biopsy is estimated to be 0.165 and 0.161 QALYs per person from the patient and societal perspectives, respectively (33). They have used the cost of PSA, biopsy, and PC treatment and maximized the net benefit of cancer screening.
5. Discussion
This study systematically reviewed the published evidence regarding the optimal policies based on MDPs and POMDPs in cancer screening, and six publications were identified. The review results suggest that the optimal cancer screening policies are control-limit types; they outperform guidelines in terms of benefits, and the cancer risk threshold is a non-decreasing function of age. The results showed that individual screening based on cancer risk and age would have more benefits.
Based on our knowledge, this is the first study that has reviewed the optimization of the economic and clinical benefits for different cancer screening programs using MDPs and POMDPs models. The first objective was to describe the optimal policy in cancer screening programs. According to the current review, all studies showed that the optimal screening policy should be a control-limit type. They suggested that at every age, there is a risk threshold such that if the cancer probability is greater than the threshold, screening should be stopped, and the patient must be referred for biopsy or treatment (14, 27, 32-35). This policy can exclude some individuals from undergoing unnecessary screenings and consequentially reduces costs.
Age and cancer risk are two crucial factors in cancer screening; nevertheless, the guidelines do not provide a quantitative relationship between these factors and screening decisions. For example, the American Cancer Society (ACS) recommends annual BC screening for average-risk women aged 45 to 54 years; nevertheless, screening decisions need to be taken based on personal characteristics, such as gender, risk score, age, and life expectancy (16, 35). One of the important objectives of this study was to explore how, when a patient gets older, the cancer risk threshold, as a function of age, changes mathematically. When a patient grows older, life expectancy decreases, and cancer detection becomes less beneficial (12, 14, 33). Therefore, biopsy referral decisions will be beneficial if the cancer probability is higher than the risk threshold (14). As a result, the referral threshold for biopsy and treatment should be a non-decreasing function of patient age. All the reviewed studies showed that the risk threshold is non-decreasing concerning age; therefore, when age increases, the referral decision will be optimal if the probability of having cancer increases (14, 27, 32-35); nevertheless, cancer screening guidelines have not mentioned this issue, and they have not used an age-dependent referral threshold for each patient in their screening decisions (12, 15-18).
Cancer screening in older adults is more complicated. Older patients usually have multi-morbidity (the co-existence of multiple diseases), and the treatment of cancer might have bad effects on other diseases. Furthermore, the probability of dying from cancer might be smaller than the risk of death from other competing diseases (29). Therefore, after some age, it is not logical to treat a patient, and screening should be stopped. The clinical reasoning behind the way to calculate stopping age is that if the expected future reward from biopsy for a 100% cancer patient is less than the expected future reward for the patient who is never referred for biopsy, then screening should be terminated. Three studies used a stopping age in cancer screening (14, 33, 35). After this age, even if the cancer probability is 100%, it is not logical to refer a patient for biopsy or treatment.
The quality-adjusted life-year is the most common criterion used in the medical decision-making literature to measure the benefits of interventions for patients (37). With regard to effectiveness outcome, the findings of this study showed that QALYs were applied in all studies. Six studies only used QALYs as an outcome to measure the health and economic effects (14, 27, 32-35).
A screening program is successful if it can attain maximum net benefits (11). Five studies compared the benefits of optimal policy to guidelines in terms of QALYs. These studies showed that the optimal policy outperforms the guidelines (14, 27, 32-35).
Finally, the benefits of screening decrease as patients grow older due to the increased mortality risk and reduction in life expectancy. Therefore, screening intervals might be changed for older patients (35); however, guidelines suggest a constant screening interval or do not mention screening intervals. For example, ACS recommended annual mammography screening for women aged 50 to 54 years and biennially screening for women aged 55 years and older (15, 16). Two studies showed that it is necessary to use a variable screening interval based on risk and age to achieve an optimal policy (32, 35).
The most important limitation was that all studies were performed in the USA, and we had to compare the optimal policies to the American guidelines. All studies, but one, have implemented MDP and POMDP formulation only using QALYs and have not considered screening and treatment costs as factors to optimize policies. Limited resources in the health sector imply that healthcare costs need to be involved in decision-making to allocate resources (38). If these models can attain QALYs and costs of the optimal policy, cost-effectiveness analysis for optimal policies and national guidelines might be carried out. Thirdly, these studies have only been performed on breast, prostate, and colorectal cancer screening.
5.1. Conclusions
This study shows that cancer screening based on guidelines for all patients has some limitations. MDPs and POMDPs suggest that age and cancer risk are important variables in the decision for cancer screening. These factors can impact screening intervals, optimal policy, and referral decisions for biopsy and treatment. Markov decision processes and POMDPs quantified these effects. These models highlight personalized screening and show that this type of screening can outperform cancer screening guidelines regarding economic and clinical benefits. This study showed that the optimal screening policy is control-limit. There is a cancer risk threshold that is non-decreasing for age, and the screening interval is not necessarily constant for a group of individuals. These models highlight the importance of individual-based screening.
References
-
1.
World Health Organization. The top 10 causes of death. World Health Organization; 2017. Available from: https://www.who.int/news-room/fact-sheets/detail/the-top-10-causes-of-death.
-
2.
Bray F, Ferlay J, Soerjomataram I, Siegel RL, Torre LA, Jemal A. Global cancer statistics 2018: GLOBOCAN estimates of incidence and mortality worldwide for 36 cancers in 185 countries. CA Cancer J Clin. 2018;68(6):394-424. [PubMed ID: 30207593]. https://doi.org/10.3322/caac.21492.
-
3.
Boyle P, Anderson BO, Andersson LC, Ariyaratne Y, Auleley GR, Barbacid M, et al. Need for global action for cancer control. Ann Oncol. 2008;19(9):1519-21. [PubMed ID: 18723552]. https://doi.org/10.1093/annonc/mdn426.
-
4.
Gersten O, Wilmoth JR. The Cancer Transition in Japan since 1951. Demographic Res. 2002;7:271-306. https://doi.org/10.4054/DemRes.2002.7.5.
-
5.
Omran AR. The epidemiologic transition: a theory of the epidemiology of population change. 1971. Milbank Q. 2005;83(4):731-57. [PubMed ID: 16279965]. [PubMed Central ID: PMC2690264]. https://doi.org/10.1111/j.1468-0009.2005.00398.x.
-
6.
Alagoz O. Optimizing Cancer Screening Using Partially Observable Markov Decision Processes. Transforming Research into Action. 2011;3:75-89. https://doi.org/10.1287/educ.1110.0087.
-
7.
American Cancer Society. Cancer Facts & Figures 2020. Atlanta: American Cancer Society; 2020.
-
8.
Schiffman JD, Fisher PG, Gibbs P. Early detection of cancer: Past, present, and future. Am Soc Clin Oncol Educ Book. 2015;35(35):57-65. [PubMed ID: 25993143]. https://doi.org/10.14694/EdBook_AM.2015.35.57.
-
9.
Kerlikowske K, Grady D, Barclay J, Sickles EA, Eaton A, Ernster V. Positive predictive value of screening mammography by age and family history of breast cancer. JAMA. 1993;270(20):2444-50. [PubMed ID: 8230621].
-
10.
Brewer NT, Salz T, Lillie SE. Systematic review: the long-term effects of false-positive mammograms. Ann Intern Med. 2007;146(7):502-10. [PubMed ID: 17404352]. https://doi.org/10.7326/0003-4819-146-7-200704030-00006.
-
11.
U. S. Preventive Services Task Force, Bibbins-Domingo K, Grossman DC, Curry SJ, Davidson KW, Epling JJ, et al. Screening for Colorectal Cancer: US Preventive Services Task Force Recommendation Statement. JAMA. 2016;315(23):2564-75. [PubMed ID: 27304597]. https://doi.org/10.1001/jama.2016.5989.
-
12.
Qaseem A, Barry MJ, Denberg TD, Owens DK, Shekelle P, Clinical Guidelines Committee of the American College of P. Screening for prostate cancer: a guidance statement from the Clinical Guidelines Committee of the American College of Physicians. Ann Intern Med. 2013;158(10):761-9. [PubMed ID: 23567643]. https://doi.org/10.7326/0003-4819-158-10-201305210-00633.
-
13.
U. S. Preventive Services Task Force, Grossman DC, Curry SJ, Owens DK, Bibbins-Domingo K, Caughey AB, et al. Screening for Prostate Cancer: US Preventive Services Task Force Recommendation Statement. JAMA. 2018;319(18):1901-13. [PubMed ID: 29801017]. https://doi.org/10.1001/jama.2018.3710.
-
14.
Zhang J, Denton BT, Balasubramanian H, Shah ND, Inman BA. Optimization of Prostate Biopsy Referral Decisions. Manufacturing & Service Operations Management. 2012;14(4):529-47. https://doi.org/10.1287/msom.1120.0388.
-
15.
Oeffinger KC, Fontham ET, Etzioni R, Herzig A, Michaelson JS, Shih YC, et al. Breast Cancer Screening for Women at Average Risk: 2015 Guideline Update From the American Cancer Society. JAMA. 2015;314(15):1599-614. [PubMed ID: 26501536]. [PubMed Central ID: PMC4831582]. https://doi.org/10.1001/jama.2015.12783.
-
16.
Saslow D, Boetes C, Burke W, Harms S, Leach MO, Lehman CD, et al. American Cancer Society guidelines for breast screening with MRI as an adjunct to mammography. CA Cancer J Clin. 2007;57(2):75-89. [PubMed ID: 17392385]. https://doi.org/10.3322/canjclin.57.2.75.
-
17.
Qaseem A, Lin JS, Mustafa RA, Horwitch CA, Wilt TJ, Clinical Guidelines Committee of the American College of P, et al. Screening for Breast Cancer in Average-Risk Women: A Guidance Statement From the American College of Physicians. Ann Intern Med. 2019;170(8):547-60. [PubMed ID: 30959525]. https://doi.org/10.7326/M18-2147.
-
18.
Siu AL, U. S. Preventive Services Task Force. Screening for Breast Cancer: U.S. Preventive Services Task Force Recommendation Statement. Ann Intern Med. 2016;164(4):279-96. [PubMed ID: 26757170]. https://doi.org/10.7326/M15-2886.
-
19.
Committee on Practice Bulletins-Gynecology. Practice Bulletin Number 179: Breast Cancer Risk Assessment and Screening in Average-Risk Women. Obstet gynecol. 2017;130:e1-e16. https://doi.org/10.1097/AOG.0000000000002158.
-
20.
Croke LM. American college of obstetricians and gynecologists updates breast cancer screening guidelines. Am Fam Physician. 2012;85(6):654.
-
21.
Wolf AM, Wender RC, Etzioni RB, Thompson IM, D'Amico AV, Volk RJ, et al. American Cancer Society guideline for the early detection of prostate cancer: update 2010. CA Cancer J Clin. 2010;60(2):70-98. [PubMed ID: 20200110]. https://doi.org/10.3322/caac.20066.
-
22.
Smith RA, Andrews KS, Brooks D, Fedewa SA, Manassaram-Baptiste D, Saslow D, et al. Cancer screening in the United States, 2018: A review of current American Cancer Society guidelines and current issues in cancer screening. CA Cancer J Clin. 2018;68(4):297-316. [PubMed ID: 29846940]. https://doi.org/10.3322/caac.21446.
-
23.
A Cancer Journal for Clinicians. Screening for colon and rectal cancer in average-risk adults. CA Cancer J Clin. 2018;68(4):282-3. [PubMed ID: 29846949]. https://doi.org/10.3322/caac.21458.
-
24.
Wolf AMD, Fontham ETH, Church TR, Flowers CR, Guerra CE, LaMonte SJ, et al. Colorectal cancer screening for average-risk adults: 2018 guideline update from the American Cancer Society. CA Cancer J Clin. 2018;68(4):250-81. [PubMed ID: 29846947]. https://doi.org/10.3322/caac.21457.
-
25.
Qaseem A, Crandall CJ, Mustafa RA, Hicks LA, Wilt TJ, Clinical Guidelines Committee of the American College of P, et al. Screening for Colorectal Cancer in Asymptomatic Average-Risk Adults: A Guidance Statement From the American College of Physicians. Ann Intern Med. 2019;171(9):643-54. [PubMed ID: 31683290]. [PubMed Central ID: PMC8152103]. https://doi.org/10.7326/M19-0642.
-
26.
White MC, Holman DM, Boehm JE, Peipins LA, Grossman M, Henley SJ. Age and cancer risk: a potentially modifiable relationship. Am J Prev Med. 2014;46(3 Suppl 1):S7-15. [PubMed ID: 24512933]. [PubMed Central ID: PMC4544764]. https://doi.org/10.1016/j.amepre.2013.10.029.
-
27.
Chhatwal J, Alagoz O, Burnside ES. Optimal Breast Biopsy Decision-Making Based on Mammographic Features and Demographic Factors. Oper Res. 2010;58(6):1577-91. [PubMed ID: 21415931]. [PubMed Central ID: PMC3057079]. https://doi.org/10.1287/opre.1100.0877.
-
28.
Armstrong K, Moye E, Williams S, Berlin JA, Reynolds EE. Screening mammography in women 40 to 49 years of age: a systematic review for the American College of Physicians. Ann Intern Med. 2007;146(7):516-26. [PubMed ID: 17404354]. https://doi.org/10.7326/0003-4819-146-7-200704030-00008.
-
29.
Steimle LN, Denton BT. Markov Decision Processes for Screening and Treatment of Chronic Diseases. In: Boucherie RJ, van Dijk NM, editors. Markov Desicion Process in practice. 248. Springer; 2017. p. 189-222. https://doi.org/10.1007/978-3-319-47766-4_6.
-
30.
Puterman ML. Markov decision processes: discrete stochastic dynamic programming. John Wiley & Sons; 2014.
-
31.
Steimle LN, Kaufman DL, Denton BT. Multi-model Markov decision processes. Optimization Online; 2018. Available from: https://optimization-online.org/2018/01/6434/.
-
32.
Ayer T, Alagoz O, Stout NK. OR Forum—A POMDP Approach to Personalize Mammography Screening Decisions. Oper Res. 2012;60(5):1019-34. https://doi.org/10.1287/opre.1110.1019.
-
33.
Zhang J, Denton BT, Balasubramanian H, Shah ND, Inman BA. Optimization of PSA screening policies: a comparison of the patient and societal perspectives. Med Decis Making. 2012;32(2):337-49. [PubMed ID: 21933990]. [PubMed Central ID: PMC3288242]. https://doi.org/10.1177/0272989X11416513.
-
34.
Alagoz O, Chhatwal J, Burnside ES. Optimal Policies for Reducing Unnecessary Follow-up Mammography Exams in Breast Cancer Diagnosis. Decis Anal. 2013;10(3):200-24. [PubMed ID: 24501588]. [PubMed Central ID: PMC3910299]. https://doi.org/10.1287/deca.2013.0272.
-
35.
Erenay FS, Alagoz O, Said A. Optimizing Colonoscopy Screening for Colorectal Cancer Prevention and Surveillance. Manuf Serv Oper Manag. 2014;16(3):381-400. https://doi.org/10.1287/msom.2014.0484.
-
36.
Crown W, Buyukkaramikli N, Thokala P, Morton A, Sir MY, Marshall DA, et al. Constrained Optimization Methods in Health Services Research-An Introduction: Report 1 of the ISPOR Optimization Methods Emerging Good Practices Task Force. Value Health. 2017;20(3):310-9. [PubMed ID: 28292475]. https://doi.org/10.1016/j.jval.2017.01.013.
-
37.
Packer AH. Applying Cost-Effectiveness Concepts to the Community Health System. Oper Res. 1968;16(2):227-53. https://doi.org/10.1287/opre.16.2.227.
-
38.
Bambha K, Kim WR. Cost-effectiveness analysis and incremental cost-effectiveness ratios: uses and pitfalls. Eur J Gastroenterol Hepatol. 2004;16(6):519-26. [PubMed ID: 15167152]. https://doi.org/10.1097/00042737-200406000-00003.