Abstract
Objectives:
This study aimed to identify and prioritize the dimensions and indicators of measuring customer lifetime value (CLV) for the supplemental health insurance industry.Methods:
In this exploratory qualitative study, first, the initial dimensions and indicators of CLV were extracted through a scoping review. Next, 20 key informants, including academics and insurance industry experts, were selected by purposive sampling and then interviewed to identify and extract other dimensions and indicators of CLV for the supplemental health insurance industry. The data were collected using an in-depth and semi-structured interview and analyzed using the fuzzy analytic hierarchy process (AHP).Results:
Out of 232 retrieved articles, 13 studies were eligible to include in this scoping review. After reviewing this study, seven general factors, including customer profitability, service cost, customer retention rate, revenue, discount rate, and time and price paid by the customer, were identified as effective factors in CLV. Moreover, 61 comments were identified after interviewing experts (at the first stage of the interview). Then, after three stages of semi-structured interviews, 17 comments were removed, and 44 comments were approved. Finally, three dimensions, including profitability, customer loyalty, and value co-creation, and 13 indicators, including customer satisfaction, trust, repurchase intention, customer trust, brand performance to repurchase, financial purchasing pattern, service delivery pattern, information search, information sharing, co-production, feedback, helping, and tolerance, were extracted. After conducting the fuzzy AHP, profitability (0.652), customer loyalty (0.226), and customer value co-creation (0.122) were the most important dimensions for CLV, respectively. Additionally, purchase pattern (0.274), financial procedures (0.261), and service delivery pattern (0.117) had the highest priority among the indicators, respectively.Conclusions:
According to the results, although customer profitability was the most important dimension to measure CLV, CLV is a combinatorial concept. Therefore, the dimensions of customer loyalty and customer value co-creation should be taken into consideration as effective dimensions in predicting and measuring the concept.Keywords
Customer Lifetime Value Customer Profitability Customer Value Co-creation Customer Loyalty Supplementary Health Insurance
1. Background
The origin concept of value is used when companies would like to utilize different technologies to analyze the customer buying behavior and maximize the value of the transaction with the customer (1). This criterion has been widely accepted in the marketing literature (2). In any sustainable business, companies, at first, create value by offering suggestions to the customer and then gain value from the customer (by making a profit) (3).
Issues related to the insurance industry in the complementary health sector are related to poverty due to imposing medical costs, expectations related to the health sector, and the growing number of customers in the insurance industry (4). Insurance companies are constantly faced with the challenge of whether the resources spent on customers are tailored to the amount of profit they make (5). Every year, insurers lose 15% of their customers, which by reducing this figure by 5%, the average growth of companies can be increased by more than 50% (6).
Insurance companies are considered active and profitable institutions in numerous countries worldwide (7). However, despite significant changes in the last two decades, the Iranian insurance industry is still facing a low penetration rate (8). Therefore, customer retention is one of the most important functions of marketing management. Management researchers have considered this for two important reasons; firstly, the cost of attracting new customers in unstable market environments is very high; secondly, customers have gained more profit as they continue to do business with companies (9).
For the creation of competitive advantage, organizations need to examine the behavioral characteristics of their customers (10). Improving customer relationships and business performance requires formulating analytical programs, such as the estimation of customer value over the life of the customer (11). The mechanism of this concept is to promote the management knowledge of companies in competitive environments (1), which is obtained by measuring all future profits obtained from the customer and the losses incurred by the company during the customer’s purchase life (12). There are several known models in this field as follows: (1) the recency, frequency, monetary value models in which the financial attitude is not so important and relies more on the customer’s previous purchases to predict the customer’s behaviors in the future (13); (2) SOW model in which the most emphasis is on the customer’s share of purchases from a company; (3) PCV model in which the most emphasis is on the performance and profitability of the customer in the past; (4) ROI model in which the amount of return on investment is considered the main factor in calculating customer value and understanding what value is crucial (14).
The calculation of customer retention rates allows companies to evaluate the value of the transaction with the customer, provides the information needed to make decisions about customer retention, and helps better manage the customer portfolio (15).
2. Objectives
This study aimed to identify the dimensions and indicators of the lifetime value of supplementary health insurance customers to prioritize these concepts and the correct understanding of the concept of customer lifetime value (CLV) to establish a win-win relationship between insurance companies and the insured.
3. Methods
This exploratory qualitative study was carried out on 20 faculty members of Ahvaz Jundishapur University, Khuzestan, Iran, and the insurance industries using the purposive sampling method. The data were collected using the scoping review approach and in-depth and semi-structured interviews. The data were classified using the data-driven method and the fuzzy analytic hierarchy process (AHP) and analyzed using Excel software (version 2010) (Figure 1). For the identification and prioritization of the dimensions and indicators of CLV (Figure 2), the following steps were taken:
Process of designing and explaining the indicators of customer lifetime value
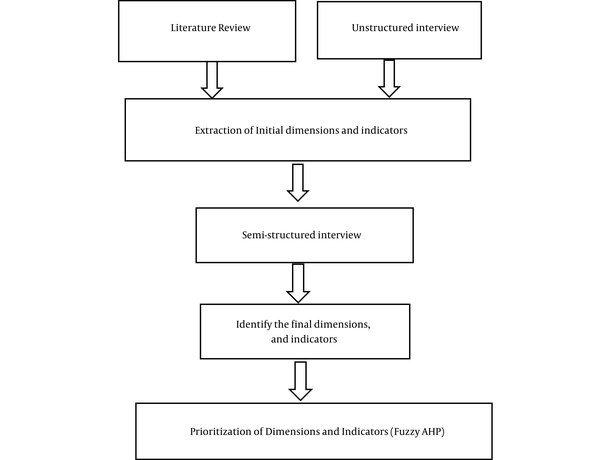
Diagram of the scoping review process
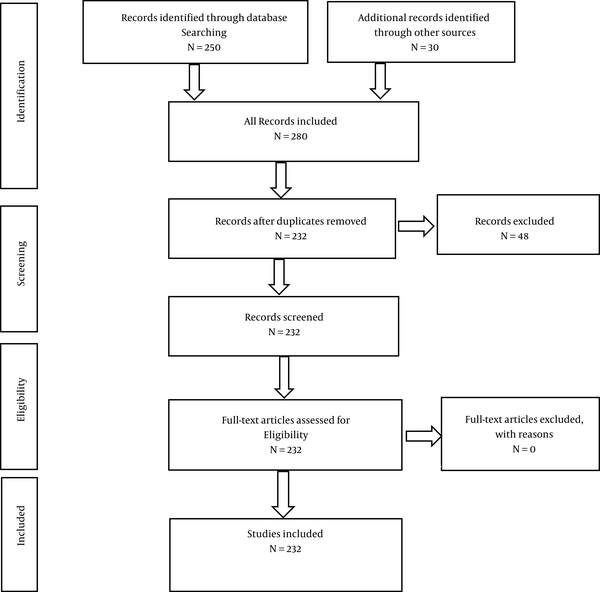
3.1. Identification of Dimensions and Indicators of CLV
For the determination of initial indicators and factors affecting CLV, this study first identified the main research question (i.e., what factors are related to the CLV of supplementary health insurance clients?). Then, after developing the search strategy, keywords related to customer longevity value, customer profitability, customer value loyalty, and customer value co-creation were combined. The literature search was performed in several electronic databases, namely IranDoc, Civilica, Google Web, SID, Scholar Scopus, Emerald, and Elsevier, within 2019 to 2020.
The studies published within 2015 - 2020 were reviewed. Considering the novelty of the research and the lack of correlation of all dimensions with the concept of CLV in previous studies in this field, the search strategy in this study was selected. Firstly, the concept of CLV was examined to identify the relevant literature of the CLV concept and the existing gaps in this area. Secondly, the literature associated with factors affecting the CLV concept was searched. Then, the articles that met the inclusion criteria were evaluated. The inclusion criteria were analytical and descriptive studies and articles that included the aforementioned keywords. Finally, after reviewing the full text of the articles, the required data were extracted. An unstructured interview was employed to complete the results of the study. Next, for interviewing the experts, an interview guide was designed based on the framework extracted from the review study and unstructured interviews. Finally, experts’ opinions were extracted through data coding (using the data-driven technique), and the results were given to the experts to measure CLV.
3.2. Prioritization of Dimensions and Indicators of CLV (Fuzzy AHP)
In this step, after identifying and confirming dimensions and indicators by the experts, the fuzzy AHP was used to prioritize dimensions. Accordingly, the pairwise comparison matrices of the criteria were formulated and given to the experts. After answering the pairwise comparisons matrices, the rate of incompatibility of the tables was calculated. Then, using the geometric mean formula, the answers were combined and integrated into pairwise comparisons matrices. The weight of pairwise comparisons matrices were also calculated using the geometric mean formula.
4. Results
4.1. Demographic Profile (Expert Panel Members)
In the present study, 18 male and 3 female subjects were included (Table 1).
Demographic Results
Variables | Frequency | Percentage |
---|---|---|
Educational status | ||
Bachelor’s degree | 7 | 35 |
Master’s degree | 8 | 40 |
PhD | 5 | 25 |
Age (y) | ||
30 - 40 | 5 | 25 |
40 - 50 | 13 | 65 |
Over 50 | 2 | 10 |
Gender | ||
Male | 18 | 90 |
Female | 2 | 10 |
Total | 20 | 100 |
4.2. Results of Extracted Dimensions and Indicators of CLV
A total of 280 articles were identified through electronic databases, of which 250 articles were excluded, and 30 were grey literature (e.g., books and dissertations). After removing 48 duplicates, 232 articles were remained to examine the indicators and dimensions of the model (Figure 2). After reviewing the concepts, seven categories related to the CLV concept, including customer profitability, service cost, customer retention rate, revenue, discount rate, and time and price paid by the customer, were identified (Table 2). Furthermore, the factors affecting CLV were extracted in the form of three dimensions, including profitability (4 indicators), customer loyalty (8 indicators), and customer value co-creation (20 indicators) (Table 3).
Indicators of Customer Lifetime Value
Authors | Price | Time | Discount | Income | Retention Rate | Cost of Providing Services | Customer Profitability |
---|---|---|---|---|---|---|---|
Paid by the Customer | Purchase Horizon and Last Purchase Time | Discount Rate | Round Financial Income and Repeat Purchases | Average Absorption Cost, Absorption Rate, Probability of Customer Retention, and Probability of Leaving the Customer | Advertising, Attraction Cost, Customer Attraction Rate, Marketing Cost for Additional Sales, and Total Production Cost | Annual Profit, Profit Margin, Average Fixed Profit, Last Year Profit, Potential Profit, Profitability, and Purchase Financial Value | |
McNeil and Carpenter (1995) (16) | * | * | * | * | * | ||
Blatterbag and Dayton (1996) (17) | * | * | * | * | |||
Gupta and Lehmann (2003) (18) | * | * | * | ||||
Reinartz and Kumar (2003) (19) | * | * | * | * | * | ||
Burger and Nasr (1998) (20) | * | ||||||
Blatterbag et al. (2001) (21) | * | * | * | ||||
Blatterbag et al. (2008) (22) | * | * | * | ||||
Kumar et al. (2007) (23) | * | * | * | * | |||
Hwang et al. (2004) (24) | * | * | |||||
Blatterbag et al. (2008) (25) | * | * | |||||
Heitz et al. (2011) (26) | * | * | * | ||||
Jain and Singh (2002) (27) | * | * | * | ||||
Busacca and Bertoli (2009) (28) | * | * | * |
Factors Affecting Customer Lifetime Value
Row | Dimensions | Proposed Indicators |
---|---|---|
1 | Customer profitability | Service delivery pattern; Purchasing pattern; Financial procedures; Cross-selling |
2 | Customer loyalty | Intention to repurchase; Brand preference; Customer trust; Word of mouth; Satisfaction; Product quality; Commitment; Trust |
3 | Customer value co-creation | Information search; Co-production; Feedback; Helping; Information sharing; Tolerance; Communication; Defense; Advocacy; Responsible behavior; Personal interaction; Awareness; Brain activity; Co-learning; Risk assessment; Accessibility; Transparency; Co-production use; Citizen behavior; Conversation |
After analyzing the interviews with experts, out of 61 comments coded in this study, 17 codes were removed (due to low frequency), and 44 codes remained. Additionally, three dimensions with 13 indicators were identified in the literature review stage, including customer loyalty (5 indicators and 21 items), customer profitability (3 indicators and 10 items), and customer value co-creation (6 indicators and 13 items) (Table 4).
Results of Foundation Data Analysis
Axial Coding | Concepts in Open Coding | Frequency |
---|---|---|
Customer loyalty | ||
Customer satisfaction | 20 | |
Appropriateness of the per capita membership fee rate in the supplementary health insurance contract | 17 | |
Order in conducting insurance affairs | 16 | |
Appropriate information on how to provide complementary health insurance services | 15 | |
Reasonability of documents required to pay for medical expenses | 14 | |
Providing online services and medical centers of the contracting party | 13 | |
Lack of time to pay for medical expenses | 14 | |
Timely processing of complaints of the insured | 16 | |
High ceilings and coverage of obligations | 13 | |
Polite behavior of company employees | 12 | |
No waiting period in providing services | 9 | |
Single membership rate for all age groups | 17 | |
Consistency in the quality of service delivery | 13 | |
Repurchase intent | 20 | |
Providing positive experiences and benefits of insurance company services with friends and colleagues | 12 | |
Encouraging others to buy insurance policies from the insurance company | 14 | |
Customer trust | 20 | |
Privacy (content of medical records) | 10 | |
Feeling of security | 16 | |
Treating the customer honestly | 12 | |
Brand performance | 20 | |
Trained, skilled, and experienced staff | 13 | |
Well-known and reputable brand of the insurance company | 13 | |
Intent to repurchase | 20 | |
The first choice to receive supplementary health insurance services | 13 | |
Intention to buy insurance policy in the future from the insurance company | 13 | |
Customer profitability | ||
Financial procedures | 17 | |
Cash purchase instead of installment purchase | 17 | |
Low customer purchase discounts | 16 | |
Purchasing pattern | 16 | |
Lots of money to buy from this insurance company | 17 | |
Frequency of purchases from this insurance company | 16 | |
Predictability of purchase | 15 | |
Cost of advertising the services of the insurance company | 13 | |
Purchase of other insurance policies by the customer from the insurance company | 16 | |
Service delivery pattern | 16 | |
Requesting fast delivery of services from the insurance company | 12 | |
Low training costs and benefits of insurance services | 13 | |
Low loss ratio of insurance policies purchased from the insurance company | 16 | |
Customer value co-creation | ||
Information search | 12 | |
Looking for information about complementary health insurance services | 12 | |
Easy access to information | 10 | |
Information sharing | 14 | |
Providing the required information and documents for the insurance company | 12 | |
Providing helpful ideas on how to improve services | 13 | |
Co-production | 16 | |
Participating in the design of commitments and how to provide complementary health insurance services | 13 | |
Participating in problem-solving | 12 | |
Feedback | 17 | |
Providing problem and constructive criticism of the insurance company | 14 | |
A good and honest relationship with the insurance company | 13 | |
Helping | 17 | |
Helping other customers | 14 | |
Advising other customers | 13 | |
Tolerance | 16 | |
Being patient to receive medical expenses | 16 | |
Tolerance in case of mistakes in providing services | 13 |
3.3. Results of Fuzzy AHP Analysis
The rate of incompatibility of the tables was estimated at less than 0.1, indicating the stability and reliability of pairwise comparisons. The indicators were ranked, respectively, as follows: (1) purchasing pattern (weight: 0.274); (2) financial procedure (weight: 0.261); (3) service delivery pattern (weight: 0.117); (4) customer satisfaction (weight: 0.061); (5) purchase intention (weight: 0.05); (6) brand preference (weight: 0.044); (7) customer trust (weight: 0.035); (8) recommendation to others (weight: 0.33); (9) helping (weight: 0.033); (10) co-production (weight: 0.032); (11) information sharing (weight: 0.023); (12) tolerance (weight: 0.019); (13) feedback (weight: 0.015); and (14) information search (weight: 0.014) (Table 5)
Results of Fuzzy Analytic Hierarchy Process Analysis
Criterion/Index | Relative Weight | Final Weight | Rank |
---|---|---|---|
Customer loyalty | |||
Customer satisfaction | 0.269 | 0.061 | 4 |
Brand performance | 0.196 | 0.044 | 6 |
Word of mouth | 0.147 | 0.033 | 8 |
Intent to repurchase | 0.233 | 0.053 | 5 |
Customer trust | 0.155 | 0.035 | 7 |
Customer profitability | |||
Service delivery pattern | 0.179 | 0.117 | 3 |
Financial procedures | 0.401 | 0.261 | 2 |
Purchasing pattern | 0.420 | 0.274 | 1 |
Customer value co-creation | |||
Co-production | 0.267 | 0.032 | 9 |
Helping | 0.267 | 0.033 | 8 |
Information sharing | 0.192 | 0.023 | 10 |
Tolerance | 0.152 | 0.019 | 11 |
Feedback | 0.122 | 0.015 | 12 |
Information search | 0.115 | 0.014 | 13 |
5. Discussion
Given the characteristics of each industry, the basis for assessing CLV is different (29). This study aimed to identify and prioritize the dimensions and indicators of measuring CLV in the supplemental health insurance industry using a multidimensional approach to accurately predict CLV and create appropriate value for policyholders and insurance companies. In this study, CLV was examined using the data-driven method and by identifying 13 indicators in three dimensions (e.g., customer profitability, customer loyalty, and customer value co-creation). This study was examined at two levels due to multilateralism and lack of a purely financial view of the subject. Therefore, it is understandable why some relationships with the concept of CLV have not been considered in other studies.
Given the similarities and differences between the included studies, the obtained results were classified into two levels, namely dimension (i.e., the relationship with the concept of CLV) and indicator (i.e., the relationships of indicators with dimensions). In the current study, profitability in measuring CLV was the most important priority, which is consistent with the results of a study by Wang and Hong (2006) (30). However, there are differences in profitability calculations in the study of Wang and Hong (i.e., the calculations based on accounting criteria, such as income and expenses), which might be due to the concept of profitability. Therefore, it is suggested to take the indicators of financial procedures, purchasing patterns, and service delivery patterns into account to more accurately measure customer profitability in different industries and make more effective decisions about how to treat customers.
The financial practice indicator was identified as the second in the present study, which is in line with the results of a study by Dawson et al. (2017) (31). It is suggested to help managers and researchers adopt appropriate strategies using these indicators. The buying pattern indicator was the most important priority, which is in line with the results of studies by Leon et al. (2020) (32) and Vickers (2020) (33). In the present study, the indicator of customer loyalty was identified as the second important and effective dimension in measuring the value of customer longevity, which is consistent with the results of studies by Wu and Li (2018) (34), Flint et al. (2010) (35), Meyer-Warden (2007) (36), and Chen et al. (2015) (37) who examined and confirmed the relationship between these two concepts. However, there are differences in the organization and application of indicators between studies, which is important due to the industry requirements examined in this study. Therefore, for the indication of the importance of the indicators of loyalty in the industry, researchers, and decision-makers of the insurance industry should apply the indicators studied in the present study in other insurance industry sectors using the existing capacities.
The importance of loyalty indicators in the insurance industry was confirmed in studies by Abror et al. (2019) (38) and Singh et al. (2017) (39). Loyalty indicators are among the most important factors in the insurance industry that experts should consider and prioritize. However, due to some differences in utilizing these indicators, it is recommended to prioritize each of these indicators.
The findings of the current study also showed a close association between customer value co-creation and CLV. Due to the nonuse of this dimension in studies in CLV, researchers need to examine and pay more attention to this dimension in other industries to measure CLV and improve customer relationship productivity. The obtained results of customer value co-creation in the present study are in line with the results of studies by Nadeem et al. (2020) (40), Assiouras et al. (2019) (41), Tommasetti et al. (2015) (42), and Yi and Gong (2013) (43), who have developed and reviewed some indicators of customer value in different industries. The disparity between studies can be attributed to the lack of specialized indicators in complementary health insurance and the use of scoping review methods, interviews, AHP method, and structural equation methods to simultaneously analyze the concept. Therefore, it is suggested to use the aforementioned methods simultaneously to compile effective and efficient indicators in each industry sector. Furthermore, the model can be used to measure the value of customers’ longevity to indicate the choice of the right path to the identification of the factors affecting the dimension.
This study has some limitations. Firstly, due to the complexity and frequency of variables and the lack of relevant studies in this area, the effect of moderator variables was not considered. Secondly, the interviews were not conducted face-to-face due to the coronavirus disease 2019 pandemic.
5.1. Conclusions
According to the results, although customer profitability was the most important dimension to measure CLV, CLV is a multidimensional concept. Therefore, the dimensions of customer loyalty and customer value co-creation should be taken into consideration as effective dimensions in predicting and measuring the concept. The results of this study can be helpful for other industries to identify and formulate the measurement dimensions of CLV. Additionally, conducting comparative studies in different industries and different countries and identifying the moderating variables in the current study can be helpful to make better use of the concept of CLV.
References
-
1.
Weng C, Huang TC. Knowledge acquisition of association rules from the customer-lifetime-value perspective. Kybernetes. 2018;47(3):441-57. https://doi.org/10.1108/k-03-2016-0042.
-
2.
Ho MH, Chung HF. Customer engagement, customer equity and repurchase intention in mobile apps. J Bus Res. 2020;121:13-21. https://doi.org/10.1016/j.jbusres.2020.07.046.
-
3.
Xiao M, Ma Q, Li M. The impact of customer resources on customer value in co-creation: The multiple mediating effects. Journal of Contemporary Marketing Science. 2020;3(1):33-56. https://doi.org/10.1108/jcmars-08-2019-0032.
-
4.
Vahedi S, Rezapour A, Khiavi FF, Esmaeilzadeh F, Javan-Noughabi J, Almasiankia A, et al. Decomposition of socioeconomic inequality in catastrophic health expenditure: An evidence from Iran. Clin Epidemiology Glob Health. 2020;8(2):437-41. https://doi.org/10.1016/j.cegh.2019.10.004.
-
5.
Kumar V, Choi JB, Greene M. Synergistic effects of social media and traditional marketing on brand sales: Capturing the time-varying effects. J Acad Mark Sci. 2016;45(2):268-88. https://doi.org/10.1007/s11747-016-0484-7.
-
6.
Desirena G, Diaz A, Desirena J, Moreno I, Garcia D, editors. Maximizing customer lifetime value using stacked neural networks: An insurance industry application. 18th IEEE International Conference On Machine Learning And Applications (ICMLA). 2019; Florida, USA. 2019. p. 541-4.
-
7.
Kalaimani G. P’s of services marketing in insurance and banking services. J Manage Sci. 2013;1(3):1-169.
-
8.
Mazloomi N, Haghighi Kaffash M, Khashei V, Nateghi AA. Strategic management risks model in the Iranian insurance industry. Commercial Strategies. 2020;15(12):125-42.
-
9.
Darzi MA, Bhat SA. Personnel capability and customer satisfaction as predictors of customer retention in the banking sector. Int J Bank Mark. 2018;36(4):663-79. https://doi.org/10.1108/ijbm-04-2017-0074.
-
10.
Jamrozy U, Lawonk K. The multiple dimensions of consumption values in ecotourism. Int J Cult Tour Hosp Res. 2017;11(1):18-34. https://doi.org/10.1108/ijcthr-09-2015-0114.
-
11.
Çavdar AB, Ferhatosmanoğlu N. Airline customer lifetime value estimation using data analytics supported by social network information. J Air Transp Manag. 2018;67:19-33. https://doi.org/10.1016/j.jairtraman.2017.10.007.
-
12.
Hajipour B, Esfahani M. Delta model application for developing customer lifetime value. Marketing Intelligence & Planning. 2019;37(3):298-309. https://doi.org/10.1108/mip-06-2018-0190.
-
13.
Akroush MN, Mahadin BK. An intervariable approach to customer satisfaction and loyalty in the internet service market. Internet Res. 2019;29(4):772-98. https://doi.org/10.1108/IntR-12-2017-0514.
-
14.
Rashid Y, Tanveer A, Shaukat Z, Sadiq I. Value co-creation features: an empirical case study of B2B collaboration and interactions in New Zealand. Digit Libr Perspect. 2020;36(3):219-29. https://doi.org/10.1108/dlp-02-2020-0006.
-
15.
Channa HS. Customer lifetime value: An ensemble model approach. In: Balas VE, Sharma N, Chakrabarti A, editors. Data management, analytics and innovation: Proceedings of ICDMAI 2018. 1. Singapore: Springer; 2019. p. 353-63. https://doi.org/10.1007/978-981-13-1402-5_27.
-
16.
McNeil R, Carpenter M. Customer Satisfaction really reflected in Traditional Customer Satisfaction Measures. Amsterdam: Customer Satisfaction Research, European Society for Opinion and Marketing Research; 1995.
-
17.
Blattberg RC, Deighton J. Manage marketing by the customer equity test. Harv Bus Rev. 1996;74(4):136-44. [PubMed ID: 10158473].
-
18.
Gupta S, Lehmann DR. Customers as assets. J Interact Mark. 2003;17(1):9-24. https://doi.org/10.1002/dir.10045.
-
19.
Reinartz WJ, Kumar V. The impact of customer relationship characteristics on profitable lifetime duration. J Mark. 2018;67(1):77-99. https://doi.org/10.1509/jmkg.67.1.77.18589.
-
20.
Berger PD, Nasr NI. Customer lifetime value: Marketing models and applications. J Interact Mark. 1998;12(1):17-30. https://doi.org/10.1002/(sici)1520-6653(199824)12:1<17::aid-dir3>3.0.co;2-k.
-
21.
Blattberg R, Getz G, Thomas JS. Customer equity: Building and managing relationships as valuable assets. Boston, USA: Harvard Business Review Press; 2001.
-
22.
Blattberg RC, Kim B, Neslin SA. Why Database Marketing? Database marketing. 18. New York, USA: Springer; 2008. p. 13-46. https://doi.org/10.1007/978-0-387-72579-6_2.
-
23.
Kumar V, Petersen JA, Leone RP. How valuable is word of mouth? Harv Bus Rev. 2007;85(10):139-44-166. [PubMed ID: 17972501].
-
24.
Hwang H, Jung T, Suh E. An LTV model and customer segmentation based on customer value: A case study on the wireless telecommunication industry. Expert Syst Appl. 2004;26(2):181-8. https://doi.org/10.1016/s0957-4174(03)00133-7.
-
25.
Blattberg RC, Malthouse EC, Neslin SA. Customer lifetime value: Empirical generalizations and some conceptual questions. J Interact Mark. 2009;23(2):157-68. https://doi.org/10.1016/j.intmar.2009.02.005.
-
26.
Heitz C, Dettling M, Ruckstuhl A. Modelling customer lifetime value in contractual settings. Int J Serv Technol Manag. 2011;16(2):172. https://doi.org/10.1504/ijstm.2011.042595.
-
27.
Jain D, Singh SS. Customer lifetime value research in marketing: A review and future directions. J Interact Mark. 2002;16(2):34-46. https://doi.org/10.1002/dir.10032.
-
28.
Busacca B, Bertoli G. [Valore per il cliente, soddisfazione, fedeltà: il contributo del marleting al valore dell'impresa]. Brussels, Belgium: Egea Tools; 2009. Italian.
-
29.
Permana D, Pasaribu US, Indratno SW; Suprayogi. Classification of customer lifetime value models using Markov chain. Journal of Physics: Conference Series. 2017;893:12026. https://doi.org/10.1088/1742-6596/893/1/012026.
-
30.
Wang H, Hong W. Managing customer profitability in a competitive market by continuous data mining. Ind Mark Manag. 2006;35(6):715-23. https://doi.org/10.1016/j.indmarman.2005.06.005.
-
31.
Dawson B, Young L, Murray JM, Wilkinson I. Drivers of supplier-customer relationship profitability in China: Assessing International Joint Ventures versus State Owned Enterprises. Ind Mark Manag. 2017;66:29-41. https://doi.org/10.1016/j.indmarman.2017.06.009.
-
32.
León C, Barucca P, Acero O, Gage G, Ortega F. Pattern recognition of financial institutions’ payment behavior. Latin American Journal of Central Banking. 2020;1(1-4):100011. https://doi.org/10.1016/j.latcb.2020.100011.
-
33.
Vickers NJ. Animal communication: When i'm calling you, will you answer too? Curr Biol. 2017;27(14):R713-5. [PubMed ID: 28743020]. https://doi.org/10.1016/j.cub.2017.05.064.
-
34.
Wu Y, Li EY. Marketing mix, customer value, and customer loyalty in social commerce. Internet Res. 2018;28(1):74-104. https://doi.org/10.1108/IntR-08-2016-0250.
-
35.
Flint DJ, Blocker CP, Boutin PJ. Customer value anticipation, customer satisfaction and loyalty: An empirical examination. Ind Mark Manag. 2011;40(2):219-30. https://doi.org/10.1016/j.indmarman.2010.06.034.
-
36.
Meyer-Waarden L. The effects of loyalty programs on customer lifetime duration and share of wallet. Journal of Retailing. 2007;83(2):223-36. https://doi.org/10.1016/j.jretai.2007.01.002.
-
37.
Chen Q, Zhang M, Zhao X. Analysing customer behaviour in mobile app usage. Ind Manag Data Syst. 2017;117(2):425-38. https://doi.org/10.1108/imds-04-2016-0141.
-
38.
Abror A, Patrisia D, Engriani Y, Evanita S, Yasri Y, Dastgir S. Service quality, religiosity, customer satisfaction, customer engagement and Islamic bank’s customer loyalty. J Islam Mark. 2019;11(6):1691-705. https://doi.org/10.1108/jima-03-2019-0044.
-
39.
Singh G, Singh I, Vij S. Antecedents and consequences of customer loyalty: A conceptual model. Int J Appl Bus Econ Res. 2017;15(23):237-51.
-
40.
Nadeem W, Juntunen M, Shirazi F, Hajli N. Consumers’ value co-creation in sharing economy: The role of social support, consumers’ ethical perceptions and relationship quality. Technol Forecast Soc Change. 2020;151:119786. https://doi.org/10.1016/j.techfore.2019.119786.
-
41.
Assiouras I, Skourtis G, Giannopoulos A, Buhalis D, Koniordos M. Value co-creation and customer citizenship behavior. Ann Tour Res. 2019;78:102742. https://doi.org/10.1016/j.annals.2019.102742.
-
42.
Tommasetti A, Troisi O, Vesci M. Measuring customer value co-creation behavior. J Serv Theory Pract. 2017;27(5):930-50. https://doi.org/10.1108/jstp-10-2015-0215.
-
43.
Yi Y, Gong T. Customer value co-creation behavior: Scale development and validation. J Bus Res. 2013;66(9):1279-84. https://doi.org/10.1016/j.jbusres.2012.02.026.