Abstract
Background:
The present study aims at visualizing and analyzing the scientific productions of data mining in medical sciences in the Web of Science (WoS) database to identify knowledge structure in this field.Methods:
The present study was carried out using co-word analysis, which is a scientometrics method. Also, the analysis of the WoS approach was used for the visualization and analysis of word co-occurrence networks in the data mining domain. The research population consisted of 14,430 records related to data mining published in WoS from 2008 to 2016. The data were analyzed after retrieval using VoSviewer scientometric software.Results:
The research findings showed that the average annual growth rate of scientific productions in the data mining field had an upward trend and reached its peak in 2016. The findings also showed that the USA was the most productive country, with 8123 degrees, claiming the first rank by 27.4% of the total scientific production, and Iran was in the 13th rank with 678 degrees. Among universities and institutions, the Chinese Academy of Sciences (692 degrees), the University of California (621 degrees), and the National Center for Scientific Research (CNSR) (514 degrees) were ranked first to third globally. Among Iranian institutions and universities, Islamic Azad University (IAU), Tehran University, and Iran University of Technological Sciences were ranked 41st, 94th, and 200th in the world with 140, 105, and 66 degrees, respectively. The results of the current research also showed that the field of data mining was formed based on four vocabulary clusters with 108, 82, 73, and 42 words, respectively.Conclusions:
The recognition of Iran as a successful country in the field of data mining should encourage researchers from other countries to communicate with Iranian scientists.Keywords
Scientometrics Co-word Analysis Network Analysis Medical Sciences Visualizing Data Mining
1. Background
Today, in most organizations, data and information are being collected and stored rapidly. Nevertheless, despite this massive amount of data, organizations face a shortage of knowledge for decision-making (1, 2). In today’s electronic and advanced world, along with the increasing progress of database applications, the amount of recorded data is exponentially increasing annually. In the meantime, the emergence of the world wide web (WWW), the growth of information and banking systems, and electronic commerce have caused a continuous increase in the volume of data in scientific databases and have created massive warehouses. So, today, there is a need for methods that require minor user interventions and can discover data automatically and extract patterns accurately and quickly to discern logical relationships in these databases. Meanwhile, data mining is one of the most essential methods in this area (3-5).
During the process of data mining, patterns, models, and relationships between different elements in the database are identified while using minimal user intervention. This information is then provided to users, from simple users to managers and policymakers, as well as analysts at a broad level. Moreover, based on this information, important and vital decisions can be made in organizations (6). Data mining technology enables systems to exploit their data capital and use it to support the decision-making process (7). Therefore, to efficiently optimize data management for organizational and social promotion from a broad perspective, research reviews and discussions in the field of data mining have become particularly important (8).
In the field of information science and epistemology, where the library is the most apparent workplace, a vast amount of information and data is produced daily, rising problems with the management of this heavy load of data (9). Computer tools used for this purpose are often only responsible for routine queries and support for management issues and short-term administrative planning. However, diving deep into these data can reveal fascinating patterns and hidden relationships between different parameters. As a result, data mining uncovers hidden information that can be critical for strategic and long-term planning (10).
As mentioned above, data mining is used in many fields, and this application and its close connection with other scientific fields have revealed mutual connections in this field and increased the emergence of high-quality and valuable scientific outputs (11, 12). Since it is necessary to be aware of these mutual patterns and discover new subject areas in the field of data mining, it is important to make progress and improve by illuminating the shadow of knowledge to effectively direct researchers towards elusive domains and stop them from doing repetitive research and rework. On the other hand, the information obtained from data mining will lead to efficient management in organizations, especially in terms of cost and human resource management (13). Today, collecting data on various diseases has an important place in medical sciences, where evaluating the course of diseases and expressing their relationships are among the primary goals. The importance of data mining in this field has become more apparent due to the emergence of integrated systems and the growth of information technology (14, 15). In the present research, the goal was the visualization and analysis of the scientific production map of medical sciences based on the data mining indexed in the Web of Science (WoS) database to determine the position of Iran over the 2008 - 2016 period.
2. Objectives
In the present research, the goal was the visualization and analysis of the scientific production map of medical sciences based on the data mining indexed in the Web of Science (WoS) database to determine the position of Iran over the 2008 - 2016 period.
3. Methods
The present study drew and analyzed the scientific map of the field of data mining using the scientific-metric method and the approach of synonym analysis of words. For this purpose, scientific productions in various fields of medical sciences were scrutinized and analyzed by WoS analytic indicators.
3.1. Research Method
The current research was an applied descriptive study conducted based on the scientometrics method and using the synonym analysis of words approach as one of the analytic methods in scientometrics. In this method, by analyzing the words used in articles and other scientific texts, the intellectual map of the field of data mining is drawn and visualized.
In this research, first, the keywords of the texts were extracted, and synonyms, singular, and plural words were then assimilated. Then, through trial and error, the appropriate threshold was determined, according to which words with acceptable occurrence were selected. Finally, to illustrate and draw a scientific map using the synonym analysis of words, a matrix was designed to draw and analyze the map of scientific productions and clarify the direction of movement and the dynamics of the scientific field of data mining. Using micro measures, the relationships between the components of the scientific map were scrutinized. Furthermore, by analyzing the macro parameters of this scientific map, the characteristics of the scientific network were identified through data mining.
3.2. Statistical Society of Research
The research population included 14,430 records of the scientific outputs of "data mining" indexed in the WoS database between 2008 and 2016.
3.3. Data Collection Tools and Methods
Since the validity and acceptability of any research depend on the validity of its data, we used the citation profile of the science and social science databases of WoS to collect the data relevant to the topic of "data mining". Global validity and acceptability were the main reasons for choosing this database as a reliable platform in terms of the subject matter, time, and credibility, as well as the wide coverage of an enormous volume of scientific publications worldwide.
The data of the current research were collected and analyzed in four steps. In the beginning, after going to the WoS database and making the necessary settings, the term "Data Mining" and its other synonyms were entered in the subject field, and these keywords were combined with the "OR" operator. Then, the period from 2008 to 2016 was selected. Finally, the search was conducted by selecting the profile of medical sciences, leading to the retrieval of 14430 documents. In this regard, the following search strategy was used. Initially, medical subject heading (MeSH) terms for data mining in medicine (“Information Science Category”, “Information Science Informatics”, “Medical Informatics”, “Medical Informatics Applications”, “Information Storage and Retrieval”, and “Data Mining”) were selected and then combined with other key terms (“Medicine” and “Medical”).
In this step, only 500 records were extracted due to the limitations of WoS. Therefore, the recovered records (n = 14430) were extracted in the form of 28 files, each containing 500 records and 430 additional records in plain text, which were saved on a personal computer. Finally, after the completion of data collection, all the files were merged into a separate file named data.txt. Then, the data obtained were entered into VoSviewer software to extract keywords and calculate the frequency of their synonyms. Next, a synonym map of the words was drawn, and different synonym matrices were extracted.
3.4. Analysis of Research Data
In the present research, the synonym word analysis method was used to analyze data and draw a mental (conceptual) map for the field of data mining. The necessary analyses were performed using the outputs of specialized WoS analysis software (i.e., VoSviewer) to answer research questions. The data obtained in this research were analyzed using the micro-indexes of WoS analysis (including the measures of centrality, proximity, and eigenvector), as well as macro-indexes (including density, clustering coefficient, average distance, network diameter, cohesion, and network components).
4. Results
The search retrieved 14430 data mining records registered in the WoS database between 2008 and 2016. Figure 1 shows the growth trend of scientific outputs in the field of data mining during the research period.
The growth trend of the publications related to scientific outputs in the field of data mining in the period of 2008 - 20016 in the Web of Science database
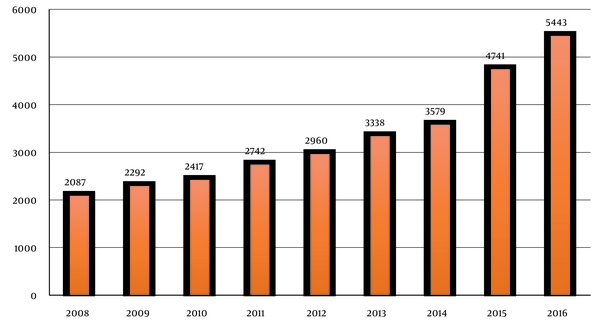
Publications indexed in the WoS database in the field of data mining showed a growing trend in the 9-year period from 2008 to 2016 (Figure 2). This process started with the publication of 2087 documents in 2008, and the number of published documents increased every year thereafter, reaching 5443 records in 2016, showing the highest number of documents registered in the WoS database compared to the number of documents published in 2009 (n = 2292), 2010 (n = 2417), 2011 (n = 2742), 2012 (n = 2960), 2013 (n = 3338), 2014 (n = 3579), and 2015 (n = 4741).
The thesaurus map of words in the domain of data mining in the Web of Science database in the period of 2008 - 2016 (drawn by Voice Viewer software)
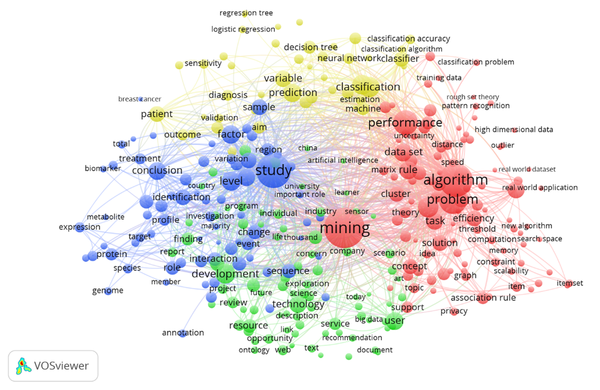
The analysis of 14,430 documents retrieved showed that 12,943 institutes and universities participated in the production and publication of works related to data mining in the investigated database from 2008 to 2016. Table 1 shows the list of these institutions, the number of documents published by each of them, and the percentage share of each of these universities and institutions from the total scientific output in the field of data mining from 2008 to 2016.
List of the Top Ten Institutions and Universities Participating in Publishing Documents on Data Mining in the Web of Science Database from 2008 to 2016
Name of University/Institute | Number of Documents (Percentage from the Total Output) |
---|---|
Chinese Academy of Science | 692 (2.38) |
University of California System | 621 (2.098) |
Center National Research Scientific | 514 (1.737) |
State University System of Florida | 324 (1.095) |
Helmholts Association | 295 (0.99) |
China University of Mining Technology | 281 (0.94) |
University of Texas System | 276 (0.93) |
United States Department of Energy | 269 (0.9) |
Harvard University | 254 (0.85) |
Pennsylvania Commonwealth System of Higher Education | 246 (0.83) |
The Chinese Academy of Sciences, the University of California system, and the National Center for Scientific Research (CNSR) each produced 692, 621, and 514 titles, standing in the first to third ranks, respectively, as the most productive institutions regarding publications on the field of data mining (Table 1).
Regarding the position of Iranian institutions and universities, Islamic Azad University (IAU) ranked 41st among the top universities in the world. The University of Tehran ranked 94th in the world by producing 105 documents in the field of data mining, followed by Iran University of Science and Technology (ranking 200th by producing 66 degrees) and Isfahan University of Technology (ranking 492nd with 33 degrees). The noteworthy point was that the Islamic Azad University and the University of Tehran were able to surpass Oxford University regarding WoS publications in the field of data mining in the period of 2008 - 2016.
A review of 14,430 documents published on data mining in the WoS database in 2008 - 2016 showed that this record number was produced by 209 countries. Table 2 shows the list of the top countries and the position of Iran among them.
Top Countries Regarding the Number of Publications on the Data Mining Field in the Web of Science Database from 2008 to 2016 and the Position of Iran
Countries | Number of Outputs (Percentage from the Total Output) |
---|---|
USA | 8123 (27.4) |
China | 4865 (16.4) |
UK | 1829 (17.6) |
Australia | 1745 (8.5) |
Germany | 1704 (7.5) |
Canada | 1372 (6.4) |
Spain | 1341 (5.4) |
Taiwan | 1217 (1.4) |
Italy | 1216 (1.4) |
Franc | 1172 (9.3) |
South Korea | 925 (1.3) |
Japan | 828 (7.2) |
Iran | 678 (2.2) |
Poland | 658 (2.2) |
Brazil | 646 (2.1) |
Nederland | 568 (1.9) |
Turkey | 567 (1.9) |
Belgium | 496 (1.9) |
The United States, China, and the United Kingdom (UK) claimed the first to third ranks by producing 8123, 4865, and 1829 records, respectively. The position of other countries up to the 14th rank has also been specified in Table 2. Regarding the position of Iran, with the production of 678 records, our country ranked 13th among 209 countries that have contributed the most in the production of data mining publications, which seems to be an acceptable position. By obtaining this rank, Iran has been able to gain a better rank than countries such as Poland, Brazil, the Netherlands, Turkey, and Belgium, all of which have been seated in a lower position compared to Iran. Among Asian countries, after China, Taiwan, South Korea, and Japan, Iran ranked fifth, indicating a better and more successful performance than other Asian countries in terms of the number of scientific productions in the field of data mining.
5. Discussion
The analysis of the findings showed that the publication of scientific resources on the topic of data mining in the nine years of the research period had a growing trend, and the number of documents registered in the WoS database increased year by year. The lowest number of data mining documents were registered in this database in 2008. Also, the highest number of documents registered in WoS was in 2016.
In terms of the frequency of publications on the topic of data mining, the findings of this research are in line with the opinion of Larose (16), who stated that data mining would face revolutionary development in the coming decades. Moreover, it can be said that we are now in the development era of data mining, and the proof of this claim is the continuous growth of scientific outputs observed in this field in the nine years investigated. Since the growth rate of publications on data mining has been uniform in recent years, it is expected that this growth will continue in the coming years (17).
Considering that the emergence of data mining dates back to the late 1980s, researchers are now interested in new and up-to-date topics, encouraging them to refer to more deeply investigating data mining (18, 19). In addition, given that data mining can be used wherever there is data, it can be considered an interdisciplinary field (20). In this way, researchers from different fields can conduct data mining-related research with specific goals (21). On the other hand, because this field is closely related to technology, it can be introduced to the world day-by-day more than in the past, and it will probably be used with new and diverse purposes in various research areas (22).
As the findings showed, 12943 institutes and universities participated in the conduct and publication of research works related to data mining. The analysis of the scientific outputs published by these institutions and universities showed that the Chinese Academy of Sciences, the University of California, and the National Center for Scientific Research claimed the first to third ranks of the most productive institutions in publishing works on the topic of data mining.
Regarding the position of Iranian institutions and universities, this research showed that the Islamic Azad University, University of Tehran, and Iran University of Science and Technology were the most successful Iranian universities in terms of the quantitative production of scientific documents on the subject of data mining in the WoS database during 2008 - 2016. Also, our review of 14,430 documents published on data mining in the WoS database in this period showed that this record number was produced by 209 countries, among which China, the United States, and the UK attained the first to third ranks by producing 8123, 4865, and 1829 documents, respectively. Regarding Iran's position, our country was ranked 13th, which seems to be an acceptable position. From this point of view, Iran has been able to be placed in a higher position than countries such as the Netherlands, Russia, Turkey, and Sweden. In Asia, Iran ranked fifth after China, Taiwan, South Korea, and Japan. The recognition of Iran as a successful country in the field of data mining should encourage researchers from other countries to communicate with Iranian scientists. This can provide a basis for acquiring new information and experiences and opening new horizons.
Our investigations showed that this network consisted of 19 separate clusters, the main three of which included more factors than other clusters. Accordingly, the largest cluster had 19 authors; the second cluster was the result of the collaboration of 12 authors, and the third cluster consisted of 10 researchers. The analysis of existing relationships in this network showed that all communications were bi-directional. Accordingly, it can be noted that the expansion of this network has been the result of communication between renowned authors already existing in the network or the debut of new researchers.
Based on our findings, the lexical map of the scientific output of data mining publications consisted of four clusters, the largest of which had 108 words. The second, third, and fourth clusters contained 82, 73, and 42 words, respectively. The clusters present in the lexical synonym network of the data mining field were named as follows: Cluster no. 1: Data mining, cluster no. 2: Research topics in the data mining field, cluster no. 3: Technology in data mining, and cluster no. 4: Classification in data mining. Examining these four clusters showed that cluster no. 1, dealing with the nature of data mining, was the largest cluster with 108 words. The smallest cluster, with 42 words, was cluster no. 4, which dealt with classification in the field of data mining. Overall, it can be stated that the nature of data mining has been the subject of more research, generating a cluster larger than other subjects. Therefore, researchers who wish to conduct research in modern data mining fields can use the keywords introduced in these clusters to identify the main fields connected with these terms.
5.1. Conclusions
It can be concluded that the most important and credible keywords in the field of data mining in terms of frequency include exploration, technology, research, classifier, data set, error, experiment, mechanism, and prediction. It should be noted that word exploration, frequency, data set, error, and test have the most contiguity and connection with other words in this field. According to our findings, Iranian authors gained an acceptable position in the international ranking system, so it is suggested that Iranian researchers increase their scientific cooperation with top researchers and universities worldwide to improve the scientific position and qualifications of not only their own but also their universities and institutions in the field of data mining.
References
-
1.
Yu YC, Zhang W, O'Gara D, Li JS, Chang SH. A moment kernel machine for clinical data mining to inform medical decision making. Sci Rep. 2023;13(1):10459. [PubMed ID: 37380721]. [PubMed Central ID: PMC10307844]. https://doi.org/10.1038/s41598-023-36752-7.
-
2.
Wu WT, Li YJ, Feng AZ, Li L, Huang T, Xu AD, et al. Data mining in clinical big data: the frequently used databases, steps, and methodological models. Mil Med Res. 2021;8(1):44. [PubMed ID: 34380547]. [PubMed Central ID: PMC8356424]. https://doi.org/10.1186/s40779-021-00338-z.
-
3.
Herland M, Khoshgoftaar TM, Wald R. A review of data mining using big data in health informatics. J Big Data. 2014;1(1):2. https://doi.org/10.1186/2196-1115-1-2.
-
4.
Zia A, Aziz M, Popa I, Khan SA, Hamedani AF, Asif AR. Artificial Intelligence-Based Medical Data Mining. J Pers Med. 2022;12(9):1359. [PubMed ID: 36143144]. [PubMed Central ID: PMC9501106]. https://doi.org/10.3390/jpm12091359.
-
5.
Hong M, Jacobucci R, Lubke G. Deductive data mining. Psychol Methods. 2020;25(6):691-707. [PubMed ID: 31916800]. https://doi.org/10.1037/met0000252.
-
6.
Alonso SG, de la Torre-Diez I, Hamrioui S, Lopez-Coronado M, Barreno DC, Nozaleda LM, et al. Data Mining Algorithms and Techniques in Mental Health: A Systematic Review. J Med Syst. 2018;42(9):161. [PubMed ID: 30030644]. https://doi.org/10.1007/s10916-018-1018-2.
-
7.
Wu C, Kao SC, Shih CH, Kan MH. Open data mining for Taiwan's dengue epidemic. Acta Trop. 2018;183:1-7. [PubMed ID: 29549012]. https://doi.org/10.1016/j.actatropica.2018.03.017.
-
8.
Chen LA, Fawcett TN. Using Data Mining Strategies in Clinical Decision Making: A Literature Review. Comput Inform Nurs. 2016;34(10):448-54. [PubMed ID: 27532327]. https://doi.org/10.1097/CIN.0000000000000282.
-
9.
Groenhof TKJ, Koers LR, Blasse E, de Groot M, Grobbee DE, Bots ML, et al. Data mining information from electronic health records produced high yield and accuracy for current smoking status. J Clin Epidemiol. 2020;118:100-6. [PubMed ID: 31730918]. https://doi.org/10.1016/j.jclinepi.2019.11.006.
-
10.
Izdparst SM, Vahdat D. [Data mining and its application in libraries and educational institutions]. Scientific Communication Monthly. 2009;12(2). Persian.
-
11.
Gholamhosseini L, Damroodi M. [Evaluation of Data Mining Applications in the Health System]. Paramedical Sciences and Military Health. 2015;10(1):39-48. Persian.
-
12.
Lan K, Wang DT, Fong S, Liu LS, Wong KKL, Dey N. A Survey of Data Mining and Deep Learning in Bioinformatics. J Med Syst. 2018;42(8):139. [PubMed ID: 29956014]. https://doi.org/10.1007/s10916-018-1003-9.
-
13.
Iddamalgoda L, Das PS, Aponso A, Sundararajan VS, Suravajhala P, Valadi JK. Data Mining and Pattern Recognition Models for Identifying Inherited Diseases: Challenges and Implications. Front Genet. 2016;7:136. [PubMed ID: 27559342]. [PubMed Central ID: PMC4979376]. https://doi.org/10.3389/fgene.2016.00136.
-
14.
Quesado I, Duarte J, Silva A, Manuel M, Quintas C. Data Mining Models for Automatic Problem Identification in Intensive Medicine. Procedia Comput Sci. 2022;210:218-23. [PubMed ID: 36406201]. [PubMed Central ID: PMC9659707]. https://doi.org/10.1016/j.procs.2022.10.140.
-
15.
Huang Z, Juarez JM, Li X. Data Mining for Biomedicine and Healthcare. J Healthc Eng. 2017;2017:7107629. [PubMed ID: 29065638]. [PubMed Central ID: PMC5585672]. https://doi.org/10.1155/2017/7107629.
-
16.
Larose DT. Discovering Knowledge in Data: An Introduction to Data Mining. Hoboken, NJ: John Wiley & Sons; 2005.
-
17.
Obstfeld AE, Patel K, Boyd JC, Drees J, Holmes DT, Ioannidis JPA, et al. Data Mining Approaches to Reference Interval Studies. Clin Chem. 2021;67(9):1175-81. [PubMed ID: 34402506]. https://doi.org/10.1093/clinchem/hvab137.
-
18.
Gauthier J, Vincent AT, Charette SJ, Derome N. A brief history of bioinformatics. Brief Bioinform. 2019;20(6):1981-96. [PubMed ID: 30084940]. https://doi.org/10.1093/bib/bby063.
-
19.
Wrona RM. Medical data mining: The search for knowledge in workers' compensation claims. Am J Ind Med. 2019;62(9):729-32. [PubMed ID: 31209908]. https://doi.org/10.1002/ajim.22990.
-
20.
Joudaki H, Rashidian A, Minaei-Bidgoli B, Mahmoodi M, Geraili B, Nasiri M, et al. Using data mining to detect health care fraud and abuse: a review of literature. Glob J Health Sci. 2014;7(1):194-202. [PubMed ID: 25560347]. [PubMed Central ID: PMC4796421]. https://doi.org/10.5539/gjhs.v7n1p194.
-
21.
Bellazzi R, Diomidous M, Sarkar IN, Takabayashi K, Ziegler A, McCray AT. Data analysis and data mining: current issues in biomedical informatics. Methods Inf Med. 2011;50(6):536-44. [PubMed ID: 22146916]. [PubMed Central ID: PMC3233983]. https://doi.org/10.3414/ME11-06-0002.
-
22.
Gil J, Marques-Pamies M, Sampedro M, Webb SM, Serra G, Salinas I, et al. Data mining analyses for precision medicine in acromegaly: a proof of concept. Sci Rep. 2022;12(1):8979. [PubMed ID: 35643771]. [PubMed Central ID: PMC9148300]. https://doi.org/10.1038/s41598-022-12955-2.