1. Background
Oil refineries, especially sulfur recovery units (SRU), are exposed to various types of hazardous events and risks in daily operations, including process risks, risks due to human error (HE), toxicity and mechanical failures, material release, and fire and explosion risks. Thus, it is very important to identify hazardous events and risks, perform risk assessments, and take proper interventions to mitigate hazardous events and risks (1, 2).
Risk assessment with FTA is a method for calculating the occurrence probability of hazardous events or system failure. The FTA is an up-down and deductive approach, by which an undesirable hazardous event, referred to as the top event (TE), is graphically decomposed to possible intermediate and basic causes in increasing detail to determine the causes or combinations of causes of TE. Fault tree provides a graphical approach for determining how failures could occur both qualitatively and quantitatively (3).
Given that the precise and relevant failure rate of BEs, normally required for the quantitative phase of conventional FTA, are not available for process industries, expert judgments are often suggested to assess failure occurrence probability of BEs. Unfortunately, expert judgments are afflicted with qualitative and subjective uncertainties (4, 5). The concept of fuzzy set or fuzzy logic was first proposed by Zadeh (1965) to solve the subjective and qualitative uncertainties of real engineering problems. Fuzzy logic uses possibility distributions to represent expert judgments (6-8).
Fuzzy sets and fuzzy membership functions have been applied for calculating the occurrence probability of hazardous events where relevant failure data of BE are not available. The FTA using fuzzy membership functions was first suggested by Tanaka et al. (1983), as a comprehensive method to solve problems, where exact failure occurrence probabilities of BE are not available (9). Another study of FTA, based on fuzzy sets, was performed by Shi et al. (2014). They suggested an algorithm using Fuzzy FTA (FFTA) and Fuzzy Analytical Hierarchy Process (FAHP) in order to perform risk assessment during a fire and explosion accident for steel oil storage tanks in petroleum industries (5). Rajakarunakaran et al. (2015) showed that expert judgments in the form of qualitative FPDs are appropriate for FTA when relevant failure rate data are not available (6). In addition to the above studies, Mohsendokht (2017) established a model to assess the uranium hexafluoride release from a uranium conversion plant by using fuzzy set theory, possibility concept, and the conventional approach (4). All the studies mentioned above, represent the ability of expert subjective judgment based on fuzzy set theory for solving the lack of data problem in risk and safety assessment studies.
The application of fuzzy-based approach overcomes the limitation of the risk assessment by conventional FTA of the Claus furnace package of SRU by taking expert subjective judgments to assess process failures occurrence probabilities.
2. Objectives
This research aimed at assessing failure occurrence probability of hazardous events based on fuzzy logic, expert judgment, and FTA in lack of data conditions.
3. Methods
The study was carried out at a petroleum refinery during June and December 2016. In the current study, FTA according to fuzzy-based and conventional approaches was applied to assess the failure of burner shutdown in the SRU. The overall framework of the study is shown in Figure 1.
3.1. Fault Tree Construction Based on Risk Identification
In this step, a critical hazardous event in the SRU is first initiated, called the TE of the fault tree. To construct the fault tree, it is essential to identify hazardous fault events i.e., BEs, intermediate events (IEs), and TE. The logic gates, such as “AND” and “OR” gates, could be used to connect these fault events in the fault tree diagram. The “OR” gate shows that the output event (i.e. TE or IE) occurs if any of the input events (i.e. BEs or IEs) occur. The “AND” gate shows that the output event (i.e. TE or IE) occurs only when all the input events (i.e. BEs or IEs) occur at the same time. The goal of risk identification is to enable identification of all hazardous fault events associated with SRU at different hazardous event levels, e.g. from TE level to IE and BE levels with a view to construct a fault tree diagram. Moreover, construction of a fault tree helps find its cut sets (CSs) while its quantitative analysis calculates the failure occurrence probability of TE using its BEs’ failure occurrence probabilities. A cut set is a set of BEs, whose simultaneous existence involves the occurrence of the TE, and which is independent of the occurrence of the other BEs of the fault tree (3, 6).
3.2. Failure Probabilities of BEs Calculation Based on the Conventional Approach
In the conventional approach, the failure rates of components (or BE) are required to calculate the failure occurrence probabilities of BEs. In this approach, failure probability functions can be used to transform the failure rates of BEs to their failure occurrence probabilities. There are 4 major types of component failure probability functions for calculating the failure occurrence probabilities of BE, which include constant failure probability (failure per demand), non-repairable components, repairable components with revealed failure mode, and repairable components with the periodically proof test (3, 4). For non-repairable components, the failure occurrence probability of BEs can be calculated using Equation 1.

Where λ is the failure rate of BE (number of failures per hour); t is mission time.
Moreover, the BE failure occurrence probability of repairable components could be calculated using Equation 2.
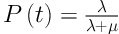
Where μ is the repair rate of BE (number of repairs per hour).
3.3. Failure Probabilities of BEs Calculation Through the Fuzzy Logic Approach
The steps of fuzzy logic approach or fuzzy-based approach are described as follows.
Step 1: Expert weighting evaluation
The first step of the fuzzy-based approach is to select experts and expert weighting evaluation. To perform the FTA based on expert judgments, a group of experts based on Cooke et al. (2008) indicators need to be assembled and selected (10). In some cases, the credibility of experts based on various criteria, such as title (professional position), age, experience level, and educational qualification, is widely different. Therefore, this study considered the relative weighting factor of each expert from 0 to 1. The expert criteria and their corresponding scores are shown in Table 1. For expert j, the relative weighting factor could be calculated using Equation 3 (6, 11):
Row | Constitution | Classification | Score |
---|---|---|---|
1 | Title | Chief Engineer | 5 |
Supervisor | 4 | ||
Engineer | 3 | ||
Technician | 2 | ||
Operator | 1 | ||
2 | Experience | Greater than 30 | 5 |
20 - 30 | 4 | ||
10 - 19 | 3 | ||
6 - 9 | 2 | ||
Less than 6 | 1 | ||
3 | Educational qualification | PHD | 5 |
Master | 4 | ||
Bachelor | 3 | ||
HND | 2 | ||
School level | 1 | ||
4 | Age | Greater than 50 | 4 |
4 | |||
40 - 50 | 3 | ||
30 - 39 | 2 | ||
Less than 30 | 1 |
Expert Criteria and Weighting Scores

Where pj is the sum of scores of expert j and n is the number of experts.
Step 2: Select the FPD and their corresponding fuzzy membership functions
In this study, the FPD was used to describe the relationship between an expert subjective judgment (linguistic variable) and its fuzzy membership function value, which is applied in the expert subjective evaluation process (4, 5). This FPD is used to represent failure possibilities of BEs. Based on Miller’s study, the proposed FPD includes 6 linguistic variables, namely, very low (VL), low (L), fairly low (FL), fairly high (FH), High (H), and very high (VH). Based on Chen-Hwang scales (12, 13), the fuzzy triangular membership functions μà (r1, r2, r3) for VL, L, FL, FH, H, and VH are presented in Equations 4 to 9.

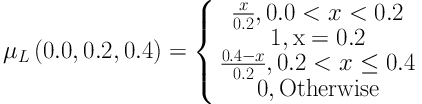
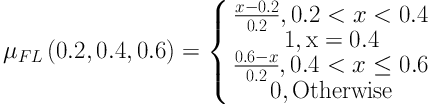
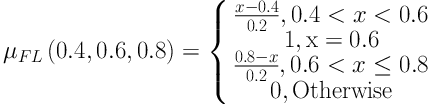
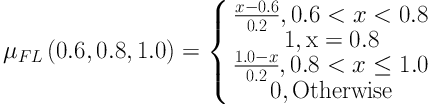

Step 3: Expert subjective evaluation process
The purpose of this evaluation was to gather the qualitative failure possibility of a BE from a group of experts using interviews. Using the linguistic variables defined in Equations 4 to 9, each expert in the risk assessment team provided their subjective judgment of the failure possibility of each selected BE.
Step 4: Aggregating the fuzzy failure possibilities obtained
This step was performed to aggregate different failure possibilities obtained from experts. The sum-product method could be used to aggregate different fuzzy failure possibilities of a BE and it is expressed by the following Equation (5):

Where μAggregated (x) is the aggregated fuzzy failure possibilities score (AFFPS), νj represents a relative weighting factor of expert j and μij is the fuzzy triangular membership function i assigned by expert j. k is the number of fuzzy membership functions and n is the number of experts.
Step 5: De-fuzzifying the aggregated fuzzy failure possibilities obtained
The next step of fuzzy logic approach is to de-fuzzify aggregated fuzzy failure possibilities score (AFFPS) to Crisp Failure Possibilities Score (CFPS). Area De-fuzzification technique (ADT) method is the defuzzification process of the AFFPS to a CFPS. The ADT method for a fuzzy triangular membership function μA ̃(r1, r2, r3) is described as follows (14):

Where x* is a defuzzified output equal to CFPS.
Step 6: Converting the crisp failure possibilities to failure probabilities of BE
To generate failure occurrence probabilities of BE, the CFPS values must be converted to Failure Probability (FP) values. The conversion function could be done by Onisawa logarithmic function, which is described as follows (11, 14):

3.4. Calculating the Occurrence Probability of TE
In the current study, based on the failure occurrence probability of BEs, the occurrence probability of a CS was calculated using Equation 13. Also, Equation 14 was used to calculate the occurrence probability of the TE (5, 6).


Where Q(TE) is the occurrence probability of the TE, Q(CSi) is the occurrence probability of cut set i, n is the number of CSs, and m is the number of BEs in the largest CS.
3.5. BEs and CSs Importance Measure Analysis
In this study, importance measure (IM) analysis was performed to assess the contribution of each BE to the TE occurrence and to identify the critical weak points of the burner H-901. The importance measure of BEs can be calculated using the Fussell-Vesely (F-V) equation (3, 4):

Where Q(CSi)j is the occurrence probability of the cut set i containing basic event j, Q(TE\BEj = 0) is the occurrence probability of TE by setting the occurrence probability of basic event j to 0, and nj is the total number of the CSs containing basic event j.
4. Results
4.1. Case Study Description
An SRU recovers sulfur that has to be removed from acid gas (hydrogen sulfide) in oil and gas refineries. In terms of sulfur removal efficiency and safety, the burner of the Claus furnace package is highly critical and important in the SRU. The duty of this stage is to add the quantity of oxygen (combustion process air) required for the Claus reaction and to remove the heat resulting from the reaction between the oxygen and the combustibles in the sour/acid gas. In the operation phase, the Fuel Gas (FLG) flow is needed for continuous firing operation in burner H-901. When the temperature is over the minimum set point (about 900°C), the process Acid Gas (ACG) can flow to the combustion chamber. An air blower is provided for combustion process air (PRA).
4.2. Fault Tree Construction
Based on the brainstorming approach, FMEA, and HAZOP, some important undesired TEs were identified, such as burner H-901 shutdown, gas release, fire, and explosion. In this research, there were 3 major reasons for selecting the shutdown scenario of the burner H-901 due to no flow of feeds (feed = process air + acid gas + fuel gas):
If shutdown scenario of the burner happens, toxic release, fire, and explosion accidents can easily occur.
The various existing shutdown factors for burner of the Claus furnace package process.
The destruction efficiency of the sour/acid gas is more than 65%.
At this stage, according to HAZOP and FMEA, the fault tree was constructed. The fault tree of the burner H-901 shutdown was constructed and is shown in Figure 2.
In qualitative analysis of FTA, the CSs of the fault tree for burner H-901 shutdown scenario were obtained by applying the Top-Down algorithm. In quantitative analysis, the BEs and CSs probabilities are very important for calculating the TE occurrence probability.
4.3. Calculating Failure Occurrence Probabilities of BEs Through the Fuzzy Logic Approach
Due to lack of failure data, an expert investigation for the failure occurrence probability of BEs of the burner H-901 shutdown was performed by interviewing a total of 4 experts, including a supervisor, chief engineer, process design, and operation personnel of SRU. First, using Equations 3, the relative weighting factors of 4 experts based on Table 1 was calculated and presented in Table 2. Next, using Equations 4 to 9 and interviews with experts, the failure possibilities for all BE were obtained in Table 3. Then, the AFFPSs for 32 BE were calculated using Equation 10 and presented in Table 3. Thereafter, the AFFPSs for all BEs in Table 3 were defuzzified using Equation 11 and presented in Table 4. Finally, using the Onisawa function in Equation 12, the failure occurrence probability for all BEs were calculated and presented in Table 4.
Experts | Title | Education | Experience | Age | Scores | Weight |
---|---|---|---|---|---|---|
E1 | Supervisor | Bachelor | 20 - 30 | 40 - 50 | 14 | 0.291667 |
E2 | Chief engineer | Master | 10 - 19 | 30 - 39 | 14 | 0.291667 |
E3 | Technician | Master | 6 - 9 | 30 - 39 | 10 | 0.208333 |
E4 | Engineer | Master | Less than 6 | 30 - 39 | 10 | 0.208333 |
Sum = 48 | Sum = 1 |
Relative Weighting Factors for 4 Experts
BEs | Failure Mode Description | Judgments of Experts | AFFPS | |||
---|---|---|---|---|---|---|
X1 | No flow of fuel gas from upstream | VL | L | L | VL | (0.00,0.10,0.30) |
X2 | PV-09717 fail closed | FL | FL | H | L | (0.24,0.44,0.64) |
X3 | FLG-4 pipe blockage | L | L | L | L | (0.00,0.20,0.40) |
X4 | FLG-2 pipe blockage | L | FH | FL | L | (0.16,0.36,0.56) |
X5 | Demister on V-908 blockage due to fouling | L | FL | FL | L | (0.10,0.30,0.50) |
X6 | XV-09105 fail closed | FL | FL | H | L | (0.24,0.44,0.64) |
X7 | XV-09107 fail closed | FL | FL | H | L | (0.24,0.44,0.64) |
X8 | FV-09115 fail closed | FL | FL | H | L | (0.24,0.44,0.64) |
X9 | Check valve on FLG-2 pipe plugging | L | L | L | L | (0.00,0.20,0.40) |
X10 | Check valve on FLG-4 pipe plugging | L | L | L | L | (0.00,0.20,0.40) |
X11 | Manual valve on FLG-2 pipe closure (HE) | VL | L | FL | FL | (0.08,0.23,0.43) |
X12 | Manual valve on FLG-2 plugging/blockage | L | L | L | L | (0.00,0.20,0.40) |
X13 | No flow of acid gas from upstream | FH | H | VH | FH | (0.54,0.74,0.90) |
X14 | ACG-10 pipe Blockage | L | L | FL | VL | (0.04,0.20,0.40) |
X15 | ACG-12 pipe Blockage | L | L | FL | VL | (0.04,0.20,0.40) |
X16 | Demister on V-902 blockage due to fouling | FH | FH | FL | FL | (0.32,0.52,0.72) |
X17 | FV-09111 fail closed | FH | FH | H | L | (0.36,0.56,0.76) |
X18 | XV-09114 fail closed | FH | FH | H | L | (0.36,0.56,0.76) |
X19 | Manual valve on ACG-12 pipe closure (HE) | VL | L | L | L | (0.00,0.14,0.34) |
X20 | Manual valve on ACG-12 plugging/blockage | L | L | L | VL | (0.00,0.15,0.36) |
X21 | Air pre-heater (E-905) blockage | VL | L | FL | VL | (0.04,0.14,0.34) |
X22 | XV-09101 fail closed | FL | FL | H | L | (0.24,0.44,0.64) |
X23 | FV-09113 fail closed | FL | FL | H | L | (0.24,0.44,0.64) |
X24 | FV-09114 fail closed | FL | FL | H | L | (0.24,0.44,0.64) |
X25 | PRA-20 pipe redundant section blockage | VL | L | VL | VL | (0.00,0.06,0.26) |
X26 | PRA-8 pipe blockage | L | FL | L | L | (0.06,0.26,0.46) |
X27 | Blower C-901 fail while running | H | H | VH | H | (0.64,0.84,1.00) |
X28 | PRA-20 pipe blockage | VL | L | VL | VL | (0.00,0.06,0.26) |
X29 | PRA-24 pipe blockage | VL | L | VL | VL | (0.00,0.06,0.26) |
X30 | Check valve on PRA-20 pipe plugging | VL | L | VL | VL | (0.00,0.06,0.26) |
X31 | Manual valve on PRA-20 pipe closure (HE) | VL | VL | VL | VL | (0.00,0.00,0.20) |
X32 | Manual valve on PRA-20 plugging/blockage | VL | L | VL | VL | (0.00,0.06,0.26) |
Expert Subjective Evaluation and Fuzzy Aggregation Process
BEs | CFPS | Fuzzy (FP) | F-V IM | Rank | Conventional (FP) |
---|---|---|---|---|---|
X1 | 0.022 | 7.54E-09 | 7.93E-09 | 18 | 3.04E-06 |
X2 | 0.114 | 2.76E-05 | 2.90E-05 | 5 | 5.96E-05 |
X3 | 0.033 | 8.54E-08 | 8.98E-08 | 15 | 4.96E-09 |
X4 | 0.086 | 8.78E-06 | 9.24E-06 | 8 | 1.34E-08 |
X5 | 0.067 | 2.85E-06 | 3.00E-06 | 10 | 1.86E-05 |
X6 | 0.114 | 2.76E-05 | 2.90E-05 | 5 | 5.76E-05 |
X7 | 0.114 | 2.76E-05 | 2.90E-05 | 5 | 5.76E-05 |
X8 | 0.114 | 2.76E-05 | 2.90E-05 | 5 | 3.90E-05 |
X9 | 0.033 | 8.54E-08 | 8.98E-08 | 15 | 3.86E-05 |
X10 | 0.033 | 8.54E-08 | 8.98E-08 | 15 | 3.86E-05 |
X11 | 0.055 | 1.12E-06 | 1.18E-06 | 13 | 4.00E-06 |
X12 | 0.033 | 8.54E-08 | 8.98E-08 | 15 | 1.20E-05 |
X13 | 0.212 | 2.71E-04 | 7.03E-05 | 4 | - |
X14 | 0.053 | 3.21E-07 | 8.34E-08 | 16 | 2.67E-09 |
X15 | 0.053 | 3.21E-07 | 8.34E-08 | 16 | 1.11E-09 |
X16 | 0.125 | 5.93E-05 | 1.54E-05 | 7 | 1.86E-05 |
X17 | 0.153 | 8.46E-05 | 2.20E-05 | 6 | 1.29E-04 |
X18 | 0.153 | 8.46E-05 | 2.20E-05 | 6 | 6.97E-05 |
X19 | 0.027 | 2.43E-08 | 6.32E-09 | 20 | 4.00E-06 |
X20 | 0.029 | 3.62E-08 | 9.39E-09 | 19 | 1.20E-05 |
X21 | 0.036 | 1.33E-07 | 2.80E-04 | 3 | 4.99E-02 |
X22 | 0.114 | 2.76E-05 | 5.80E-02 | 2 | 3.92E-02 |
X23 | 0.114 | 2.76E-05 | 1.66E-06 | 11 | 2.67E-05 |
X24 | 0.114 | 2.76E-05 | 1.60E-06 | 12 | 2.67E-05 |
X25 | 0.018 | 1.61E-09 | 9.64E-11 | 21 | 1.34E-09 |
X26 | 0.053 | 9.46E-07 | 5.49E-08 | 17 | 1.77E-09 |
X27 | 0.245 | 4.48E-04 | 9.42E-01 | 1 | 7.96E-02 |
X28 | 0.018 | 1.61E-09 | 3.38E-06 | 9 | 2.60E-06 |
X29 | 0.018 | 1.61E-09 | 3.38E-06 | 9 | 2.18E-07 |
X30 | 0.018 | 1.61E-09 | 3.38E-06 | 9 | - |
X31 | 0.011 | 5.35E-11 | 1.12E-07 | 14 | - |
X32 | 0.018 | 1.61E-09 | 3.38E-06 | 9 | - |
BEs Failure Occurrence Probability and Their F-V Importance Measures
4.4. Calculating Failure Occurrence Probabilities of BEs Through the Conventional Approach
OREDA (2002) and Lees (2004) are the main two sources used for assessment of the reliability of data for the conventional approach (3, 15). In the conventional approach, the failure occurrence probabilities of all BEs based on Equations 1 were calculated and presented in Table 4. Due to lack of failure data, the failure occurrence probability for some of the BE could not be calculated, such as the BEs X13, X30, X31, and X32.
4.5. Calculating Occurrence Probability of CSs and TE
Based on the fuzzy-based approach, the occurrence probabilities for 108 CSs were calculated using Equation 13. By assigning the occurrence probabilities of CSs, the occurrence probability of TE was 4.76 E-04 per year, which was calculated using Equation 14. In the conventional approach, it is not possible to calculate the TE and CSs occurrence probabilities, because of the lack of BEs failure data.
4.6. BEs importance Analysis
Equation 15 has been applied to rank the BE based on the F-V importance measure. Table 4 represents the F-V importance analysis results for 32 BE of the fault tree. Basic events F-V importance analysis revealed that process failures, such as X27 (blower C-901 fail while running), X22 (shut-off valve XV-09101 fail close), and X21 (air pre-heater E-905 blockage) play a very important role in burner shutdown occurrence.
5. Discussion
Results from Table 4 showed that failure probabilities of the 4 BE were not available in the conventional approach. These results are consistent with the findings of Shi et al. (2014) and Mohsendokht (2017) on the weakness of the conventional approach in calculating some of the BE in lack of data situations (4, 5). Moreover, the failure occurrence probabilities for some of the BE, such as the X3, X4, X14, X15, X25, X26, X28, and X29, were afflicted with high uncertainty levels. The main causes of this uncertainty were application of irrelevant and low confidence failure data to calculate the failure occurrence probability of BEs. Based on the findings mentioned above, the proposed fuzzy-based approach was required to calculate the failure occurrence probability of BE in this study.
Results of the studies of Omidvari et al. (2014) and Mohsendokht (2017) showed that the process HAZOP was useful for identifying process failures in the fault tree construction phase. However, it cannot be used to identify some of the hazardous events, such as human errors and some of the mechanical failures (4, 11). Therefore, the current study developed a combined HAZOP, brainstorming, and FMEA model to overcome the weakness of process HAZOP to identify the different hazardous events associated with TE. The results revealed that the proposed model is able to identify mechanical and process failures and human errors.
It was observed from importance analysis that process failures were ranked first in contributing to the TE occurrence in the current study, while results in Mohsendokht (2017) showed that a set of process failures and human errors caused TE occurrence (4). Results from Omidvari et al.’s study (2014) showed that a type of process failure, i.e. the obstruction of catalyst had the highest importance measure in fault tree (11). All findings mentioned above, revealed that process failures play a very important role in TEs occurrence for process hazardous scenarios.
Komal et al. (2015) proposed a new fuzzy approach to calculate the failure occurrence probability of BEs based on failure probability function fuzzy parameters in the reliability and risk studies. In their approach, Komal et al. (2015) developed a fuzzy method for computing various failure probability function fuzzy parameters, namely failure rate, repair rate, mean time to repair (MTTR), and expected number of failures (ENOF), for any process industry (16). In this study, fuzzy parameters were used to calculate the failure occurrence probabilities of BEs using the fuzzy lambda-tau method, while FPDs were used to calculate the BEs failure occurrence probability in the current research. Based on the findings mentioned above, in order to calculate the failure occurrence probability of BEs, a fuzzy-based approach using fuzzy failure and repair rate could be used in future studies.
6. Conclusions
In this study, lack of failure data for quantitative phase of conventional FTA was a major issue in the Claus section of SRU. To overcome lack of data, the fuzzy-based approach was applied to calculate the failure occurrence probability of BEs in this study. The main advantage of using FPDs in this study was that the proposed approach could directly express failure occurrence probability of each BE. Also, the results revealed that the proposed approach could eliminate the limitation of conventional FTA by using fuzzy logic.