Abstract
Context:
Psoriatic arthritis (PsA), a seronegative, irreversible inflammatory arthritis, displays a heterogeneous clinical presentation that frequently impedes early detection of PsA.Objectives:
Since extensive inflammatory and musculoskeletal damage makes early therapeutic intervention quite challenging, this study was conducted to include and review all available markers, with an emphasis on upcoming reliable biomarkers for the early and timely prediction of possible transition to PsA in patients with cutaneous psoriasis (PsO).Methods:
A thorough literature search was performed using databases such as PubMed, SCOPUS, Embase, and Google Scholar. The aim was to review the latest research on clinical, radiological, serological, and biochemical predictors that could help in the early and timely recognition of the possible transition to PsA in patients with cutaneous PsO.Results:
Early detection and monitoring for developing PsA have relied mostly on the evaluation of clinical features of disease, the genetic profile of patients, serial imaging, and a few serological markers. Recent research focuses on employing a multi-omic approach for the quick diagnosis of at-risk patients by scanning their genetic, protein, lipid, and metabolite profiles. Digital technology is the latest approach to evaluate early PsA with high accuracy and precision. It includes machine learning algorithms, specific immune profiles, and neural networks to decode distinct PsA phenotypes in psoriatic patients with a higher likelihood of developing arthritic features.Conclusions:
Early identification of signs and symptoms will enable dermatologists to timely refer at-risk patients to rheumatologists, thus preventing irreversible joint damage. In the absence of a single specific marker, efforts should be made to adopt a holistic approach for early screening of PsA patients and initiating definitive treatment as early as possible. This intervention is of critical value since the prevention/reduction of PsA development is now being suggested as a new clinical endpoint in PsO trials.Keywords
Psoriatic Arthritis Cutaneous Psoriasis Indicators Early Multiomics
1. Context
Psoriatic arthritis (PsA) is a chronic immune-mediated disease with a variable prevalence of 0.05% to 0.25% in the general population and 6% to 42% in psoriasis (PsO) patients (1-7). This seronegative, irreversible inflammatory arthritis displays a broad clinical spectrum, ranging from mild oligoarthritis to mutilating polyarticular forms, along with enthesitis and dactylitis (4, 8). Such heterogeneous clinical presentation frequently impedes the early detection of PsA. The shift in the site of disease activity from the skin to the joints (early or developing PsA) is punctuated by mildly symptomatic to almost asymptomatic (clinically quiet) phases, namely the preclinical phase (aberrant immune activation from the skin), subclinical phase (imaging findings without appreciable clinical symptoms, i.e., asymptomatic synovio-enthesial imaging abnormalities), and prodromal phase (arthralgia and fatigue without synovitis and/or enthesitis on examination) (8-10).
The time interval in the progression of PsA from PsO creates an “early” transition window, which can be exploited for identification, interception, and intervention to halt joint disease progression (2, 4, 6, 8-10). Early patient identification will enable dermatologists to timely refer at-risk patients to rheumatologists, who are otherwise consulted when significant, irreversible joint damage has already occurred (11). Currently, there are no specific diagnostic markers for early PsA (10, 12, 13). Prevention/reduction of PsA development has now been suggested as a new clinical endpoint in PsO trials. Since extensive inflammatory and musculoskeletal damage makes early therapeutic intervention quite challenging, there is an urgent need to identify distinct and reliable biomarkers for the early and timely prediction of possible transition to PsA in PsO (14).
2. Evidence Acquisition
A comprehensive literature search was conducted on the PubMed, Web of Science, EMBASE, and SCOPUS databases using keywords such as 'investigation of psoriatic arthritis,' 'early psoriatic arthritis,' 'subclinical PsA,' ‘multiomics in PsA,’ and ‘artificial intelligence in PsA.' All relevant articles were reviewed to investigate current modalities for early PsA, with a special emphasis on newer serological biomarkers and recent research on the role of machine learning and artificial intelligence in the development of PsA.
3. Results
The current predictive/diagnostic protocol in PsA involves extensive clinical questionnaires based on genetic and environmental components in medical history, physical examination, along with biochemical (immunological) and imaging (radiological) studies (15).
3.1. Clinical (Long-Term) Predictors
These include early age of onset of PsO, severe cutaneous disease, inverse PsO, nail pitting, obesity, PsO-affected first/second-degree relative, and uveitis as predictive clinical markers (5, 6, 9, 10, 16, 17). Non-specific musculoskeletal symptoms, such as joint pain/tenderness and arthralgia, might be suggestive, yet not completely diagnostic of PsA (6). Enthesitis (inflammation at the site of attachment of tendons, ligaments, and joint capsules to bone) is a prominent pathological sign, while peripheral oligoarthritis is often the most frequent pattern of PsA presentation (4). Dermatologists also utilize PsA screening and evaluation (PASE), psoriasis epidemiology screening tool (PEST), Toronto psoriatic arthritis screen (ToPAS), updated ToPAS II, early arthritis for psoriatic patients (EARP), CONTEST questionnaire, simple psoriatic arthritis screening (SiPAS), and modified Rheumatic Disease Comorbidity Index as screening tools (1, 6). Latest studies have explored graphical models, such as Bayesian Network correlating clusters of patient symptoms (18). However, robust utilization of these clinical tools is limited by their overall low sensitivity for early PsA (8). In practice, an objective evaluation of patient symptomatology is often difficult due to the non-specific nature of the musculoskeletal symptoms, limited data for their accurate definition, and diverse views regarding the relative significance that should be assigned to the proposed risk factors. This leads to an overall limited ability to precisely predict true disease progression (8).
3.2. Genetic (Long-Term) Predictors
Both PsO and PsA show characteristic genetic markers in HLA (human leukocyte antigen) regions (genes for antigen presentation and immune recognition) and non-HLA regions (genes for inflammation and immune activation, including cytokine expression, intracellular signaling, and T-cell effector function). Many of these genetic associations are not identical, and certain genes are associated with specific phenotypes in PsA (2, 17, 19). For instance, PsA has a weaker association with HLA-B*57:01 and HLA-C*06:02 (psoriasis susceptibility region 1 or PSORS1) and lacks association with interleukin (IL)-12B (5). Similarly, HLA-B*07, HLA-B*08, HLA-B*27, HLA-B*38, HLA-B*39, and HLA-C*12 are strong genetic risk factors for PsA development (8, 14, 19). Further, HLA-C*06 has been shown to delay PsA onset, thus conferring some protection to at-risk patients. Major histocompatibility complex class I chain-related A (MICA) alleles are also implicated in PsA (10, 15, 16). Non-HLA genes include interleukin 23 receptor (IL-23R), tumor necrosis factor alpha-induced protein 3 (TNFAIP3), human c-rel gene (REL), FBX19, and protein tyrosine phosphatase non-receptor type 22 (PTPN22) (10, 16). Other genes exclusively associated with PsA include B3GNT2, IL, KIR2D, KIF3A, and PTPN22 (14). Methylated DNA and CpG markers are additional predictive markers (20).
3.3. Imaging/Radiological (Short-Term) Predictors
PsA-affected joints can show diverse structural (new bone formation, gross joint destruction, erosions, "pencil-in-cup" deformity, joint space narrowing) and inflammatory (synovitis, tenosynovitis, periarticular inflammation, bone marrow edema) changes, driven by altered ‘osteoremodelling’ with increased pathological bone resorption and aberrant periarticular new bone formation (6, 8, 11). While structural features are mostly detectable by conventional radiography, inflammatory features are better identified via magnetic resonance imaging (MRI) (6, 9, 11). Musculoskeletal ultrasound (MSUS), MRI, and Positron emission tomography (PET) further identify subtle preclinical vascularization changes and subdermal inflammation as the earliest changes in PsA transition (5). Positron emission tomography reliably differentiates synovitis from PsA enthesitis. Fluorescence optical imaging (FOI) has recently emerged as successful at accurately detecting hypervascularization during PsA transition that may evade detection by MRI (10, 16). More advanced imaging modalities include High-resolution peripheral quantitative CT (HR-pQCT) (for structural entheseal lesions demonstrating low cortical volumetric bone mineral density), Shear-Wave Elastography using Gray Scale (GS) ultrasound and Power Doppler (for Achilles tendinopathy), and bone scintigraphy (for subclinical joint involvement in PsA without clinical arthropathy) (6, 8).
3.4. Biochemical/Serological (Short-Term) Predictors
Due to altered inflammatory cascade and osteoremodelling, certain related biomarkers (Table 1) have been investigated for their potential role in predicting PsA transition (18-24).
Biochemical/Serological (Short Term) Predictors
Variables | Predictors |
---|---|
1. Markers of inflammation | CRP, high-sensitivity-CRP |
IL-6 | |
Serum calprotectin | |
S100A8/S100A9 | |
2. Bone and cartilage turnover biomarkers | |
Catabolic markers (quantifying collagen/bone ECM breakdown) | PRO-C1 (propeptide) |
PRO-C3 | |
PRO-C4 | |
COMP | |
Anabolic markers (estimating formation peptides of bony collagens | C1M |
C2M | |
C3M | |
C4M | |
C10C | |
Others | Dickkopf-1 |
Sclerostin | |
RANKL | |
Bone alkaline phosphatase | |
OPG | |
3. Proteases | MMP-3 |
Stromelysin-1 | |
4. Proteins | |
Autoantibodies | Anti- MCV |
Anti-PsA peptide | |
Anti-N-RAP | |
Anti-ADAMSTS5 | |
Anti-cathelicidin LL37 (LL37) | |
Carbamethylated anti-LL37 | |
IgA anti-oxidized collagen type II (oxPTMCII) | |
Others | C-X-C motif chemokine ligand10 (CXCL10) |
Soluble IL-2 | |
ITGB5 | |
M2BP | |
Myeloperoxidase |
The predictor models currently in application chiefly encompass inputs from patient clinical history, radiological details, and laboratory serological investigations for a definitive answer. While patient history and evaluation of clinical features may not always reveal the absolute features of transition to PsA, the inflammatory and musculoskeletal damage depicted in imaging studies is usually quite extensive by the time patients are referred for rheumatological intervention, thus derailing early therapeutic intervention to halt the PsO-to-PsA transition (14). Among the serological markers, some studies have demonstrated their strong, positive, but not predictive role. However, other studies have shown conflicting results, thus limiting their utility by clinicians (2, 10). So far, only CXCL-10 has emerged as the marker with definite positive predictive value (2). Negative traditional arthritic markers such as rheumatoid factor (RF) and/or anti–cyclic citrullinated peptide antibodies (ACPA) limit their diagnostic role in PsA (10). Due to the paucity of a single specific disease predictor/indicator, there is a recent thrust on devising a multi-specialty approach incorporating soluble biochemical markers, imaging techniques, and multi-omics in PsO-to-PsA transition (Figure 1). Furthermore, digital investigative medicine and artificial intelligence are the latest research hotspots, as explained below.
PsO-to-PsA continuum depicting predictive indicators (clinical and imaging features with risk factors) for progression to PsA. “Created with BioRender.com”. PsA, psoriatic arthritis; PsO, psoriatic arthritis; 'HLA, human leukocyte antigen; MSK, musculoskeletal; BMI, body mass index; FACS, fluorescence-activated cell sorting analysis.
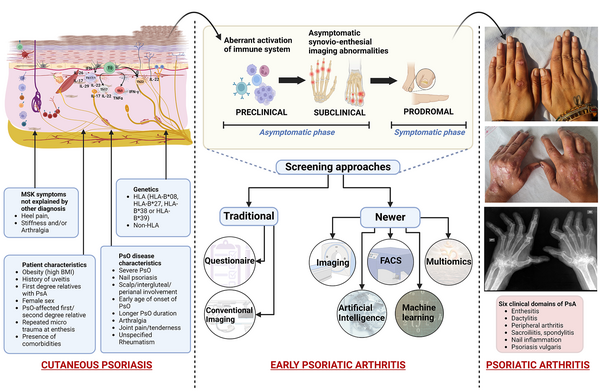
3.5. The “Omics” Technology
This technology allows for deciphering complete gene profiles (genomics and epigenomics), mRNA profiles (transcriptomics), protein profiles (proteomics), metabolite profiles (metabolomics), and lipid profiles (lipidomics) in a specific biological sample (14, 25-28). The EU-funded HIPPOCRATES project is one such collaboration aimed at analyzing and combining multiple molecular markers with conventional screening tools (13).
3.5.1. Genomics
Both PsO and PsA possess shared as well as distinct genetic pools (5, 8, 14, 18). Genome-wide genotyping arrays, Genome-wide association studies (GWAS), and immunoprecipitation techniques have shortlisted > 20 additional non-HLA loci, some of which are exclusive to PsA (14). Additionally, epigenetic events such as DNA methylation, histone modification, and small regulatory RNAs are being analyzed. The Oxford Biodynamics EpiSwitch platform has focused on specific 5-marker chromosome conformation signatures (sequences controlling 3D genomic structure) in genomic regions of IL-21R, IFNAR1, IL-23, IL-17A, and CXCL13 (14, 19, 25). Faster, cheaper, and easy-to-automate Next-generation sequencing platforms are also being explored (13).
3.5.2. Proteomics
Analysis of proteins in peripheral blood, serum, plasma, synovial fluid, urine, or skin specimens is being attempted, targeting > 3000 proteins using different selectivity concepts and amplification capabilities (25-27). Synovial fluid mass spectrometry has revealed 12 candidate PsA markers, including MMP3, S100A9, and CRP. Periostin (related to cell adhesion molecules and angiogenesis) has also been identified (14, 19, 25). More recently, spatial immunoproteomics approaches defining specific antigens in the epidermis and dermis are being studied to better understand humoral and cell-mediated immune mechanisms and how they function in PsA transition. This information can further be crucial for treatment protocols in PsA (25).
3.5.3. Metabolomics (Lipidomics)
Specimens like human plasma, urine, and feces, containing abundant metabolites with abnormal disease-specific temporal profiles, are being tested. These include modified levels of glucuronic acid, α-ketoglutaric acid, serum choline metabolite trimethylamine N-oxide (TMAO), and differences in bile acids (glycoursodeoxycholic acid sulfate) and butyrate in PsA development (28). Glycerol 1-hexadecanoate, α/β-turmerone, dihydrosphingosine, glutamine, and pantothenic acid have been elucidated as potential fecal metabolic biomarkers. Urine metabolomics has shown elevated glutamine, histamine, xanthine, phenylacetic acid, xanthurenic acid, lignoceric acid, and creatinine, with reduced citrate, alanine, methylsuccinate, trigonelline, ethanolamine, p-hydroxyphenylpyruvic acid, and phosphocreatine in PsA patients (14, 19, 28).
3.5.4. Single-Cell Phenotyping and Quantitative “Tissue Fluorescence-Activated Cell Sorting Analysis”
Single-cell phenotyping of immune cells and their interaction in PsA pathogenesis has emerged as a diagnostic approach with a key focus on immune checkpoint signaling, as demonstrated through fluorescence-activated cell sorting (FACS) in early studies. These technologies contribute to understanding the cellular mechanisms involved and can assist in developing therapeutic agents targeting those mechanisms (28).
3.5.5. Digital Technology
Digital technology is the latest weapon in the armory for detecting early PsA, aimed at filling the delicate gap between developing PsA and visible symptoms. The digital tools, apart from electronic records and virtual visits, include telemedicine, smartphone technology, artificial intelligence, and machine learning (29, 30). Machine learning algorithms are the newest tool under evaluation for diagnosing early PsA with high accuracy and precision (31, 32). Shapiro et al. devised the ‘PredictAI™’ model to accurately detect undiagnosed PsA (33) Similarly, a specialized ‘Disease Activity Score 28 (DAS28-CRP)’ utilizing machine learning-based permutation targeting ‘tenderness’ in PsA activity staging is also being developed (34). Machine learning analytics have also been expanded to identify distinct PsA phenotype clusters based on joints and skin/nail involvement, dactylitis, enthesitis, and axial manifestations (35). Using unsupervised clustering analysis, the machine learning algorithm ‘FlowSOM’ revealed higher memory T-helper (Th) cells in PsA (36). Some PsA risk prediction models are using 2-digit HLA alleles imputed using the SNP2HLA algorithm for stratifying and validating datasets to effectively segregate at-risk PsA patients (37). Using PsA-specific immune profiles via a machine learning approach, Mulder et al. showed an association with CD197+ monocytes and memory CD8+CD45RACD197- effector T-cells. Along with in-depth flow cytometry, this machine learning could identify distinct PsA immune cell profiles (2). ResNet neural networks aim to detect early PsA based on MRI-dependent joint inflammatory patterns, while the Disease-gene Immune cell Expression (DIME) network captures crucial PsA genes (38, 39).
Digital tools are now a revolution in medicine. The employment of various modes of digital technology can effectively harness multidimensional information, ranging from not just the classical factors like patients’ clinical features, imaging and serological findings, but even more specific genetic, metabolic, and immunologic profiles. The digital models have the advantage of being able to scan and analyze large patient databases for suspected undiagnosed PsA patients. Also, information from massive electronic medical records can be easily and rapidly assessed, thus tackling the manpower logistics (33-35, 39). Validated models can, in the future, serve as important point-of-care support tools for the general population, greatly reducing the need for more elaborate and time-consuming laboratory testing. Flagging even the subtle signs can address the outlier patients, which is currently a challenge for clinicians (35). Appropriately designed machine learning and AI models can provide the benefit of specific focus on relevant factors and can further be tailored to include treatment response as well. These tools can reduce the overall variability in the assessment and classification of at-risk PsO patients and also recognize patterns that may be missed by humans (33-35, 39). Thus, digital technology is crucial for early identification and interception of disease progression in PsO patients.
4. Conclusions
Several clinical trials have emphasized that even a slight delay in rheumatologic intervention in PsA can lead to irreversible joint damage, motor disability, and an overall impaired quality of life (1, 4). Timely and accurate identification of ‘at-risk’ PsO patients will serve as the foundation for appropriate intervention. The important checkpoints for this intervention include the timely detection of early PsA and its differentiation from other common PsA-mimicking arthropathies (osteoarthritis, OA, and fibromyalgia, FBM). An accurate prediction of PsA development will allow for escalation or modification of ongoing treatment, making it more comprehensive, preventing misdiagnosis and overtreatment due to mimickers, and facilitating prognostic and monitoring information (4, 8, 14, 15).
Hence, a holistic multi-specialty approach encompassing multi-omics, machine learning, and artificial intelligence, in addition to conventional investigative modalities, appears extremely promising with its successful utilization. This strongly underlines the need for close collaboration between dermatologists (the first point of contact for PsO patients), immunopathologists (investigative workforce), and rheumatologists (late consultation contact for PsA).
References
-
1.
Ogdie A, Harrison RW, McLean RR, Lin TC, Lebwohl M, Strober BE, et al. Prospective cohort study of psoriatic arthritis risk in patients with psoriasis in a real-world psoriasis registry. J Am Acad Dermatol. 2022;87(6):1303-11. [PubMed ID: 35987397]. https://doi.org/10.1016/j.jaad.2022.07.060.
-
2.
Mulder MLM, van Hal TW, Wenink MH, Koenen H, van den Hoogen FHJ, de Jong E, et al. Clinical, laboratory, and genetic markers for the development or presence of psoriatic arthritis in psoriasis patients: a systematic review. Arthritis Res Ther. 2021;23(1):168. [PubMed ID: 34127053]. [PubMed Central ID: PMC8201808]. https://doi.org/10.1186/s13075-021-02545-4.
-
3.
Tenazinha C, Barros R, Fonseca JE, Vieira-Sousa E. Histopathology of Psoriatic Arthritis Synovium-A Narrative Review. Front Med (Lausanne). 2022;9:860813. [PubMed ID: 35847785]. [PubMed Central ID: PMC9283901]. https://doi.org/10.3389/fmed.2022.860813.
-
4.
Floris A, Mugheddu C, Sichi L, Dessi M, Anedda J, Frau A, et al. The Challenging Differentiation of Psoriatic Arthritis from Other Arthropathies and Nonspecific Arthralgias in Patients with Psoriasis: Results of a Cross-Sectional Rheumatologic Assessment of a Large Dermatologic Cohort. J Clin Med. 2023;12(18). [PubMed ID: 37763030]. [PubMed Central ID: PMC10532027]. https://doi.org/10.3390/jcm12186090.
-
5.
Tiwari V, Brent LH. Psoriatic Arthritis. Treasure Island (FL): StatPearls; 2024.
-
6.
Gottlieb AB, Merola JF. A clinical perspective on risk factors and signs of subclinical and early psoriatic arthritis among patients with psoriasis. J Dermatolog Treat. 2022;33(4):1907-15. [PubMed ID: 34176399]. https://doi.org/10.1080/09546634.2021.1942423.
-
7.
Alinaghi F, Calov M, Kristensen LE, Gladman DD, Coates LC, Jullien D, et al. Prevalence of psoriatic arthritis in patients with psoriasis: A systematic review and meta-analysis of observational and clinical studies. J Am Acad Dermatol. 2019;80(1):251-265 e19. [PubMed ID: 29928910]. https://doi.org/10.1016/j.jaad.2018.06.027.
-
8.
Perez-Chada LM, Haberman RH, Chandran V, Rosen CF, Ritchlin C, Eder L, et al. Consensus terminology for preclinical phases of psoriatic arthritis for use in research studies: results from a Delphi consensus study. Nat Rev Rheumatol. 2021;17(4):238-43. [PubMed ID: 33589818]. [PubMed Central ID: PMC7997804]. https://doi.org/10.1038/s41584-021-00578-2.
-
9.
De Marco G, Zabotti A, Baraliakos X, Iagnocco A, Aletaha D, Gisondi P, et al. Characterisation of prodromal and very early psoriatic arthritis: a systematic literature review informing a EULAR taskforce. RMD Open. 2023;9(2). [PubMed ID: 37349122]. [PubMed Central ID: PMC10314670]. https://doi.org/10.1136/rmdopen-2023-003143.
-
10.
Gisondi P, Bellinato F, Maurelli M, Geat D, Zabotti A, McGonagle D, et al. Reducing the Risk of Developing Psoriatic Arthritis in Patients with Psoriasis. Psoriasis (Auckl). 2022;12:213-20. [PubMed ID: 35975124]. [PubMed Central ID: PMC9375973]. https://doi.org/10.2147/PTT.S323300.
-
11.
Merola JF, Chakravarty SD, Choi O, Chan D, Gottlieb AB. A clinical review of structural damage in psoriatic arthritis for dermatologists: From pathogenesis to ongoing controversies. J Am Acad Dermatol. 2024;90(2):349-57. [PubMed ID: 37852305]. https://doi.org/10.1016/j.jaad.2023.10.021.
-
12.
Dorochow E, Kohm M, Hahnefeld L, Gurke R. Metabolic Profiling in Rheumatoid Arthritis, Psoriatic Arthritis, and Psoriasis: Elucidating Pathogenesis, Improving Diagnosis, and Monitoring Disease Activity. J Pers Med. 2022;12(6). [PubMed ID: 35743709]. [PubMed Central ID: PMC9225104]. https://doi.org/10.3390/jpm12060924.
-
13.
Karmacharya P, Wright K, Achenbach SJ, Bekele D, Crowson CS, Ogdie A, et al. Diagnostic Delay in Psoriatic Arthritis: A Population-based Study. J Rheumatol. 2021;48(9):1410-6. [PubMed ID: 33589556]. [PubMed Central ID: PMC8364557]. https://doi.org/10.3899/jrheum.201199.
-
14.
Gurke R, Bendes A, Bowes J, Koehm M, Twyman RM, Barton A, et al. Omics and Multi-Omics Analysis for the Early Identification and Improved Outcome of Patients with Psoriatic Arthritis. Biomedicines. 2022;10(10). [PubMed ID: 36289648]. [PubMed Central ID: PMC9598654]. https://doi.org/10.3390/biomedicines10102387.
-
15.
Mahendran SM, Chandran V. Exploring the Psoriatic Arthritis Proteome in Search of Novel Biomarkers. Proteomes. 2018;6(1). [PubMed ID: 29364831]. [PubMed Central ID: PMC5874764]. https://doi.org/10.3390/proteomes6010005.
-
16.
Koehm M, Ohrndorf S, Foldenauer AC, Rossmanith T, Backhaus M, Werner SG, et al. Fluorescence-optical imaging as a promising easy-to-use imaging biomarker to increase early psoriatic arthritis detection in patients with psoriasis: a cross-sectional cohort study with follow-up. RMD Open. 2022;8(2). [PubMed ID: 36597973]. [PubMed Central ID: PMC9730423]. https://doi.org/10.1136/rmdopen-2022-002682.
-
17.
Scher JU, Ogdie A, Merola JF, Ritchlin C. Preventing psoriatic arthritis: focusing on patients with psoriasis at increased risk of transition. Nat Rev Rheumatol. 2019;15(3):153-66. [PubMed ID: 30742092]. https://doi.org/10.1038/s41584-019-0175-0.
-
18.
Green A, Tillett W, McHugh N, Smith T; Prompt Study Group. Using Bayesian networks to identify musculoskeletal symptoms influencing the risk of developing psoriatic arthritis in people with psoriasis. Rheumatol (Oxford). 2022;61(2):581-90. [PubMed ID: 33769484]. [PubMed Central ID: PMC8824425]. https://doi.org/10.1093/rheumatology/keab310.
-
19.
Wirth T, Balandraud N, Boyer L, Lafforgue P, Pham T. Biomarkers in psoriatic arthritis: A meta-analysis and systematic review. Front Immunol. 2022;13:1054539. [PubMed ID: 36532039]. [PubMed Central ID: PMC9749424]. https://doi.org/10.3389/fimmu.2022.1054539.
-
20.
Deng M, Su Y, Wu R, Li S, Zhu Y, Tang G, et al. DNA methylation markers in peripheral blood for psoriatic arthritis. J Dermatol Sci. 2022;108(1):39-47. [PubMed ID: 36404219]. https://doi.org/10.1016/j.jdermsci.2022.11.001.
-
21.
Holm Nielsen S, Magee C, Groen SS, Sinkeviciute D, Bay-Jensen AC, Karsdal MA, et al. Differentiating patients with psoriasis from psoriatic arthritis using collagen biomarkers. Clin Exp Rheumatol. 2023;41(3):574-80. [PubMed ID: 35916294]. https://doi.org/10.55563/clinexprheumatol/jmt9jv.
-
22.
Biedron G, Czepiel M, Siedlar M, Korkosz M. Serum concentration of dickkopf-related protein 1 (DKK1) in psoriatic arthritis in the context of bone remodelling. Rheumatol Int. 2023;43(12):2175-83. [PubMed ID: 37750896]. [PubMed Central ID: PMC10587027]. https://doi.org/10.1007/s00296-023-05452-w.
-
23.
Geneva-Popova M, Popova-Belova S, Popova V, Chompalov K, Batalov A. Assessment of serum and synovial fluid MMP-3 and MPO as biomarkers for psoriatic arthritis and their relation to disease activity indices. Rheumatol Int. 2022;42(9):1605-15. [PubMed ID: 35708757]. https://doi.org/10.1007/s00296-022-05159-4.
-
24.
Abji F, Lee KA, Pollock RA, Machhar R, Cook RJ, Chandran V, et al. Declining levels of serum chemokine (C-X-C motif) ligand 10 over time are associated with new onset of psoriatic arthritis in patients with psoriasis: a new biomarker? Br J Dermatol. 2020;183(5):920-7. [PubMed ID: 32037514]. https://doi.org/10.1111/bjd.18940.
-
25.
Sobolev VV, Soboleva AG, Denisova EV, Pechatnikova EA, Dvoryankova E, Korsunskaya IM, et al. Proteomic Studies of Psoriasis. Biomedicines. 2022;10(3). [PubMed ID: 35327421]. [PubMed Central ID: PMC8945259]. https://doi.org/10.3390/biomedicines10030619.
-
26.
Chularojanamontri L, Charoenpipatsin N, Silpa-Archa N, Wongpraparut C, Thongboonkerd V. Proteomics in Psoriasis. Int J Mol Sci. 2019;20(5). [PubMed ID: 30845706]. [PubMed Central ID: PMC6429319]. https://doi.org/10.3390/ijms20051141.
-
27.
Qi F, Tan Y, Yao A, Yang X, He Y. Psoriasis to Psoriatic Arthritis: The Application of Proteomics Technologies. Front Med (Lausanne). 2021;8:681172. [PubMed ID: 34869404]. [PubMed Central ID: PMC8635007]. https://doi.org/10.3389/fmed.2021.681172.
-
28.
Looby N, Roszkowska A, Reyes-Garces N, Yu M, Baczek T, Kulasingam V, et al. Serum metabolic fingerprinting of psoriasis and psoriatic arthritis patients using solid-phase microextraction-liquid chromatography-high-resolution mass spectrometry. Metabolomics. 2021;17(7):59. [PubMed ID: 34137950]. [PubMed Central ID: PMC8211611]. https://doi.org/10.1007/s11306-021-01805-3.
-
29.
Giovannini I, Bosch P, Dejaco C, De Marco G, McGonagle D, Quartuccio L, et al. The Digital Way to Intercept Psoriatic Arthritis. Front Med (Lausanne). 2021;8:792972. [PubMed ID: 34888334]. [PubMed Central ID: PMC8650082]. https://doi.org/10.3389/fmed.2021.792972.
-
30.
Bragazzi NL, Bridgewood C, Watad A, Damiani G, Kong JD, McGonagle D. Harnessing Big Data, Smart and Digital Technologies and Artificial Intelligence for Preventing, Early Intercepting, Managing, and Treating Psoriatic Arthritis: Insights From a Systematic Review of the Literature. Front Immunol. 2022;13:847312. [PubMed ID: 35359924]. [PubMed Central ID: PMC8960164]. https://doi.org/10.3389/fimmu.2022.847312.
-
31.
Reed M, Rampono B, Turner W, Harsanyi A, Lim A, Paramalingam S, et al. A multicentre validation study of a smartphone application to screen hand arthritis. BMC Musculoskelet Disord. 2022;23(1):433. [PubMed ID: 35534813]. [PubMed Central ID: PMC9081322]. https://doi.org/10.1186/s12891-022-05376-9.
-
32.
Lee LT, Yang HC, Nguyen PA, Muhtar MS, Li YJ. Machine Learning Approaches for Predicting Psoriatic Arthritis Risk Using Electronic Medical Records: Population-Based Study. J Med Internet Res. 2023;25. e39972. [PubMed ID: 36976633]. [PubMed Central ID: PMC10055385]. https://doi.org/10.2196/39972.
-
33.
Shapiro J, Getz B, Cohen SB, Jenudi Y, Underberger D, Dreyfuss M, et al. Evaluation of a machine learning tool for the early identification of patients with undiagnosed psoriatic arthritis - A retrospective population-based study. J Transl Autoimmun. 2023;7:100207. [PubMed ID: 37577138]. [PubMed Central ID: PMC10412462]. https://doi.org/10.1016/j.jtauto.2023.100207.
-
34.
Rischke S, Poor SM, Gurke R, Hahnefeld L, Kohm M, Ultsch A, et al. Machine learning identifies right index finger tenderness as key signal of DAS28-CRP based psoriatic arthritis activity. Sci Rep. 2023;13(1):22710. [PubMed ID: 38123604]. [PubMed Central ID: PMC10733369]. https://doi.org/10.1038/s41598-023-49574-4.
-
35.
Richette P, Vis M, Ohrndorf S, Tillett W, Ramirez J, Neuhold M, et al. Identification of PsA phenotypes with machine learning analytics using data from two phase III clinical trials of guselkumab in a bio-naive population of patients with PsA. RMD Open. 2023;9(1). [PubMed ID: 37001920]. [PubMed Central ID: PMC10069583]. https://doi.org/10.1136/rmdopen-2022-002934.
-
36.
den Braanker H, Razawy W, Wervers K, Mus AC, Davelaar N, Kok MR, et al. Characterizing memory T helper cells in patients with psoriasis, subclinical, or early psoriatic arthritis using a machine learning algorithm. Arthritis Res Ther. 2022;24(1):28. [PubMed ID: 35045868]. [PubMed Central ID: PMC8767727]. https://doi.org/10.1186/s13075-021-02714-5.
-
37.
Jalali-Najafabadi F, Stadler M, Dand N, Jadon D, Soomro M, Ho P, et al. Application of information theoretic feature selection and machine learning methods for the development of genetic risk prediction models. Sci Rep. 2021;11(1):23335. [PubMed ID: 34857774]. [PubMed Central ID: PMC8640070]. https://doi.org/10.1038/s41598-021-00854-x.
-
38.
Folle L, Bayat S, Kleyer A, Fagni F, Kapsner LA, Schlereth M, et al. Advanced neural networks for classification of MRI in psoriatic arthritis, seronegative, and seropositive rheumatoid arthritis. Rheumatol (Oxford). 2022;61(12):4945-51. [PubMed ID: 35333316]. https://doi.org/10.1093/rheumatology/keac197.
-
39.
Devaprasad A, Radstake T, Pandit A. Integration of Immunome With Disease-Gene Network Reveals Common Cellular Mechanisms Between IMIDs and Drug Repurposing Strategies. Front Immunol. 2021;12:669400. [PubMed ID: 34108969]. [PubMed Central ID: PMC8181425]. https://doi.org/10.3389/fimmu.2021.669400.