Abstract
Keywords
Mass Gathering Medicine Arbaeen Pilgrimage Health Artificial Intelligence Telemedicine
1. Background
Artificial intelligence (AI) is a multidisciplinary field centered on enabling machines to exhibit intelligence, perception, and information inference. By employing various mathematical algorithms—including fuzzy logic, neural networks, optimization algorithms, and machine learning—AI has revolutionized numerous industries, notably the medical and health sectors. Mass gathering medicine (1), characterized by high complexity and uncertainty, is well-suited for the application of AI-based technologies. These technologies, through telemedicine and virtual consultations, help reduce the number of patients requiring in-person clinic visits. Currently, the primary focus in mass gathering medicine is on infectious disease management and risk assessment (2, 3).
In mass gathering medicine, on-site medical professionals are often limited in availability, making remote healthcare support an invaluable tool. Through video consultations, remote expert physicians can evaluate patient conditions and provide treatment recommendations to on-site, often younger medical staff who may be under stress or working in critical situations. This support aids in timely and effective decision-making, ultimately improving patient outcomes. Artificial intelligence-based telemedicine, using real-time communication in environments with limited specialist resources, further enhances the quality of care. For instance, in (4), AI-based decision-making is used for skin cancer recognition through multiclass probabilities, while (5) discusses how the integration of metaverse technology and AI supports AI-based medical practices. Additionally, a review on the application of AI in chronic obstructive pulmonary disease highlights its potential and challenges (6).
Artificial intelligence is also advancing the next generation of prosthetic hands, where brain/machine neural interconnections enable movement and sensation (7). Another application, described in (8), uses AI to detect COVID-19 through cough, breathing, and speech recordings. Moreover, (9) reviews the application of AI in analyzing electrocardiograms (ECG) for personalized cardiovascular disease management.
Given the broad applications of AI in medicine, this paper proposes an AI-based telemedicine platform for mass gathering medicine during the Arbaeen pilgrimage. This platform aims to provide telemedical services and monitor the health of pilgrims remotely. Specifically, we propose online medical clinics to deliver services remotely, alongside AI-based health services that process patient data and deliver results directly to the patient.
The remainder of this paper is structured into three sections: Section 2 outlines the proposed method, Section 3 presents the results, and Section 4 concludes the study.
2. Objectives
This cross-sectional study was conducted with patients who participated in the Arbaeen pilgrimage mass gathering and utilized in-person medical services.
3. Methods
Participants were asked to complete a brief questionnaire to assess the potential benefits of AI-based telemedicine platforms in conjunction with on-site medical services. The questionnaire included questions such as:
- Did you use a medical Mokeb?
- How long did you wait to receive medical services?
- Which medical issues had the highest occurrence during your pilgrimage?
- Was an in-person visit necessary for your case?
- Do you think a remote consultation could also have helped you?
- Would you prefer to have the option of using telemedicine and AI-based medical services next time?
Most respondents emphasized the necessity of AI-based telemedicine platforms, expressing that such services would reduce the demand for in-person consultations with on-site medical staff. They were also asked to identify the most common medical issues experienced during the Arbaeen pilgrimage.
Based on patients’ experiences and expert recommendations, a selection of key medical specialties has been chosen for the virtual clinics. These online clinics will include surgeons, doctors, nurses, and paramedics who have registered their availability in advance and provide remote services at designated times. When patients require medical assistance, they can describe their condition through a short questionnaire, using text, voice, or video message, with the option to communicate in their preferred language. Online medical staff will review these descriptions, offer consultations, or direct patients to the nearest in-person medical clinics if necessary. For urgent situations, patients can share their location information. Additionally, patients may provide local real-time information to assist medical staff in making informed decisions.
Figure 1 illustrates the first proposed setup: In Figure 1A, patients in a mass gathering can consult with an online surgeon or doctor by sending relevant data, such as images. In Figure 1B, an online doctor or surgeon reviews patient comments and consults with the patient remotely, using the information sent by the patient.
Arbaeen health telemedicine platform. A, patient at the mass gathering asking questions by sending data e.g. by sending an image to an online surgeon; B an online surgeon consulting the patient at the mass gathering from remote by investigating data e.g. image which is sent by the patient.
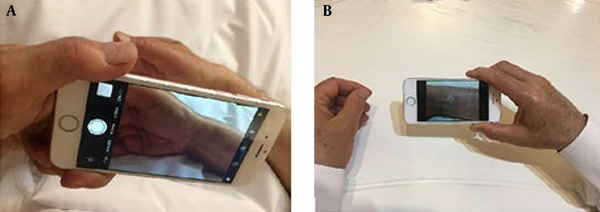
In the second component, AI-based health services are provided. Approved AI medical platforms, registered in the system, deliver services by receiving essential input information, such as images or sounds. Patients are required to enter demographic details and answer specific questions related to their symptoms, depending on their medical category. Using machine learning algorithms, the AI system processes this data, identifies hidden patterns, and performs the detection process once the learning phase is complete. Figure 2 illustrates the proposed AI-based telemedicine setup.
As a test case for AI-based telemedicine, we propose an AI-powered heat stroke prediction system to detect Exertional heat stroke (EHS), a life-threatening condition triggered by factors such as intense physical activity and environmental heat. Exertional heat stroke leads to a rapid rise in core body temperature and dysfunction in the central nervous system, necessitating immediate cooling to prevent further illness progression.
Artificial intelligence (AI)-based telemedicine. The intelligent system is trained by different data using machine learning algorithms and service patients as a web-based mobile platform. When a patient at the mass gathering enters data in the intelligent system, it will diagnose and recommend medical consultation or recommend the patient to go to an on-site medical clinic in an urgent case.
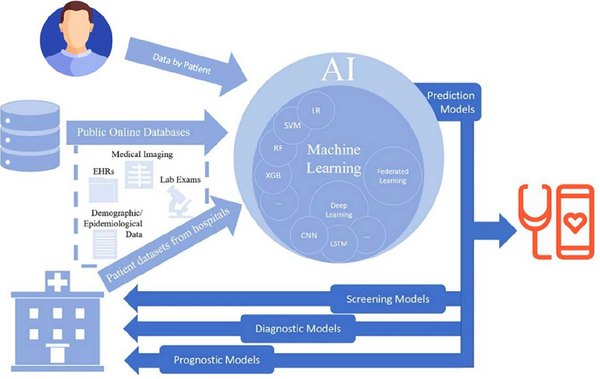
To implement the system, we utilized data from 608 patients diagnosed with heat stroke, as referenced in (10). This dataset comprises 27 features, of which we selected 25 relevant attributes for our analysis, including Daily ingested water (DIW) in liters, time of year (month), history of cardiovascular disease, dehydration status, Heat Index (HI), diastolic blood pressure (DBP), environmental temperature in celsius, systolic blood pressure (SBP), weight in kilograms, patient’s core temperature, rectal temperature, relative humidity, sun exposure, Body Mass Index (BMI), barometric pressure, heat stroke diagnosis, heart rate or pulse, age, sweating presence, skin color (with values such as flushed/normal = 1, pale = 0.5, cyanotic = 0), exercise engagement, nationality, gender, skin condition (hot/dry), and time of day.
After completing data preprocessing, we applied Pearson’s correlation matrix to reduce the impact of correlated variables in the prediction model. Subsequently, we used four machine learning algorithms: Adaboost, Bagging Decision Tree, Bagging KNeighbors, and Multi-Layer Perceptron (MLP) to perform the prediction.
4. Results
We begin by analyzing the results of Pearson’s correlation matrix. Figure 3 presents the correlation matrix, which indicates that the parameters are largely uncorrelated. Next, we examine pairwise relationships between parameters to identify key factors associated with heat stroke. As shown in Figure 4, age, heart/pulse rate, rectal temperature, patient temperature, SBP, and DBP emerge as the most significant parameters in predicting heat stroke. These identified parameters could be valuable in developing AI-based point-of-care sensors for early heat stroke detection.
Pearson’s correlation matrix for 17 main features
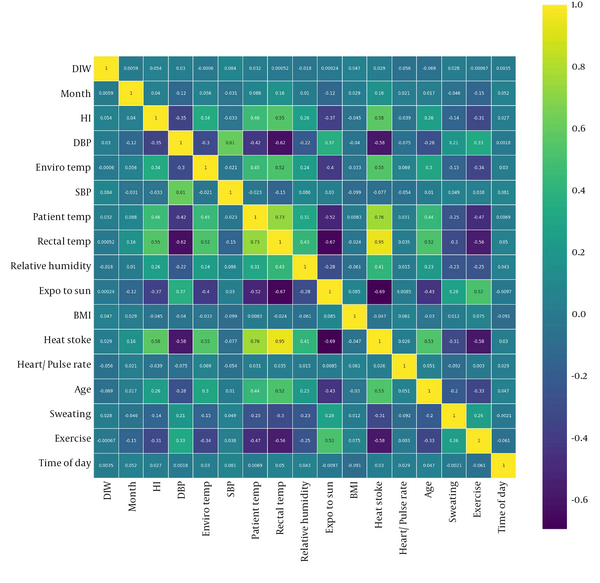
Pairwise relationships of 16 main features of heat stroke
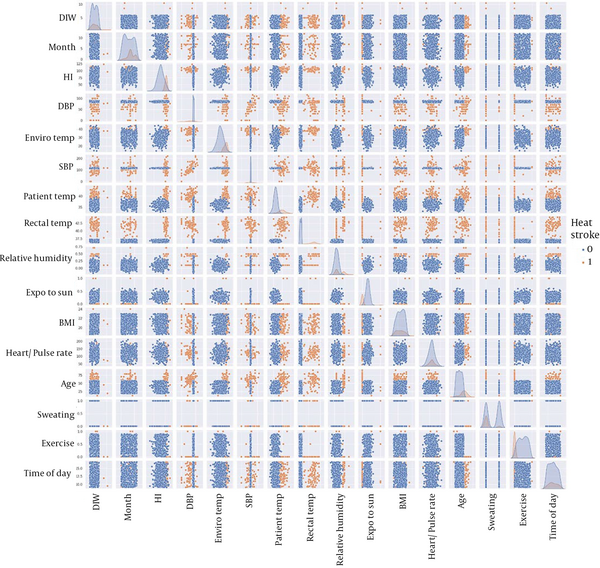
Subsequently, we apply four machine learning algorithms—Adaboost, Bagging Decision Tree, Bagging KNeighbors, and MLP—to perform heat stroke prediction. Table 1 provides a comparison of the average results across 10 runs. The results indicate that Adaboost consistently outperforms the other algorithms in predictive accuracy.
Comparison of the Average of 10 Runs of Each Model
Model Prediction | Accuracy |
---|---|
Adaboost | 0.94 |
Bagging_decision tree | 0.92 |
Bagging_KNeighbors | 0.75 |
MLP | 0.7 |
5. Discussion
This research proposes an AI-based platform designed to deliver telemedicine during the Arbaeen pilgrimage, enabling remote telemedical and health services as well as health monitoring in mass gathering scenarios. The AI telemedicine system offers two types of telemedicine: Online virtual clinics and AI-based medical services. Future developments will leverage the recorded data to support point-of-care personal telemedicine through wearable sensors and the internet of things (IoT). Notably, this medical platform also has potential applications in providing health services during natural disasters.
References
-
1.
Memish ZA, Steffen R, White P, Dar O, Azhar EI, Sharma A, et al. Mass gatherings medicine: public health issues arising from mass gathering religious and sporting events. Lancet. 2019;393(10185):2073-84. [PubMed ID: 31106753]. [PubMed Central ID: PMC7159069]. https://doi.org/10.1016/S0140-6736(19)30501-X.
-
2.
Glaria AA, Mullen L, Kovokovich A, Gabrielli AF, Grant R, Emiroglu N, et al. Mass-gathering decision making and its implementation during the COVID-19 pandemic. Lancet Public Health. 2023;8(12):e912-3. [PubMed ID: 38000886]. https://doi.org/10.1016/S2468-2667(23)00275-X.
-
3.
Al-Shaery AM, Hejase B, Tridane A, Farooqi NS, Al Jassmi H. Evaluating COVID-19 control measures in mass gathering events with vaccine inequalities. Sci Rep. 2022;12(1):3652. [PubMed ID: 35256669]. [PubMed Central ID: PMC8901904]. https://doi.org/10.1038/s41598-022-07609-2.
-
4.
Tschandl P, Rinner C, Apalla Z, Argenziano G, Codella N, Halpern A, et al. Human-computer collaboration for skin cancer recognition. Nat Med. 2020;26(8):1229-34. [PubMed ID: 32572267]. https://doi.org/10.1038/s41591-020-0942-0.
-
5.
Wang G, Badal A, Jia X, Maltz JS, Mueller K, Myers KJ, et al. Development of metaverse for intelligent healthcare. Nat Mach Intell. 2022;4(11):922-9. [PubMed ID: 36935774]. [PubMed Central ID: PMC10015955]. https://doi.org/10.1038/s42256-022-00549-6.
-
6.
Exarchos K, Aggelopoulou A, Oikonomou A, Biniskou T, Beli V, Antoniadou E, et al. Review of Artificial Intelligence Techniques in Chronic Obstructive Lung Disease. IEEE J Biomed Health Inform. 2022;26(5):2331-8. [PubMed ID: 34914601]. https://doi.org/10.1109/JBHI.2021.3135838.
-
7.
Luu DK, Nguyen AT, Jiang M, Drealan MW, Xu J, Wu T, et al. Artificial Intelligence Enables Real-Time and Intuitive Control of Prostheses via Nerve Interface. IEEE Trans Biomed Eng. 2022;69(10):3051-63. [PubMed ID: 35302937]. https://doi.org/10.1109/TBME.2022.3160618.
-
8.
Husain M, Simpkin A, Gibbons C, Talkar T, Low D, Bonato P, et al. Artificial Intelligence for Detecting COVID-19 With the Aid of Human Cough, Breathing and Speech Signals: Scoping Review. IEEE Open J Eng Med Biol. 2022;3:235-41. [PubMed ID: 36819937]. [PubMed Central ID: PMC9933914]. https://doi.org/10.1109/OJEMB.2022.3143688.
-
9.
Joy SI, Kumar KS, Palanivelan M, Lakshmi D. Review on Advent of Artificial Intelligence in Electrocardiogram for the Detection of Extra-Cardiac and Cardiovascular Disease. IEEE Canada J Electric Comput Eng. 2023;46(2):99-106. https://doi.org/10.1109/icjece.2022.3228588.
-
10.
Deaton J, Jaffe A, Gong D. Real-time heat stroke prediction via wearable sensors. Bioengineering Senior Capstone 2016-17; 2017. Available from: https://github.com/jondeaton/Heat-Stroke-Prediction.