Abstract
Background:
Hemodialysis is the most common renal replacement therapy in patients with end stage renal disease (ESRD).Objectives:
The present study compared the performance of various parametric models in a survival analysis of hemodialysis patients.Methods:
This study consisted of 270 hemodialysis patients who were referred to Imam Khomeini and Fatima Zahra hospitals between November 2007 and November 2012. The Akaike information criterion (AIC) and residuals review were used to compare the performance of the parametric models. The computations were done using STATA Software, with significance accepted at a level of 0.05.Results:
The results of a multivariate analysis of the variables in the parametric models showed that the mean serum albumin and the clinic attended were the most important predictors in the survival of the hemodialysis patients (P < 0.05). Among the parametric models tested, the results indicated that the performance of the Weibull model was the highest.Conclusions:
Parametric models may provide complementary data for clinicians and researchers about how risks vary over time. The Weibull model seemed to show the best fit among the parametric models of the survival of hemodialysis patients.Keywords
Hemodialysis Survival Analysis Parametric Models Accelerated Failure Time (AFT) Assumption Akaike Information Criterion (AIC)
1. Background
Survival analysis is used in various fields for data analysis existing between the event incidence or the conditions and situations changing time (1). Generally, there are two regression models for the survival data: Cox proportional risks model as a semi-parametric model (2) and accelerated failure time (AFT) models as parametric models. Standard parametric models include Weibull, exponential, and log-normal (3). If the assumptions of the parametric models exist, a stronger analysis can be conducted compared to semi-parametric methods. Considering some suppositions and selection of a hypothetical probability distribution for survival times makes statistical inference more precise, causing the standard deviations to become smaller than the time when such suppositions did not exist (4).
In end stage renal disease (ESRD), the kidneys are not able to do the metabolic actions and create liquids and metabolites equilibrium in the body (5). In such cases, the survival of the patient is only possible with a kidney transplant. Although there are geographical differences in the treatment of ESRD, hemodialysis remains the most common therapy for this disease (6). Due to increased access to dialysis, many ESRD patients now live longer than ever before. Worldwide, the ESRD-related mortality rate in Europe and Japan is relatively low, whereas it is very high in developing countries due to limited access to hemodialysis. In the U.S., the mortality rate of patients receiving dialysis is almost 18% - 20%, and their 5-year survival rate is about 30% - 35 % (7). In Iran, the overall 1-, 2-, 3-, 4-, and 5-year survival rates of hemodialysis patients were 75%, 63%, 50%, 41%, and 23%, respectively. This study reported a low survival rate among hemodialysis patients (8).
2. Objectives
The aim of the present study was to compare a number of parametric models (Weibull, exponential, and log-normal) to determine the best model for analyzing the survival of hemodialysis patients and to investigate the factors influencing the survival of these patients at two treatment centers in Iran.
3. Methods
This study consisted of 270 hemodialysis patients who were referred to the Sari-based Imam Khomeini and Fatima Zahra hospitals between November 2007 and November 2012. Data were obtained from the hospitals’ archives. The sampling was done by census, and the patients’ information was collected via a checklist the validity of which was confirmed by this plan statistical and medical counselor. Some data were gathered by calling, and each patient’s survival time was estimated in months. The subjects alive (survived) at the end of the study and those lost to follow-up were considered the right censor. The study was approved by the ethical committee of Mazandaran University of Medical Science.
The following covariates were included as prognostic factors in the models: gender, age (years), education level, marital status, employment, smoking record, diseases leading to dialysis (diabetes, hypertension, renal stones and obstructions, polycystic kidney disease, congenital diseases, uncertain, and other), age (years) at the time of disease diagnosis, cardiovascular disease, age (years) at the initiation of dialysis, mean serum albumin (mg/dL), mean serum creatinine (mg/dL), mean urea (mg/dL), and clinic attended.
3.1 Exponential Distribution
The exponential distribution is the simplest and most important distribution in survival studies. Since 1940, researchers have used the exponential distribution to show the life pattern for electronic systems. Like a normal distribution in other statistical areas, exponential distribution has played an important role in lifetime studies. Being independent of prior information, it is known as a “lack of memory” distribution requiring that the present age of the living organism does not influence its future survival (9).
3.2 Weibull Distribution
The Weibull distribution, a generalized form of the exponential distribution, is widely used for weather forecast modeling in meteorology and determining the wind distribution in radar modeling. The Weibull distribution was subsequently used in medical research to analyze survival data (10). When its medical application was analyzed, it was observed that the Weibull distribution is an important model due to the possibility of uniform increasing or decreasing the death rate in patients. A previous study employed the Weibull distribution to calculate the survival of children suffering from anemia (11).
3.3. Logs-Normal Distribution
Skew distributions, low values mean, high variance, and non-negative values, such as species variety and the distribution of minerals in Earth’s crust, and generally based on log-normal distributions are commonly used for responding to stimulant biological substances, repair, financial researchers, and studying the load price . As the application of log-normal distribution was tested, there were some examples of geology, metallurgy, health, environment, ecology, linguistics, social sciences, and economics (12).
3.4. Accelerated Failure Time (AFT) Assumption
Many parametric models are AFT models rather than PH models. The underlying assumption in AFT models is that the effect of the covariates is multiplicative (proportional) with respect to the survival time. In a regression framework, the acceleration factor γ can be parameterized as the exp (α), where α is the parameter to be estimated from the data. With this parameterization, the AFT assumption can be expressed as S 2 (t) = S 1 (exp (α) t) or equivalently as S 2 (exp (- α) t) = S 1 (t) fort ≥ 0. The acceleration factor is a ratio of survival times corresponding to any fixed value of S(t) (13) whose values more than 1 result in longer survival time for the patient (14).
In the present study, the Akaike information criterion (AIC) was employed to compare the performance of the models. In statistical models, the AIC denotes which model shows a better fit to the data (15). A lower AIC value denotes a better fit. The Formula 1 for the statistical estimation of the AIC was as follows:

Where P is the number of parameters, and K (constant factor) equals 1 in the exponential model and equals 2 in the Weibull and log-normal models. The execution of the models and data analysis were done using STATA software, version 8, and the significance level was set as 0.05.
4. Results
In the present study, of the 270 patients followed up, 142 (52.6%) died, and 128 (47.4%) were employed as censors. In terms of gender incidence, 114 (42.2%) of the patients were women, and 156 (57.8%) were men (Table 1). To estimate the survival rate, the Kaplan-Meier method was used (Figure 1). The 1-, 2-, 3-, 4-, and 5-year survival probability of the hemodialysis patients was 73.9%, 60.3%, 50.2%, 43.6%, and 18.4%, respectively. The average survival time was 36.21 months, with a confidence level of around 95% (33.148, 39.263), and the median survival time was 40 months.
Demographic and Clinical Data on the Patients With Hemodialysis
Characteristics | No. (%) |
---|---|
Gender | |
Male | 156 (57.8) |
Female | 114 (42.2) |
Education status | |
Less than a diploma | 129 (47.8) |
Diploma and higher | 141 (52.2) |
Marital status | |
Single | 27 (10) |
Married | 243 (90) |
Employment | |
Housewife | 107 (39.6) |
Jobless | 16 (5.6) |
Office worker | 23 (8.5) |
Farmer | 31 (11.5) |
Retired | 52 (19.3) |
Other | 41 (15.2) |
Smoking record | |
No | 191 (70.7) |
Yes | 79 (29.3) |
Diseases leading to dialysis | |
Diabetes | 138 (51.1) |
Hypertension | 40 (14.8) |
Renal obstructions | 13 (4.8) |
Polycystic kidney disease | 4 (1.5) |
Congenital disease | 2 (0.7) |
Unknown | 54 (20) |
Other | 19 (7.1) |
Cardiovascular diseases | |
No | 143 (53) |
Yes | 127 (47) |
Clinic | |
Imam Khomeini | 135 (50) |
Fatima Zahra hospital | 135 (50) |
Kaplan-Meier Diagram of the 5-Year Survival of Hemodialysis Patients
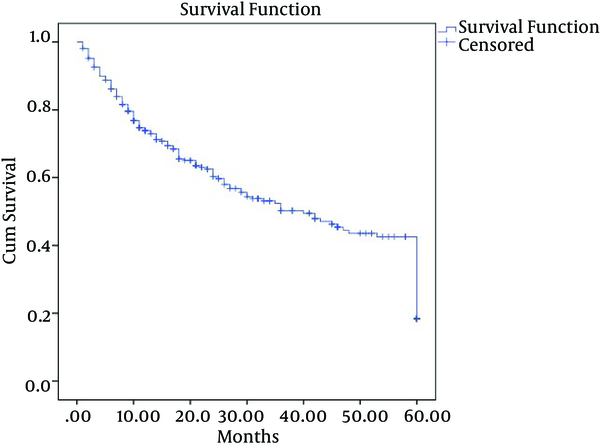
Multivariate Analysis of Parametric Models of the Survival of the Hemodialysis Patients
Variable | Exponentiala(P value) | Weibull (P Value)a | Log-Normal (P value)a |
---|---|---|---|
Age | 0.99 (0.560) | 0.99 (0.557) | 1 (0.981) |
Marital status | 0.36 (0.160) | 0.42 (0.070) | 1.38 (0.653) |
Employment | 1.39 (0.732) | 1.04 (0.481) | 0.96 (0.719) |
Smoking record | 0.76 (0.484) | 0.78 (0.308) | 1.14 (0.763) |
Diabetes | 0.89 (0.827) | 1.05 (0.852) | 0.84 (0.729) |
Hypertension | 1.45 (0.479) | 1.55 (0.167) | 1.02 (0.975) |
Renal obstructions | 0.32 (0.176) | 0.35 (0.047)b | 1 (0.993) |
Age at the time of disease diagnosis | 0.99 (0.819) | 1 (0.945) | 0.99 (0.755) |
Cardiovascular diseases | 0.66 (0.307) | 0.69 (0.147) | 1.08 (0.854) |
Age at the initiation of dialysis | 1 (0.307) | 1 (0.705) | 1 (0.710) |
Mean serum albumin | 1.90 (0.044)b | 1.76 (0.004)b | 1.05 (0.885) |
Mean serum creatinine | 0.89 (0.792) | 0.96 (0.901) | 1.11 (0.807) |
Mean urea | 1.60 (0.237) | 1.39 (0.200) | 1.10 (0.777) |
Clinic | 0.23 (0.022)b | 0.41 (0.035)b | 1.09 (0.875) |
In the first step, to compare the survival rates in the study variables subgroups, first the factors influencing the patients survival time were identified using a rank logarithm test. Then, all variables getting meaningful in the mentioned test and also those despite not getting meaningful with a p value less than 0.25 were introduced into the parametric models. The variables that were meaningful according to the log-rank test were: age, marital status, smoking record, disease leading to dialysis (hypertension), disease leading to dialysis diagnosis age (years), cardiovascular disease, age (years) at the initiation of dialysis, mean serum albumin (mg/dL), mean urea (mg/dL), and clinic attended. The following variables had a p value less than 0.25: employment, type of disease leading to dialysis (diabetes, renal stone, and obstructions), and mean serum creatinine (mg/dL).
Table 2 reports details of the multivariate analysis of the parametric models based on the γ for each variable. The mean serum albumin level and clinic attended were significant factors in the exponential and Weibull models but not in the log-normal model. None of the variables in the log-normal model were significant prognostic factors.
A similar conclusion was reached using the AIC. The AIC of each model in the study is given in Table 3. The best scores were achieved under the Weibull model. The lowest AIC value was obtained using the log-normal model.
Overview of the Akaike Information Criterion (AIC) Scores
Parametric Models | AIC |
---|---|
Exponential | 175.1264 |
Weibull | 160.2775 |
Log-normal | 205.3388 |
5. Discussion
This study compared the performance of various parametric models of the survival of hemodialysis patients. Parametric models were selected to estimate the survival probabilities in this research because they have been shown to be more accurate for projections.
Parametric models make more efficient use of the data because estimation is based on both time and event information. There are several parametric models with specific hazard shapes. The simplest type of parametric model, the exponential model, assumes that the hazard is constant over time. Breaking time into pieces and using this model, we found that the form of the hazard for access failure is consistent with the Weibull distribution, which assumes that the hazard declines (or increases) monotonically over time. The main advantage of Weibull regression is that the effects can be formulated in terms of time ratios (16). In analyses of survival data, parametric models, such as the Weibull and log-normal, can interpret the survival time based on a specific distribution, irrespective of the proportional hazard. If the survival times use a Weibull or exponential distribution, the analysis with parametric models will be stronger. This means that, under special conditions, parametric models, such as the Weibull and log-normal, may have more accurate results than the Cox model (17). As survival times usually have an exponential or a Weibull distribution, a parametric model is more efficient and similar than its corresponding nonparametric or semi-parametric models. Moreover, parametric models provide more flexibility with regard to the addition of covariates to the model (4).
In the present study, exponential and Weibull models were chosen because they are relatively easy to understand, and both have hazard and time metrics, allowing comparison of the two interpretations. For some survival problems or different populations, nonproportional solutions or different parametric models may perform better (18). In the present study, the empirical hazard function curve indicated ascending risk. Given the shape of the hazard function curve (not estimated here), the Weibull distribution showed the best fit to the survival data on the hemodialysis patients (19). This result is in agreement with that of models developed in other studies (18, 20).
Renal natural performances include various cellular processes acting to maintain homeostasis (internal body condition balance), and dysfunction in any these processes can lead to abnormal states, which can have adverse health consequences (7). Irreversible loss of renal function lasting more than 3 months is called chronic renal failure. Patients with advanced-stage renal failure (i.e., ESRD) require dialysis or a transplant for survival (21). Out of the common therapies, such as kidney replacement, hemodialysis is considered the dominant method in most of ESRD patients (22).
In the present study, the gender ratio was 1.4, which was in line with that reported in a Canadian population (20), although some studies reported a higher ratio (4, 18). The mean age of the hemodialysis patients was 62.04 ± 15.43, with the largest patient group aged 61 - 80. In previous studies conducted in France and the U.S., the mean ages of the study populations were 53.8 ± 17.2 and 39.4 ± 8.9, respectively (23, 24). In the present study, the median survival time was 40 months, at the 95% confidence interval (28.67, 51.33). A study conducted in the U.S. reported a median survival time of 48.4 months (18), whereas another study conducted in Australia reported a median survival time of 52.3 months (25). Many studies found that a mean low serum albumin was associated with lower survival (18, 23, 26). In the present study, regarding the estimated parameter of the acceleration factor under the Weibull model , the survival time of the hemodialysis patients with a mean serum albumin higher than 4 was 1.76 times greater than that of hemodialysis patients whose mean serum albumin was less than 4. As a result, a mean serum albumin level lower than 4 was one of the major causes of hemodialysis-related deaths. The clinic that the patient attended was also a significant variable affecting patient survival. Regarding the acceleration factor estimated parameter, the survival time of the hemodialysis patients referred to Fatima Zahra hospital dialysis ward was 0.41 times than that of the patients referred to Imam Khomeini hospital. The aforementioned may be due to differences in the facilities, services, capacity, and numbers of dialysis machines at these centers, in addition to other unknown factors. This finding matches the research of Sa Carvalho et al. (27).
A strength of the present study was the inferential analysis based on an AFT assumption and the acceleration factor γ, which could be parameterized as exp(α), where α was a parameter estimated from the data.
In the present study, among the parametric models tested, the Weibull model showed the best fit in analyzing the survival of hemodialysis patients. On the one hand, the variables as mean serum albumin and clinic getting meaningful in hemodialysis patients’ survival using the Weibull model can be attributed to some unknown factors, such as personal and environmental traits of the patient being special to the person and that they create a certain trait for the patient. To cope with such a phenomenon, the Weibull and gamma frailty model could be used to evaluate the effects of prognostic factors on the survival of hemodialysis patients. In conclusion, parametric models can provide complementary data for clinicians and researchers on how risks vary over time.5.1. Limitations
The present study has some limitations. These include the flaw in the patients’ files and memory, their telephone number and addresses changing and not accessing them.
Acknowledgements
References
-
1.
Lee ET, Go OT. Survival analysis in public health research. Annu Rev Public Health. 1997;18:105-34. [PubMed ID: 9143714]. https://doi.org/10.1146/annurev.publhealth.18.1.105.
-
2.
Cox DR. Regression models and life-tables. J R Stat Soc Series B Stat Methodol. 1972;34(2):187-220.
-
3.
Therneau TM. Modeling survival data: extending the Cox model. USA: Springer; 2000.
-
4.
Hashemian AH, Beiranvand B, Rezaei M, Reissi D. A Comparison Between Cox Regression and Parametric Methods in Analyzing Kidney Transplant Survival. World App Sci J. 2013;26(4):502-7. https://doi.org/10.5829/idosi.wasj.2013.26.04.12203.
-
5.
Smeltzer S, Bare B, Hinkle J, Cheever K. Brunner & suddarth's textbook of medical-surgical nursing. 9. UK: Lippincott Williams & Wilkins; 2010. p. 1328-42.
-
6.
Fauci A, Kasper D, Hauser S, Jameson J, Loscalzo J. Harrison's Principles of Internal Medicine, Kidney & Urinary Tract Disorders. 18 ed. Iran: Samat; 2008. p. 130-6.
-
7.
Fauci, Braunwald, Kasper, Hauser, Longo. Harrison's internal medicine(disorders of the kidney & urinary tract). 17 ed. USA: Harrison's; 2008.
-
8.
Montaseri M, Yazdani J, Espahbodi F, Mousavi SJ. Five-year survival rate in hemodialysis patients Attending Sari Imam Khomeini Hospital. J Mazand Univ Med Sci. 2013;23(101):78-85.
-
9.
Lee E. Statistical Method for survival data analysis. New York: Wiley; 1992. p. 8-17.
-
10.
Weibull W. A statistical distribution function of wide applicability. J Appl Mechanics. 1951;18(3):293-7.
-
11.
Viscomi S, Pastore G, Dama E, Zuccolo L, Pearce N, Merletti F, et al. Life expectancy as an indicator of outcome in follow-up of population-based cancer registries: the example of childhood leukemia. Ann Oncol. 2006;17(1):167-71. [PubMed ID: 16249212]. https://doi.org/10.1093/annonc/mdj050.
-
12.
Limpert EW, Stahel A, Abbt M. Log-normal distributions across the sciences: keys and clues. BioScience. 2001;51(5):341-52. https://doi.org/10.1641/0006-3568(2001)051[0341:LNDATS]2.0.CO;2.
-
13.
Kleinbaum DG, Klein M, Gail M, Krickeberg K, Samet J, Tsiatis A, et al. Survival Analysis-A Self-Learning Text. 2 ed. United States of America: Springer; 2005. p. 294-5.
-
14.
Duchateau L, Janssen P. Statistics for Biology and Health-The Frailty Model. USA: Springer; 2008. p. 32-6.
-
15.
Klein JP, Moeschberger ML. Survival analysis : techniques for censored and truncated data. 2 ed. New York: Springer-Verlag; 2003.
-
16.
Cleves M, Gould W, Gutierrez R. An Introduction to Survival Analysis Using STATA. Press Publication; 2004.
-
17.
Pourhoseingholi MA, Hajizadeh E, Moghimi Dehkordi B, Safaee A, Abadi A, Zali MR. Comparing Cox regression and parametric models for survival of patients with gastric carcinoma. Asian Pac J Cancer Prev. 2007;8(3):412-6. [PubMed ID: 18159979].
-
18.
Argyropoulos C, Chang CC, Plantinga L, Fink N, Powe N, Unruh M. Considerations in the statistical analysis of hemodialysis patient survival. J Am Soc Nephrol. 2009;20(9):2034-43. [PubMed ID: 19643932]. https://doi.org/10.1681/ASN.2008050551.
-
19.
Nikulin M, Haghighi F. A Chi-Square test for Power Generalized Weibull Family for the Head-and-Neck cancer censored data. J Mathematical Sci. 2007;133:1333-52. https://doi.org/10.1007/s10958-006-0043-8.
-
20.
Ravani P, Parfrey P, MacRae J, James M, Quinn R, Malberti F, et al. Modeling survival of arteriovenous accesses for hemodialysis: semiparametric versus parametric methods. Clin J Am Soc Nephrol. 2010;5(7):1243-8. [PubMed ID: 20413435]. https://doi.org/10.2215/CJN.06190809.
-
21.
Tammadondar M, Aghighi M. Nurse and dialysis. 28. Iran: Saha; 2010.
-
22.
Shariati A, Mojerloo M, Hesam M, Mollaei E, Abbasi A, Asayesh H. Hemodialysis efficacy in patients with end stage renal disease (ESRD) in Gorgan, Northern Iran (2008). J Gorgan Uni Med sci. 2010;12(1):80-4.
-
23.
Jungers P, Massy Z, Nguyen-Khoa T, Choukroun G, Robino C, Fakhouri F. Longer Duration of Predialysis Nephrological Care is Associated with improved Long-Term Survival of Dialysis Patients. Nephrol Dialysis Transplant. 2001;16:2357-64.
-
24.
Ahuja T, Grady J, Khan S. Changing Trends in the Survival of Dialysis Patients with Human Immunodeficiency Virus in the United States. J Am Society Nephrol. 2002;13:1889-93. [PubMed ID: 12089385].
-
25.
Yeates A, Hawley C, Mundy J, Pinto N, Haluska B, Shah P. Treatment outcomes for ischemic heart disease in dialysis-dependent patients. Asian Cardiovasc Thorac Ann. 2012;20(3):281-91. [PubMed ID: 22718716]. https://doi.org/10.1177/0218492312437383.
-
26.
Jablonski A. The multidimensional characteristics of symptoms reported by patients on hemodialysis. Nephrol Nurs J. 2007;34(1):29-37. [PubMed ID: 17345690].
-
27.
Sa Carvalho M, Henderson R, Shimakura S, Sousa IP. Survival of hemodialysis patients: modeling differences in risk of dialysis centers. Int J Qual Health Care. 2003;15(3):189-96. [PubMed ID: 12803346].