Abstract
Background:
The prediction of normal tissue complications in treatment planning plays a critical role in radiation therapy of cancer.Objectives:
The aim of the current study was to evaluate mathematical models and clinical-dosimetric variables for prediction of radiation-induced hypothyroidism (RHT) in patients with head-and-neck cancer (HNC) and breast cancer (BC).Methods:
Clinical and dose-volume data from 62 patients treated with three-dimensional conformal radiation therapy were prospectively analyzed in terms of HNCs and BC. Thyroid function assessment was monitored by the level of thyroid hormones from patients’ serum samples. Cox semi-parametric regression models were used to predict the risk of RHT. Model performance and model ranking were evaluated in accordance with the area under the receiver operating characteristic curve (AUC) and Akaike’s information criterion (AIC), respectively.Results:
Out of 62 patients, 17 persons developed RHT at a median follow-up of 11.4 months after radiation therapy. Thyroid volumes above the cut-off points of 14.2 cc and 11.4 cc showed a decrease in RHT risk for patients with HNC and BC, respectively. Moreover, the thyroid mean dose above the cut-off points of 53 and 27 Gy increased the risk of RHT for patients with HNC and BC, respectively. Simple and Multiple Cox regression analyses of the complete dataset revealed that thyroid volume and thyroid mean dose were the strongest predictors of RHT. According to AUC, Boomsma’s model, and the generalized equivalent-uniform-dose (EUD) model in the HNC dataset outperformed the BC dataset.Conclusions:
The probability of RHT rises with an increase in the mean dose to the thyroid gland; however, it decreases with increasing thyroid gland volume. Regarding the AUC analysis, gEUD model showed an acceptable predictive performance; however, the logistic Boomsma’s model was somehow more effective in predicting RHT on the HNC dataset. Cella’s model revealed a relatively acceptable prediction of RHT on the BC dataset.Keywords
Radiation-induced Hypothyroidism NTCP Model Radiation Therapy gEUD Model Boomsma’s Model
1. Background
Thyroid gland, as an organ at risk, can be located inside the therapeutic beam due to its proximity to the planning tumor volume (PTV) in radiation therapy of supraclavicular lymph nodes for breast cancer (BC), neck region for head-and-neck cancer (HNC) and Hodgkin’s lymphoma (HL). Consequently, radiation-induced hypothyroidism (RHT), a frequent side-effect of radiation therapy, occurs after the therapeutic irradiation of the mentioned neoplasms (1-3). The prevalence of subclinical hypothyroidism is 8% in females and 3% in males, and the prevalence of clinical hypothyroidism varies from 1% to 2%; however, the incidence of hypothyroidism varies from 20% to 50% after radiation therapy for HNC and HL (4-6). Nevertheless, the incidence of this disease after the irradiation of the supraclavicular region in patients with BC has still remained unclear. RHT occurs at a median interval of 1.4 - 1.8 years; however, it has been reported to range from 3 months to 20 years with a peak incidence of one to three years after radiation therapy (2, 5, 7, 8). The main risk factors associated with RHT include age, gender, the addition of chemotherapy (6, 9), neck surgery, radiation dose, fractionation regimen, pretreatment thyroid volume, the irradiated volume of thyroid, and follow-up periods (10). Although previous studies have proposed a dose-dependent risk for thyroid gland dysfunction, the dose-response relationship is not clearly defined (5, 11). The studies have suggested a range of 30 - 45 Gy as the dose constraint for the thyroid gland; however, a narrow range of threshold dose has been a hotbed of debate.
The estimation of normal tissue complication probability (NTCP) has become an important factor to rank rival treatment plans and quantify mechanisms affecting the response of normal tissues and organs in radiation therapy (12, 13). Considering the fact that the radiation therapy mainly aims to eradicate the tumor by minimizing adjacent normal tissue complications, it is crucial to estimate normal tissue response to radiation more accurately during treatment planning. In this regard, NTCP models have been developed based on either radiobiological or clinical-dosimetric parameters to estimate normal tissue complications in recent decades. It is particularly crucial to use these models where a maximum therapeutic ratio is expected to accomplish (14). Thus, it would be possible to improve cancer patients’ quality of life after radiation therapy by minimizing iatrogenic disorders such as RHT.
Few studies estimated optimum parameter values of some radiobiological NTCP models in patients treated for HNCs and HL using follow-up data of patients’ serum samples (4, 15). Furthermore, several multivariable logistic NTCP models have been proposed with regard to clinical and dosimetric parameters to predict RHT (16-18).
2. Objectives
This study aimed to determine the hazard of hypothyroidism among patients treated for HNC and BC. Clinical and dosimetric factors, as well as dose-response models, were examined for associations with RHT. The diagnostic performance of the models was assessed and then compared.
3. Methods
3.1. Study Design and Participants
A prospective cohort of 66 patients with head-and-neck (n = 34) and breast (n = 32) cancers were selected using a consecutive sampling technique. Radiation therapy was performed from June 2018 to September 2019 at the Department of Radiation Oncology. The patients were treated with 6 MV photon beams using the 3D conformal technique. The procedures were performed in accordance with the ethical standards of the responsible committee on human experimentation (Tabriz University of Medical Sciences; Ethics Code: TBZMED-1026515-1395). The inclusion criteria were met in patients who obtained normal results on thyroid blood tests (thyroid-stimulating hormone (TSH), triiodothyronine (T3), thyroxine (T4), free tri-iodothyronine (FT3), and free thyroxine (FT4)) before the initiation of radiation therapy. Moreover, the authors included the patients with nasopharyngeal cancer, whose pituitary gland (DVH based) was < 40 Gy. This dose was selected according to previous studies, as > 40 - 45 Gy, which may induce central hypothyroidism. Exclusion criteria were as follows: (1) existence of primary tumors of the thyroid gland; (2) pre-existence of thyroid gland disorders; and (3) a history of partial thyroid surgery. Out of the 66 patients, three patients with HNC and one patient with BC were excluded due to missing thyroid hormone measurements during the follow-up period.
3.2. Treatment Planning and Contouring Thyroid Gland
All treatment planning procedures were performed using the treatment planning system of TiGRT (LinaTech, Sunnyvale, California) (19, 20). CT scan images with a slice thickness of 5 mm were obtained from all the patients, and dose calculations were performed with a voxel size of 2× 2× 2 mm3. To certify the accuracy of the dose calculations, quality control tests of the TiGRT system were carried out according to the International Atomic Energy Agency TECDOC 1583 report. In all the patients, the whole thyroid or a part of it was within the radiation field. The thyroid gland (consisting of the right lobe, isthmus, and left lobe) of each patient was contoured on the CT scan images in the TiGRT system. Then a cumulative dose-volume histogram (cDVH) of the thyroid gland was derived from the individual dose plans of the patients. The patients with head and neck cancers were treated with a fraction dose of 2 Gy up to total doses of 66-70 Gy in 5 consecutive days per week. The patients with breast cancer were treated either with a fraction dose of 2 Gy up to a total dose of 50 Gy or a fraction dose of 2.66 Gy up to a total dose of 42.56 Gy in 5 consecutive days per week.
3.3. Thyroid Function Assessment and Endpoint Definition
The Follow-up program included the prospective evaluation of thyroid gland function before the onset of radiation therapy and after the end of the curative radiation therapy performed every other three months, lasting for one year. Patients with hypothyroidism symptoms were excluded from the follow-up phase and were treated with levothyroxine. It was complicated to confirm the clinical diagnosis of hypothyroidism during the follow-up phase since there was no reliablie finding to distinguish whether certain symptoms of hypothyroidism could be attributed to the disease itself or the cancer treatment. Accordingly, subclinical and clinical hypothyroidism definitions were combined to create a single endpoint variable as hypothyroidism as such the patients with raised levels (> 5 μIU/mL) were classified as having hypothyroidism with or without considering reduced FT4 levels (< 0.8 ng/dL) during the one-year follow-up phase (8, 21).
3.4. NTCP Models and Dose Considerations
The complication probability of the thyroid gland (including clinical and subclinical hypothyroidism) for each patient in the HNC dataset was calculated based on four radiobiological models using BIOPLAN version 1.3.3 (22) and MATLAB (The Mathworks Inc., Natick, USA) software packages. The applied models were empirical (i.e., LKB, mean dose, and gEUD), including an organ architecture model (i.e., relative seriality) and a logistic multivariate model based on clinical-dosimetric parameters (i.e., Boomsma’s model). Moreover, the complication probability of the thyroid gland for each patient in the BC dataset was calculated using a logistic multivariate model based on clinical-dosimetric parameters (i.e., Cella’s model). The values of the parameters in the radiobiological models were extracted from the literature (5). In the case of the thyroid gland, the α/β ratio was considered to be 3 Gy (4). The NTCP models used in this study were as follows:
3.4.1. The Lyman-Burman-Kutcher (LKB) Model
The traditional Lyman model was designed to describe the complication probabilities for uniformly-irradiated whole or partial organ volumes (23). For non-uniform irradiation, several DVH-reduction algorithms were developed to convert a heterogeneous dose distribution into a homogenous partial or whole organ irradiation, resulting in the same NTCP. Among these algorithms, the effective volume method proposed by Kutcher and Burman is the most commonly used to complement the Lyman model. The combined formula is referred to as the Lyman-Kutcher-Burman (LKB) model. Model parameters are D50, n, and m. The LKB model is based on the probit formula and uses the power-law relationship defined by Mohan et al. (24), as presented in Equations 1-3.
where, Deff is the effective dose that will lead to the same NTCP as the actual non-uniform dose distribution if it is given uniformly to the entire volume, D50 is the uniform dose given to the entire organ resulting in 50% complication risk, m describes the slope of the sigmoid curve, n defines the volume effect, and Vi is the fractional organ volume receiving a dose bin of Di.
3.4.2. Generalized Equivalent Uniform Dose (gEUD) Model ()
This model is a logistic regression model coupled with the generalized-EUD reduction method presented in Equation 4.
where, k describes the slope of the response curve at D50 and is calculated as k= 4γ50, i.e., γ50 denotes the slope of dose-response curves at 50% probability of damage.
3.4.3. The Mean Dose (MD) Model
This model is derived from the LKB model when the volume effect parameter (n) is equal to one; thus, DVH is reduced to the mean dose (Dmean) (23) as presented in Equations 5-7.
3.4.4. The Relative Seriality (RS) Model
This model, known as the Källman model (26), is based on Poisson statistics and accounts for the architecture of the organ using relative seriality, s. The complication probability is presented in Equation 8. Model parameters are D50, γ, and s.
where P(D) is the Poisson dose-response relationship, D50 is the uniform dose leading to a 50% probability of damage, and γ describes the slope of the response curve at D50.
3.4.5. Boomsma’s Model
This NTCP model was first developed in a prospective cohort study conducted by Boomsma et al. for the HNC dataset for RHT complication probability. This model is a logistic regression model based on two input variables, including Vthyroid and Dmean, as well as corresponding regression coefficients (16) as presented in Equations 9
3.4.6. Cella’s Model
This NTCP model is a logistic regression model based on three input variables, including gender, the absolute thyroid volume exceeding 30 Gy, and Vthyroid as well as corresponding regression coefficients. This model was developed for RHT complication probability and confirmed the external validation of the HL and BC datasets (17), as presented in Equations 11
Furthermore, to consider the impact of non-uniform irradiation of the normal tissues, EUD (27) was used for dose distributions in empirical models, as presented in Equation 13:
where, N is the total number of voxels, Di is the dose in the ith voxel, and ‘a’ is a tissue-specific parameter describing the volume effect. Conceptually, EUD is identical to Deff presented in Equation 1.
3.5. Statistical Analysis
Both descriptive and analytical methods were applied for each dataset separately. Kaplan-Meier survival graphs were produced to present time-to-event survival curves with regard to hypothyroidism as the endpoint and to compare the survival grouped by cancer type. Survival curves are presented on a scale ranging from zero to one; however, for a better interpretation of survival, survival curves are reported in terms of percentage. Multiple semi-parametric Cox regression model was used to investigate and detect potential predictors of hypothyroidism hazard by incorporating each radiobiological model, clinical factors, and dose-volume parameters. The correlations between the variables were calculated, and collinearity between variables was considered when r > 0.8 (Spearman coefficient matrix correlation). The proportional hazard assumption was checked and confirmed. Hazard ratios, along with their 95% Cis, were reported. In simple Cox regression analysis, a preliminary P < 0.2 was considered to be statistically significant; however, P < 0.05 was considered to be statistically significant for making decisions in multiple Cox regression analyses. The models were ranked and confirmed using Akaike’s information criterion (AIC), receiver operating characteristic (ROC) curve, and area under the ROC curve (AUC) analysis. The Youden index was used to identify the cut-off point with the optimized sensitivity and specificity of the variables associated with hypothyroidism in multiple Cox regression analyses.
4. Results
4.1. Patient Characteristics
The study sample consisted of 62 patients (with a median of 53 years and a range of 31 - 85 years). Seventeen patients developed RHT at a median follow-up of 11.4 months (range 3.1 - 12.9). Of these 17 patients, 12 patients (38.7%) belonged to the HNC group, and five patients (16.1%) belonged to the BC group. Table 1 shows the demographic, clinical, and treatment characteristics of patients.
Demographic, Clinical, and Treatment Characteristics of Patients
Characteristics | No. of HNC Patients (%) | No. of BC Patients (%) |
---|---|---|
Gender | ||
Female | 11 (64.52) | 31 |
Male | 20 (35.48) | |
Age (y) | ||
Mean | 55.45 | 51.61 |
Median | 58 | 50 |
Range | 31 - 85 | 37 - 72 |
Cancer site | ||
Nasopharynx | 6 (19.35) | |
Oral cavity | 5 (16.13) | |
Hypopharynx | 7 (22.58) | |
Larynx | 6 (19.36) | |
Other | 7 (22.61) | |
Right breast | 17 (36.96) | |
Left breast | 14 (30.43) | |
Chemotherapy | ||
Concomitant chemo- radiation | 20 (64.52) | 9 (29.03) |
No concomitant chemo-radiation | 11 (35.48) | 22 (70.97) |
Neck surgery | ||
Yes | 10 (16.13) | |
No | 52 (83.87) | |
Breast surgery | ||
Lumpectomy | 5 (16.3) | |
Mastectomy | 26 (83.87) | |
Mean dose of thyroid gland (Gy) | ||
Minimum dose | 34.90 | 1.42 |
Maximum dose | 67.05 | 53.69 |
Mean dose | 55.17 | 22.06 |
Volume of thyroid gland (cc), mean ± SD | 15.80 ± 5.12 | 19.07 ± 13.03 |
4.2. The Kaplan-Meier Curves
The hazard of developing RHT after one year, which was defined as the time-to-event analysis for the complete dataset, was 27.4%, as depicted in Figure 1A. Moreover, the hazard of developing RHT after one year was categorized by cancer type (Figure 1B).
(A) Kaplan-Meier curve of radiation-induced hypothyroidism in 62 BC and HNC patients after completion of treatment. The cumulative incidence was 27.4% after one year; (B) Kaplan-Meier curve of radiation-induced hypothyroidism categorized by cancer type
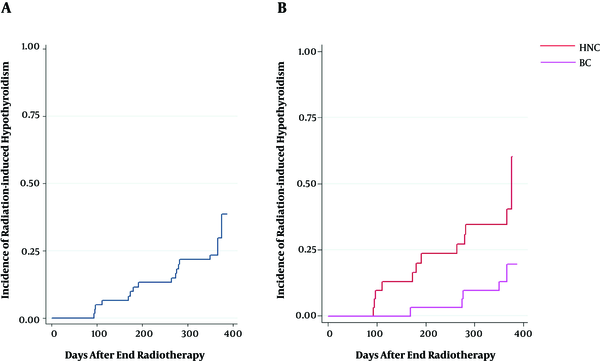
4.3. Simple Cox Regression Analysis
Among the clinical variables, only cancer type was associated with RHT in the complete dataset. A hazard ratio of 0.323 showed that the hazard of developing RHT in the BC dataset was low, compared to the HNC dataset (Table 2). In addition, the gender variable in the HNC dataset revealed that the hazard of RHT was 2.255 times greater in females than in males.
Simple Cox Regression Analysis Regarding Potential Factors and Hypothyroidism Risk
Variable | Complete Dataset | HNC Dataset | BC Dataset | |||
---|---|---|---|---|---|---|
P Value | HR (95% CI) | P Value | HR (95% CI) | P Value | HR (95% CI) | |
Gender | 0.903 | 1.061 (0.407 - 2.763) | 0.16 | 2.256 (0.725 - 7.019) | ||
Age | 0.991 | 0.669 (0.952 - 1.032) | 0.841 | 0.996 (0.956 - 1.039) | 0.204 | 0.933 (0.838 - 1.038) |
Chemotherapy | 0.812 | 0.894 (0.354 - 2.253) | 0.485 | 0.662 (0.208 - 2.105) | 0.809 | 0.760 (0.082 - 7.041) |
Cancer type | 0.034 | 0.323 (0.113 - 0.918) | ||||
Neck surgery | 0.646 | 0.729 (0.189 - 2.809) | ||||
Vthyroid | 0.001 | 0.823 (0.731 - 0.927) | 0.035 | 0.867 (0.759 - 0.990) | 0.012 | 0.704 (0.537 - 0.925) |
Dmean | 0.001 | 1.046 (1.019 - 1.074) | 0.021 | 1.069 (1.010 - 1.132) | 0.081 | 1.127 (0.341 - 0.947) |
Dmin | 0.021 | 1.024 (1.003 - 1.045) | 0.545 | 1.010 (0.978 - 1.044) | 0.07 | 2.066 (0.941 - 4.534) |
Dmax | 0.001 | 1.068 (1.029 - 1.109) | 0.03 | 1.057 (1.005 - 1.112) | 0.557 | 1.095 (0.810 - 1.480) |
V10 | 0.013 | 1.027 (1.006 - 1.048) | 0.454 | 1.035 (0.946 - 1.133) | ||
V20 | 0.012 | 1.024 (1.005 - 1.044) | 0.428 | 1.043 (0.939 - 1.157) | 0.392 | 1.043 (0.947 - 1.149) |
V30 | 0.012 | 1.016 (1.023 - 1.041) | 0.416 | 1.028 (0.962 - 1.098) | 0.284 | 1.053 (0.958 - 1.158) |
V30 (cc) | 0.036 | 0.523 (0.285 - 0.957) | ||||
V40 | 0.013 | 1.021 (1.004 - 1.037) | 0.453 | 1.014 (0.978 - 1.052) | 0.203 | 1.061 (0.968 - 1.163) |
V50 | 0.003 | 1.019 (1.006 - 1.032) | 0.172 | 1.013 (0.994 - 1.032) | 0.073 | 1.061 (0.995 - 1.132) |
V60 | 0.021 | 1.018 (1.003 - 1.033) | ||||
V70 | 0.003 | 1.028 (1.009 - 1.046) | ||||
EUD | 0.006 | 1.067 (1.018 - 1.118) | ||||
LKB | 0.187 | 1.020 (0.990 - 1.050) | ||||
gEUD | 0.035 | 1.037 (1.002 - 1.072) | ||||
MD | 0.176 | 1.021 (0.991 - 1.052) | ||||
RS | 0.159 | 1.026 (0.990 - 1.062) | ||||
Boomsma | 0.008 | 1.051 (1.013 - 1.090) | ||||
Cella | 0.004 | 1.209 (1.063 - 1.376) |
In the simple Cox regression analysis on the complete dataset, the dosimetric variables, including Vthyroid, Dmean, Dmin, Dmax, V10, V20, V30, V40, and V50 were significantly associated with RHT (Table 2).
In the simple Cox regression analysis on the HNC dataset, six dosimetric variables, including Vthyroid, Dmean, Dmax, V60, V70, EUD, and two of the dose-response models (namely gEUD and Boomsma) were independently significant predictors. According to the hazard ratios, Boomsma and gEUD models were 1.051 and 1.037 times more likely to predict RHT for the NHC dataset, respectively (Table 2).
In the simple Cox regression analysis on BC dataset, five dosimetric variables, including Vthyroid, Dmean, Dmin, V30 (cc), V50, as well as Cella’s model, could independently predict RHT. According to the hazard ratio, Cella’s model was 1.209 times more likely to predict RHT for the BC dataset (Table 2).
4.4. Multiple Cox Regression Analysis
The variables with a significant relationship with RHT in the simple Cox regression analysis (P value < 0.2) were selected for multiple analyses. On multiple cox regression analysis for the whole studied population and the HNC dataset, variables from simple cox were employed. Also, the optimal model included two variables of Dmean and Vthyroid (Table 3). Moreover, the pretreatment Vthyroid and gEUD model remained highly statistically significant for the HNC dataset. However, for this dataset, V70 was the strongest predictor in the presence of LKB, MD, and RS models (Table 3).
Multiple Cox Regression Analysis of NTCP Models Regarding Potential Factors and the Risk of Hypothyroidism
Variables | Complete Dataset | HNC Dataset | BC Dataset | |||
---|---|---|---|---|---|---|
P Value | HR (95% CI) | P Value | HR (95% CI) | P Value | HR (95% CI) | |
Model 1 | ||||||
Vthyroid | 0.002 | 0.830 (0.739 - 0.932) | 0.037 | 0.870 (0.763 - 0.992) | 0.033 | 0.672 (0.466 - 0.969) |
Dmean | 0.002 | 1.045 (1.017 - 1.074) | 0.027 | 1.066 (1.007 - 1.128) | 0.672 | 0.949 (0.745 - 1.209) |
Model 2 | ||||||
gEUD | 0.044 | 1.034 (1.001 - 1.069) | ||||
Vthyroid | 0.041 | 0.871 (0.763 - 0.994) | ||||
Cella | 0.016 | 1.309 (1.052 - 1.628) | ||||
Dmean | 0.318 | 0.852 (0.622 - 1.166) | ||||
Model 3 | ||||||
LKB | 0.654 | 0.993 (0.963 - 1.024) | ||||
V70 | 0.011 | 1.031 (1.007 - 1.056) | ||||
Cella | 0667 | 1.096 (0.722 - 1.664) | ||||
Vthyroid | 0.656 | 0.837 (0.383 - 1.831) | ||||
Model 4 | ||||||
MD | 0.685 | 0.994 (0.964 - 1.024) | ||||
V70 | 0.012 | 1.031 (1.007 - 1.055) | ||||
Cella | 0.025 | 1.202 (1.023 - 1.413) | ||||
V50 | 0.904 | 1.006 (0.905 - 1.119) | ||||
Model 5 | ||||||
RS | 0.734 | 0.994 (0.961 - 1.028) | ||||
V70 | 0.014 | 1.030 (1.006 - 1.055) |
4.5. Models’ Ranking and Comparison
Akaike’s information criterion (AIC) was used to rank the models as such the models with smaller AIC values were considered to provide a better estimation. Regarding AIC, Boomsam’s model showed the smallest value, and the gEUD model offered the next smallest value of this index in the HNC dataset (Table 4). The models were then compared using the AUCs of the ROC curves (Figures 2 and 3). In the HNC dataset, the comparison of the ROC curves revealed no significant difference in the prediction power of the NTCP models (Table 5).
Model Ranking Based on AIC for HNC Dataset
Models Used for HNC Dataset | AIC |
---|---|
Boomsma et al. | 62.513 |
gEUD | 66.597 |
Relative seriality | 70.246 |
Mean dose | 70.572 |
LKB | 70.699 |
Area Under the Receiver Operating Characteristic Curve (AUC) and 95% Confidence Intervals for Models Used in This Study on Head-and-Neck Cancer and Breast Cancer Datasets
Variable | Cutpoint | AUC | CI | P Value |
---|---|---|---|---|
HNC dataset | ||||
LKB model | 0.680 | 0.470 - 0.890 | 0.110lg, 0.731lm, 0.479lr, 0.063lb | |
gEUD model | 0.741 | 0.550 - 0.932 | 0.188gm, 0.184gr, 0.204gb | |
MD model | 0.678 | 0.466 - 0.889 | 1.000mr, 0.060mb | |
RS model | 0.678 | 0.467 - 0.888 | 0.060rb | |
Boomsma’s model | 0.844 | 0.696 - 0.993 | ||
Dmean (Gy) | 53.33 | 0.728 | 0.530 - 0.926 | |
Vthyroid (cc) | 14.21 | 0.702 | 0.505, 0.898 | |
V70 (%) | 36.79 | 0.724 | ||
BC dataset | ||||
Cella’s model | 0.804 | 0.594 - 1.000 | ||
Dmean (Gy) | 27.26 | 0.715 | 0.386 - 1.000 | |
Vthyroid (cc) | 11.42 | 0.877 | 0.633 - 1.000 | |
V30 (%) | 52.56 | 0.861 | 0.727 - 0.996 |
ROC curves for models on head-and-neck cancer dataset
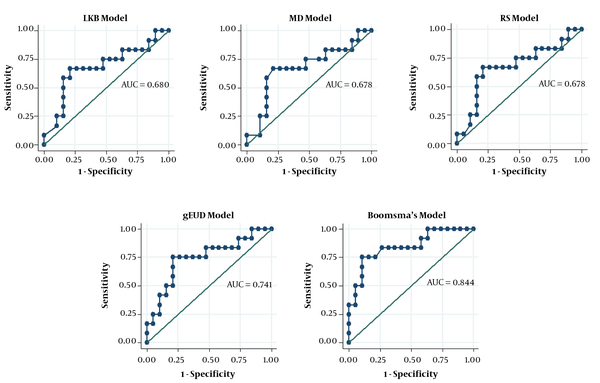
ROC curves for Cella’s model on breast cancer dataset
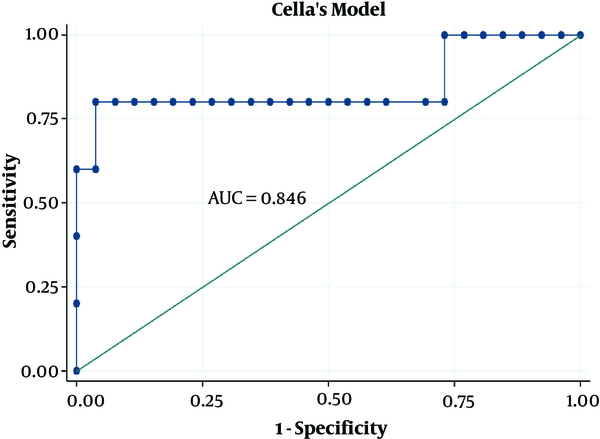
On ROC analysis of the dosimetric variables in the HNC dataset, the cut-off points evaluating the pretreatment thyroid volume in the HNC and BC datasets were 14.21 cc and 11.42 cc, respectively. The cut-off points evaluating the thyroid mean dose in HNC and BC datasets were 53.33 Gy and 27.26 Gy, respectively. Among all the dosimetric variables associated with hypothyroidism in simple Cox regression analysis, AUC was highest for the Dmean (AUC = 0.728; CI: 0.530 - 0.926) in the HNC dataset and Vthyroid (AUC = 0.877; CI: 0.633 - 1.000) in the BC dataset. Moreover, the threshold identified for V30cc was 52.6% in the BC dataset.
5. Discussion
The present study aimed to investigate the incidence rate, predictive variables, and effectiveness of mathematical models to predict RHT in patients treated by external radiation therapy for HNC and BC.
Previous studies have reported a wide range of incidence for RHT in various cohorts of patients with HNCs and HL (5, 7, 10, 11, 18, 21, 28-35); however, most papers reported the incidence of RHT following radiation therapy of HNC to vary from 20% to 50%. Similarly, the incidence rate of 38.7% found on the HNC dataset in the current study fell within this range. On the other hand, some studies have analyzed the dosimetric variables for thyroid dysfunction after radiation therapy of BC, according to which the incidence of RHT varies from 6% to 21%. In the present study, half of the patient population consisted of patients with BC, for whom the incidence rate of 16.1% was estimated. It should be noted that unclear incidences of RHT are reported in the literature for patients with BC. However, the present study showed that the incidence rates of RHT were lower for BC than HNC (3, 36, 37), as presented in Figure 1B. Furthermore, the RHT appearance depends on time, and the latency period of RHT could occur as long as 20 years (6, 38, 39). Additionally, the incidence rate might be underestimated due to inadequate follow-up periods, the decreased number of patients in the follow-up phase, and the small sample size; hence, long-term surveys are recommended to achieve a more reliable and accurate incidence rates.
Various clinical factors, such as age, gender, chemotherapy, and neck surgery are significantly associated with the development of hypothyroidism. In the present study, there was an association between gender and development of RHT, which was in accordance with the results of a previous study conducted on the patients with HL (16). However, Multiple Cox regression analyses of gender revealed no significant association with RHT.
The current analysis of this prospective study also revealed that Vthyroid was a strong significant factor of RHT in both datasets. Some authors reported a decrease in the risk of RHT with an increase in Vthyroid (16, 21, 40). Boomsma et al., Cella et al., and Ronjom et al. identified thyroid volume and a certain percentage of thyroid volume or thyroid mean dose as the most predictive factors using a logistic NTCP model (16-18, 41). However, no specific relationship between Vthyroid and RHT was clearly defined. Boomsma et al. and Diaz et al. noticed that an increase in Vthyroid corresponded to a decrease in NTCP with almost 5%/cc and 7%/cc of thyroid volume, respectively. In the present study, a value of 14.2 cc for Vthyroid in the HNC dataset was determined as a cut-off point, indicating that the RHT incidence was high for Vthyroid < 14.2 cc, This finding is in agreement with those of Zhai et al., who found a 5-fold greater risk of RHT in patients with Vthyroid < 16 cc treated for nasopharyngeal cancer (40). Similarly, the incidence rate of RHT was high among patients in the BC dataset with Vthyroid < 11.4 cc. Multiple Cox analysis also suggested that Dmean was another significant predictor of RHT in both cancers. In this study, the cut-off point revealed that Dmean > 53 Gy and Dmean > 27 Gy would be the threshold values to predict the risk of RHT in HNC and BC datasets, respectively. Similarly, in a study by Kanyilmaz et al., Dmean > 21Gy was a threshold value for the development of RHT (42). In this study, the relationship between V30 and RHT agrees well with the findings of Akyurek et al. (36). Likewise, V30 reported by Cella et al. and Pinnix et al. was the single independent predictor associated with RHT in patients treated for HL with intensity-modulated radiation therapy (31, 43). In a study by Johansen et al., patients with BC and small thyroid volumes are at risk of developing RHT since fewer tissues with irradiation doses < 30 Gy are accessible for the sufficient production of thyroxin. However, no statistically significant intergroup variations were detected between V20 and V50 in their study. Kim et al. reported that the thyroid V45 value could predict the development of RHT, and a V45 of 50% could be considered as a threshold value in treatment planning. In contrast, Diaz et al. announced that Dmin, Dmean, Dmax, and V10 to V70 were not associated with RHT. In addition, Alterio et al. indicated that Dmean, Dmin, Dmax, V10, V30, and V50 were not associated with RHT. In this study, it was noticed that two final models (namely model 1, including Vthyroid and Dmean and model 2 gEUD with Vthyroid listed in Table 3) suggested a relationship with RHT when applied on the HNC dataset.
Moreover, the findings showed that, according to the AIC index, Boomsma’s model and gEUD model were ranked as the best models when applied to the HNC dataset. It would provide more benefits to focus on these models for further investigations and their application in the clinic.
The radiobiological and logistic NTCP models applied to the study dataset are shown in Figure 3. According to the AUC analysis and confidence interval, Cella’s model was performed on the BC dataset. The robustness of Cella’s model was previously confirmed by Cella et al., who developed this model to predict RHT in HL survivors (AUC = 0.804, 95% CI 0.594 - 1.000). Furthermore, Boomsma’s model and gEUD model had high performances (AUCBoomsma = 0.844, 95% CI 0.696 - 0.993; AUCgEUD = 0.741, 95% CI 0.550 - 0.932) on HNC dataset (Table 4). This result is completely incompatible with the AUC value of 0.850 (95% CI 0.78 - 0.92) reported by Boomsma et al., who developed a model to predict RHT in HNC survivors on their HNC dataset (16).
According to the AUC values, confidence intervals, and pairwise P values obtained for each model, other models remained robust to predict RHT. These results might emphasize the necessity of using these two models for the prediction of RHT in the HNC survivors of the present research. In another study, the authors announced slight effectiveness of Cella’s model, compared to LKB and RS models applied on the LH dataset (15). Further investigations are required to validate whether radiobiological models or logistic models offer the perfect effectiveness. Finally, it should be stressed here that a reliable NTCP model can provide the required data to choose the optimum plan among the rival treatment plans for a given patient.
5.1. Conclusions
According to the present research findings, 27.4% of the participants (n = 62) developed hypothyroidism after HNC and BC irradiation. The risk of RHT in HNC and BC is strongly associated with thyroid irradiated volumes as well as thyroid mean dose. To achieve plan optimization goals, it is recommended to keep Dmean < 53 Gy for HNC and Dmean < 27 Gy for BC to reduce the risk of developing hypothyroidism remarkably. Due to the hypothyroidism risk, regular thyroid function could be helpful after radiation therapy for all patients who receive radiation for HNC and BC, especially for the irradiation of the supraclavicular region. According to the AIC and AUC values, Boomsma’s model and gEUD model offered higher prediction power for hypothyroidism after the irradiation of head-and-neck regions. In addition, Cella’s three-variable model revealed a relatively acceptable prediction of RHT following the radiation therapy of the supraclavicular region in patients with breast cancer. It is recommended to consider these two models to calculate NTCP for the thyroid gland during the treatment planning process.
Acknowledgements
References
-
1.
Alterio D, Jereczek-Fossa BA, Franchi B, D'Onofrio A, Piazzi V, Rondi E, et al. Thyroid disorders in patients treated with radiotherapy for head-and-neck cancer: a retrospective analysis of seventy-three patients. Int J Radiat Oncol Biol Phys. 2007;67(1):144-50. [PubMed ID: 17084554]. https://doi.org/10.1016/j.ijrobp.2006.08.051.
-
2.
Bethge W, Guggenberger D, Bamberg M, Kanz L, Bokemeyer C. Thyroid toxicity of treatment for Hodgkin's disease. Ann Hematol. 2000;79(3):114-8. [PubMed ID: 10803932]. https://doi.org/10.1007/s002770050565.
-
3.
Reinertsen KV, Cvancarova M, Wist E, Bjoro T, Dahl AA, Danielsen T, et al. Thyroid function in women after multimodal treatment for breast cancer stage II/III: comparison with controls from a population sample. Int J Radiat Oncol Biol Phys. 2009;75(3):764-70. [PubMed ID: 19286332]. https://doi.org/10.1016/j.ijrobp.2008.11.037.
-
4.
Bakhshandeh M, Hashemi B, Mahdavi SR, Nikoofar A, Vasheghani M, Kazemnejad A. Normal tissue complication probability modeling of radiation-induced hypothyroidism after head-and-neck radiation therapy. Int J Radiat Oncol Biol Phys. 2013;85(2):514-21. [PubMed ID: 22583606]. https://doi.org/10.1016/j.ijrobp.2012.03.034.
-
5.
Boomsma MJ, Bijl HP, Langendijk JA. Radiation-induced hypothyroidism in head and neck cancer patients: a systematic review. Radiother Oncol. 2011;99(1):1-5. [PubMed ID: 21459468]. https://doi.org/10.1016/j.radonc.2011.03.002.
-
6.
Hancock SL, Cox RS, McDougall IR. Thyroid diseases after treatment of Hodgkin's disease. N Engl J Med. 1991;325(9):599-605. [PubMed ID: 1861693]. https://doi.org/10.1056/NEJM199108293250902.
-
7.
Bhandare N, Kennedy L, Malyapa RS, Morris CG, Mendenhall WM. Primary and central hypothyroidism after radiotherapy for head-and-neck tumors. Int J Radiat Oncol Biol Phys. 2007;68(4):1131-9. [PubMed ID: 17446000]. https://doi.org/10.1016/j.ijrobp.2007.01.029.
-
8.
Kuten A, Lubochitski R, Fishman G, Dale J, Stein ME. Postradiotherapy hypothyroidism: Radiation dose response and chemotherapeutic radiosensitization at less than 40 Gy. Journal of Surgical Oncology. 1996;61(4):281-3. https://doi.org/10.1002/(sici)1096-9098(199604)61:4<281::aid-jso10>3.0.co;2-9.
-
9.
Norris AA, Amdur RJ, Morris CG, Mendenhall WM. Hypothyroidism when the thyroid is included only in the low neck field during head and neck radiotherapy. Am J Clin Oncol. 2006;29(5):442-5. [PubMed ID: 17023776]. https://doi.org/10.1097/01.coc.0000217831.23820.85.
-
10.
Murthy V, Narang K, Ghosh-Laskar S, Gupta T, Budrukkar A, Agrawal JP. Hypothyroidism after 3-dimensional conformal radiotherapy and intensity-modulated radiotherapy for head and neck cancers: prospective data from 2 randomized controlled trials. Head Neck. 2014;36(11):1573-80. [PubMed ID: 23996654]. https://doi.org/10.1002/hed.23482.
-
11.
Vogelius IR, Bentzen SM, Maraldo MV, Petersen PM, Specht L. Risk factors for radiation-induced hypothyroidism: a literature-based meta-analysis. Cancer. 2011;117(23):5250-60. [PubMed ID: 21567385]. https://doi.org/10.1002/cncr.26186.
-
12.
Mesbahi A, Rasouli N, Mohammadzadeh M, NasiriMotlagh B, Ozan T. Comparison of Radiobiological Models for Radiation Therapy Plans of Prostate Cancer: Three-dimensional Conformal versus Intensity Modulated Radiation Therapy. J Biomed Phys Eng. 2019;9(3):267-78. [PubMed ID: 31341872]. [PubMed Central ID: PMC6613163]. https://doi.org/10.31661/jbpe.v9i3Jun.655.
-
13.
Mesbahi A, Rasuli N, Nasiri B. Radiobiological model-based comparison of three-dimensional conformal and intensity-modulated radiation therapy plans for nasopharyngeal carcinoma. Iranian Journal of Medical Physics. 2017;14(4):190-6.
-
14.
El Naqa I, Pater P, Seuntjens J. Monte Carlo role in radiobiological modelling of radiotherapy outcomes. Phys Med Biol. 2012;57(11):R75-97. [PubMed ID: 22571871]. https://doi.org/10.1088/0031-9155/57/11/R75.
-
15.
D'Avino V, Conson M, Palma G, Liuzzi R, Magliulo M, Pacelli R, et al. Normal Tissue Complication Probability Models for Radiation-Induced Hypothyroidism in Hodgkin Lymphoma Survivors. Int J Radiat Oncol Biol Phys. 2016;96(2). E638. https://doi.org/10.1016/j.ijrobp.2016.06.2227.
-
16.
Boomsma MJ, Bijl HP, Christianen ME, Beetz I, Chouvalova O, Steenbakkers RJ, et al. A prospective cohort study on radiation-induced hypothyroidism: development of an NTCP model. Int J Radiat Oncol Biol Phys. 2012;84(3):e351-6. [PubMed ID: 22717243]. https://doi.org/10.1016/j.ijrobp.2012.05.020.
-
17.
Cella L, Conson M, Liuzzi R, Pacelli R. A prospective cohort study on radiation-induced hypothyroidism: development of an NTCP model. Int J Radiat Oncol Biol Phys. 2013;85:11.
-
18.
Ronjom MF, Brink C, Bentzen SM, Hegedus L, Overgaard J, Johansen J. Hypothyroidism after primary radiotherapy for head and neck squamous cell carcinoma: normal tissue complication probability modeling with latent time correction. Radiother Oncol. 2013;109(2):317-22. [PubMed ID: 23891099]. https://doi.org/10.1016/j.radonc.2013.06.029.
-
19.
Mesbahi A, Dadgar H, Ghareh-Aghaji N, Mohammadzadeh M. A Monte Carlo approach to lung dose calculation in small fields used in intensity modulated radiation therapy and stereotactic body radiation therapy. J Cancer Res Ther. 2014;10(4):896-902. [PubMed ID: 25579525]. https://doi.org/10.4103/0973-1482.137989.
-
20.
Mesbahi A, Dadgar H. Dose calculations accuracy of TiGRT treatment planning system for small IMRT beamlets in heterogeneous lung phantom. International Journal of Radiation Research. 2015;13(4):345-54.
-
21.
Diaz R, Jaboin JJ, Morales-Paliza M, Koehler E, Phillips JG, Stinson S, et al. Hypothyroidism as a consequence of intensity-modulated radiotherapy with concurrent taxane-based chemotherapy for locally advanced head-and-neck cancer. Int J Radiat Oncol Biol Phys. 2010;77(2):468-76. [PubMed ID: 19577867]. https://doi.org/10.1016/j.ijrobp.2009.05.018.
-
22.
Sanchez-Nieto B, Nahum AE. Bioplan: software for the biological evaluation of radiotherapy treatment plans. Med Dosim. 2000;25(2):71-6. https://doi.org/10.1016/s0958-3947(00)00031-5.
-
23.
Lyman JT. Complication Probability as Assessed from Dose-Volume Histograms. Radiat Res Suppl. 1985;8. S13. https://doi.org/10.2307/3583506.
-
24.
Mohan R, Mageras GS, Baldwin B, Brewster LJ, Kutcher GJ, Leibel S, et al. Clinically relevant optimization of 3-D conformal treatments. Med Phys. 1992;19(4):933-44. [PubMed ID: 1518482]. https://doi.org/10.1118/1.596781.
-
25.
Gay HA, Niemierko A. A free program for calculating EUD-based NTCP and TCP in external beam radiotherapy. Phys Med. 2007;23(3-4):115-25. [PubMed ID: 17825595]. https://doi.org/10.1016/j.ejmp.2007.07.001.
-
26.
Kallman P, Agren A, Brahme A. Tumour and normal tissue responses to fractionated non-uniform dose delivery. Int J Radiat Biol. 1992;62(2):249-62. [PubMed ID: 1355519]. https://doi.org/10.1080/09553009214552071.
-
27.
Niemierko A. Reporting and analyzing dose distributions: a concept of equivalent uniform dose. Med Phys. 1997;24(1):103-10. [PubMed ID: 9029544]. https://doi.org/10.1118/1.598063.
-
28.
Akgun Z, Atasoy BM, Ozen Z, Yavuz D, Gulluoglu B, Sengoz M, et al. V30 as a predictor for radiation-induced hypothyroidism: a dosimetric analysis in patients who received radiotherapy to the neck. Radiat Oncol. 2014;9:104. [PubMed ID: 24885512]. [PubMed Central ID: PMC4029831]. https://doi.org/10.1186/1748-717X-9-104.
-
29.
Jereczek-Fossa BA, Alterio D, Jassem J, Gibelli B, Tradati N, Orecchia R. Radiotherapy-induced thyroid disorders. Cancer Treat Rev. 2004;30(4):369-84. [PubMed ID: 15145511]. https://doi.org/10.1016/j.ctrv.2003.12.003.
-
30.
Bonato C, Severino RF, Elnecave RH. Reduced thyroid volume and hypothyroidism in survivors of childhood cancer treated with radiotherapy. J Pediatr Endocrinol Metab. 2008;21(10):943-9. [PubMed ID: 19209616]. https://doi.org/10.1515/jpem.2008.21.10.943.
-
31.
Cella L, Conson M, Caterino M, De Rosa N, Liuzzi R, Picardi M, et al. Thyroid V30 predicts radiation-induced hypothyroidism in patients treated with sequential chemo-radiotherapy for Hodgkin's lymphoma. Int J Radiat Oncol Biol Phys. 2012;82(5):1802-8. [PubMed ID: 21514076]. https://doi.org/10.1016/j.ijrobp.2010.09.054.
-
32.
Cella L, Liuzzi R, Conson M, D'Avino V, Salvatore M, Pacelli R. Development of multivariate NTCP models for radiation-induced hypothyroidism: a comparative analysis. Radiat Oncol. 2012;7:224. [PubMed ID: 23270411]. [PubMed Central ID: PMC3573930]. https://doi.org/10.1186/1748-717X-7-224.
-
33.
Hancock SL, McDougall I, Constine LS. Thyroid abnormalities after therapeutic external radiation. Int J Radiat Oncol Biol Phys. 1995;31(5):1165-70. https://doi.org/10.1016/0360-3016(95)00019-u.
-
34.
Kim MY, Yu T, Wu HG. Dose-volumetric parameters for predicting hypothyroidism after radiotherapy for head and neck cancer. Jpn J Clin Oncol. 2014;44(4):331-7. [PubMed ID: 24482412]. https://doi.org/10.1093/jjco/hyt235.
-
35.
Kumpulainen EJ, Hirvikoski PP, Virtaniemi JA, Johansson RT, Simonen PM, Terävä MT, et al. Hypothyroidism after radiotherapy for laryngeal cancer. Radiotherapy and Oncology. 2000;57(1):97-101. https://doi.org/10.1016/s0167-8140(00)00276-0.
-
36.
Akyurek S, Babalioglu I, Kenan KOSE, GOKCE SC. Thyroid dysfunction following supraclavicular irradiation in the management of carcinoma of the breast. International Journal of Hematology and Oncology. 2014;29(4):139-44.
-
37.
Kikawa Y, Kosaka Y, Hashimoto K, Hohokabe E, Takebe S, Narukami R, et al. Prevalence of hypothyroidism among patients with breast cancer treated with radiation to the supraclavicular field: a single-centre survey. ESMO Open. 2017;2(1). e000161. [PubMed ID: 28761733]. [PubMed Central ID: PMC5519789]. https://doi.org/10.1136/esmoopen-2017-000161.
-
38.
Mercado G, Adelstein DJ, Saxton JP, Secic M, Larto MA, Lavertu P. Hypothyroidism: a frequent event after radiotherapy and after radiotherapy with chemotherapy for patients with head and neck carcinoma. Cancer. 2001;92(11):2892-7. https://doi.org/10.1002/1097-0142(20011201)92:11<2892::aid-cncr10134>3.0.co;2-t.
-
39.
Tell R, Lundell G, Nilsson B, Sjodin H, Lewin F, Lewensohn R. Long-term incidence of hypothyroidism after radiotherapy in patients with head-and-neck cancer. Int J Radiat Oncol Biol Phys. 2004;60(2):395-400. [PubMed ID: 15380571]. https://doi.org/10.1016/j.ijrobp.2004.03.020.
-
40.
Zhai RP, Kong FF, Du CR, Hu CS, Ying HM. Radiation-induced hypothyroidism after IMRT for nasopharyngeal carcinoma: Clinical and dosimetric predictors in a prospective cohort study. Oral Oncol. 2017;68:44-9. [PubMed ID: 28438291]. https://doi.org/10.1016/j.oraloncology.2017.03.005.
-
41.
Ronjom MF, Brink C, Bentzen SM, Hegedus L, Overgaard J, Petersen JB, et al. External validation of a normal tissue complication probability model for radiation-induced hypothyroidism in an independent cohort. Acta Oncol. 2015;54(9):1301-9. [PubMed ID: 26248025]. https://doi.org/10.3109/0284186X.2015.1064160.
-
42.
Kanyilmaz G, Aktan M, Koc M, Demir H, Demir LS. Radiation-induced hypothyroidism in patients with breast cancer: a retrospective analysis of 243 cases. Med Dosim. 2017;42(3):190-6. [PubMed ID: 28502654]. https://doi.org/10.1016/j.meddos.2017.03.003.
-
43.
Pinnix CC, Cella L, Andraos TY, Ayoub Z, Milgrom SA, Gunther J, et al. Predictors of Hypothyroidism in Hodgkin Lymphoma Survivors After Intensity Modulated Versus 3-Dimensional Radiation Therapy. Int J Radiat Oncol Biol Phys. 2018;101(3):530-40. [PubMed ID: 29681481]. [PubMed Central ID: PMC7189963]. https://doi.org/10.1016/j.ijrobp.2018.03.003.