Abstract
Background:
Alzheimer’s disease is one form of dementia in old age. Alzheimer’s disease is among the incurable diseases which usually show its symptoms in the seventh decade of human life. The disease may be present in the body for years without the incidence of the symptoms. Considering many research studies on this disease, the cause of Alzheimer’s disease is still unknown. Alzheimer’s disease occurs hereditary or accidentally in old people.Objectives:
Based on the importance of Alzheimer’s disease and lack of a cure (definitive treatment) for this disease, we decided to use Data mining method to identify the hidden patterns in effective genes causing the incidence of this disease.Materials and Methods:
In this analytical study, we identified the genes with altered expressions in patients diagnosed with Alzheimer’s disease. In this research, we identified genes with an increase and decrease in gene expression in patients diagnosed with Alzheimer’s disease and then presented the important sequences found in each gene of this disease as Alzheimer’s biomarkers. Microarray libraries related to Alzheimer’s disease were used and finally, we weighted the data using 10 data mining methods.Results:
The sequences gain a weight more than 0.5 in at least two of the weighting algorithms, would be chosen as the most important sequenses.our work shows that TGCCCC; AGCCTG; GAATAT, AATTG; And AAATTG are the most important sequences since these sequences have been chosen as the most important sequences in 8 repetition of weithening algorithmsConclusions:
The results of this thesis are consistent with the results obtained from the previous researches and confirm the previous assumptions. The important effect of beta amyloid and E- T PAZ and Kinase and microglobulin genes in Alzheimer’s disease is validated in the previous researches too.Keywords
1. Background
Alzheimer’s is type of dementia [1] that cause problems with memory, thinking and behavior and appears in old and middle age persons [2, 3]. Alzheimer’s can cause death with killing the neuronal tissues. This disease was first announced by Alois Alzheimer, a German psychologist and neuropathologist. Dr. Alzheimer diagnosed this disease through changes in the brain tissue of a woman who had died of an unusual mental illness [2, 3]. Her symptoms included memory loss, language problems, and unpredictable behavior. The spread of Alzheimer’s is almost the same through all the world. In 2001 three occurrences of Alzheimer’s through each 100,000 person under 60 and 125 occurrences of Alzheimer’s through each 100,000 person over 60 years old was reported [4]. In 2002 nearly 1 percent of the people in developed countries were diagnosed with Alzheimer’s [5, 6]. Rapid increase in the Alzheimer’s make this disease a social concern in many countries [2, 3]. As the Alzheimer’s begins with slow loss in memory, most of the time this symptom is linked to natural aging mistakenly. This disease often can be diagnosed Retrospective and in the beginning of the disease and this make prognosis of Alzheimer very critical and essential. Unfortunately Alzheimer’s appears gradual and with some symptoms including memory loss, disorder in speaking and finding appropriate word that can be mistaken with fatigue or daily routines [1]. But as mentioned earlier prognosis of Alzheimer is very difficult through observation and traditional medical tests. Number of factors including age, environment and genetics can increase or decrease a person chance of developing the disease [7]. Since genetics is an important factor in Alzheimer’s, analyzing the gene sequences can help to prognosis the Alzheimer’s. In recent years extensive links between various fields of knowledge have been seen, especially in the fields of data mining and different sciences [8]. Data mining is related to different sciences in a wide variety of areas, Fraud detection, pattern recognition, natural language processing, data mining, bioinformatics are among some issues that have a wide link with data mining [2]. In the field of Medical Sciences, diagnosis, prognostic and treatment response rate of treatment procedures are evident examples of using data mining in medicine [3]. Given the importance of Alzheimer’s disease and the absence of a definite treatment for this disease, we decided to use data mining techniques to identify hidden patterns in effective genes involved in the incidence of the disease. In this study, data mining techniques had an important role in identifying and extracting the repetitive patterns and their importance in the incidence of Alzheimer’s disease. We used microarray library as a prototype in this study. DNA microarrays are usually identified as DND chips or biochips as well, which are a collection of microscopic DNA points connected to a solid surface. Scientists use DNA microarrays to measure the expression levels of large numbers of genes simultaneously, or the genotype various parts of a gene [9]. This might be a short segment of a gene element or other DNDs, which is used to link cDNA [4] or cRNA [5] in the above and difficult conditions. Since an array can contain tens of thousands of probes [6], we can perform many genetic tests in parallel with a microarray experiment; so microarray dramatically accelerates many types of studies [8].
2. Objectives
The aim of the research sample is to help understand better and prevent the development and spread of diseases such as Alzheimer’s disease, which there is no definitive method of treatment.
3. Materials and Methods
in this analytical study, First, we collect experiments relevant to the subject matter that are conducted by the microarray method in order to build the corresponding database, because the database for data mining models should be prepared based on the microarray method. Then, we search the microarray libraries that are appropriate to the subject under study. To do this, we should visit the (national center for biotechnology information) NCBI site. Anybody can access and download public GEO data [10]. The GEO database is designed to provide and encourage access within the scientific community to the most up to date and comprehensive gene expression. The gene expression omnibus (GEO) is a public repository that archives and freely distributes microarray, next-generation sequencing, and other forms of high-throughput functional genomic data submitted by the scientific community [11]. Alzheimer’s disease is the subject of our study. After searching the microarray library concerning Alzheimer’s’ disease, we continued our work.
Each library consists of three subcategories and their codes are also provided with their abbreviations: Sample: each microarray library consists of a set of conducted experiment samples and is formed in different times and situations and starts with the letters GSM (e.g: GSM 32536).
3.1. Series
A set of samples that are placed together to create a complete test of microarray starting with the letters JS (e.g.: GSE 8322).
3.2. Platform
Starts with the extension JPL and is a collection of series. In Figures 1, a test platform is seen.
A Platform for a Micro-Array Test
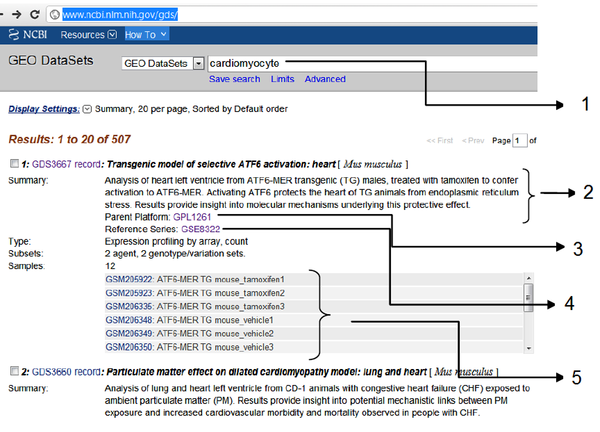
From the libraries that were found in the search, 5 Libraries had our desired conditions. Each is described in Table 1 respectively.
Downloaded Micro-Array Libraries
The Sampled Region | Sample Number | Year | Study Item | Series | |
---|---|---|---|---|---|
Control | Sick | ||||
Hippocampal Genes | 22 | 9 | 2004 | Alive Human | GSE1297 |
Entorhinal cortex | 10 | 10 | 2006 | Alive Human | GSE4757 |
Hippocampal Genes | 22 | 88 | 2011 | Alive Human | GSE28146 |
Hippocampal Genes | 8 | 8 | 2012 | Alive Mice | GSE32536 |
Temporal cortex - Frontal cortex–Hippocampal | 32 | 47 | 2013 | Alive Human | GSE6980 |
To normalize the data, it was necessary to enter them into expression console software .The reason for normalization is that normally this data contain some noise, the data must be normalized in order to remove the noise resulted from the light intensity during the test. We used RMI algorithm for normalization. RMI algorithm is (robust multi-array average: RMA), a powerful linear model in probe levels for minimizing the effect of affinity difference between specific probes. This approach increases the sensitivity to small changes in test and control samples. RMI is a multi-chip and parallel approach; therefore, all intended arrays for comparison must be included together in the summarization stage (last stage). Then we normalize all of the 5 test data and prepare them for comparing sick and healthy samples. In the next stage, we designed the test. In this stage, Bayes T was conducted on each sample v.s control sample and we can categorize, compare, and perform the algorithms according to the method proposed in the paper. Bayes T test is conducted in a way that a comparison must be made between two groups. In here, we compare two groups of sick and Healthy to identify the gene changes after being diagnosed with of Alzheimer’s disease. Then, we collect the information related to the annotation of each gene taken from the valid data base. We prepare the information to enter it into the RapidMiner software (version5) by obtaining the sequence of each gene. In this step, 10 feature selection (weighting) algorithms is applied on the data, The implementation of these algorithms is done in the RapidMiner software and as a result, we briefly represent the feature selection method in each. For more information refer to [12].
1) Algorithm information gain: this algorithm calculates feature correlation based on data use. If it is required that data collection be divided based on one feature, this operator calculates the correlation of that feature by using data use in the class distribution and attributes weights to the features.
2) Algorithm information gain ratio: the ratio of data use for class distribution
3) Algorithm rule: this algorithm obtains the correlation of one feature in a data collection by calculating the error rate of one ONE_Rule model and establishing a single rule for each of the features excluding the selected feature.
4) Algorithm deviation: this operator calculates the weights from standard deviation of all the features of a data collection. These weights can be normalized with the help of mean values, minimum and maximum of features. Normalize weights parameter activates normalization of the weights. Normalize parameter determines whether the standard deviation be divided based on minimum or the maximum amount of that feature.
5) Algorithm Chi Squared statistic: this operator calculates each set of input features by chi-squared criterion with respect to the label feature and weights the features based on it.
6) Algorithm Gini index: this weight algorithm extracts each feature with Gini index from the distribution in the relevant class
7) Algorithm uncertainty: this algorithm calculates the relationship between each input data by measuring the "symmetric uncertainty" criterion which is defined according to the equation below (Equation 1):

8) Algorithm relief: the level of association of a feature is measured by a sampled example and comparing it to the amount of the preferred feature for the closeset example in the preferred class and a different class. The obtained weight is normalized in the 0 to 1 interval.
9) Algorithm support vector machine (SVM): they are vector machines supporting a series of regulatory communicative learning procedures (supervised) for data analysis and pattern recognition and are used for classification and regression analysis. In this study, for weighting features, coefficients corresponding to the normal vector of a linear SVM are used.
10) Algorithm PCA: they use the first feature as the weighting feature and give weight to other features based on it.
4. Results
By applying the feature selection algorithms on the data, more important sequences were identified. By applying 10 feature selection algorithms on 1092 sequences, 23 sequences which had more than 5.0 weights in two algorithms were identified to be more important than other sequences. The results of each weighting algorithm or feature selection are as follows:
They are mentioned completely in Table 2. Among these sequences, TGCCCC, AGCCTG, AATTG, GAATAT, and AAATTG sequences with 8 times repetition were identified to be the most important sequences.
The Most Important Sequences
Attribute | Count |
---|---|
AATTC | 8 |
TGCCCC | 8 |
AGCCTG | 8 |
GAATAT | 8 |
AAATTG | 8 |
TGCCC | 7 |
CCTG | 6 |
ATGC | 3 |
CAGCT | 2 |
AGCTC | 2 |
GCCTG | 2 |
GCCCC | 2 |
AAATTGGC | 2 |
AAGGAGT | 2 |
AAAAAATA | 2 |
AACAATT | 2 |
AAAGN | 2 |
CCTGT | 2 |
TTGT | 2 |
CCCTGT | 2 |
G | 2 |
A | 2 |
C | 2 |
5. Discussion
The results of this study are consistent with the results obtained from the previous researches and confirm the previous assumptions. Several researches have been conducted in this field, for example; a gene expression profile of Alzheimer’s disease; gene expression biomarkers in the brain of a mouse model for Alzheimer’s disease: mining of microarray data by logic classification and feature selection; microarray analysis in Alzheimer’s disease and normal aging; a serial analysis of gene expression profile of the Alzheimer’s disease Tg2576 mouse mode; can be mentioned as studies that have been conducted in this field. In the following of this section we will compare our results with the results of these papers.
The important effect of Beta Amyloid and E- T PAZ and Kinase and macroglobulin genes in Alzheimer’s disease is validated in the previous researches too. In a research conducted in 2001 in Saint Mateu, California about Alzheimer’s disease, 31 genes increased in their expression and 87 genes decreased in their expression which was completely consistent with our samples and some of the unknown genes also were added to the list of genes previously had a role in the incidence of Alzheimer’s disease [13]. In the paper mentioned earlier the affected and unaffected regions of the brain were compared in order to find sequences with changes in their expression. The regions were divided in nine controls and six Alzheimer’s cases. “Out of 7050 sequences 118 of them were differently expressed in the amygdala and cingulate cortex on a broadly representative CDNA micro array. As the result the paper reports That identifying of these genes shows up regulated physiological correlated of pathology involve chronic inflammation, cell adhesion, cell proliferation, and protein synthesis and Conversely, down regulated correlates of pathology involve signal transduction, energy metabolism, stress response, synaptic vesicle synthesis and function, calcium binding, and cytoskeleton( 87 down regulated genes)” [13].
In our study some of the xist genes were added to the list of genes, with increase or decrease in their expressions. This result was not reported in pervious researches and shows the effect of these genes in Alzheimer’s disease.
According to difference in samples and difference in intensity of the disease between samples used in this study and other studies, some differences between the intensity of increase and decrease in gene expression can be seen. As an example, in 2004 a study conducted entitled “Micro-array analysis in Alzheimer’s disease and normal aging” which identified genes with expression change by sampling brain cortex. The results of this experiment is consistent with the results obtained from this study, as an example beta, Actin, 21 Ribosomal protein L, Eukaryotic translation initiation factor 5A, Neuronal thread protein genes in this study introduced with the highest expression [14] which also are present in the result of our study, but are not present because of the less expression change are not present between the mentioned genes, as mentioned only slight differences exist in gene expression level which is normal according to the difference in the samples.
Alzheimer’s biomarkers were identified in some mice in a research in 2011 with the title Gene expression biomarkers in the brain of a mouse model for Alzheimer’s disease: mining of microarray data by logic classification and feature selection [15]. Using methods we use in this paper. Our results match the results in that paper but for more accurate and trusted results we expand the research to human samples. To this end we use data gathered from different parts of human brain.
For us the importance of identifying the gene with change in their expression is the ability to control the disease, through the gene expression control, in many cases, after finding these genes using biomarkers.
In this study, as already expressed, samples included human and mouse with different disease intensity and male and female gender and also sampled regions in the brain including, temporal and frontal and hippocampal brain cortexes which will present comprehensive results which no study has been conducted to this extent.
Early studies similar to this study worked on a certain cortex of the brain or a certain sample, such as dead or alive human and/or mouse.
That is the reason our results are more comprehensive than other studies and genes besides the previously identified samples have been added to the list of biomarkers we identified important sequences in these genes after finding genes with expression changes.
We achieved an exact classification of sequences by performing data mining algorithm which is not relevant to the subject of this study According to the searches conducted in the research database, no research on identifying the sequences of this disease has been done in this field and this research is the first example of such researches.
After analyzing the data, we concluded that the TGCCCC, AGCCTG, GAATAT, and AAATTG expressed sequences have a significant role in the incidence of Alzheimer’s disease.
Acknowledgements
References
-
1.
Brunton LL, Chabner B, Knollmann BC. Goodman and Gilman's the pharmacological basis of therapeutics. 12. New York: McGraw-Hill Medical; 2011.
-
2.
Waldemar G, Dubois B, Emre M, Georges J, McKeith IG, Rossor M, et al. Recommendations for the diagnosis and management of Alzheimer's disease and other disorders associated with dementia: EFNS guideline. Eur J Neurol. 2007;14(1):e1-26. [PubMed ID: 17222085]. https://doi.org/10.1111/j.1468-1331.2006.01605.x.
-
3.
Tabert MH, Liu X, Doty RL, Serby M, Zamora D, Pelton GH, et al. A 10-item smell identification scale related to risk for Alzheimer's disease. Ann Neurol. 2005;58(1):155-60. [PubMed ID: 15984022]. https://doi.org/10.1002/ana.20533.
-
4.
Ropper AH. Adams and Victor's principles of neurology. 179. New York: McGraw-Hill Medical Pub. Division; 2005.
-
5.
Bacskai BJ, Klunk WE, Mathis CA, Hyman BT. Imaging amyloid-beta deposits in vivo. J Cereb Blood Flow Metab. 2002;22(9):1035-41. [PubMed ID: 12218409]. https://doi.org/10.1097/00004647-200209000-00001.
-
6.
Mori H. Untangling Alzheimer's disease from fibrous lesions of neurofibrillary tangles and senile plaques. Neuropathology. 2000;20 Suppl:S55-60. [PubMed ID: 11037189].
-
7.
Preventing Alzheimer's disease: what do we know?. 2015. Available from: https://www.nia.nih.gov/alzheimers/publication/preventing-alzheimers-disease/risk-factors-alzheimers-disease.
-
8.
DNA microarray. The Free Encyclopedia; 2015 Oct 30, 02:09 UTC. 2015. Available from: https://en.wikipedia.org/w/index.php?title=DNA_microarray&oldid=688167172.
-
9.
Blennow K, de Leon MJ, Zetterberg H. Alzheimer's disease. Lancet. 2006;368(9533):387-403. [PubMed ID: 16876668]. https://doi.org/10.1016/S0140-6736(06)69113-7.
-
10.
Frequently Asked Questions. 2015. Available from: http://www.ncbi.nlm.nih.gov/geo/info/faq.html.
-
11.
About GEO DataSets. 2015. Available from: http://www.ncbi.nlm.nih.gov/geo/info/datasets.html.
-
12.
Ebrahimie E, Ebrahimi M, Sarvestani NR, Ebrahimi M. Protein attributes contribute to halo-stability, bioinformatics approach. Saline Systems. 2011;7(1):1. [PubMed ID: 21592393]. https://doi.org/10.1186/1746-1448-7-1.
-
13.
Loring JF, Wen X, Lee JM, Seilhamer J, Somogyi R. A gene expression profile of Alzheimer's disease. DNA Cell Biol. 2001;20(11):683-95. [PubMed ID: 11788046]. https://doi.org/10.1089/10445490152717541.
-
14.
Brotons M, Koger SM. The impact of music therapy on language functioning in dementia. J Music Ther. 2000;37(3):183-95. [PubMed ID: 10990596].
-
15.
Arisi I, D'Onofrio M, Brandi R, Felsani A, Capsoni S, Drovandi G, et al. Gene expression biomarkers in the brain of a mouse model for Alzheimer's disease: mining of microarray data by logic classification and feature selection. J Alzheimers Dis. 2011;24(4):721-38. [PubMed ID: 21321390]. https://doi.org/10.3233/JAD-2011-101881.