Abstract
Background:
This study aimed to investigate the oral health presentations of coronavirus disease 2019 (COVID-19) inpatients using statistical analysis and machine learning methods before infection, during hospitalization, and after discharge from the hospital.Methods:
This cross-sectional study was conducted on 140 hospitalized COVID-19 patients with reverse transcription-polymerase chain reaction diagnosis and severe symptoms. Demographic data, clinical characteristics, oral health habits, and oral manifestations in three periods (i.e., before infection, during hospitalization, and after discharge from the hospital) were recorded through a questionnaire and oral examination. Statistical analysis and machine learning methods were used for the analysis of patients’ data.Results:
Xerostomia, dysgeusia, hypogeusia, halitosis, and a metallic taste were the most frequent oral symptoms during hospitalization, with the incidence of 68.6%, 51.4%, 49.3%, 31.4%, and 29.3% in patients, respectively. Using tobacco significantly increased the incidence of xerostomia, dysgeusia, hypogeusia, halitosis, and a metallic taste during hospitalization (P = 0.011, P = 0.001, P = 0.002, P = 0.0001, and P = 0.0001, respectively). Smoking led to increasing dysgeusia, hypogeusia, halitosis, and a metallic taste during hospitalization (P = 0.019, P = 0.014, P = 0.013, and P = 0.006, respectively). The micro-average receiver operating characteristic (ROC) curve analysis revealed that the machine learning logistic regression model achieved the highest area under the ROC curve with a value of 0.83.Conclusions:
Xerostomia and dysgeusia are the most common oral symptoms of COVID-19 patients and could be used to predict COVID-19 infection. Dysgeusia correlates with xerostomia, and it is hypothesized that xerostomia is an etiologic factor for dysgeusia. The early detection of COVID-19 can help reduce the enormous burden on healthcare systems, and machine learning is advantageous for this purpose.Keywords
COVID-19 Xerostomia Oral Health Oral Symptoms Machine Learning
1. Background
Severe acute respiratory syndrome Coronavirus 2 (SARS-CoV-2) is a single-chain ribonucleic acid virus that causes coronavirus disease 2019 (COVID-19) (1). The COVID-19 induces a global pandemic that is the most common cause of death in 2020 and 2021. The most common symptoms of COVID-19 infection are fever, sore throat, cough, dyspnea, nausea, and vomiting (2). The receptor of SARS-CoV-2 is angiotensin-converting enzyme 2 (ACE2) (3). Tongue mucosa and salivary glands have ACE-2 receptors; therefore, these organs might be the host cells for the virus that causes an inflammatory response in these organs (4). The SARS-CoV-2 can alter the oral microbiome equilibrium and predispose the patient to oral opportunistic infections (5).
In addition, pharmacotherapy for COVID-19 infection is a factor that influences oral health (6). Inflammatory cytokines and oxidative stress compounds are the other causative factors for the oral manifestations of COVID-19 infection (7). The oral signs and symptoms of COVID-19 infection include dysgeusia, oral ulcers, desquamative gingivitis, petechiae, candidiasis, red and white plaques, necrotizing ulcerative gingivitis, and herpes simplex virus lesions (8, 9). Moreover, the detection of SARS-CoV-2 in the saliva specimens is more feasible and accurate than in nasal samples (10, 11).
Mucosal ulceration is the most common oral manifestation of COVID-19 infection that is strongly affected by dental and oral hygiene (12). Moreover, the data received from the oral examination of COVID-19 patients is limited due to personal protective considerations (13). It seems that the incidence of COVID-19 oral signs and symptoms is simultaneous with decreased taste and smell (14).
2. Objectives
With regard to the lack of enough data about the oral manifestations of COVID-19 infection and the effects of oral habits on the oral manifestations of patients, this study aimed to investigate the oral health presentations of COVID-19 infection in COVID-19 inpatients before infection, during hospitalization, and 1 week to 4 months after discharge from the hospital. This study will help understand the approaches for the prediction and management of COVID-19 oral side effects.
3. Methods
This cross-sectional study was conducted in Imam Khomeini hospital, Tehran University of Medical Sciences, Tehran, Iran, from 1 September 2020 to 17 October 2020.
3.1. Ethical Statement
This study was approved by the Ethics Committee of Tehran University of Medical Sciences (ethics code: IR.TUMS.VCR.REC.1399.369). After describing the study objectives, written informed consent was obtained from all parents.
3.2. Participants
A total of 210 hospitalized patients with a definite diagnosis of COVID-19 by reverse transcription-polymerase chain reaction and severe symptoms (according to the latest World Health Organization joint report) were enrolled in this study. After obtaining signed informed consent from the participants to complete the questionnaire, the researcher completed the questionnaire by interview and oral examination of patients and collected the information about the demographic characteristics, oral health habits, oral examination, oral manifestations, and other symptoms in three periods, namely before infection, during hospitalization, and 1 week to 4 months after discharge from the hospital. Finally, from the patients enrolled in this study, the data of 140 patients’ questionnaires were acceptable and complete to perform analyses.
3.3. Questionnaire
A questionnaire was designed for this study because most of the available questionnaires did not include the epidemiological and various oral, taste, and olfactory manifestations of COVID-19 infection. The questionnaire comprised six sections with 56 items. The first section includes demographic information; the second section includes observing the oral health habits of the individual before infection; the third section includes the data related to oral and nonoral symptoms before infection; the fourth section includes the study of oral and nonoral symptoms during hospitalization; the fifth section includes observing the oral hygiene habits of the individual after discharge from the hospital; the sixth section includes the examination of the oral symptoms 1 week to 1 month after discharge from the hospital.
The questionnaires were completed over three time periods, before infection, during hospitalization, and after discharge from the hospital. The patient was asked to record his/her experiences from 1 week before hospitalization up to hospitalization to complete the questionnaire. In addition, regarding the information related to after discharge from the hospital, the patient reported his/her experiences up to 1 month after discharge from the hospital. Regarding the data during hospitalization, the questionnaire was filled by the oral examination and interview of the patients.
Demographic data included gender (female/male), age, hospitalization (day), tooth brushing (yes/no) and the number of times per day, flossing (yes/no) and the number of times per day, mouthwash use (yes/no) and the number of times per day, smoking (yes/no) and the number of times per day, tobacco use (yes/no) and the number of times per week, and alcohol consumption (yes/no) and the number of times per week. Regarding the oral symptoms of patients, xerostomia, dysgeusia, hypogeusia, halitosis, a metallic taste, oral ulcer, oral pain, oral candidiasis, gum swelling, gum bleeding, any other symptoms and oral manifestations that were not mentioned in the questionnaire items were asked before infection, during hospitalization, and after discharge from the hospital. However, oral examination during hospitalization was performed by the researcher (dentist and oral medicine specialist).
3.4. Statistical Analysis
The SPSS software (version 22; SPSS Inc., Chicago, IL, USA) and GraphPad Prism for Windows (version 9.0.0; GraphPad Software, San Diego, California) were applied to analyze the results. The Wilcoxon signed-rank test for quantitative variables and McNemar’s test for qualitative variables were used to compare the observed oral health habits measured twice (before infection and after discharge from the hospital). Spearman’s correlation analysis, logistic regression, and Wald test were used for interpretation. For comparing and modeling oral symptoms, logistic regression was used based on oral health habits. The receiver operating characteristic (ROC) curve analysis evaluated the logistic regression model as a differentiation method to predict oral manifestations in hospitalized patients versus hospitalized patients without those oral manifestations. Spearman’s correlation was calculated to identify the correlation between demographic data, oral health status, and the oral manifestations of COVID-19 patients. The results were presented as means± standard deviation, and a P-value ≤ 0.05 was considered statistically significant.
3.5. Machine Learning Analysis
The artificial neural network is a supervised machine learning method used in various classification and prediction tasks. In this study, three multiclass feed-forward fully connected neural network models were constructed to predict the oral symptoms of patients before infection, during hospitalization, and after discharge from the hospital. The first model used demographic data as input features and predicted the patients’ oral syndrome before infection. In addition to the demographic data, the second model used patients’ oral symptoms before infection and predicted patients’ oral symptoms during hospitalization. Finally, in the third model, in addition to using the demographic data and oral syndrome before infection, the oral symptoms of patients during hospitalization were used as input features. In the last model, the oral symptoms of patients after discharge from the hospital were predicted. The resultant predicted vectors of entire models were composed of five features describing the patient’s xerostomia, dysgeusia, hypogeusia, halitosis, and a metallic taste simultaneously before infection, during hospitalization, and after discharge from the hospital for up to 1 month.
The vector elements were labeled as 1 or 0, showing infection or noninfection of corresponding features for each patient. For the implementation of the proposed models, a multi-label classification task was performed using the Keras library (15), which is a high-level neural network API. In the experiments, 80% of data samples were used during the training and validation phase, and 20% of the remaining were used during the test phase. The 5-fold cross-validation was used, and the Adam optimizer was utilized for learning the neural network parameters (16). The hyperparameter settings are 100 for batch size, 0.001 for the learning rate, and 0.2 for the dropout probability. The constructed models have two hidden layers, and each hidden layer has 32 neurons. The ReLU activation function and the sigmoid activation function were used for the hidden layers and the output layer, respectively.
For the construction of the models as mentioned above, the binary cross-entropy loss function was used in the following form:
Where is the total number of classes (i.e., 5, L is the ground-truth label, and
4. Results
Table 1 shows the epidemiologic and demographic data and oral health habits of 140 participants before infection and after discharge from the hospital. Of 140 patients, 39 cases did not use a toothbrush daily. Furthermore, 113 and 121 subjects did not floss daily and did not use mouthwash weekly before infection, respectively. In addition, after discharge from the hospital, 70 patients did not use a toothbrush daily. Moreover, 120 and 131 subjects did not floss daily and did not use mouthwash weekly, respectively. Furthermore, 125, 122, and 132 subjects did not smoke daily, did not use tobacco weekly, and did not consume alcohol weekly before infection, respectively. After discharge from the hospital, smoking, tobacco use, and alcohol consumption did not change significantly. Five of the patients had type 2 diabetes. Xerostomia and dysgeusia before infection and during hospitalization were observed in all of these patients. Two of the patients had xerostomia and dysgeusia even after discharge from the hospital. In addition, two patients developed type 2 diabetes after discharge from the hospital, among whom xerostomia and dysgeusia were observed after discharge from the hospital.
Variables | Patients (n = 140) | (Maximum-Minimum) |
---|---|---|
Section (A) Demographic Data | ||
Age (y) (mean ± SD) | 53.78 ± 17.44 | (15 - 92) |
Gender | ||
Male | 62 (44.29) | |
Female | 78 (55.71) | |
Denture | ||
Yes | 42 (30.0) | |
No | 98 (70.0) | |
Diabetes type 2 | ||
Yes | 5 (2.85) | |
No | 135 (97.15) | |
Hospitalization (day) | 7.41 ± 5.24 | (1 - 31) |
Dental visit (yearly) | 0.63 ± 1.01 | (0 - 5) |
Yes | 52 (37.14) | |
No | 88 (62.86) | |
Section (B) Before Infection | ||
Toothbrush/daily | 1.19 ± 0.99 | (0 - 5) |
Flossing/daily | 0.30 ± 0.69 | (0 - 3) |
Mouthwash/daily | 0.21 ± 0.61 | (0 - 3) |
Smoking/daily | 0.34 ± 1.43 | (0 - 10) |
Tobacco/weekly | 0.34 ± 0.98 | (0 - 5) |
Alcohol/weekly | 0.11 ± 0.58 | (0 - 6) |
Section (C) After Discharge from the Hospital | ||
Toothbrush/daily | 0.89 ± 1.11 | (0 - 5) |
Flossing/daily | 0.23 ± 0.64 | (0 - 3) |
Mouthwash/daily | 0.08 ± 0.37 | (0 - 3) |
Smoking/daily | 0.33 ± 1.15 | (0 - 6) |
Tobacco/weekly | 0.26 ± 0.83 | (0 - 4) |
Alcohol/weekly | 0.029 ± 0.17 | (0 - 1) |
Regarding the comparison of oral health habits before and during hospitalization and based on the Wilcoxon signed-rank test, there was a significant decrease in tooth brushing (P = 0.001), mouthwash use (P = 0.001), weekly tobacco use (P = 0.005), and weekly alcohol consumption (P = 0.02) after discharge from hospital. However, the difference between the frequency of dental flossing (P = 0.113) and smoking (P = 0.167) was not statistically significant. Figure 1A depicts the frequencies of oral symptoms in patients and their comparisons before infection, during hospitalization, and after discharge from the hospital. As shown in Figure 1A, xerostomia, dysgeusia, hypogeusia, halitosis, and a metallic taste were the most frequent oral symptoms during hospitalization. Figures 1A and B illustrate the comparison of the oral symptoms in different periods (i.e., before infection, during hospitalization, and after discharge from the hospital) with P-values. Oral ulcers, oral pain, and oral candidiasis were oral symptoms with less frequency and no significant difference between before infection and during hospitalization. Gum swelling (12.85%) and gum bleeding (12.14%) during hospitalization were reported in some patients.
Frequency of A, oral; and B, Nonoral symptoms in patients and their comparisons before infection, during hospitalization, and after discharge from hospital (* P > 0.05, ** P < 0.05, *** P < 0.001); Incidence of Xerostomia in Coronavirus diseases 2019 patients categorized based on gender and age in three periods; before C, infection; D, during Hospitalization; and E, after discharge from hospital.
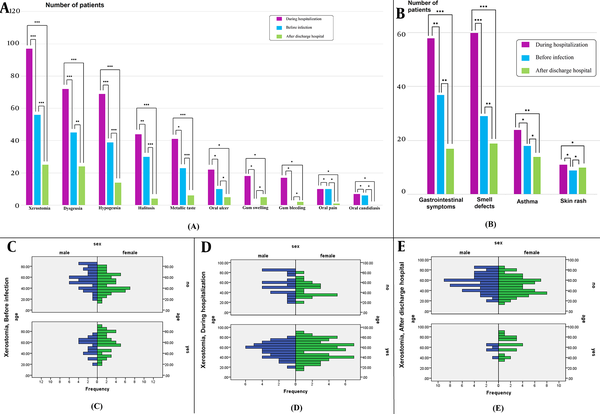
4.1. Xerostomia
The incidence of xerostomia in COVID-19 patients during hospitalization was 68.6% (n = 96), which was significantly higher than before and after discharge from the hospital (P < 0.001). There was no statistically significant difference in xerostomia between male and female patients before infection (P = 0.298) and during hospitalization (P = 0.201) based on Fisher’s exact test. However, xerostomia after discharge from the hospital was significantly lower in female patients than in male patients (P = 0.006) (Figures 1C - E). Tobacco use had a statistically significant effect on the incidence of xerostomia during hospitalization (P = 0.011). Based on the Mann-Whitney U test, with increasing age, xerostomia after discharge from the hospital increased significantly (P = 0.008).
4.2. Dysgeusia
The incidence of dysgeusia during hospitalization was 51.4% (n = 72). Based on Cochran’s test, the incidence of dysgeusia during hospitalization was significantly higher than before infection and after discharge from the hospital (P ≤ 0.001). The incidence of dysgeusia before the disease was significantly higher in female patients than in male patients (P = 0.006); however, there was no significant difference between male and female subjects in dysgeusia during hospitalization and after discharge from the hospital. Dysgeusia during hospitalization in smoking patients was increased (P = 0.019). Using tobacco increased dysgeusia before infection (P = 0.007) and during hospitalization (P = 0.001), respectively.
4.3. Hypogeusia
In this study, 69 patients (49.3%) experienced hypogeusia during hospitalization. Smoking led to increasing hypogeusia during hospitalization (P = 0.014). Using tobacco increased hypogeusia before infection (P = 0.002) and during hospitalization (P = 0.002). Using mouthwash led to decreasing hypogeusia during hospitalization (P = 0.001).
4.4. Halitosis
The rate of halitosis during hospitalization was 31.4% (n = 44). Tooth brushing caused decreased halitosis before infection (P = 0.009) and during hospitalization (P = 0.024). Smoking (P = 0.013) and using tobacco (P = 0.001) increased halitosis during hospitalization, respectively.
4.5. Metallic Taste
The rate of metallic taste sensation during hospitalization was 29.3% (n = 44). Tooth brushing caused a decreased metallic taste sensation before (P = 0.001) and during hospitalization (P = 0.026). During hospitalization, metallic taste sensation significantly increased in smokers (P = 0.006) and tobacco users (P = 0.0001). Using mouthwash decreased metallic taste sensation during hospitalization (P = 0.035).
4.6. Nonoral Symptoms
The common nonoral symptoms of hospitalized COVID-19 patients were gastrointestinal symptoms, smell defects, asthma, and skin rashes (Figure 1B). Other nonoral symptoms reported by patients were cough, malaise, myalgia, anorexia, respiratory distress, and olfactory dysfunction. Spearman’s correlations were performed to examine the relationship between oral health status and oral and nonoral symptoms. There was a statistically significant positive correlation between tobacco with (1) dysgeusia before infection (r = 0.638, P < 0.001); (2) hypogeusia before infection (r = 0.582, P < 0.001); (3) xerostomia during hospitalization (r = 0.662, P < 0.001); (4) dysgeusia during hospitalization (r = 0.675, P < 0.001); (5) hypogeusia during hospitalization (r = 0.667, P < 0.001); (6) halitosis during hospitalization (r = 0.624, P < 0.001); and (7) a metallic taste during hospitalization (r = 0.640, P < 0.001).
Logistic regression was used to model oral symptoms based on the demographic data and oral health habits. Due to the numerous variables and the probability of their interaction with each other, it was preferred to perform logistic regression that showed the real and independent effect of each variable without being affected by other variables on the oral symptoms (Table 2). Along with the results of correlation analysis, as shown in Table 2, tobacco use was a more effective factor than other oral health factors in the development of oral symptoms. The odds ratio (OR) and confidence interval (CI) of tobacco use in the logistic regression model of dysgeusia before infection were 6.307 and 1.620 - 24.554 (95% CI), respectively. The OR and CI of using tobacco in the logistic regression model of hypogeusia before infection were 9.172 and 2.353 - 35.745 (95% CI), respectively.
Evaluation of Demographic Data and Oral Health Status as Predictors of Oral Symptoms in Coronavirus Disease 2019 Patients Using Logistic Regression a
Variables | P-Value, Odds Ratio for P ≤ 0.05 | |||||||||
---|---|---|---|---|---|---|---|---|---|---|
Overall Percentage | Age (y) | Gender | Hospitalization (day) | Toothbrush | Smoking | Alcohol | Mouthwash | Tobacco | Flossing | |
Before Infection | ||||||||||
Xerostomia | 66.9 | 0.198 | 0.416 | 0.833 | 0.026, 0.373 | 0.449 | 0.682 | 0.236 | 0.211 | 0.223 |
Dysgeusia | 74.1 | 0.040, 0.027 | 0.0001, 0.113 | 0.642 | 0.888 | 0.032, 0.53 | 0.187 | 0.224 | 0.008, 6.307 | 0.811 |
Hypogeusia | 75.5 | 0.035, 0.029 | 0.013, 0.264 | 0.863 | 0.614 | 0.341 | 0.238 | 0.235 | 0.001, 9.172 | 0.418 |
Halitosis | 84.2 | 0.716 | 0.548 | 0.723 | 0.016, 0.254 | 0.362 | 0.699 | 0.905 | 0.207 | 0.920 |
Metallic taste | 79.1 | 0.918 | 0.685 | 0.814 | 0.001, 0.159 | 0.742 | 0.494 | 0.730 | 0.525 | 0.241 |
Oral ulcer | 92.8 | 0.342 | 0.615 | 0.177 | 0.249 | 0.775 | 0.999 | 0.998 | 0.839 | 0.997 |
Oral pain | 92.8 | 0.586 | 0.664 | 0.243 | 0.955 | 0.998 | 0.314 | 0.897 | 0.998 | 0.641 |
During Hospitalization | ||||||||||
Xerostomia | 69.1 | 0.119 | 0.038, -0.933 | 0.310 | 0.484 | 0.637 | 0.317 | 0.152 | 0.024, 20.330 | 0.478 |
Dysgeusia | 70.5 | 0.187 | 0.007. 1.167 | 0.202 | 0.512 | 0.038, 5.028 | 0.880 | 0.515 | 0.003, 14.717 | 0.683 |
Hypogeusia | 65.5 | 0.205 | 0.025, 0.956 | 0.198 | 0.832 | 0.046, 4.616 | 0.778 | 0.023, 0.196 | 0.007, 10.141 | 0.353 |
Halitosis | 79.9 | 0.921 | 0.631 | 0.263 | 0.119 | 0.155 | 0.040, .061 | 0.371 | 0.003, 11.611 | 0.175 |
Metallic taste | 76.3 | 0.360 | 0.238 | 0.756 | 0.064 | 0.099 | 0.964 | 0.282 | 0.001, 11.011 | 0.523 |
Oral ulcer | 84.9 | 0.088 | 0.623 | 0.415 | 0.087 | 0.358 | 0.614 | 0.515 | 0.018, 6.455 | 0.374 |
Oral pain | 92.8 | 0.746 | 0.260 | 0.097 | 0.538 | 0.673 | 0.999 | 0.998 | 0.998 | 0.998 |
Gum swelling | 87.1 | 0.374 | 0.445 | 0.250 | 0.084 | 0.790 | 0.421 | 0.732 | 0.196 | 0.836 |
Gum bleeding | 87.8 | 0.021, 0.046 | 0.813 | 0.540 | 0.378 | 0.839 | 0.145 | 0.469 | 0.296 | 0.581 |
After Discharge from Hospital | ||||||||||
Xerostomia | 84.9 | 0.011, 0.039 | 0.080 | 0.567 | 0.019, 3.656 | 0.855 | 0.909 | 0.998 | 0.999 | 0.885 |
Dysgeusia | 83.5 | 0.012, 0.042 | 0.240 | 0.188 | 0.829 | 0.026, 12.336 | 0.896 | 0.053 | 0.999 | 0.616 |
Hypogeusia | 89.9 | 0.858 | 0.683 | 0.583 | 0.132 | 0.339 | 0.647 | 0.253 | 0.999 | 0.569 |
4.7. ROC Analysis
The ROC curve analyses were carried out based on the demographic data and oral health status using logistic regression to predict xerostomia, dysgeusia, hypogeusia, halitosis, and a metallic taste during hospitalization in COVID-19 patients (Figure 2A). The ROC curve analysis based on the demographic data, oral health status, and oral manifestations before infection showed the ability of ROC curve analysis to predict xerostomia, dysgeusia, hypogeusia, halitosis, and a metallic taste in COVID-19 patients after discharge from the hospital (Figure 2B). This study showed that xerostomia, dysgeusia, hypogeusia, halitosis, metallic taste sensation, and gingival bleeding increased significantly during hospitalization.
A, Receiver operating characteristic (ROC) curve of demographic data and oral health status before infection in predicting xerostomia, dysgeusia, hypogeusia, halitosis, and metallic taste during hospitalization in Coronavirus diseases 2019 (COVID-19) patients; B, ROC curve analysis of demographic data, oral health status, and oral manifestations during hospitalization in predicting xerostomia, dysgeusia, hypogeusia, halitosis, and metallic taste after discharge from hospital in COVID-19 patients.
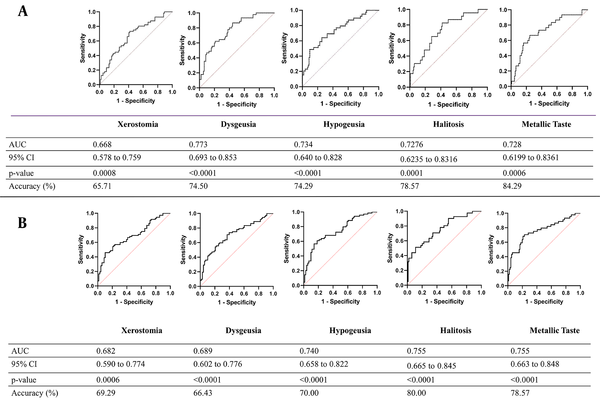
4.8. Machine Learning Results
The implementation of the above-mentioned models to predict the oral symptoms of patients before infection, during hospitalization, and after discharge from the hospital revealed that the sensitivity, specificity, and accuracy of model A were 76.70, 85.01, and 26.80, respectively; the aforementioned values for model B were 81.40, 74.50, and 77.62, respectively. The sensitivity, specificity, and accuracy of model C were 93.40, 79.22, and 54.55. The cut-off value of 0.5 was used for all the classes.
It was observed that model C, which used the demographic data, the oral syndrome before infection, and the oral symptoms of patients during hospitalization as the input features, compared to models A and B, achieved the highest accuracy. The micro-average ROC curve analysis (17) of the models revealed that model C obtained the highest area under the ROC curve (AUC) with a value of 0.83. Model B achieved the value of 0.73, and model A had the lowest AUC with 0.69.
As depicted in Figure 3, in another experiment, a feed-forward fully connected neural network model was constructed for each oral symptom separately to predict its value using demographic features and other oral symptoms before infection, during hospitalization, and after discharge from the hospital. The ROC analysis was used to predict oral symptoms separately before infection using demographic features. It was observed that the AUC-ROC of the model, which predicted xerostomia, had a value of 0.69 and achieved the highest value in other models. The AUC-ROC values for other models that predicted dysgeusia, hypogeusia, halitosis, and a metallic taste were 0.57, 0.50, 0.55, and 0.47, respectively.
Receiver operating characteristic curve analysis of prediction of oral symptoms before infection, during hospitalization, and after discharge from hospital.
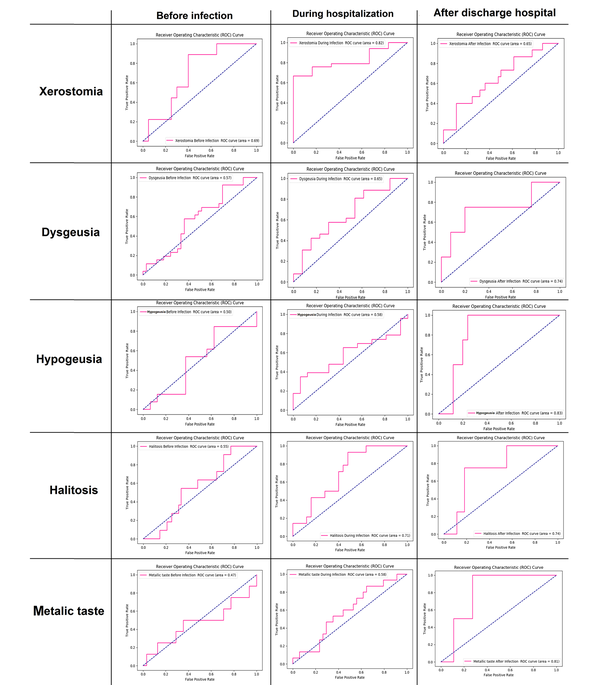
The ROC analysis for predicting oral symptoms during hospitalization using demographic features and oral symptoms before infection showed that the model that predicted xerostomia achieved the highest value in other models. The AUC-ROC of the model related to xerostomia had a value of 0.82. The AUC-ROC value for other models related to dysgeusia, hypogeusia, halitosis, and a metallic taste were 0.65, 0.58, 0.71, and 0.58, respectively. Using oral symptoms before infection in addition to demographic features improved the results of the previous experiment, wherein merely demographic features were used as the input of models. The ROC analysis for predicting oral symptoms after discharge from the hospital using demographic features, oral symptoms before infection, and oral symptoms during hospitalization showed that, unlike previous experiments, the model that predicted hypogeusia achieved the highest AUC-ROC with the value of 0.83. The AUC-ROC values for other models related to xerostomia, dysgeusia, halitosis, and a metallic taste were 0.65, 0.74, 0.74, and 0.81, respectively. The results showed the effectiveness of demographic features, oral symptoms before infection, and oral symptoms during hospitalization in the diagnosis of oral symptoms after discharge from the hospital.
5. Discussion
Coronavirus infection has various manifestations in systemic organs. In the present study, the most commonly reported oral symptom was xerostomia (68.6%). Xerostomia is defined as the sensation of dry mouth (18). There are various hypotheses for the etiology of xerostomia in COVID-19 patients. Some authors attribute xerostomia to the neuroinvasive and neurotropism potential of the SARS-CoV-2 (19). The other cause of xerostomia is ACE2, as the major entry site of SARS-CoV-2, in the salivary gland ductal cells (20). Therefore, xerostomia results from salivary gland infection (21). The incidence of xerostomia in female subjects is lower than in male subjects, contradicting the results of Biadsee et al.’s study (22).
The results of the present study indicated that there was a significant relationship between xerostomia, dysgeusia, and hypogeusia. It has been hypothesized that SARS-CoV-2 alters saliva composition by salivary gland infection (23). Alteration in salivary composition resulted in dysgeusia and hypogeusia (11). Xerostomia is a common symptom of COVID-19 infection approved in a study by Tsuchiya. (24). Additionally, xerostomia and dysgeusia are mentioned as oral manifestations of diabetic patients (25, 26). The observations of diabetic patients in this study showed that xerostomia and dysgeusia were exacerbated in diabetic patients and even after discharge from the hospital.
Dysgeusia is the other common symptom more prevalent in smokers and those who use tobacco (27, 28). Dysgeusia is reported in previous studies in almost half of COVID-19 patients (29, 30). One suggested mechanism for dysgeusia is the potential of SARS-CoV-2 to bind to ACE2, which is expressed in the taste buds on the tongue (31, 32). The infection of taste buds by COVID-19 results in dysgeusia or ageusia (29, 33). In the present study, dysgeusia correlated with xerostomia, and it was hypothesized that xerostomia was an etiologic factor for dysgeusia.
Smoking and using tobacco increased the incidence of halitosis, and tooth brushing and using mouthwash were accompanied by a lower prevalence of halitosis. Halitosis in COVID-19 patients is attributed to the epithelial alterations of desquamated keratinized tongue mucosa, xerostomia, and mouth breathing (21). Additionally, antiviral medications prescribed to treat COVID-19 alter the microbiome of the oral environment, and the proliferation of certain bacterial species results in halitosis (4, 5). Furthermore, the negative impact of disease on oral hygiene habits is the other cause of halitosis.
The findings of the present study indicated that oral hygiene habits, including tooth brushing and using mouthwash, decreased xerostomia, halitosis, and dysgeusia, which is in line with the results of other studies. Oral hygiene habits, including tooth brushing and using mouthwash, decreased significantly during hospitalization, and the causes of oral symptom exacerbation during hospitalization are important. It could be concluded that dentists’ role in the diagnosis and management of COVID-19 oral symptoms and oral hygiene instructions during hospitalization is critical. Xerostomia and dysgeusia are the most common oral symptoms of COVID-19 patients and could predict COVID-19 infection.
Machine learning has significant applications in the COVID-19 pandemic (34). The applications of machine learning in the COVID-19 pandemic include the analysis of medical imaging of infected patients, provision of an intelligent platform for healthcare, precise and personalized treatment of the patient, identification of fever, cough, and cold symptoms, proper health monitoring, patient screening, and prediction of future disease symptoms (35). In this study, machine learning results indicated that before and during hospitalization, the prediction of xerostomia achieved the highest accuracy in other oral symptoms. After discharge from the hospital, the model which predicted hypogeusia achieved the highest accuracy in other symptoms; the aforementioned results are in line with the statistical analysis of the present study.
Studies used machine learning methods to predict the symptoms of COVID-19 infection (36, 37). Callejon-Leblic et al. indicated that machine learning algorithms are helpful tools for COVID-19 diagnosis based on taste and smell disorders (38). The present study confirms the role of machine learning in predicting COVID-19 infection and surveying comprehensive oral symptoms for more accurate and reliable prediction and diagnosis. The early detection of COVID-19 can help reduce the enormous burden on healthcare systems, and machine learning is advantageous for this purpose.
References
-
1.
Castagnoli R, Votto M, Licari A, Brambilla I, Bruno R, Perlini S, et al. Severe Acute Respiratory Syndrome Coronavirus 2 (SARS-CoV-2) Infection in Children and Adolescents: A Systematic Review. JAMA Pediatr. 2020;174(9):882-9. [PubMed ID: 32320004]. https://doi.org/10.1001/jamapediatrics.2020.1467.
-
2.
Sun P, Ren J, Li K, Qie S, Liu Z, Xi J. Response to: Sore throat in COVID-19: comment on "Clinical characteristics of hospitalized patients with SARS-CoV-2 infection: A single arm meta-analysis". J Med Virol. 2020;92(7):716-8. [PubMed ID: 32239736]. [PubMed Central ID: PMC7228208]. https://doi.org/10.1002/jmv.25818.
-
3.
Ni W, Yang X, Yang D, Bao J, Li R, Xiao Y, et al. Role of angiotensin-converting enzyme 2 (ACE2) in COVID-19. Crit Care. 2020;24(1):422. [PubMed ID: 32660650]. [PubMed Central ID: PMC7356137]. https://doi.org/10.1186/s13054-020-03120-0.
-
4.
Atukorallaya DS, Ratnayake RK. Oral Mucosa, Saliva, and COVID-19 Infection in Oral Health Care. Front Med (Lausanne). 2021;8:656926. [PubMed ID: 33968961]. [PubMed Central ID: PMC8100190]. https://doi.org/10.3389/fmed.2021.656926.
-
5.
Soffritti I, D'Accolti M, Fabbri C, Passaro A, Manfredini R, Zuliani G, et al. Oral Microbiome Dysbiosis Is Associated With Symptoms Severity and Local Immune/Inflammatory Response in COVID-19 Patients: A Cross-Sectional Study. Front Microbiol. 2021;12:687513. [PubMed ID: 34248910]. [PubMed Central ID: PMC8261071]. https://doi.org/10.3389/fmicb.2021.687513.
-
6.
Dziedzic A, Wojtyczka R. The impact of coronavirus infectious disease 19 (COVID-19) on oral health. Oral Dis. 2021;27(Suppl 3):703-6. [PubMed ID: 32304276]. [PubMed Central ID: PMC7264805]. https://doi.org/10.1111/odi.13359.
-
7.
Botros N, Iyer P, Ojcius DM. Is there an association between oral health and severity of COVID-19 complications? Biomed J. 2020;43(4):325-7. [PubMed ID: 32713780]. [PubMed Central ID: PMC7258848]. https://doi.org/10.1016/j.bj.2020.05.016.
-
8.
Farid H, Khan M, Jamal S, Ghafoor R. Oral manifestations of Covid-19-A literature review. Rev Med Virol. 2022;32(1). e2248. [PubMed ID: 34028129]. [PubMed Central ID: PMC8209937]. https://doi.org/10.1002/rmv.2248.
-
9.
Halboub E, Al-Maweri SA, Alanazi RH, Qaid NM, Abdulrab S. Orofacial manifestations of COVID-19: a brief review of the published literature. Braz Oral Res. 2020;34. e124. [PubMed ID: 33146320]. https://doi.org/10.1590/1807-3107bor-2020.vol34.0124.
-
10.
Teo AKJ, Choudhury Y, Tan IB, Cher CY, Chew SH, Wan ZY, et al. Author Correction: Saliva is more sensitive than nasopharyngeal or nasal swabs for diagnosis of asymptomatic and mild COVID-19 infection. Sci Rep. 2021;11(1):12538. [PubMed ID: 34108515]. [PubMed Central ID: PMC8188150]. https://doi.org/10.1038/s41598-021-89880-3.
-
11.
da Silva Pedrosa M, Sipert CR, Nogueira FN. Altered taste in patients with COVID-19: The potential role of salivary glands. Oral Dis. 2021;27 Suppl 3:798-800. [PubMed ID: 32564416]. [PubMed Central ID: PMC7323242]. https://doi.org/10.1111/odi.13496.
-
12.
Wu YH, Wu YC, Lang MJ, Lee YP, Jin YT, Chiang CP. Review of oral ulcerative lesions in COVID-19 patients: A comprehensive study of 51 cases. J Dent Sci. 2021;16(4):1066-73. [PubMed ID: 34306539]. [PubMed Central ID: PMC8279930]. https://doi.org/10.1016/j.jds.2021.07.001.
-
13.
Gallagher JE, Johnson I, Verbeek JH, Clarkson JE, Innes N. Relevance and paucity of evidence: a dental perspective on personal protective equipment during the COVID-19 pandemic. Br Dent J. 2020;229(2):121-4. [PubMed ID: 32710059]. [PubMed Central ID: PMC7380159]. https://doi.org/10.1038/s41415-020-1843-9.
-
14.
Villarreal IM, Morato M, Martinez-RuizCoello M, Navarro A, Garcia-Chilleron R, Ruiz A, et al. Olfactory and taste disorders in healthcare workers with COVID-19 infection. Eur Arch Otorhinolaryngol. 2021;278(6):2123-7. [PubMed ID: 32725271]. [PubMed Central ID: PMC7385204]. https://doi.org/10.1007/s00405-020-06237-8.
-
15.
Chollet F. Keras. San Francisco, USA: GitHub Inc; 2015. Available from: https://github.com/keras-team/keras.
-
16.
Kingma DP, Ba J, editors. Adam: A method for stochastic optimization. 3rd International Conference for Learning Representations (ICLR). 2014; San Diego, USA. Addis Ababa, Ethiopia; 2014.
-
17.
Sun C, Bai Y, Chen D, He L, Zhu J, Ding X, et al. Accurate classification of COVID-19 patients with different severity via machine learning. Clin Transl Med. 2021;11(3). e323. [PubMed ID: 33784017]. [PubMed Central ID: PMC7908044]. https://doi.org/10.1002/ctm2.323.
-
18.
Diep MT, Jensen JL, Skudutyte-Rysstad R, Young A, Sodal ATT, Petrovski BE, et al. Xerostomia and hyposalivation among a 65-yr-old population living in Oslo, Norway. Eur J Oral Sci. 2021;129(1). e12757. [PubMed ID: 33501713]. [PubMed Central ID: PMC7986810]. https://doi.org/10.1111/eos.12757.
-
19.
Saniasiaya J. Xerostomia and COVID-19: Unleashing Pandora's Box. Ear Nose Throat J. 2021;100(2_suppl):139S. [PubMed ID: 32955342]. https://doi.org/10.1177/0145561320960353.
-
20.
Roshanravan N, Ghaffari S, Hedayati M. Angiotensin converting enzyme-2 as therapeutic target in COVID-19. Diabetes Metab Syndr. 2020;14(4):637-9. [PubMed ID: 32428864]. [PubMed Central ID: PMC7214324]. https://doi.org/10.1016/j.dsx.2020.05.022.
-
21.
Brandini DA, Takamiya AS, Thakkar P, Schaller S, Rahat R, Naqvi AR. Covid-19 and oral diseases: Crosstalk, synergy or association? Rev Med Virol. 2021;31(6). e2226. [PubMed ID: 33646645]. [PubMed Central ID: PMC8014590]. https://doi.org/10.1002/rmv.2226.
-
22.
Biadsee A, Biadsee A, Kassem F, Dagan O, Masarwa S, Ormianer Z. Olfactory and Oral Manifestations of COVID-19: Sex-Related Symptoms-A Potential Pathway to Early Diagnosis. Otolaryngol Head Neck Surg. 2020;163(4):722-8. [PubMed ID: 32539587]. [PubMed Central ID: PMC7298562]. https://doi.org/10.1177/0194599820934380.
-
23.
Abduljabbar T, Alhamdan RS, Al Deeb M, AlAali KA, Vohra F. Association of Salivary Content Alteration and Early Ageusia Symptoms in COVID-19 Infections: A Systematic Review. Eur J Dent. 2020;14(S 01):S152-8. [PubMed ID: 33242917]. [PubMed Central ID: PMC7775251]. https://doi.org/10.1055/s-0040-1716986.
-
24.
Tsuchiya H. Oral Symptoms Associated with COVID-19 and Their Pathogenic Mechanisms: A Literature Review. Dent J (Basel). 2021;9(3). [PubMed ID: 33799583]. [PubMed Central ID: PMC7999671]. https://doi.org/10.3390/dj9030032.
-
25.
Matsunaga K, Yoshida Y, Takemaru M, Yamashiro K, Monden I, Inohara K, et al. Multidisciplinary clinical approach by sharing oral examination information to treat a diabetes patient with dysgeusia. Clin Case Rep. 2019;7(5):877-80. [PubMed ID: 31110707]. [PubMed Central ID: PMC6509891]. https://doi.org/10.1002/ccr3.2111.
-
26.
Afsaneh Abadi P, Koopaie M, Montazeri R. Comparison of salivary nitric oxide and oral health in diabetic patients with and without xerostomia. Diabetes Metab Syndr. 2020;14(1):11-5. [PubMed ID: 31809967]. https://doi.org/10.1016/j.dsx.2019.11.014.
-
27.
Gallo O. Risk for COVID-19 infection in patients with tobacco smoke-associated cancers of the upper and lower airway. Eur Arch Otorhinolaryngol. 2021;278(8):2695-702. [PubMed ID: 33216184]. [PubMed Central ID: PMC7677601]. https://doi.org/10.1007/s00405-020-06456-z.
-
28.
Sharif N, Opu RR, Ahmed SN, Sarkar MK, Jaheen R, Daullah MU, et al. Prevalence and impact of comorbidities on disease prognosis among patients with COVID-19 in Bangladesh: A nationwide study amid the second wave. Diabetes Metab Syndr. 2021;15(4):102148. [PubMed ID: 34186349]. [PubMed Central ID: PMC8236060]. https://doi.org/10.1016/j.dsx.2021.05.021.
-
29.
Aziz M, Perisetti A, Lee-Smith WM, Gajendran M, Bansal P, Goyal H. Taste Changes (Dysgeusia) in COVID-19: A Systematic Review and Meta-analysis. Gastroenterology. 2020;159(3):1132-3. [PubMed ID: 32387496]. [PubMed Central ID: PMC7199692]. https://doi.org/10.1053/j.gastro.2020.05.003.
-
30.
Zayet S, Klopfenstein T, Mercier J, Kadiane-Oussou NJ, Lan Cheong Wah L, Royer PY, et al. Contribution of anosmia and dysgeusia for diagnostic of COVID-19 in outpatients. Infection. 2021;49(2):361-5. [PubMed ID: 32410112]. [PubMed Central ID: PMC7221233]. https://doi.org/10.1007/s15010-020-01442-3.
-
31.
Doyle ME, Appleton A, Liu QR, Yao Q, Mazucanti CH, Egan JM. Human Type II Taste Cells Express Angiotensin-Converting Enzyme 2 and Are Infected by Severe Acute Respiratory Syndrome Coronavirus 2 (SARS-CoV-2). Am J Pathol. 2021;191(9):1511-9. [PubMed ID: 34102107]. [PubMed Central ID: PMC8179718]. https://doi.org/10.1016/j.ajpath.2021.05.010.
-
32.
Mahmoud MM, Abuohashish HM, Khairy DA, Bugshan AS, Khan AM, Moothedath MM. Pathogenesis of dysgeusia in COVID-19 patients: a scoping review. Eur Rev Med Pharmacol Sci. 2021;25(2):1114-34. [PubMed ID: 33577069]. https://doi.org/10.26355/eurrev_202101_24683.
-
33.
Lozada-Nur F, Chainani-Wu N, Fortuna G, Sroussi H. Dysgeusia in COVID-19: Possible Mechanisms and Implications. Oral Surg Oral Med Oral Pathol Oral Radiol. 2020;130(3):344-6. [PubMed ID: 32703719]. [PubMed Central ID: PMC7320705]. https://doi.org/10.1016/j.oooo.2020.06.016.
-
34.
Vaishya R, Javaid M, Khan IH, Haleem A. Artificial Intelligence (AI) applications for COVID-19 pandemic. Diabetes Metab Syndr. 2020;14(4):337-9. [PubMed ID: 32305024]. [PubMed Central ID: PMC7195043]. https://doi.org/10.1016/j.dsx.2020.04.012.
-
35.
Kushwaha S, Bahl S, Bagha AK, Parmar KS, Javaid M, Haleem A, et al. Significant Applications of Machine Learning for COVID-19 Pandemic. J Ind Integration Manag. 2020;5(4):453-79. https://doi.org/10.1142/s2424862220500268.
-
36.
Muhammad LJ, Algehyne EA, Usman SS, Ahmad A, Chakraborty C, Mohammed IA. Supervised Machine Learning Models for Prediction of COVID-19 Infection using Epidemiology Dataset. SN Comput Sci. 2021;2(1):11. [PubMed ID: 33263111]. [PubMed Central ID: PMC7694891]. https://doi.org/10.1007/s42979-020-00394-7.
-
37.
Zoabi Y, Deri-Rozov S, Shomron N. Machine learning-based prediction of COVID-19 diagnosis based on symptoms. NPJ Digit Med. 2021;4(1):3. [PubMed ID: 33398013]. [PubMed Central ID: PMC7782717]. https://doi.org/10.1038/s41746-020-00372-6.
-
38.
Callejon-Leblic MA, Moreno-Luna R, Del Cuvillo A, Reyes-Tejero IM, Garcia-Villaran MA, Santos-Pena M, et al. Loss of Smell and Taste Can Accurately Predict COVID-19 Infection: A Machine-Learning Approach. J Clin Med. 2021;10(4). [PubMed ID: 33546319]. [PubMed Central ID: PMC7913595]. https://doi.org/10.3390/jcm10040570.