Abstract
Background:
Fasciolosis is a global parasitic disease caused by liver trematodes such as Fasciola hepatica, affecting various mammals, including sheep, goats, cattle, pigs, and humans worldwide. Cathepsin L (Cat L) found in the life stages of F. gigantica is associated with the pathology of the fluke. Given the detrimental impact of fasciolosis on animal health and production, along with reports of drug resistance and concerns regarding drug residues in animal products and the environment, there has been an increasing emphasis on developing a potent epitope-based vaccine to combat this infection.Objectives:
The present study aimed at bioinformatically analyzing the characteristics of Cat L of F. gigantica newly encysted juvenile (FgNEJ) to identify potential epitopes for constructing an effective vaccine against the infection.Methods:
The Cat L of FgNEJ was analyzed using several reliable bioinformatics tools.Results:
Analysis of the second structure of the Cat L amino acid sequence demonstrated that the alpha-helix, random coil, and extended strand ratios were 77 (23.62%), 164 (50.31%), and 85 (26.07%), respectively. Analysis of B-cell, T-cell, and MHC epitopes predicted on the sequence suggested Cat L of FgNEJ as an ideal target for vaccine design.Conclusions:
The current study introduces significant information on Cat L of FgNEJ, as well as some promising epitopes that can be used as strong candidates in studies involved in developing a vaccine against fasciolosis.Keywords
Cathepsin L Fasciola gigantica T-cell Epitopes B-cell Epitopes Bioinformatics
1. Background
Fasciolosis is an important helminthic disease caused by liver flukes, such as Fasciola gigantica, affecting a wide variety of domestic and wild animals, including cattle, sheep, goats, buffalo, deer, rabbit, and humans all around the world, especially in temperate and tropical climates. This host diversity contributes notably to the global spread of the infection (1). Fasciola gigantica is one of the most important parasitic helminths in Asia and Africa, affecting individual and small farming communities. It causes severe economic losses by decreasing animal production and livestock mortality. It is estimated that fasciolosis inflicts over US$3 billion in losses on the global animal industry (2). Fasciolosis has also been reported in humans in numerous countries. Iran is one of the countries where fasciolosis is a serious issue (3). Juvenile stages of Fasciola migrate through the circulatory system to the liver and mature into adults in the biliary ducts. The major cause of pathogenesis and clinical features of the infection is related to the migration of the parasite to the liver and the presence of adults in the biliary ducts (4).
Today, anti-parasitic drugs against fasciolosis are less effective due to the emergence of drug resistance. It has become a drastic problem in veterinary and medical sciences (5); thus, the attempts focus on designing a novel vaccine against the infection. Some candidates, including protease enzymes, have been proposed as the vaccine target (6). Proteases are secreted by different forms of parasites in order to facilitate parasitism and help the pathogens survive in their host for a long time (7). Cathepsin L (Cat L) enzyme, found in all stages of the liver flukes, plays a significant role in the biological functions of the parasites, such as migration, nutrition, and immune evasion (2). Vaccination with Cat L resulted in different protection rates against fasciolosis (8).
Bioinformatics has become a significant part of biology. Bioinformatics tools are irrefutable in comparing, analyzing, and interpreting genetic and genomic data and understanding evolutionary aspects of molecular biology and protein structure prediction. Increasing the knowledge about various aspects of the epitopes will result in the development and construction of novel epitope-based vaccines against pathogens.
2. Objectives
As far as we know, there is no published report regarding bioinformatics analysis of the Cat L sequences of FgNEJ. Therefore, the current study used bioinformatics methods to predict and identify B and T-cell epitopes and general features of Cat L of FgNEJ.
3. Methods
3.1. Post-Translation Modification and Topology Prediction
The sequence of Cat L of FgNEJ amino acid was extracted from Gene Bank, National Centre for Biotechnology Information (NCBI), through the server https://www.ncbi.nlm.nih.gov/nuccore/FJ617001.1/. The Molecular Weight (MW) and the isoelectric point (PI) of the protein sequence were calculated using the pI/Mw computing tool (http://www.expasy.ch/tools/pi_tool.html). Then, the SignalP 3.0 server (https://services.healthtech.dtu.dk/) was employed to signal peptide prediction. The WolfPSORT server (http://wolfpsort.seq.cbrc.jp/) was applied to predict the subcellular localization.
3.2. Secondary Structure Prediction
The secondary structure of the sequence was predicted using the GOR IV server, which is available at http://npsa-pbil.ibcp.fr/.
3.3. B-cell Epitope Prediction
Valid bioinformatics servers and software including BCPREDS (B-cell epitope prediction server) (http://ailab.ist.psu.edu/bcpred/predict.html), IEDB (Immune Epitope Database) (http://tools.iedb.org/bcell/), ABCpred (Artificial neural network based B-cell epitope prediction) (http://crdd.osdd.net/), and Bcepred (B- cell epitope prediction) were used to predict and analyze B-cell epitopes on the Cat L sequence. BCPREDS uses the combination of SSK (subsequence kernel) and SVM (support vector machine) methods to provide a prediction of continuous B-cell epitopes (9). The software obtains a single amino acid sequence in a plain format as input and gives each epitope a single score. Epitopes with high scores are listed in a table. The following items were set up as default to run the program: Epitope length: 20 amino acids, Classifier specificity: 75%, and the use of an overlap filter. Also, the continuous antibody epitopes of the sequence were predicted using the IEDB server. This server applies the following parameters to predict B-cell epitopes: flexibility (10), hydrophilicity (11), antigenicity (12), beta-turn (13), and surface accessibility (14). IEDB presents the results as graphs and tables. On the graphs, the Y-axes depict the correspondent score for each residue (averaged in the specified window), while the X-axes depict the residue positions in the sequence. The tables provide values of calculated scores for each residue. ABCpred is a bioinformatics program in which Artificial Neural Network (ANN), with a 65.93% accuracy, is used to predict B-cell epitope(s). It predicts B-cell epitopes based on scores obtained from a trained recurrent neural network. The output data is displayed in graphical and tabular forms. The epitopes are shown in blue in the graphic form. Furthermore, peptides with a high score are listed in a table (15). The default parameters derived from this program were used in the present study. Bcepred applies physicochemical properties, with 58.7% accuracy, including flexibility, turn, accessibility, antigenicity, and hydrophilicity, to predict epitopes (16).
3.4. Prediction of MHC-I and MHC-II Binding Epitopes
For the prediction of peptides binding to Major Histocompatibility Complex (MHC) class 1 and class 2 molecules, NetMHCcons 1.1 (17) (http://www.cbs.dtu.dk/services/NetMHCcons/) and NetMHCIIpan 3.2 (18) (http://www.cbs.dtu.dk/) online servers were applied, respectively. In NetMHCcons, the user can select the MHC molecule, along with a long list of alleles, and upload protein sequences. NetMHCIIpan is applied to predict human MHC class II isotypes, including HLA-DR, HLA-DP, HLA-DQ, and mouse molecules (H-2) (19). These servers provide the value as the half maximal inhibitory concentration (IC50) in nano Molars and %Rank. In NetMHCcons, a peptide with a rank value under 0.5% or a binding affinity (IC50) under 50 nM is predicted as a strong binder. Moreover, the peptide with a rank value under 2% or a binding affinity (IC50) under 500 nM is predicted as a weak binder. In NetMHCIIpan, peptides are predicted as strong and weak binders if the rank value is below 2% and 10%, respectively. According to previous studies (20), five frequently occurring alleles, including HLA- A01:01, HLA-A02:01, HLA-A26:01, HLA-B27:05, and HLA-B39:01 as human MHC class I molecules and 5-allele HLA reference set including DRB1_0301, DRB1_0701, DRB3_0101, DRB5_0101, and HLA-DPA10103-DPB110201 as human MHC class II molecules were chosen. By default, the length of predicted peptides was 10 amino acids in NetMHCcons and 15 amino acids in NetMHCIIpan methods.
3.5. Cytotoxic T lymphocyte (CTL) Epitopes Prediction
The CTLpred online server (21) (http://www.imtech.res.in/raghava/ctlpred/index.html) was employed to predict CTL epitopes. It directly predicts CTL epitopes and uses the information of T-cell epitopes rather than MHC binders. The basis of the server is on elegant machine learning techniques: Artificial Neural Network (ANN) and Support Vector Machine (SVM). The current study used the combined method (ANN+SVM), with 76% accuracy, to predict CTL epitopes on the protein sequence. The ANN (0.51) and SVM (0.36) cutoff scores were set by default. It is used to distinguish the epitopes and non-epitopes.
4. Results
4.1. Post-translation Modification and Topology Prediction
The complete amino acid sequence of Cat L submitted to the SignalP 3.0 server revealed a signal peptide composed of 15 amino acids, with a cleavage site between 15 and 16: VFA-SN (Figure 1). The WolfPSORT subcellular localization analysis classified Cat L of FgNEJ into 32 different families of proteins belonging to extracellular 24, lysozyme 4, integral membrane protein 1, mitochondrial inner membrane 1, endoplasmic reticulum membrane 1, cytoplasmic 1, and peroxisomal 1. The MW of Cat L was predicted to be 37 Kd. Also, the aliphatic index of the protein sequence was calculated to be 61.82.
Protein signal peptide prediction of Cat L of FgNEJ using the SignalP 3.0 server according to SignalP Neural Networks (SignalPNN). Sp (Sec/SPI) corresponds to amino acids with a high probability of composing the signal peptide. The cleavage site (CS) was found between 15 and 16.
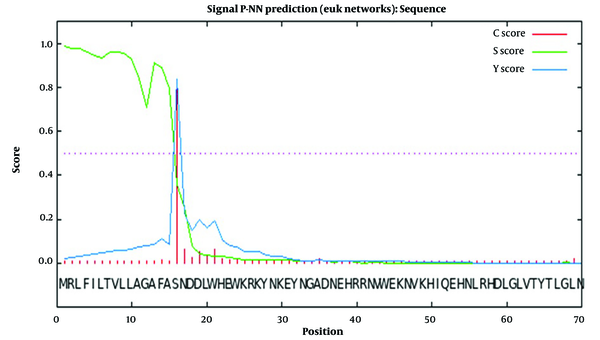
4.2. Secondary Structure Prediction
The secondary structure analysis was performed using the GOR secondary structure prediction online server. The results showed that the random coil, alpha-helix, and extended strand ratios on the Cat L sequence were 164 (50.31%), 77 (23.62%), and 85 (26.07%), respectively (Figure 2).
Secondary structure analysis of Cat L of FgNEJ using the GOR IV secondary structure prediction method (h, helix; e, extended strand; c, coil)
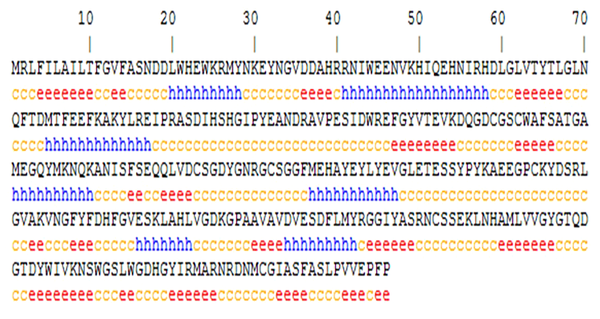
4.3. B-cell Epitopes Prediction
The BCPREDS server was applied to predict Linear B-cell epitopes on the sequence. Further, a score over the threshold was considered the B-cell epitope. Table 1 presents 5 epitopes with the highest score.
Linear B-cell Epitopes Predicted on Cat L of FgNEG Using BCPREDS
Position | Epitope | Score |
---|---|---|
188 | GLETESSYPYKAEEGPCKYD | 0.97 |
159 | QLVDCSGDYGNRGCSGGFME | 0.965 |
272 | LVVGYGTQDGTDYWIVKNSW | 0.954 |
90 | RASDIHSHGIPYEANDRAVP | 0.866 |
117 | FGYVTEVKDQGDCGSCWAFS | 0.842 |
Furthermore, the results of the IEDB prediction are drawn in Figure 3. The least, average, and highest threshold scores for the used parameters were as follows: beta-turn (0.567, 1.010, 1.414), surface accessibility (0.064, 1.00, 4.847), flexibility (0.890, 0.988, 1.092), antigenicity (0.865, 1.016, 1.178), and hydrophilicity (-7.243, 1.697, 6.829). Subsequently, the ABCpred server was applied to predict the epitopes of Cat L of FgNEJ. It ranked the predicted epitopes based on their scores. A higher score for any epitope indicates an increased probability as an epitope. This server predicted 32 epitopes on the sequence. The highest score (0.96) was for linear epitope HGYIRMARNRDNMCGI. Ten epitopes with higher scores were noted in Table 2. Also, the Bcepred server predicted 5 chemo-physical parameters of the epitopes (Table 3). These results demonstrated potential B-cell epitopes on Cat L of the FgNEJ sequence, which are key targets for further diagnostic and vaccine studies.
The output of the IEDB server is based on parameters including (A) Hydrophilicity, (B) Antigenicity, (C) Flexibility, (D) Surface accessibility, and (E) Beta turn. The residue with a higher score represents that the residue might have a higher probability of being part of the epitope (those residues are colored in yellow on the graphs)
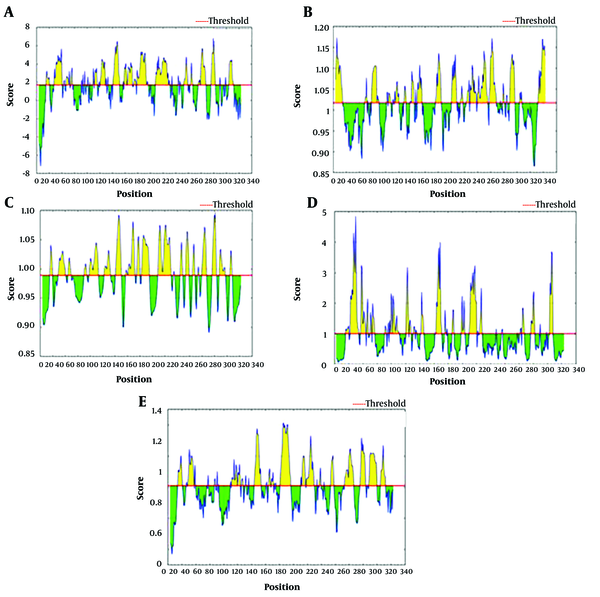
B-cell Epitopes Predicted on Cat L of FgNEJ Using ABCpred
Rank | Sequence | Start Position | Score |
---|---|---|---|
1 | HGYIRMARNRDNMCGI | 298 | 0.96 |
2 | YGTQDGTDYWIVKNSW | 276 | 0.94 |
3 | GSLWGDHGYIRMARNR | 292 | 0.92 |
4 | YKAEEGPCKYDSRLGV | 197 | 0.91 |
5 | DWREFGYVTEVKDQGD | 113 | 0.91 |
6 | GLETESSYPYKAEEGP | 188 | 0.90 |
7 | QQLVDCSGDYGNRGCS | 158 | 0.90 |
8 | KHIQEHNIRHDLGLVT | 49 | 0.89 |
9 | RGGIYASRNCSSEKLN | 253 | 0.89 |
10 | HEWKRMYNKEYNGVDD | 22 | 0.89 |
B-cell Epitopes Predicted on Cat L of FgNEJ Using Bcepred
Parameter | Epitope Sequence |
---|---|
Hydrophilicity | NGVDDAH, DDAHRRN, PYEANDR, YEANDRA, EVKDQGD, VKDQGDC, KDQGDCG, DQGDCGS, QGDCGSC, DCSGDYG, SGDYGNR, EYNGVDD |
Antigenicity | RLFILAI, LFILAIL, FILAILT, ILAILTF, IRHDLGL, RHDLGLV, HDLGLVT, DLGLVTY, LGLVTYT, RAVPESI, FGYVTEV, FASLPVV, ASLPVVE, SLPVVEP, LPVVEPF |
Flexibility | NGVDDAH, REIPRAS, EIPRASD, IPRASDI, AVPESID, VTEVKDQ, TEVKDQG, EVKDQGD, VKDQGDC, KDQGDCG, DQGDCGS, QGDCGSC, YKAEEGP |
Accessibility | YNKEYN, NKEYNG, KRMYNK, RMYNKE, DDAHRR, YEANDR, QYMKNQ, YMKNQK, ARNRDN, RNRDNM, |
Turn | GVFASND, ASNDDLW, SNDDLWH, YNKEYNG, NKEYNGV, KEYNGVD, EYNGVDD, YNGVDDA, HSHGIPY, SHGIPYET KDQGDCG, GDCGSCW, GNRGCSG, GDYGNRG |
4.4. Prediction of MHC-I and MHC-II Binding Epitopes
Data from NetMHCcons and NetMHCIIpan servers are presented in Tables 4, and 5, respectively. For each MHC, 5 alleles were selected. The data used by the servers included a table containing the position, used allele, predicted peptide, Affinity/IC50 (nM), %Rank, and binding level (strong or weak). Five peptides with high affinity to MHC molecules were selected for each allele. The results revealed several epitopes strongly coupled to MHC I and MHC II molecules in Cat L. Strongest binders belonged to HLA-A01:01 (MCH I) and DRB1_0701 (MHC II).
Prediction of MHC-I Binding Epitopes on Cat L of FgNEJ Using NetMHCcons
Allele and Position | Peptide | Affinity | Binding Level |
---|---|---|---|
HLA-A01:01 | |||
71 | FTDMTFEEFK | 396.76 | SB |
74 | MTFEEFKAKY | 454.22 | SB |
109 | ESIDWREFGY | 1097.07 | SB |
110 | SIDWREFGYV | 5500.22 | SB |
175 | FMEHAYEYLY | 55.68 | SB |
HLA-A02:01 | |||
3 | FILAILTFGV | 4.57 | SB |
25 | RMYNKEYNGV | 25.41 | SB |
248 | FLMYRGGIYA | 14.17 | SB |
311 | GIASFASLPV | 39.38 | SB |
277 | TQDGTDYWIV | 289.91 | WB |
HLA-A26:01 | |||
74 | MTFEEFKAKY | 47.34 | SB |
109 | ESIDWREFGY | 44.84 | SB |
135 | SATGAMEGQY | 1874.27 | WB |
185 | EVGLETESSY | 534.26 | WB |
HLA-B27:05 | |||
38 | HRRNIWEENV | 784.45 | WB |
39 | RRNIWEENVK | 138.16 | SB |
55 | IRHDLGLVTY | 223.61 | WB |
300 | IRMARNRDNM | 168.78 | WB |
303 | ARNRDNMCGI | 1311.49 | WB |
HLA-B39:01 | |||
57 | HDLGLVTYTL | 309.35 | WB |
113 | WREFGYVTEV | 405.44 | WB |
262 | EHAYEYLYEV | 229.74 | WB |
264 | TQDGTDYWIV | 149.03 | WB |
Prediction of MHC-II Binding Epitopes on Cat L of FgNEJ Using NetMHCIIpan
Allele and Position | Peptide | Affinity | Binding Level |
---|---|---|---|
DRB1_0301 | |||
50 | HIQEHNIRHDLGLVT | 223.82 | WB |
51 | IQEHNIRHDLGLVTY | 107.03 | SB |
52 | QEHNIRHDLGLVTYT | 89.42 | SB |
53 | EHNIRHDLGLVTYTL | 79.73 | SB |
54 | HNIRHDLGLVTYTLG | 87.56 | SB |
DRB1_0701 | |||
310 | MCGIASFASLPVVEP | 38.94 | SB |
309 | NMCGIASFASLPVVE | 35.04 | SB |
307 | RDNMCGIASFASLPV | 47.03 | WB |
308 | DNMCGIASFASLPVV | 33.80 | SB |
311 | CGIASFASLPVVEPF | 44.05 | WB |
DRB3_0101 | |||
50 | HIQEHNIRHDLGLV | 221.36 | WB |
51 | IQEHNIRHDLGLVTY | 125.50 | WB |
52 | QEHNIRHDLGLVTYT | 115.17 | WB |
53 | EHNIRHDLGLVTYTL | 101.56 | WB |
54 | HNIRHDLGLVTYTLG | 121.59 | WB |
DRB5_0101 | |||
296 | GDHGYIRMARNRDNM | 41.12 | WB |
297 | DHGYIRMARNRDNMC | 42.07 | WB |
298 | HGYIRMARNRDNMCG | 39.00 | WB |
299 | GYIRMARNRDNMCGI | 48.01 | WB |
300 | YIRMARNRDNMCGIA | 112.03 | WB |
HLA-DPA10103-DPB110201 | |||
71 | QFTDMTFEEFKAKYL | 115.56 | SB |
72 | FTDMTFEEFKAKYLR | 140.86 | WB |
73 | TDMTFEEFKAKYLRE | 203.14 | WB |
74 | DMTFEEFKAKYLREI | 166.61 | WB |
75 | MTFEEFKAKYLREIP | 172.08 | WB |
4.5. Prediction of CTL Epitopes
Prediction of cytotoxic T lymphocytes from the CTL server was performed based on their scores. Finally, the top 10 epitopes with the highest score were obtained by CTLpred, as presented in Table 6.
Predicted CTL Epitopes of Cat L of FgNEJ Using CTLpred
Peptide Rank | Start Position | Sequence | Score (ANN/SVM) |
---|---|---|---|
1 | 1 | MRLFILAIL | 1 |
2 | 209 | RLGVAKVNG | 1 |
3 | 278 | TQDGTDYWI | 1 |
4 | 16 | SNDDLWHEW | 0.990 |
5 | 89 | PRASDIHSH | 0.990 |
6 | 176 | FMEHAYEYL | 0.990 |
7 | 187 | VGLETESSY | 0.990 |
8 | 63 | VTYTLGLNQ | 0.980 |
9 | 105 | DRAVPESID | 0.980 |
10 | 231 | HLVGDKGPA | 0.980 |
5. Discussion
Parasitic infections are a major threat to humans and animals, leading to serious diseases (22, 23). The control strategy for Fasciola infection is currently based on using antihelmintic drugs. However, due to the long-term use of drugs, drug resistance has been reported in many countries, including Australia, Ireland, and Argentina (24). Vaccination seems to be the best way to control fasciolosis infection (25) Vaccines are more sustainable, cost-effective, and environmentally friendly tools that significantly boost immunity against pathogens. They mostly consist of killed or attenuated pathogens, which may sometimes be dangerous for the host due to problems in its preparation process. Thus, it is safer to apply an epitope-based vaccine, inducing and stimulating an immune response against the specific pathogen (26). Different antigens have been described as vaccine targets against fasciolosis. It has been reported that cysteine proteinases are promising vaccine antigens as they play a vital role in host-parasite interaction. Cathepsins are from the family of cysteine proteases expressed in Fasciola species. Several isoforms of Cat L and B are expressed in different stages of Fasciola parasites (27).
One of the most valuable ways to design and deliver an epitope-based vaccine is by analyzing the peptide signal and antigen properties using bioinformatics tools (20, 28). Introductory bioinformatics analysis of potential vaccine candidates causes a significant reduction in the number of used animals in vaccine trials and provides important and essential data about the protein of interest in an easy and simple way (29). In order to construct an ideal vaccine with perfect protection against pathogens, it is crucial to understand the entire structure of their antigens (30). Therefore, computational analysis can be pivotal in the vaccine design process. The present study employed various bioinformatics tools to design the vaccine to learn more about the Cat L of FgNEJ. The signal peptide is the most common characteristic researchers analyze when their target is to find secreted or membrane-bound proteins in the amino acid sequence (31). In the present study, the Cat L analysis revealed an N-terminal signal peptide using the bioinformatics software.
The prediction of protein structures improves our knowledge regarding the function and biological aspects of the protein. The secondary structure plays a significant role in epitope function (32). In the current study, the maximum parts of the secondary structure belonged to the random coil, which is introduced on the protein surface and may be a potential epitope (33). Additionally, alpha-helix and beta-turn are commonly found in the internal part of the protein, and they retain proteins by high chemical-bond energy. It does not seem to work as an epitope.
The epitope is part of the antigen, detected by B-cell and T-cell molecules of the mammalian immune system. Only a few amino acids of a protein (instead of the total protein) can provide enough protective response. Therefore, predicting or identifying this part of the amino acid can be vital to understanding the mechanisms of immunity and pathogenesis of pathogens (32). Most importantly, these epitopes can be used to design epitope-based vaccines (34). Prediction of B-cell and T-cell epitopes is a key step for vaccine design, development of diagnostic reagents, and understanding the antigen-antibody interactions on a molecular level (20). Predicting linear B-cell epitopes in Cat L of FgNEJ was performed using reliable bioinformatics software to find potential targets for vaccines against Fasciola infection.
The current study applied bioinformatics tools such as BCPREDS, ABCpred, Bcepred, and IEDB to predict B-cell epitopes on Cat L of FgNEJ. The results of BCPREDS identified 5 potential antigenic epitopes on the sequence. Also, based on the data from ABCpred software, of 32 epitopes predicted on Cat L, 10 high-score epitopes can be used as targets in vaccine research. Additionally, we applied Bcepred to predict linear B-cell epitopes. The software used physical and chemical properties such as hydrophilicity, accessibility, flexibility/mobility, exposed surface, and turns in order to predict B-cell epitopes. Each of these properties predicted several B-cell epitopes on Cat L. Some protein parameters, such as flexibility, hydrophilicity, turns, accessibility, polarity, exposed surface, and antigenic propensity, have been associated with the localization of continuous epitopes. In the present study, the data obtained from IEDB disclosed some potential epitopes on Cat L of FgNEJ. The results from these tools indicated potential epitopes on this protein that can be strong candidates for designing an effective vaccine against fasciolosis (after testing in animal models). In a study performed on antibody recognition of cathepsin L, the characteristics of known linear B-cell epitopes on F. hepatica Cat L protein using the sera of F. hepatica infected or vaccinated cattle in two independent experiments were identified, and these results were confirmed by other studies (35-37). The study also demonstrated that vaccinated animals with Cat L showed fluke burden reduction with eliciting antibodies (8). Another study on the Plasmodium vivax AMA-1 protein found similar results using the same bioinformatics tools, reported several promising epitopes on AMA-1, and suggested that these bioinformatics tools were valid to predict and identify epitopes on proteins (20).
The MHC molecules represent T-cell epitopes to T-cells. Peptide binding to MHC is a major factor in the selection of potential epitopes and is an essential step in presenting the antigen to T-cells. Prediction of peptide-MHC binding epitopes could be vital for constructing a reliable epitope-based vaccine against infections (38). The significant information to understand the process of infection pathogenesis was provided by the prediction and characterization of both CD8 and CD4 T-cell epitopes on a protein (39). Another major step in vaccine development is the identification of CTL-stimulating peptides. In this study, 10 epitopes were predicted using CTLpred software. Responses derived from cytotoxic T-cells are induced during a pathway composed of intracellular antigens refining upon even epitopes as common targets.
5.1. Conclusions
The vaccine design against infectious Fasciola is still a major necessity. In fact, no commercial vaccine is available to control human and animal fasciolosis. High-quality vaccines can be designed with accurate analysis of antigens by valid bioinformatics tools. This requires the identification of potential antigens with robust protective responses. Computational analysis provides substantial information to recognize and represent proteins with immunogenic properties, which facilitates finding promising epitopes for vaccine design. In the present study, various properties, structures, and B and T-cell epitopes of Cat L of FgNEJ were predicted using valid bioinformatics tools, suggesting potential epitopes on Cat L to design an efficient vaccine against fasciolosis. Moreover, the data from the current study can be used as basic and useful information for further in vivo studies.
Acknowledgements
References
-
1.
Mehmood K, Zhang H, Sabir AJ, Abbas RZ, Ijaz M, Durrani AZ, et al. A review on epidemiology, global prevalence and economical losses of fasciolosis in ruminants. Microb Pathog. 2017;109:253-62. [PubMed ID: 28602837]. https://doi.org/10.1016/j.micpath.2017.06.006.
-
2.
Morphew RM, Wright HA, Lacourse EJ, Porter J, Barrett J, Woods DJ, et al. Towards delineating functions within the fasciola secreted cathepsin l protease family by integrating in vivo based sub-proteomics and phylogenetics. PLoS Negl Trop Dis. 2011;5(1). e937. [PubMed ID: 21245911]. [PubMed Central ID: PMC3014944]. https://doi.org/10.1371/journal.pntd.0000937.
-
3.
Ashrafi K. The Status of Human and Animal Fascioliasis in Iran: A Narrative Review Article. Iran J Parasitol. 2015;10(3):306-28. [PubMed ID: 26622287]. [PubMed Central ID: PMC4662732].
-
4.
Toet H, Piedrafita DM, Spithill TW. Liver fluke vaccines in ruminants: strategies, progress and future opportunities. Int J Parasitol. 2014;44(12):915-27. [PubMed ID: 25200351]. https://doi.org/10.1016/j.ijpara.2014.07.011.
-
5.
Venturina VM, Alejandro MA, Baltazar CP, Abes NS, Mingala CN. Evidence of Fasciola spp. resistance to albendazole, triclabendazole and bromofenofos in water buffaloes (Bubalus bubalis). Ann Parasitol. 2015;61(4):283-9. [PubMed ID: 26878627]. https://doi.org/10.17420/ap6104.20.
-
6.
Changklungmoa N, Kueakhai P, Riengrojpitak S, Chaithirayanon K, Chaichanasak P, Preyavichyapugdee N, et al. Immunization with recombinant leucine aminopeptidase showed protection against Fasciola gigantica in mice. Parasitol Res. 2013;112(10):3653-9. [PubMed ID: 23917327]. https://doi.org/10.1007/s00436-013-3553-6.
-
7.
Morphew RM, Wright HA, LaCourse EJ, Woods DJ, Brophy PM. Comparative proteomics of excretory-secretory proteins released by the liver fluke Fasciola hepatica in sheep host bile and during in vitro culture ex host. Mol Cell Proteomics. 2007;6(6):963-72. [PubMed ID: 17308300]. https://doi.org/10.1074/mcp.M600375-MCP200.
-
8.
Garza-Cuartero L, Geurden T, Mahan SM, Hardham JM, Dalton JP, Mulcahy G. Antibody recognition of cathepsin L1-derived peptides in Fasciola hepatica-infected and/or vaccinated cattle and identification of protective linear B-cell epitopes. Vaccine. 2018;36(7):958-68. [PubMed ID: 29373193]. https://doi.org/10.1016/j.vaccine.2018.01.020.
-
9.
El-Manzalawy Y, Dobbs D, Honavar V. Predicting linear B-cell epitopes using string kernels. J Mol Recognit. 2008;21(4):243-55. [PubMed ID: 18496882]. [PubMed Central ID: PMC2683948]. https://doi.org/10.1002/jmr.893.
-
10.
Karplus PA, Schulz GE. Prediction of chain flexibility in proteins. Naturwissenschaften. 1985;72(4):212-3. https://doi.org/10.1007/bf01195768.
-
11.
Parker JM, Guo D, Hodges RS. New hydrophilicity scale derived from high-performance liquid chromatography peptide retention data: correlation of predicted surface residues with antigenicity and X-ray-derived accessible sites. Biochemistry. 1986;25(19):5425-32. [PubMed ID: 2430611]. https://doi.org/10.1021/bi00367a013.
-
12.
Kolaskar AS, Tongaonkar PC. A semi-empirical method for prediction of antigenic determinants on protein antigens. FEBS Lett. 1990;276(1-2):172-4. [PubMed ID: 1702393]. https://doi.org/10.1016/0014-5793(90)80535-q.
-
13.
Chou PY, Fasman GD. Prediction of the secondary structure of proteins from their amino acid sequence. Adv Enzymol Relat Areas Mol Biol. 1978;47:45-148. [PubMed ID: 364941]. https://doi.org/10.1002/9780470122921.ch2.
-
14.
Emini EA, Hughes JV, Perlow DS, Boger J. Induction of hepatitis A virus-neutralizing antibody by a virus-specific synthetic peptide. J Virol. 1985;55(3):836-9. [PubMed ID: 2991600]. [PubMed Central ID: PMC255070]. https://doi.org/10.1128/JVI.55.3.836-839.1985.
-
15.
Saha S, Raghava GP. Prediction of continuous B-cell epitopes in an antigen using recurrent neural network. Proteins. 2006;65(1):40-8. [PubMed ID: 16894596]. https://doi.org/10.1002/prot.21078.
-
16.
Saha S, Raghava GPS. BcePred: Prediction of Continuous B-Cell Epitopes in Antigenic Sequences Using Physico-chemical Properties. International Conference on Artificial Immune Systems. Springer; 2004. p. 197-204.
-
17.
Karosiene E, Lundegaard C, Lund O, Nielsen M. NetMHCcons: a consensus method for the major histocompatibility complex class I predictions. Immunogenetics. 2012;64(3):177-86. [PubMed ID: 22009319]. https://doi.org/10.1007/s00251-011-0579-8.
-
18.
Jensen KK, Andreatta M, Marcatili P, Buus S, Greenbaum JA, Yan Z, et al. Improved methods for predicting peptide binding affinity to MHC class II molecules. Immunology. 2018;154(3):394-406. [PubMed ID: 29315598]. [PubMed Central ID: PMC6002223]. https://doi.org/10.1111/imm.12889.
-
19.
Andreatta M, Nielsen M. Bioinformatics Tools for the Prediction of T-Cell Epitopes. Methods Mol Biol. 2018;1785:269-81. [PubMed ID: 29714025]. https://doi.org/10.1007/978-1-4939-7841-0_18.
-
20.
Jahangiri F, Jalallou N, Ebrahimi M. Analysis of Apical Membrane Antigen (AMA)-1 characteristics using bioinformatics tools in order to vaccine design against Plasmodium vivax. Infect Genet Evol. 2019;71:224-31. [PubMed ID: 30953716]. https://doi.org/10.1016/j.meegid.2019.04.001.
-
21.
Bhasin M, Raghava GP. Prediction of CTL epitopes using QM, SVM and ANN techniques. Vaccine. 2004;22(23-24):3195-204. [PubMed ID: 15297074]. https://doi.org/10.1016/j.vaccine.2004.02.005.
-
22.
Shaddel M, Sharifi I, Karvar M, Keyhani A, Baziar Z. Cryotherapy of cutaneous leishmaniasis caused by Leishmania major in BALB/c mice: A comparative experimental study. J Vector Borne Dis. 2018;55(1):42-6. [PubMed ID: 29916447]. https://doi.org/10.4103/0972-9062.234625.
-
23.
Zareian P, Mirzaii Dizgah I, Shaddel M, Khodabandehloo F. Effects of Toxoplasma gondii infection on anxiety, depression and ghrelin level in male rats. J Parasit Dis. 2016;40(3):688-93. [PubMed ID: 27605768]. [PubMed Central ID: PMC4996174]. https://doi.org/10.1007/s12639-014-0561-0.
-
24.
Olaechea F, Lovera V, Larroza M, Raffo F, Cabrera R. Resistance of Fasciola hepatica against triclabendazole in cattle in Patagonia (Argentina). Vet Parasitol. 2011;178(3-4):364-6. [PubMed ID: 21277090]. https://doi.org/10.1016/j.vetpar.2010.12.047.
-
25.
Jaros SŁAWOMIR, Zygner W, Januszkiewicz K, Jaros D, Wędrychowicz HALINA. Molecular cloning and bioinformatic characterisation of FhPcW1, a new isoform of cathepsin L from adult Fasciola hepatica. Bull Vet Inst Pulawy. 2010;54(4):585-90.
-
26.
Arinaminpathy N, Ratmann O, Koelle K, Epstein SL, Price GE, Viboud C, et al. Impact of cross-protective vaccines on epidemiological and evolutionary dynamics of influenza. Proc Natl Acad Sci U S A. 2012;109(8):3173-7. [PubMed ID: 22323589]. [PubMed Central ID: PMC3286944]. https://doi.org/10.1073/pnas.1113342109.
-
27.
Cwiklinski K, Dalton JP, Dufresne PJ, La Course J, Williams DJ, Hodgkinson J, et al. The Fasciola hepatica genome: gene duplication and polymorphism reveals adaptation to the host environment and the capacity for rapid evolution. Genome Biol. 2015;16(1):71. [PubMed ID: 25887684]. [PubMed Central ID: PMC4404566]. https://doi.org/10.1186/s13059-015-0632-2.
-
28.
Foroutan M, Ghaffarifar F, Sharifi Z, Dalimi A, Pirestani M. Bioinformatics analysis of ROP8 protein to improve vaccine design against Toxoplasma gondii. Infect Genet Evol. 2018;62:193-204. [PubMed ID: 29705360]. https://doi.org/10.1016/j.meegid.2018.04.033.
-
29.
Meemon K, Sobhon P. Juvenile-specific cathepsin proteases in Fasciola spp.: their characteristics and vaccine efficacies. Parasitol Res. 2015;114(8):2807-13. [PubMed ID: 26099239]. https://doi.org/10.1007/s00436-015-4589-6.
-
30.
Purcell AW, Zeng W, Mifsud NA, Ely LK, Macdonald WA, Jackson DC. Dissecting the role of peptides in the immune response: theory, practice and the application to vaccine design. J Pept Sci. 2003;9(5):255-81. [PubMed ID: 12803494]. https://doi.org/10.1002/psc.456.
-
31.
Pearson MS, McManus DP, Smyth DJ, Lewis FA, Loukas A. In vitro and in silico analysis of signal peptides from the human blood fluke, Schistosoma mansoni. FEMS Immunol Med Microbiol. 2005;45(2):201-11. [PubMed ID: 16051070]. https://doi.org/10.1016/j.femsim.2005.03.009.
-
32.
Shaddel M, Ebrahimi M, Tabandeh MR. Bioinformatics analysis of single and multi-hybrid epitopes of GRA-1, GRA-4, GRA-6 and GRA-7 proteins to improve DNA vaccine design against Toxoplasma gondii. J Parasit Dis. 2018;42(2):269-76. [PubMed ID: 29844632]. [PubMed Central ID: PMC5962505]. https://doi.org/10.1007/s12639-018-0996-9.
-
33.
Zhang NZ, Huang SY, Zhou DH, Xu Y, He JJ, Zhu XQ. Identification and bioinformatic analysis of a putative calcium-dependent protein kinase (CDPK6) from Toxoplasma gondii. Genet Mol Res. 2014;13(4):10669-77. [PubMed ID: 25526188]. https://doi.org/10.4238/2014.December.18.9.
-
34.
Desai DV, Kulkarni-Kale U. T-cell epitope prediction methods: an overview. Methods Mol Biol. 2014;1184:333-64. [PubMed ID: 25048134]. https://doi.org/10.1007/978-1-4939-1115-8_19.
-
35.
Fereig RM, Metwally S, El-Alfy ES, Abdelbaky HH, Shanab O, Omar MA, et al. High relatedness of bioinformatic data and realistic experimental works on the potentials of Fasciola hepatica and F. gigantica cathepsin L1 as a diagnostic and vaccine antigen. Front Public Health. 2022;10:1054502. [PubMed ID: 36568750]. [PubMed Central ID: PMC9768368]. https://doi.org/10.3389/fpubh.2022.1054502.
-
36.
Xu J, Wu L, Sun Y, Wei Y, Zheng L, Zhang J, et al. Proteomics and bioinformatics analysis of Fasciola hepatica somatic proteome in different growth phases. Parasitol Res. 2020;119(9):2837-50. [PubMed ID: 32757109]. [PubMed Central ID: PMC7403185]. https://doi.org/10.1007/s00436-020-06833-x.
-
37.
Buffoni L, Garza-Cuartero L, Perez-Caballero R, Zafra R, Javier Martinez-Moreno F, Molina-Hernandez V, et al. Identification of protective peptides of Fasciola hepatica-derived cathepsin L1 (FhCL1) in vaccinated sheep by a linear B-cell epitope mapping approach. Parasit Vectors. 2020;13(1):390. [PubMed ID: 32736582]. [PubMed Central ID: PMC7393625]. https://doi.org/10.1186/s13071-020-04260-6.
-
38.
De Groot AS. Immunomics: discovering new targets for vaccines and therapeutics. Drug Discov Today. 2006;11(5-6):203-9. [PubMed ID: 16580597]. https://doi.org/10.1016/S1359-6446(05)03720-7.
-
39.
Tchernev G, Orfanos CE. Antigen mimicry, epitope spreading and the pathogenesis of pemphigus. Tissue Antigens. 2006;68(4):280-6. [PubMed ID: 17026461]. https://doi.org/10.1111/j.1399-0039.2006.00677.x.