Abstract
Background:
The precise identification of attention deficit-hyperactivity disorder (ADHD) is one of the challenging clinical processes. Disorganizations in functional neural networks revealed via functional magnetic resonance imaging have recently been contributing. Machine learning approaches, particularly classification methods, have commonly been employed as a framework for diverse data analysis, indicating promising medical diagnosis results. However, as the neuroimaging data are high-dimensional with a low sample size (the current dataset), this study aimed to evaluate the classification performance of the models by considering the specific contribution of the sparsity of data matrices.Methods:
This cross-sectional study analyzed the preprocessed data from the 2011 ADHD-200 Global Competition. A total of 768 and 171 data items were considered training and test, respectively. The diagnosis status was used as a response variable. Age, gender, hand dominance, and activity relationship between 116 brain regions derived from inverse covariance matrix and inverse sparse covariance matrix were used as predictive variables. Accordingly, this study compared the performance of three models, namely support vector machine (SVM), distance-weighted discrimination (DWD), and data maximum dispersion classifier (DMDC) for ADHD categorization.Results:
The highest value for the total accuracy was reported for the SVM model on the sparse covariance matrix. Moreover, the highest values for the balanced classification rate (BCR) (59%) and sensitivity (64%) were reported for DMDC on the sparse covariance matrix. The best level of specificity (99%) was obtained from DWD using the sparse covariance matrix. The highest levels of the values (i.e., total accuracy and BCR) were achieved through the model fitting on the sparse matrices. Among the six models, the DMDC model on sparse covariance matrix was the most optimal algorithm due to the superiority of the two indices (i.e., accuracy: 60% and BCR: 60%) and the favorable balance between sensitivity and specificity values.Conclusions:
Among the current studied three models, DMDC performance, applying the sparse data, remarkably improved the results of classification processes. Based on the present findings, the neuronal connectivity among subcortical structures comprising parts of the basal ganglia and cerebellum provides a distinction between ADHD subjects and healthy controls.Keywords
Classification DMDC DWD Functional Connectivity SVM HDLSS ADHD
1. Background
Attention deficit-hyperactivity disorder (ADHD) is a neurodevelopmental pathology that has phenomenally been recognized as a set of neuropsychiatric impairments, and its worldwide prevalence ranges from 5% to 10% in school-age children (1). Adults can also experience ADHD; however, the pattern is not as clear as in the childhood period (2). The clinical representation comprises a spectrum of symptoms from inattention, hyperactivity, and impulsivity to the signs of comorbid depressive and generalized anxiety disorders. ADHD is accompanied by substantial chronic difficulties, with yearly costs of approximately $36 billion/year in the United States. The diagnostic and statistical manual of mental disorders-fifth edition mainly categorizes ADHD as predominantly inattentive presentation, predominantly hyperactive/impulsive presentation, and combined condition within the intensity range from mild to severe (3).
The specific role of structural and functional disorganizations in neuronal systems has recently made a significant contribution to the explanation of clinical manifestations (4, 5). Despite the widespread and significant signs of progress in brain-mapping techniques, ADHD still encompasses obscure aspects within its etiological background, which subsequently could emerge as the numerous insufficiencies in diagnostic procedures and either pharmacotherapeutics or non-pharmacotherapeutics practical courses (6, 7). Functional magnetic resonance imaging (fMRI) is developed to facilitate addressing the characteristics of the central nervous system’s inaccessible functions with the potential of providing substantial assistance to determine the trajectory of diagnosis and mechanism of interventions through objectively monitoring functional changes across the neuronal regions that are hypothetically targeted by the etiological origins (8, 9).
Resting-state fMRI (rs-fMRI) is a relatively new and robust method for examining the activation and interactions between different brain regions with no particular stimulus presentation. The hypothesis that formulates ADHD as a disorganized brain connectivity has strongly been supported (10-12). Connectivity analyses have tried to clarify the neurobiological underpinning and have identified either lower or higher values for functional connectivity measures in various brain networks (13). The possible functional deviations of the default mode network (DMN) in ADHD have been evident throughout the literature (14). A pioneering rs-fMRI study on ADHD adults indicated lower functional connectivity values within the DMN and between the caudal parts of the DMN and the dorsal anterior cingulate cortex (15). The executive control network (ECN)-amplitude of low-frequency fluctuation was the most associating pattern for impulsivity. Abnormal trends of inter-hemispheric functional connectivity were also detected in the sensorimotor network connectivity pattern in neurodevelopmental disorders (16). Higher functional connectivity within the ECN was correlated with the more subtle clinical symptoms (17).
The fMRI data analysis is a complicated process. One promising approach to analyzing fMRI data and associated functional connectivities has been neural networks and machine learning. Classification techniques are among the principles of supervised machine learning algorithms. In these models, the blood oxygenation level-dependent (BOLD) imaging properties of voxels are considered predictor variables. Regarding the three main stages of the machine learning procedure, namely feature selection, feature extraction, and classifier selection, numerous studies have developed and improved one or more stages of this set and applied them for ADHD classification. Support vector machine (SVM) with recursive feature elimination (18), least absolute shrinkage and selection operator (LASSO) (19), and elastic net (20) are among the well-sophisticated methods that have been applied for the machine-learning-based classifications.
A graph-kernel regularized LASSO has also been applied to functional connectivity matrices, which preserves the local structure among the featured functional connectivities (FCs) (21). Similarly, for the feature extraction section, an accumulative body of evidence showing efficiency (principal component analysis and independent component analysis) was primarily introduced to learn the features (20, 22). More recently, a sparse representation model was introduced for FCs to recognize individuals with ADHD diagnosis (23). From a different perspective, since FCs could provide a brain topography, specific measures are designed to explore the features of the FC network (24, 25). Among these methods, an integrated fMRI technique (26) has attracted more attention for its ability to recognize reliable FCs through affinity propagation clustering on the FC network. Additionally, to increase classification accuracy, this method has analyzed non-imaging variables, including age, gender, and intelligence quotient (26).
To the best of our knowledge, due to the high fitness level for small-size data, the classifiers with SVM (24, 27) and extreme learning machines (28) have been frequently applied for ADHD classification. Furthermore, a bi-objective classification model based on SVM (29) was recently developed and introduced to drastically improve classification accuracy. This approach takes advantage of a bi-objective optimization function in the traditional L1-norm SVM. When dealing with high-dimensional multivariate data, SVMs, with their robust characteristics (30), are vastly used, additionally providing individual-specific predictions.
One of the widespread methods for classifying multimodal neuroimaging findings in the clinical setting is the SVM (31). This method provides a high level of classification accuracy, and with the smaller number of samples, there would not be an overfitting problem (32).
The efficient analysis of high-dimension low-sample-size (HDLSS) data has been an abiding concern when conventional machine learning methods encounter performance degradation for classification. Data pilling occurs in the HDLSS workspace through the data mapping onto the separating hyperplane. Data piling could impinge the generalization sufficiency of the SVM in some HDLSS states. Distance-weighted discrimination (DWD) (33) confronts the data-piling problem and gives the anticipated improved generalizability. To find the best-separating hyperplane, DWD estimates coefficients by solving the below optimization problem.
Although the distance-weighted approaches can resolve the data-piling phenomenon, they are computationally laborious since they require second-order cone programming rather than quadratic programming. A recently developed linear binary classifier, denoted by data maximum dispersion classifier (DMDC) (34), would be beneficial. The DMDC maximizes data dispersion in projection space, resulting in the remarkable prevention of the data-pilling problem. Additionally, it could be applied competently on HDLSS without any sensitivity to the intercept, and the implementation would be straightforward with more subtle computational complexities.
2. Objectives
Despite the remarkable results of the classification methods, these data categories are still difficult to interpret. Therefore, this study aimed to fit the three models of SVM, DWD, and DMDC on inverse covariance matrix and inverse sparse covariance matrix (as the connectional network of the brain) and compare these six types of modeling using indices of classification evaluation. In order to achieve the pattern of brain functional connectivities in two groups (i.e., ADHD subjects and healthy controls), the sparse model (eliminating the non-efficient brain regions) was applied in this study.
3. Methods
3.1. Data and Preprocessing
The online available fMRI data of the ADHD-200 Global Competition, which aimed to achieve the best differentiating model for the diagnosis and discovery of the neurological markers of ADHD, were used in this study (35). These data include the diagnosis status of ADHD, individual characteristics, and fMRI brain scans of 973 individuals aged 7 - 21 years. Regarding the inclusion/exclusion criteria, each included center had applied a set of diagnostics and complementary assessments. All the centers performed a comprehensive interview-based assessment based on the diagnostic and statistical manual of mental disorders framework by a trained psychiatrist (for a structured diagnosis of the disorder and ruling out the comorbidities). The specific details of the diagnostic procedures and rating scales are provided on the database website. The current project was performed with the approval ID of IR.SBMU.PHNS.REC.1399.193 and was evaluated by research ethics committees of the School of Public Health and Neuroscience Research Center, Shahid Beheshti University of Medical Sciences, Tehran, Iran.
In the competition, the data from 776 individuals were considered training, and the remaining 197 data were regarded as tests. The current analysis considered 768 and 171 training and test data, respectively. The available rs-fMRI data were obtained within 120 - 344 time points by eight valid imaging centers in the United States, China, and the Netherlands. The FSL software (version 6.0.1) was employed for the preprocessing parts. Six specific parameters were performed for the MCFLIRT and rigid-body motion correction sections. Moreover, the slice-timing correction was specified according to the data acquisition process exclusively for each center. The functional data were band-pass filtered (within the range of 2.8 - 60 Hz) and mapped to a template space (MNI-152) by a non-linear method. The smoothing procedure was performed by a three-dimensional Gaussian kernel (full width at half maximum = 6 mm). The region of interest-associated mean time series was calculated for each participant based on the automated anatomical labeling 1 standard atlas, and the total brain volume was divided into 116 regions. The value of the BOLD signal in each of these areas is considered the average BOLD signal of the voxels located in that area. A bash code system was developed and used to standardize and automate the preprocessing parts.
3.2. Data Analysis
The applied models were as follows respectively:
Based on a hyperplane (decision boundary), SVM categorizes data samples into two groups within a high-dimensional context. The hyperplane
Regarding the second model, DWD, to find the best-separating hyperplane, DWD estimates coefficients by solving the following optimization problem:
The DMDC solves the convex quadratic programming formulation similar to SVM as follows:
A is the eigenvector that is the highest eigenvalue of Sβ.
uj implies the mean of training samples from jth class, j = 1, 2. The term AT β controls training samples from two classes, accompanied by the projecting direction denoted by the β.
The following parts are the detailed explanation of data analysis presented respectively.
3.3. Optimization of λ Parameter for Estimation of Inverse Sparse Covariance Matrix
Only the training data and BOLD signal variables of the 116 brain regions were used in this study. There were 120 to 344 views for each person. The training data were divided into ten relatively equal sections, with 28 affected and 49 non-affected in each section randomly selected. The inverse of the sparse covariance matrix for patients and non-patients was estimated separately in each section, with the three usual values of λ, namely 0.1, 0.01, and 0.001, and the best λ was identified with the lowest Bayesian information criterion (BIC). Finally, in the two groups of patients and non-patients, the λ value with the highest frequency in the optimal λ in all the ten sections was considered the optimal λ. Moreover, the optimal λ mean in both groups was used for all data.
3.4. Estimation of Sparse Inverse Covariance Matrix with LASSO Graphical Model
At this stage, the inverse of the covariance matrix and the inverse of the sparse covariance matrix were estimated using the LASSO graphical model based on the optimal λ obtained from the previous step. Two matrices of 116 × 116 were considered for each individual such that every element represented the correlation of activity or relationship between the two brain regions.
3.5. Creation of Proper Data Structure for Classification Model
The obtained correlation was then considered an information vector for each individual. Since the inverse of the covariance matrix and the inverse of the sparse covariance matrix are symmetric, the upper triangular elements were considered. The number of these elements is 6670. The new data structure was designed to have one row per person and consider 6670 brain regions as variables. After the incorporation of metadata, such as the personal characteristics of each participant and the disorder status, into the connection space, the organized data were ready for classification. Furthermore, since the fMRI data and the individual characteristics were disparately dispersed, the values of the quantitative variables were also standardized.
3.6. Penalty Parameter C Optimization for SVM, DWD, and DMDC Models
Then, the training data were exclusively used. Since two datasets based on the inverse covariance matrix, the sparse inverse covariance matrix, and SVM, DWD, and DMDC models are considered for comparison, all of the measures in this part were implemented for all six states. In order to optimize the parameter C, the training dataset was divided into ten groups according to the details of the parameter λ optimization step. At this stage, a cross-validation scheme was used; accordingly, nine parts of the data were considered training data sets, and one part was regarded as a testing set. Then, SVM, DWD, and DMDC models were fitted to the training data. For parameter C, the values of 0.00001, 0.0001, 0.001, 0.01, 0.1, 1, 10, 100, 1000, 10000, and 100000 were computed. At every ten times fitting of the model and its evaluation, the value of C that is higher than the balanced classification rate (BCR) criterion was chosen in each test, and then the most frequent (the mode value) value for the parameter C was selected as the optimal one for the whole process. At this stage, six optimal Cs were obtained for a combination of three models and two (sparse and non-sparse) inverse covariance matrices.
3.7. Fitting SVM, DWD, and DMDC Models
By considering the optimal C values obtained from the previous step, SVM, DWD, and DMDC models were fitted to the data obtained from the inverse covariance matrix and the sparse inverse of the covariance matrix to the training data.
3.8. Evaluation of Models with Testing Data
The testing data were classified with the fitted models in the previous step, and the results were compared with the actual labels. Then, sensitivity and specificity, the overall accuracy of the two models, and BCR were calculated. The models were also compared based on the aforementioned indices. Data analysis was performed in R software (version 4.1.1). To fit the LASSO graphic model, the glasso package (1.11) was used, and SVM and DWD models were fitted on the Liblinear (2.10 - 22) and DWD Large (0.1 - 0) R package (version 4.1.1). Regarding the DMDC method, the associated code was written in R. Additionally, all the steps of estimating the optimal parameters λ and C were coded in R.
3.9. Visualization/Illustration
Subsequently, to more easily interpret the coefficients estimated by the chosen research model, these coefficients were imaged on the brain. This visualization was performed by the BrainNet Viewer module in MATLAB software (version 2013) (Figure 1).
Significant connections in attention deficit-hyperactivity disorder diagnosis based on the best-fitted model (i.e., data maximum dispersion classifier on sparse inverse covariance matrix). The more the coefficient magnitude is positively correlated with the more magnitude of edges. The blue and yellow edges are related to the positive and negative coefficients, respectively. Blue = direct correlation, yellow = inverse correlation with the diagnosis status.
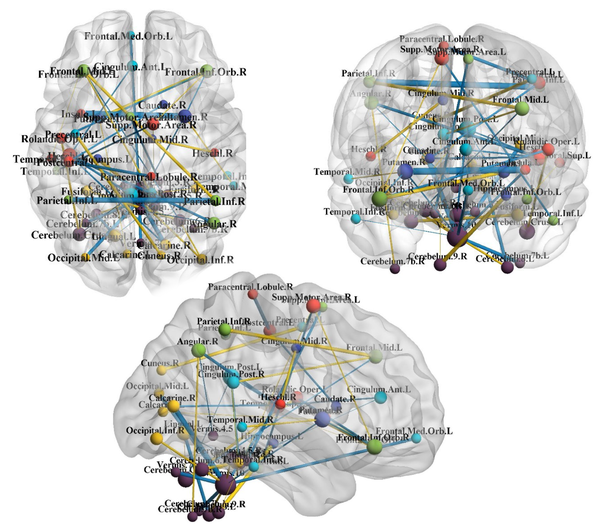
3.10. Model Assessment Criteria
In order to assess the graphical model of LASSO, the BIC was applied as follows:
In which the
4. Results
Table 1 shows a summary of the descriptive statistics of the variables for the individual characteristics. The minimum and maximum values of the brain BOLD were -181.69 and 250.81, respectively. Moreover, the median, by considering the semi-interquartile range, was 0.38.
Descriptive Statistics of Individual Properties
Variables and Classes | ADHD Subjects | Healthy Controls | Total |
---|---|---|---|
Gender | |||
Female | 72 (20.6) | 278 (79.4) | 350 (37.3) |
Male | 285 (48.4) | 304 (51.6) | 589 (62.7) |
Hand dominance | |||
Left-handed | 134 (53.6) | 116 (46.4) | 250 (26.6) |
Right-handed | 223 (32.4) | 466 (67.6) | 689 (73.4) |
Total | 357 (38) | 582 (62) | |
Age (y) | Values | ||
Minimum - maximum | 7 - 21 | ||
Median | 11 | ||
Interquartile range (Q3-Q1) | 4 (13 - 9) |
4.1. Assessment of Models
Among the three λ values considered for the inverse estimation of the sparse covariance matrix, λ = 0.1 with the lowest BIC value was selected as the optimal one. In order to illustrate the concept of sparse, examples of variance-covariance matrix and sparse inverse matrix of variance-covariance are depicted in Figure 2. Figure 2 shows the variance-covariance matrix of the activity of 116 brain regions of an individual. In this variance-covariance matrix, the values are indicated by the color spectrum.
Samples of A, variance-covariance matrix; and B, inverse of sparse variance-covariance matrix. Part A shows the variance-covariance matrix of the 116 brain-associated activities. After the sparsing process, most of the matrix entities were zero, and only the prominent connections had non-zero values.
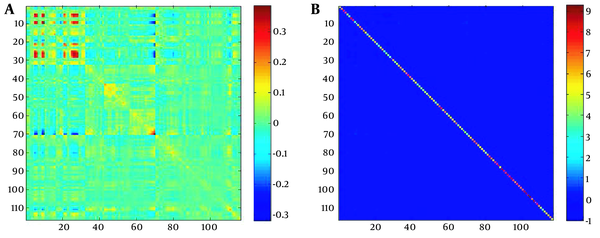
By comparing two types of matrices, it could be observed that after the sparsing process, most of the matrix items became zero, and exclusively the critical connections adopted non-zero values. As stated earlier, considering the inverse of the covariance matrix as sparse and non-sparse and the sparse and non-sparse SVM models, there were four different modeling modes; the results of each are as follows.
4.2. Evaluation of SVM, DWD, and DMDC Models on Non-sparse and Sparse Inverse Covariance Matrices
In this case, Table 2 shows the confusion matrix made by the SVM, DWD, and DMDC models and the associated evaluation indices for six states. As can be observed, the overall accuracy of the model, the ratio of correct predictions to the total number of data, is 49% for the SVM model of the inverse covariance matrix (the optimal γ value obtained from the BCR-based cross-validation as 100000). The sensitivity and its specificity were reported as 51% and 48%, respectively. The overall accuracy and BCR values were 63% and 59% for this model on the inverse of the sparse covariance matrix, respectively (the optimal γ value obtained from the BCR-based cross-validation as 1000). The SVM model for the inverse of the sparse covariance matrix was indicated to be 30% sensitive and 89% specific, which showed poorer performance in predicting affected subjects rather than non-affected ones.
Results of Support Vector Machine, Distance-Weighted Discrimination, and Data Maximum Dispersion Classifier on Non-sparse and Sparse Inverse Covariance Matrices
ADHD | Matrix | Predicted by the Model | Specificity (%) | Sensitivity (%) | Accuracy (%) | BCR (%) | |
---|---|---|---|---|---|---|---|
No | Yes | ||||||
Reality | SVM | ||||||
Inverse covariance matrix | 48 | 51 | 49 | 49 | |||
No | 45 | 49 | |||||
Yes | 38 | 39 | |||||
Inverse sparse covariance matrix | 89 | 30 | 63 | 59 | |||
No | 84 | 10 | |||||
Yes | 54 | 23 | |||||
DWD | |||||||
Inverse covariance matrix | 36 | 39 | 37 | 38 | |||
No | 34 | 60 | |||||
Yes | 47 | 30 | |||||
Inverse sparse covariance matrix | 99 | 6 | 57 | 53 | |||
No | 93 | 1 | |||||
Yes | 72 | 5 | |||||
DMDC | |||||||
Inverse covariance matrix | 40 | 64 | 51 | 52 | |||
No | 38 | 56 | |||||
Yes | 28 | 49 | |||||
Inverse sparse covariance matrix | 56 | 64 | 60 | 60 | |||
No | 45 | 36 | |||||
Yes | 32 | 58 |
Regarding the DWD model, the total accuracy of the inverse covariance matrix (the optimal γ value obtained from the BCR-based cross-validation as 100) was obtained as 37%. The sensitivity and specificity were 39% and 36%, respectively. The overall accuracy and BCR values were 57% and 53% for this model on the inverse sparse covariance matrix, respectively (the optimal γ value obtained from the BCR-based cross-validation as 10). The DWD model for the inverse of the sparse covariance matrix was indicated to be 6% sensitive and 99% specific, which showed very poor performance in predicting affected subjects and very well in non-affected ones.
The inverse covariance matrix (the optimal γ value obtained from the BCR-based cross-validation as 100) within the DMDC model showed a total accuracy of 51%, with sensitivity and specificity of 64% and 40%, respectively. The overall accuracy and BCR values were 60% and 60% for this model on the inverse of the sparse covariance matrix, respectively (the optimal γ value obtained from the BCR-based cross-validation as 10). The performance of the DWD model for the inverse of the sparse covariance matrix was 64% sensitive and 56% specific.
4.3. Comparison of Models
Figure 3 illustrates the evaluation criteria for comparing SVM, DWD, and DMDC models based on the inverse covariance matrices and inverse of the sparse covariance matrices. Accordingly, the highest value for the total accuracy was for the SVM model on the sparse covariance matrix. The highest values for the BCR and sensitivity were for DMDC on the sparse covariance matrix. Moreover, the best level of specificity was obtained from DWD using the sparse covariance matrix. The highest levels of values (i.e., total accuracy and BCR) were achieved through the model fitting on the sparse matrices. Among the six models, the DMDC model on sparse covariance matrix was the most optimal algorithm due to the superiority of the two indices and the favorable balance between sensitivity and specificity values.
Comparison of the Acc, sensitivity, specificity, and BCR, within the SVM, DWD, and DMDC models. Abbreviations: Acc, accuracy; BCR, balanced criterion rate; SVM, support vector machine; DWD, distance-weighted discrimination; DMDC, data maximum dispersion classifier.
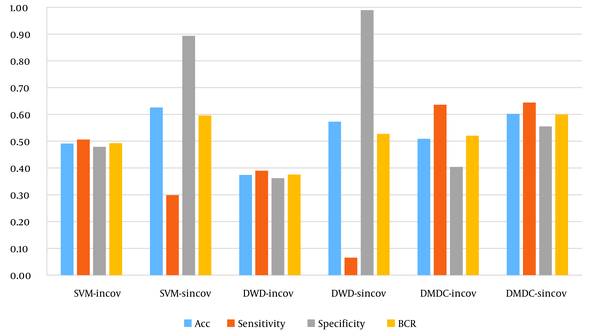
4.4. Interpretation of Selected Model Coefficients
A sparse version of DMDC was used for the pattern recognition process. This section deals with the interpretation of the coefficients of the selected model. The estimated coefficient comprises 55 non-zero in the model. Table 3 shows the coefficients for the individual characteristics. As can be observed, male gender, younger ages, and left-handedness are positively correlated with ADHD. The magnitude of the coefficients indicated the strength of the relationship; in other words, ADHD was more strongly correlated with gender and hand dominance than age.
Estimated Coefficients for Individual Characteristics by the Selected Model
Predicting Variable | Coefficients |
---|---|
Gender | 0.43 |
Age | -0.23 |
Hand dominance | -0.18 |
As mentioned earlier, other predictor variables were the covariance of the extent of activity in different brain regions. Therefore, it can be said that the non-zero coefficients of the selected model refer to the brain connections that are important in the classification of ADHD. Table 4 shows these connections and their values. Positive coefficients showed correlations directly related to ADHD. Negative coefficients indicated relationships inversely related to ADHD. The magnitude of the coefficients indicated the importance of the relationship. Figure 1 illustrates the areas affected by ADHD from the three perspectives above the head, right ear, and front of the head. In these figures, the size of an area or node is proportional to the number of communications associated with that area. In other words, larger nodes indicate the greater importance of the regions in ADHD. Figure 1 illustrates the network communication of brain regions in ADHD and part of the output of the classification model.
Positive and Negative Correlation Coefficients; Positive Values Implying Connections with Direct Association with Attention Deficit-Hyperactivity Disorder Diagnosis, and Negative Values Implying Inverse Correlations
Regional Connections | Coefficients |
---|---|
Positive Correlations | |
Putamen_R&Insula_L | 0.20 |
Parietal_Inf_L&Parietal_Inf_R | 0.175 |
Vermis_10&Calcarine_R | 0.141 |
Vermis_1_2&Cerebelum_3_L | 0.108 |
Vermis_10&Occipital_Mid_L | 0.108 |
Vermis_10&Cerebelum_7b_L | 0.098 |
Vermis_10&Vermis_7 | 0.095 |
Paracentral_Lobule_R&Postcentral_L | 0.091 |
Cerebelum_8_L&Lingual_L | 0.087 |
Vermis_10&Frontal_Med_Orb_L | 0.079 |
Heschl_L&Angular_R | 0.078 |
Vermis_10&Heschl_L | 0.062 |
Cerebelum_3_R&Supp_Motor_Area_L | 0.062 |
Rolandic_Oper_L&Frontal_Inf_Orb_R | 0.060 |
Vermis_1_2&Cingulum_Post_L | 0.051 |
Temporal_Inf_L&Supp_Motor_Area_R | 0.049 |
Caudate_R&Postcentral_L | 0.047 |
Heschl_L&Calcarine_R | 0.042 |
Heschl_L&Cingulum_Ant_L | 0.032 |
Cingulum_Post_R&Cingulum_Post_L | 0.031 |
Vermis_10&Putamen_R | 0.031 |
Heschl_L&Cingulum_Post_L | 0.030 |
Putamen_R&Frontal_Inf_Orb_R | 0.028 |
Cerebelum_6_R&Frontal_Mid_L | 0.025 |
Vermis_4_5&Angular_R | 0.013 |
Cerebelum_Crus1_L&Cingulum_Post_L | 0.007 |
Cerebelum_4_5_R&Lingual_L | 0.005 |
Vermis_1_2&Cingulum_Ant_L | 0.003 |
Vermis_10&Temporal_Inf_R | 0.003 |
Negative Correlations | |
Cerebelum_9_R&Hippocampus_L | -0.109 |
Putamen_L&Frontal_Inf_Orb_R | -0.104 |
Parietal_Inf_R&Frontal_Mid_L | -0.098 |
Cuneus_R&Precentral_L | -0.089 |
Cerebelum_6_R&Fusiform_L | -0.078 |
Heschl_R&Cingulum_Mid_R | -0.066 |
Cerebelum_Crus1_L&Rolandic_Oper_L | -0.059 |
Vermis_10&Occipital_Inf_R | -0.054 |
Vermis_10&Cerebelum_8_L | -0.053 |
Cingulum_Post_R&Frontal_Mid_L | -0.046 |
Vermis_10&Cerebelum_4_5_R | -0.039 |
Cerebelum_7b_R&Angular_R | -0.037 |
Cerebelum_6_R&Occipital_Inf_R | -0.034 |
Temporal_Mid_R&Putamen_R | -0.032 |
Supp_Motor_Area_R&Frontal_Inf_Orb_R | -0.019 |
Cerebelum_Crus1_L&Fusiform_L | -0.018 |
Fusiform_R&Cingulum_Post_L | -0.009 |
Putamen_L&Calcarine_L | -0.005 |
Cerebelum_9_R&Supp_Motor_Area_R | -0.001 |
Temporal_Sup_L&Frontal_Inf_Orb_L | -0.001 |
Cerebelum_Crus1_L&Lingual_L | -0.001 |
5. Discussion
Throughout the present study, it was aimed to apply network-based models, SVM, DWD, and DMDC, to classify ADHD-associated rs-fMRI data. The inverse of the covariance matrix was considered the item that best represents the brain communication networks and the predictive variables of the classification models in both sparse and non-sparse states. Ultimately, the classification models were fitted to the training data and were evaluated using the test data. The obtained results also demonstrated that the cerebellar and basal ganglia-related areas were more crucial in providing informative distinctions between ADHD and healthy participants. According to the results of the currently used models, it might be concluded that the inverse covariance matrices and one sparsing step could significantly improve the performance of the model and enhance the efficiencies of all models in the classification procedures. Moreover, reducing the non-zero coefficients from 6675 to 55 might bring more interpretation power in the coefficients and could provide more possibilities for creating a brain communication network that affects or emerges from the phenomenology of ADHD. When these models were applied to the sparse inverse covariance matrix, again, it generated more sensitivity and higher BCR values. Among the current models, the DMDC performance for the sparse covariance matrix gained the most optimality.
The best model presented in the Global Competition belongs to the Johns Hopkins University team, a cumulative model with 61% overall accuracy, 57.5% balanced rating, 21% sensitivity, and 94% specificity (36). The J-statistic is the sum of the sensitivity and specificity minus one and can be equal to the area below the surface of the receiver operating characteristic curve (37). The J-statistic in the present study was 0.31. In 2013, Ghiassian et al. demonstrated that as the number of predictor variables increased to 211, the classification performance was reduced, and their overall model accuracy in the balanced data was 62.5 (38). In this case, it can be said that in the present study, using the sparse vector machine eliminated the need for selecting effective predictive variables before modeling by automatically selecting the variables. In a 2013 study by Hart et al., by fitting the Gaussian process classification model to fMRI data obtained from an event-oriented design in 60 right-handed male adolescents aged 10 - 17 years for identifying ADHD, the sensitivity, specificity, and overall accuracy rates were reported as 90%, 63%, and 77% (39). The higher performance of the aforementioned study seems to be a result of employing the event-oriented design for data collection, which has a higher signal intensity than the rest state. Limiting age, gender, and hand preference can also cause a difference in performance (39).
In 2015, Rosa et al. (40) compared the performance of two models of L1-norm and L2-norm SVMs in classifying patients with acute depressive disorder with two stages of sparseness, namely the sparse inverse matrix covariance and the SVM model. Rosa et al. observed more improved classification performance. Nevertheless, in the present study, it was concluded that the sparsity of the covariance matrix, in addition to using a sparse SVM model, slightly reduced the classification performance. The conclusions of the two studies are differential, probably due to the applied atlases and the greater number of predictor variables (9316 variables) in the study by Rosa et al.
In 2015, Pastor et al. estimated the prevalence of ADHD in the United States to be 13.3% and 5.6% in male and female adolescents, respectively (41). The estimates of these studies with conclusions about gender coefficient are consistent with the results of the present study. The associations between left-handedness and ADHD have not been consistent throughout the studies. Although abnormal brain laterality is reported in children with ADHD, the correlations with the severity, age, gender, comorbid psychiatric problems, and parental characteristics are extremely vague. In a 2012 study, Ghanizadeh reported that left-handedness did not show remarkable associations with higher inattentiveness or hyperactivity (parent-reported). Although hand-use preference is not gender-specific in ADHD (42), there are still conflicting findings (43).
Schmidt et al. demonstrated that ADHD is significantly more prevalent among left-handed individuals, which is in line with the interpretation of the variable of hand dominance in the present study (44). The prevalence of ADHD in children and adolescents is higher than in adults, which is consistent with the negative age coefficient interpretation in this study, as previously discussed.
In their 2012 study, Cheng et al. concluded that the most effective brain regions for ADHD are the cerebellar and prefrontal cortices (45). In the present study, out of the 12 most influential areas, six items were in these two general areas. More specifically, in the present study, the connectivity between the right part of the putamen and the left insular regions was also observed to be positively correlated with ADHD diagnosis. A reliable line of evidence indicated the role of basal ganglia compartments (46), such as putamen (47, 48), in the pathophysiological course of ADHD.
Putamen have had integral roles in both movement-related components and somatosensory processing, and both of these are remarkably impaired in ADHD states. A novel work by Tang et al. demonstrated that the impaired basal ganglia morphological structure could be an evident distinction between ADHD male and female adolescents; this deficiency played essential roles in controlling motor responses; accordingly, male adolescents with ADHD showed increased commission error rates and greater variabilities in responses regardless of task requirements (49). Similarly, higher connectivity values were observed in the corticostriatal circuits in children with hyperactive-impulsive ADHD; nevertheless, inattentive individuals showed strong communications across the ventral part of the attentional network (50). Conversely, a recent study by Mostert et al. in adult ADHD demonstrated significant connectivity values in the anterior cingulate node of the executive control center without any distinctions in the areas of the basal ganglia and the DMN (51). Owing to the strategic site of the insular cortex, it integrally contributes to a broad spectrum of functions encompassing sensorimotor, olfaction-gustatory, socio-emotional, and cognitive functions (52). More specifically, regarding the central role of the putamen and insula in somatosensory and executive functions, it would not be surprising to see their functional link in making a distinction between patients and healthy individuals statistically significant (53).
The functional connectivity between the right parts of the cerebellum and the left parts of the hippocampus has shown an inverse correlation with the ADHD diagnosis. Despite the classical contributions of cerebellar apparatus in schematizing the movement-related parameters, different anatomical and functional sections of this highly intelligent part of the central nervous system have increasingly gained attention considering roles in the higher cognitive functions, such as fine spatiotemporal coordination and perceptions that subsequently could affect a broad range of performances (54). The hippocampus and particularly its rostral parts are connected to the cerebellum and, via this interaction, subserve different dimensions of spatial representation, spatial navigation (i.e., both allocentric and egocentric navigation), spatial learning, and pattern recognition processes (55). Regardless of the isolated roles of the hippocampus and cerebellum in ADHD, the emergent function of their associations could also be a dimension of the ADHD pathophysiology, as these patients have challenging troubles in spatial working memory tasks (56).
Regarding negative correlations in the present study, the relationship between the right posterior cingulate and the left middle frontal cortex regions was also observed to be inversely correlated with ADHD. Fair et al., in an assessment of brain communication in 7-16-year-old subjects with ADHD, showed that the communication between the posterior cingulate cortex and frontal areas was less pronounced than the subjects with this disorder (14).
A spectrum of deficits in different domains of attention, emotion processing, emotion regulation, and various manifestations of social cognition are frequently-observed phenomena in disordered populations and health conditions that challenge appropriate distinctions. This ambiguity is even more pronounced in neurodevelopmental disorders. Since the clinical manifestations are not fully completed yet, there are significant troubles in diagnostics and therapeutics in the lower age ranges, and co-occurring clinical profiles (e.g., autism and ADHD) are prevalent. Moreover, despite the high dimensionality of neuroimaging datasets, the centers do not usually provide sufficient sample size regarding neurodevelopmental disorders (probably due to the difficulties in image acquisition in this population); therefore, applying an efficient computational model which can classify the subjects with this disorder and healthy counterparts might be a promising approach. The DMDC model is one of these types and can be an optimum modeling approach regarding neuroimaging datasets. The utilization of these machine learning-based models would be more feasible if integrated with diagnostic software and applications.
5.1. Conclusions
Employing mathematical models more compatible with the HDLSS data, such as DMDC, can remarkably improve the results of classification processes and outperform the other existing methods. The application of these approaches to neuronal-associated parameters comprising functional connectivity might be an optimal tool for differentiating between ADHD individuals and healthy counterparts. Based on the present findings, the neuronal connectivity among subcortical structures comprising parts of the basal ganglia and cerebellum provides a distinction between ADHD subjects and healthy controls. Moreover, based on the present results, ADHD has a direct relationship with the male gender, left-handedness, and younger ages.
Acknowledgements
References
-
1.
Polanczyk G, de Lima MS, Horta BL, Biederman J, Rohde LA. The worldwide prevalence of ADHD: a systematic review and metaregression analysis. Am J Psychiatry. 2007;164(6):942-8. [PubMed ID: 17541055]. https://doi.org/10.1176/ajp.2007.164.6.942.
-
2.
Ghassemi F, Hassan_Moradi M, Tehrani-Doost M, Abootalebi V. Using non-linear features of EEG for ADHD/normal participants’ classification. Procedia Soc Behav Sci. 2012;32:148-52. https://doi.org/10.1016/j.sbspro.2012.01.024.
-
3.
Tannock R. Rethinking ADHD and LD in DSM-5: proposed changes in diagnostic criteria. J Learn Disabil. 2013;46(1):5-25. [PubMed ID: 23144062]. https://doi.org/10.1177/0022219412464341.
-
4.
Zhan X, Yu R. A Window into the Brain: Advances in Psychiatric fMRI. Biomed Res Int. 2015;2015:542467. [PubMed ID: 26413531]. [PubMed Central ID: PMC4564608]. https://doi.org/10.1155/2015/542467.
-
5.
Kochunov P, Hong LE, Dennis EL, Morey RA, Tate DF, Wilde EA, et al. ENIGMA-DTI: Translating reproducible white matter deficits into personalized vulnerability metrics in cross-diagnostic psychiatric research. Hum Brain Mapp. 2022;43(1):194-206. [PubMed ID: 32301246]. [PubMed Central ID: PMC8675425]. https://doi.org/10.1002/hbm.24998.
-
6.
Samea F, Soluki S, Nejati V, Zarei M, Cortese S, Eickhoff SB, et al. Brain alterations in children/adolescents with ADHD revisited: A neuroimaging meta-analysis of 96 structural and functional studies. Neurosci Biobehav Rev. 2019;100:1-8. [PubMed ID: 30790635]. [PubMed Central ID: PMC7966818]. https://doi.org/10.1016/j.neubiorev.2019.02.011.
-
7.
Oztekin I, Finlayson MA, Graziano PA, Dick AS. Is there any incremental benefit to conducting neuroimaging and neurocognitive assessments in the diagnosis of ADHD in young children? A machine learning investigation. Dev Cogn Neurosci. 2021;49:100966. [PubMed ID: 34044207]. [PubMed Central ID: PMC8167232]. https://doi.org/10.1016/j.dcn.2021.100966.
-
8.
Zanatta DP, Rondinoni C, Salmon CEG, Del Ben CM. Brain alterations in first episode depressive disorder and resting state fMRI: A systematic review. Psychol Neurosci. 2019;12(4):407-29. https://doi.org/10.1037/pne0000165.
-
9.
Specht K. Current Challenges in Translational and Clinical fMRI and Future Directions. Front Psychiatry. 2020;10:924. [PubMed ID: 31969840]. [PubMed Central ID: PMC6960120]. https://doi.org/10.3389/fpsyt.2019.00924.
-
10.
Ghaderi AH, Nazari MA, Shahrokhi H, Darooneh AH. Functional Brain Connectivity Differences Between Different ADHD Presentations: Impaired Functional Segregation in ADHD-Combined Presentation but not in ADHD-Inattentive Presentation. Basic Clin Neurosci. 2017;8(4):267-78. [PubMed ID: 29158877]. [PubMed Central ID: PMC5683684]. https://doi.org/10.18869/nirp.bcn.8.4.267.
-
11.
Castellanos FX, Aoki Y. Intrinsic Functional Connectivity in Attention-Deficit/Hyperactivity Disorder: A Science in Development. Biol Psychiatry Cogn Neurosci Neuroimaging. 2016;1(3):253-61. [PubMed ID: 27713929]. [PubMed Central ID: PMC5047296]. https://doi.org/10.1016/j.bpsc.2016.03.004.
-
12.
Gallo EF, Posner J. Moving towards causality in attention-deficit hyperactivity disorder: overview of neural and genetic mechanisms. Lancet Psychiatry. 2016;3(6):555-67. [PubMed ID: 27183902]. [PubMed Central ID: PMC4893880]. https://doi.org/10.1016/S2215-0366(16)00096-1.
-
13.
Tomasi D, Volkow ND. Abnormal functional connectivity in children with attention-deficit/hyperactivity disorder. Biol Psychiatry. 2012;71(5):443-50. [PubMed ID: 22153589]. [PubMed Central ID: PMC3479644]. https://doi.org/10.1016/j.biopsych.2011.11.003.
-
14.
Fair DA, Posner J, Nagel BJ, Bathula D, Dias TG, Mills KL, et al. Atypical default network connectivity in youth with attention-deficit/hyperactivity disorder. Biol Psychiatry. 2010;68(12):1084-91. [PubMed ID: 20728873]. [PubMed Central ID: PMC2997893]. https://doi.org/10.1016/j.biopsych.2010.07.003.
-
15.
Castellanos FX, Margulies DS, Kelly C, Uddin LQ, Ghaffari M, Kirsch A, et al. Cingulate-precuneus interactions: a new locus of dysfunction in adult attention-deficit/hyperactivity disorder. Biol Psychiatry. 2008;63(3):332-7. [PubMed ID: 17888409]. [PubMed Central ID: PMC2745053]. https://doi.org/10.1016/j.biopsych.2007.06.025.
-
16.
Anderson JS, Druzgal TJ, Froehlich A, DuBray MB, Lange N, Alexander AL, et al. Decreased interhemispheric functional connectivity in autism. Cereb Cortex. 2011;21(5):1134-46. [PubMed ID: 20943668]. [PubMed Central ID: PMC3077433]. https://doi.org/10.1093/cercor/bhq190.
-
17.
Francx W, Oldehinkel M, Oosterlaan J, Heslenfeld D, Hartman CA, Hoekstra PJ, et al. The executive control network and symptomatic improvement in attention-deficit/hyperactivity disorder. Cortex. 2015;73:62-72. [PubMed ID: 26363140]. https://doi.org/10.1016/j.cortex.2015.08.012.
-
18.
Colby JB, Rudie JD, Brown JA, Douglas PK, Cohen MS, Shehzad Z. Insights into multimodal imaging classification of ADHD. Front Syst Neurosci. 2012;6:59. [PubMed ID: 22912605]. [PubMed Central ID: PMC3419970]. https://doi.org/10.3389/fnsys.2012.00059.
-
19.
Zhao Y, Ogden RT, Reiss PT. Wavelet-based LASSO in functional linear regression. J Comput Graph Stat. 2012;21(3):600-17. [PubMed ID: 23794794]. [PubMed Central ID: PMC3685865]. https://doi.org/10.1080/10618600.2012.679241.
-
20.
Nuñez-Garcia M, Simpraga S, Jurado MA, Garolera M, Pueyo R, Igual L. FADR: Functional-Anatomical Discriminative Regions for Rest fMRI Characterization. In: Zhou L, Wang L, Wang Q, Shi Y, editors. Machine Learning in Medical Imaging. MLMI 2015. Lecture Notes in Computer Science. Vol. 9352. Cham: Springer; 2015. p. 61-8. https://doi.org/10.1007/978-3-319-24888-2_8.
-
21.
Wang M, Jie B, Bian W, Ding X, Zhou W, Wang Z, et al. Graph-Kernel Based Structured Feature Selection for Brain Disease Classification Using Functional Connectivity Networks. IEEE Access. 2019;7:35001-11. https://doi.org/10.1109/access.2019.2903332.
-
22.
Tabas A, Balaguer-Ballester E, Igual L. Spatial discriminant ICA for RS-fMRI characterisation. 2014 International Workshop on Pattern Recognition in Neuroimaging. 4-6 June 2014; Tuebingen, Germany. 2014. p. 1-4.
-
23.
Zhang Y, Tang Y, Chen Y, Zhou L, Wang C. ADHD classification by feature space separation with sparse representation. 2018 IEEE 23rd International Conference on Digital Signal Processing (DSP). 19-21 November 2018; Shanghai, China. 2018. p. 1-5.
-
24.
Dey S, Rao AR, Shah M. Attributed graph distance measure for automatic detection of attention deficit hyperactive disordered subjects. Front Neural Circuits. 2014;8:64. [PubMed ID: 24982615]. [PubMed Central ID: PMC4058754]. https://doi.org/10.3389/fncir.2014.00064.
-
25.
dos Santos Siqueira A, Biazoli Junior CE, Comfort WE, Rohde LA, Sato JR. Abnormal functional resting-state networks in ADHD: graph theory and pattern recognition analysis of fMRI data. Biomed Res Int. 2014;2014:380531. [PubMed ID: 25309910]. [PubMed Central ID: PMC4163359]. https://doi.org/10.1155/2014/380531.
-
26.
Riaz A, Asad M, Alonso E, Slabaugh G. Fusion of fMRI and non-imaging data for ADHD classification. Comput Med Imaging Graph. 2018;65:115-28. [PubMed ID: 29137838]. https://doi.org/10.1016/j.compmedimag.2017.10.002.
-
27.
Fair DA, Nigg JT, Iyer S, Bathula D, Mills KL, Dosenbach NU, et al. Distinct neural signatures detected for ADHD subtypes after controlling for micro-movements in resting state functional connectivity MRI data. Front Syst Neurosci. 2012;6:80. [PubMed ID: 23382713]. [PubMed Central ID: PMC3563110]. https://doi.org/10.3389/fnsys.2012.00080.
-
28.
Peng X, Lin P, Zhang T, Wang J. Extreme learning machine-based classification of ADHD using brain structural MRI data. PLoS One. 2013;8(11):e79476. [PubMed ID: 24260229]. [PubMed Central ID: PMC3834213]. https://doi.org/10.1371/journal.pone.0079476.
-
29.
Shao L, Xu Y, Fu D. Classification of ADHD with bi-objective optimization. J Biomed Inform. 2018;84:164-70. [PubMed ID: 30009990]. https://doi.org/10.1016/j.jbi.2018.07.011.
-
30.
Zhang X. Introduction to statistical learning theory and support vector machines. Zidonghua Xuebao. 2000;26(1):32-42. Chinese.
-
31.
Cortes C, Vapnik V. Support vector machine. Mach Learn. 1995;20(3):273-97. https://doi.org/10.1023/a:1022627411411.
-
32.
Bledsoe JC, Xiao C, Chaovalitwongse A, Mehta S, Grabowski TJ, Semrud-Clikeman M, et al. Diagnostic Classification of ADHD Versus Control: Support Vector Machine Classification Using Brief Neuropsychological Assessment. J Atten Disord. 2020;24(11):1547-56. [PubMed ID: 27231214]. https://doi.org/10.1177/1087054716649666.
-
33.
Marron JS. Distance-weighted discrimination. Wiley Interdiscip Rev Comput Stat. 2015;7(2):109-14. https://doi.org/10.1002/wics.1345.
-
34.
Shen L, Yin Q. Data maximum dispersion classifier in projection space for high-dimension low-sample-size problems. Knowl Based Syst. 2020;193:105420. https://doi.org/10.1016/j.knosys.2019.105420.
-
35.
Wang JB, Zheng LJ, Cao QJ, Wang YF, Sun L, Zang YF, et al. Inconsistency in Abnormal Brain Activity across Cohorts of ADHD-200 in Children with Attention Deficit Hyperactivity Disorder. Front Neurosci. 2017;11:320. [PubMed ID: 28634439]. [PubMed Central ID: PMC5459906]. https://doi.org/10.3389/fnins.2017.00320.
-
36.
The ADHD-200 Consortium. The ADHD-200 Consortium: A Model to Advance the Translational Potential of Neuroimaging in Clinical Neuroscience. Front Syst Neurosci. 2012;6:62. [PubMed ID: 22973200]. [PubMed Central ID: PMC3433679]. https://doi.org/10.3389/fnsys.2012.00062.
-
37.
Youden WJ. Index for rating diagnostic tests. Cancer. 1950;3(1):32-5. [PubMed ID: 15405679]. https://doi.org/10.1002/1097-0142(1950)3:1<32::aid-cncr2820030106>3.0.co;2-3.
-
38.
Ghiassian S, Greiner R, Jin P, Brown MR. Using Functional or Structural Magnetic Resonance Images and Personal Characteristic Data to Identify ADHD and Autism. PLoS One. 2016;11(12):e0166934. [PubMed ID: 28030565]. [PubMed Central ID: PMC5193362]. https://doi.org/10.1371/journal.pone.0166934.
-
39.
Hart H, Chantiluke K, Cubillo AI, Smith AB, Simmons A, Brammer MJ, et al. Pattern classification of response inhibition in ADHD: toward the development of neurobiological markers for ADHD. Hum Brain Mapp. 2014;35(7):3083-94. [PubMed ID: 24123508]. [PubMed Central ID: PMC4190683]. https://doi.org/10.1002/hbm.22386.
-
40.
Rosa MJ, Portugal L, Hahn T, Fallgatter AJ, Garrido MI, Shawe-Taylor J, et al. Sparse network-based models for patient classification using fMRI. Neuroimage. 2015;105:493-506. [PubMed ID: 25463459]. [PubMed Central ID: PMC4275574]. https://doi.org/10.1016/j.neuroimage.2014.11.021.
-
41.
Pastor P, Reuben C, Duran C, Hawkins L. Association between diagnosed ADHD and selected characteristics among children aged 4-17 years: United States, 2011-2013. NCHS Data Brief. 2015;(201):201. [PubMed ID: 25974000].
-
42.
Ghanizadeh A. Lack of association of handedness with inattention and hyperactivity symptoms in ADHD. J Atten Disord. 2013;17(4):302-7. [PubMed ID: 22286110]. https://doi.org/10.1177/1087054711429789.
-
43.
Siddiqi SU, Giordano BP. Left-handedness in children with neurodevelopmental disorders. Intern Med Rev. 2018;4(1):1-10.
-
44.
Schmidt SL, do Nascimento Simões E, Schmidt GJ, Carvalho ALN, Carvalho ALN. The Effects of Hand Preference on Attention. Psychology. 2013;4(10):29-33. https://doi.org/10.4236/psych.2013.410A006.
-
45.
Cheng W, Ji X, Zhang J, Feng J. Individual classification of ADHD patients by integrating multiscale neuroimaging markers and advanced pattern recognition techniques. Front Syst Neurosci. 2012;6:58. [PubMed ID: 22888314]. [PubMed Central ID: PMC3412279]. https://doi.org/10.3389/fnsys.2012.00058.
-
46.
Greven CU, Bralten J, Mennes M, O'Dwyer L, van Hulzen KJ, Rommelse N, et al. Developmentally stable whole-brain volume reductions and developmentally sensitive caudate and putamen volume alterations in those with attention-deficit/hyperactivity disorder and their unaffected siblings. JAMA Psychiatry. 2015;72(5):490-9. [PubMed ID: 25785435]. https://doi.org/10.1001/jamapsychiatry.2014.3162.
-
47.
Max JE, Fox PT, Lancaster JL, Kochunov P, Mathews K, Manes FF, et al. Putamen lesions and the development of attention-deficit/hyperactivity symptomatology. J Am Acad Child Adolesc Psychiatry. 2002;41(5):563-71. [PubMed ID: 12014789]. https://doi.org/10.1097/00004583-200205000-00014.
-
48.
Wellington TM, Semrud-Clikeman M, Gregory AL, Murphy JM, Lancaster JL. Magnetic resonance imaging volumetric analysis of the putamen in children with ADHD: combined type versus control. J Atten Disord. 2006;10(2):171-80. [PubMed ID: 17085627]. https://doi.org/10.1177/1087054705284242.
-
49.
Tang X, Seymour KE, Crocetti D, Miller MI, Mostofsky SH, Rosch KS. Response control correlates of anomalous basal ganglia morphology in boys, but not girls, with attention-deficit/hyperactivity disorder. Behav Brain Res. 2019;367:117-27. [PubMed ID: 30914308]. [PubMed Central ID: PMC6520987]. https://doi.org/10.1016/j.bbr.2019.03.036.
-
50.
Sanefuji M, Craig M, Parlatini V, Mehta MA, Murphy DG, Catani M, et al. Double-dissociation between the mechanism leading to impulsivity and inattention in Attention Deficit Hyperactivity Disorder: A resting-state functional connectivity study. Cortex. 2017;86:290-302. [PubMed ID: 27394716]. https://doi.org/10.1016/j.cortex.2016.06.005.
-
51.
Mostert JC, Shumskaya E, Mennes M, Onnink AM, Hoogman M, Kan CC, et al. Characterising resting-state functional connectivity in a large sample of adults with ADHD. Prog Neuropsychopharmacol Biol Psychiatry. 2016;67:82-91. [PubMed ID: 26825495]. [PubMed Central ID: PMC4788977]. https://doi.org/10.1016/j.pnpbp.2016.01.011.
-
52.
Kurth F, Zilles K, Fox PT, Laird AR, Eickhoff SB. A link between the systems: functional differentiation and integration within the human insula revealed by meta-analysis. Brain Struct Funct. 2010;214(5-6):519-34. [PubMed ID: 20512376]. [PubMed Central ID: PMC4801482]. https://doi.org/10.1007/s00429-010-0255-z.
-
53.
Anderson AJ, Ren P, Baran TM, Zhang Z, Lin F. Insula and putamen centered functional connectivity networks reflect healthy agers' subjective experience of cognitive fatigue in multiple tasks. Cortex. 2019;119:428-40. [PubMed ID: 31499435]. [PubMed Central ID: PMC6783365]. https://doi.org/10.1016/j.cortex.2019.07.019.
-
54.
Yu W, Krook-Magnuson E. Cognitive Collaborations: Bidirectional Functional Connectivity Between the Cerebellum and the Hippocampus. Front Syst Neurosci. 2015;9:177. [PubMed ID: 26732845]. [PubMed Central ID: PMC4686701]. https://doi.org/10.3389/fnsys.2015.00177.
-
55.
Arrigo A, Mormina E, Anastasi GP, Gaeta M, Calamuneri A, Quartarone A, et al. Constrained spherical deconvolution analysis of the limbic network in human, with emphasis on a direct cerebello-limbic pathway. Front Hum Neurosci. 2014;8:987. [PubMed ID: 25538606]. [PubMed Central ID: PMC4259125]. https://doi.org/10.3389/fnhum.2014.00987.
-
56.
Luo X, Guo J, Liu L, Zhao X, Li D, Li H, et al. The neural correlations of spatial attention and working memory deficits in adults with ADHD. Neuroimage Clin. 2019;22:101728. [PubMed ID: 30822718]. [PubMed Central ID: PMC6396015]. https://doi.org/10.1016/j.nicl.2019.101728.