Abstract
Background:
Differentiating types of brain tumors using magnetic resonance imaging (MRI) as a non-invasive element is essential.Objectives:
The study aimed at evaluating the difference in the first-order histogram (FOH) features obtained from apparent diffusion coefficients (ADCs) between glioblastoma (GBM) tumors with or without oligodendroglial (ODG) components.Methods:
MRIs were taken before surgical resection of the brain tumors. The brain lesions were analyzed and ADC maps were plotted to calculate FOH. Brain lesions were surgically removed, diagnosed using pathological methods, and categorized as GBM with an ODG (GBM-ODG) or without ODG. A comparison of ADC values was performed between GBM-ODG and GBM. Receiver-operator characteristic (ROC) curves were used to determine the cut-off sensitivity and specificity values.Results:
In the GBM group, 26 patients were included; 20 cases were male and 6 were female; the mean ± SD of age was 54.1 ± 1.2 and 55.2 ± 1.6 years, respectively. In the GBM-ODG group, 31 patients were included; 22 cases were male and 9 cases were female; the mean ± SD of 52.1 ± 0.8 and 55.3 ± 1.5 years, respectively. The results of peritumoral edema had several significant FOHs. In the MRIs of tumor and T1W groups, the third moment and minimum-ADC had significant results, respectively. Cut-off values of the FOHs were statistically significant outcomes in the peritumoral edema region when compared between GBM-ODG and GBM categories: Median > 1.3516, normal mean > 0.6671, third moment ≤ -0.0001, 25 percentile > 0.5929, 75 percentile > 0.7336, and 95 percentile > 0.8542. The highest sensitivity and specificity values for these FOHs were higher than 80% and sometimes near 99.9%.Conclusions:
The results showed that ADC values from peritumoral edema regions differ between these two types of tumors. Then, an MRI from the edematous areas is suggested when evaluating the patient's status and deciding to make interventions.Keywords
Apparent Diffusion Coefficient Differential Diagnosis Edema Glioblastoma Oligodendroglia
1. Background
When selecting proper interventional approaches, distinguishing the brain’s tumor types is essential for clinicians. Radiological methods and indices may play a good role in this area of diagnosis, as mentioned in a few previous published articles (1-3). These methods are necessary, especially because of relieving neurosurgeons to make operations only for diagnosis for curing, lowering the risk of interventions (4). GBM differential diagnosis using radiological evaluations is essential because of its high prevalence among adults (5). Oligodendrocytes produce the myelin material supporting the axons of the neuronal cells; with myelin's help, electrical conductivity is facilitated in the central nervous system (6). Oligodendroglial tumors develop when the oligodendrocytes become malignant. The ODG may be detectable using diffusion-weighed imaging (DWI) (7). The ADC, calculated for DWIs, has been mentioned to help diagnose some brain tumors (7-10). In addition to the images prepared from MRI from tumoral regions, with or without enhancing material, the photos from peritumoral edema have been mentioned to be worth diagnosing brain lesions and tumors (8, 11, 12). However, reports are rare in comparing ADC values among MRIs from oligodendroglial tumors without enhancement and images from edematous regions.
This study hypothesized that as the ADC values obtained from images relating to the water diffusion in the brain environment, each tumor and its environment could have its specific behavior; this behavior may help differentiate the GBM from GBMs with ODG.
2. Objectives
The present study aimed at comparing the FOHs, from ADC statistical values between GBM tumors and GBMs containing ODG components (GBM-ODG). The study purposed to analyze the MRIs from flair images of tumors, gadopentetate dimeglumine-enhanced pictures of tumors, and MRIs from edematous regions. Also, the study aimed at assessing some cut-off values for the FOH feature that could be valuable for the clinical radiologist, who reports information such as the tumor type, GBM, or GMB-ODG to a neurosurgeon.
3. Methods
3.1. Studied Groups
The Institutional Review Board approved this study. According to the surgical pathology results and three radiologic patterns, two types of malignancies were studied: (1) Flair images from tumoral regions in GBM and GBM-ODG groups (tumor group); (2) gadopentetate dimeglumine enhanced T1-weighted MRI images in GBM and GBM-ODG groups (T1W+GAD group); and (3) MRIs from peritumoral edematous regions in GBM and GBM-ODG groups (edema group). In total, 134 MRIs were studied, 57 of which were in the tumor group (GBM = 26 and GBM-ODG = 31 MRIs), 50 were in the T1W+GAD group (GBM = 26 and GBM-ODG = 24 MRIs), and 27 MRIs were in the edema group (GBM = 10 and GBM-ODG = 17 MRIs).
3.2. Magnetic Resonance Imaging
MRI (magnetic resonance imaging) was done, using a 1.5 Tesla machine (Siemens®, Avanto, Rel 16.0). Contrast-enhanced images were taken after administration of gadopentetate dimeglumine intravenously and with a concentration of 0.1 mmol/Kg of body weight. In 3 orthogonal directions, diffusion-weighted photos of the axial plan were taken, using the T2-weighted protocol. A highly experienced radiologist reviewed MRIs, and regions of interest (ROI) were selected for ADC statistical features calculations. As the control ADC values, the radiologist determined the white matter or contralateral hemisphere with average views (Figure 1).
Schematic presentation represents 3 types of MRI evaluation. Left: Tumor and edema group MRI without contrast. Right: Contrast-enhanced imaging performed at T1 weighted imaging.
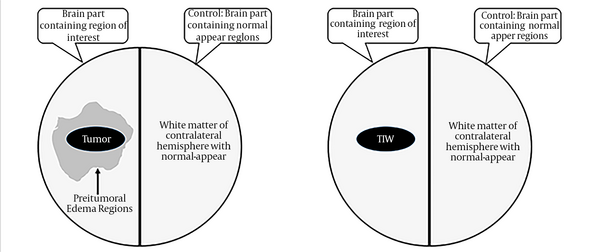
3.3. MRI Analysis and First-Order Histogram Features
FOHs (first-order histogram) were calculated from ADC values; for this purpose, the pixel in the selected ROIs was considered independently from neighbor ones. ROIs were chosen via the manual method, and each pixel's gray level within ROIs was the base of ADC statistical calculations. In the contrast-enhanced T1W and spin-echo images, the margin of tumors and enhanced regions were manually selected. The experienced radiologist was blinded to the patient’s identities, surgical pathology results, and the purpose of the study. Edematous areas also were included in the delineation selection and determining ROIs in each slice.
FMRIB Software Library v5.0 was used for similarity measure analysis of ADC maps. The ADC maps were submitted digitally to the picture archiving and communication systems (PACS) database in medicine. The image analysis was done, using ImageJ offline software accessible on the URL: https://rsb.info.nih.gov/ij/. FOH features of ADC values included mean, maximum, minimum, median, normal mean, standard deviation, smoothness, third moment, uniformity, entropy, kurtosis, 25 percentile, 75 percentile, and 95 percentile.
3.4. Surgical Pathology Differential Diagnosis
MRIs were taken before surgery for each patient. To differentiate GBM-ODG from GBM, the lesion was removed from the brain by a neurosurgeon and sent to the pathology lab. The specimens were fixed in formalin. Tissue processing was performed, paraffin-embedded blocks were prepared, sectioning with microtome was done, and the tissues were stained by hematoxylin and eosin (H&E) method. A high-experienced pathologist determined the types of each lesion. In the current report, only GBM tumors with or without ODG components have been compared, as presented in the results.
3.5. Sample Size
Using G*Power version 3.1.9.7 and considering significant level 0.05 and power 80% for comparison, and the standard effect size 0.8 for comparison of two independent groups, 21 cases were determined for assessment. Also, considering the mean of ADC (2.3 ± 1 VS. 1.7 ± 0.7) in Zali et al.’s study, the effect size calculated was estimated at 0.7, and the sample size was estimated at 26 cases. In this study, in the GBM group, 26 patients were included in the GBM-ODG group, 31 patients were included.
3.6. Statistical Analysis
MedCalc Statistical Software version 15.8 (MedCalc Software bvba, Ostend, Belgium; https://www.medcalc.org; 2015) was used for statistical analysis, and each FOH feature was calculated and reported for MRIs of the tumor, T1W+GAD, and edema groups. The normal distribution of each variable was assessed, using the Shapiro-Wilk test and ANOVA test, Kruskal Wallis, two independent samples t-test, or Mann-Whitney test performed for comparisons between GBM and GBM-ODG categories. Further, receiver operating characteristic (ROC) curves were plotted only for the edema group, with some significant results. P-values less than 0.05 were considered statistically significant when comparing GBM with GBM-ODG tumors.
4. Results
In the GBM group, 26 patients were included; 20 cases were male and 6 were female; the mean ± SD of age was 54.1 ± 1.2 and 55.2 ± 1.6 years, respectively. In the GBM-ODG group, 31 patients were included; 22 cases were male and 9 were female; the mean ± SD of age was 52.1 ± 0.8 and 55.3 ± 1.5 years, respectively.
Table 1 shows the FOH features in the tumor group. In Table 1, ADC values have been compared between GBM-ODG and GBM, according to the surgical pathology diagnosis. ADC values of total components were also shown. No one of the FOH features had significant results when comparing GBM-ODG and GBM, except the FOH, which features the third moment that is differences between two groups (CI = 0.95; P-value = 0.014). This result showed that when the glioblastoma tumor region has an oligodendroglial (ODG) component, the third moment will significantly differ from glioblastomas without ODGs.
First-Order Histogram Features of Tumor Group: Comparison of ADC Values Between GBM-ODG and GBM According to the Surgical Pathology Diagnosis. ADC Values in Total Components are Also Shown
FOH Features | ADCs a for GBM-ODG (N = 31) | ADCs a for GBM (N = 26) | ADCs a in Total (N = 57) | P-Value | |||
---|---|---|---|---|---|---|---|
Mean ± SD | Median (Minimum; Maximum) | Mean ± SD | Median (Minimum; Maximum) | Mean ± SD | Median (Minimum; Maximum) | ||
Mean | 1.234 ± 0.353 | 1.231 (0.111; 1.905) | 1.351 ± 0.460 | 1.188 (0.669; 2.554) | 1.288 ± 0.406 | 1.201 (0.111; 2.554) | 0.798 |
Maximum | 2.259 ± 0.663 | 2.205 (0.228; 3.454) | 2.290 ± 0.746 | 2.131 (1.161; 3.794) | 2.273 ± 0.696 | 2.153 (0.228; 3.794) | 0.867 b |
Minimum | 0.593 ± 0.202 | 0.556 (0.078; 0.994) | 0.686 ± 0.251 | 0.631 (0.334; 1.407) | 0.635 ± 0.228 | 0.614 (0.078; 1.407) | 0.126 b |
Median | 1.185 ± 0.353 | 1.172 (0.107; 1.920) | 1.326 ± 0.486 | 1.160 (0.659; 2.603) | 1.249 ± 0.421 | 1.168 (0.107; 2.603) | 0.689 |
Normal mean | 0.543 ± 0.089 | 0.535 (0.397; 0.746) | 0.587 ± 0.100 | 0.580 (0.359; 0.777) | 0.563 ± 0.096 | 0.552 (0.359; 0.777) | 0.086 b |
SD | 0.131 ± 0.028 | 0.130 (0.076; 0.197) | 0.123 ± 0.039 | 0.109 (0.081; 0.217) | 0.127 ± 0.033 | 0.123 (0.076; 0.217) | 0.107 |
Smoothness | 13.5×10 -9 ± 32.5×10 -9 | 81.2×10-11 (11.3×10-11; 14.8×10-8) | 98.7984×10 -10 ± 16.722112×10 -9 | 17.9378×10-10 (86×10-11; 671.70×10-10) | 118.5144×10 -10 ± 263.65655×10 -10 | 15.5879×10-10 (8.6×10-11; 1478.47×10-10) | 0.287 |
Third moment | 0.002 ± 0.001 c | 0.001 (-0.002; 0.004) c | 0.001 ± 0.002 c | 0.001 (-0.003; 0.009) c | 0.001 ± 0.002 c | 0.001 (-0.003; 0.009) c | 0.014 c |
Uniformity | 1488.795 ± 1084.923 | 1439.856 (185.397; 4152.125) | 1425.414 ± 1115.675 | 1153.173 (199.925; 4386.708) | 1459.885 ± 1089.616 | 1245.590 (185.397; 4386.708) | 0.730 |
Entropy | 6.741 ± 0.325 | 6.700 (6.002; 7.461) | 6.642 ± 0.338 | 6.696 (6.002; 7.269) | 6.696 ± 0.331 | 6.699 (6.002; 7.461) | 0.266 b |
Kurtosis | 4.177 ± 2.210 | 3.186 (2.033; 10.946) | 4.175 ± 2.235 | 3.467 (1.924; 10.691) | 4.176 ± 2.201 | 3.361 (1.924; 10.946) | 0.743 |
25 Percentile | 0.448 ± 0.091 | 0.427 (0.300; 0.681) | 0.498 ± 0.117 | 0.495 (0.231; 0.719) | 0.471 ± 0.106 | 0.462 (0.231; 0.719) | 0.073 b |
75 Percentile | 0.621 ± 0.102 | 0.622 (0.449; 0.813) | 0.669 ± 0.109 | 0.686 (0.433; 0.849) | 0.643 ± 0.107 | 0.649 (0.433; 0.849) | 0.096 b |
95 Percentile | 0.788 ± 0.093 | 0.806 (0.574; 0.936) | 0.797 ± 0.084 | 0.812 (0.622; 0.935) | 0.792 ± 0.088 | 0.807 (0.574; 0.936) | 0.719 b |
Table 2 shows the FOH features of the MRIs taken after enhancement agent administration (T1W group). The only significant FOH statistic is the Minimum value of ADC; for this index, the GBM-ODG group has fewer values than GBMs without oligodendroglia components (CI = 0.95; P-value = 0.018).
First-Order Histogram Features of T1W Group: Comparison of ADC Values Between GBM-ODG and GBM According to the Surgical Pathology Diagnosis. ADC Values in Total Components Also are Shown
FOH Features | ADCs a for GBM-ODG (N = 26) | ADCs a for GBM (N = 24) | ADCs a in Total (N = 50) | P-Value | |||
---|---|---|---|---|---|---|---|
Mean ± SD | Median (Minimum; Maximum) | Mean ± SD | Median (Minimum; Maximum) | Mean ± SD | Median (Minimum; Maximum) | ||
Mean | 1.186 ± 0.357 | 1.203 (0.112; 2.038) | 1.392 ± 0.404 | 1.288 (0.927; 2.318) | 1.285 ± 0.391 | 1.244 (0.112; 2.318) | 0.151 |
Maximum | 2.129 ± 0.566 | 2.153 (0.256; 3.275) | 2.352 ± 0.656 | 2.267 (1.500; 3.784) | 2.236 ± 0.615 | 2.204 (0.256; 3.784) | 0.472 |
Minimum | 0.584 ± 0.183 b | 0.591 (0.080; 0.966) b | 0.734 ± 0.248 b | 0.702 (0.374; 1.592) b | 0.656 ± 0.227 b | 0.671 (0.080; 1.592) b | 0.018 b,c |
Median | 1.155 ± 0.373 | 1.136 (0.106; 2.158) | 1.378 ± 0.456 | 1.248 (0.880; 2.412) | 1.262 ± 0.426 | 1.188 (0.106; 2.412) | |
Normal mean | 0.546 ± 0.099 | 0.537 (0.382; 0.721) | 0.592 ± 0.112 | 0.583 (0.382; 0.888) | 0.568 ± 0.107 | 0.571 (0.382; 0.888) | 0.137 c |
Standard deviation | 0.119 ± 0.019 | 0.115 (0.081; 0.150) | 0.124 ± 0.040 | 0.113 (0.074; 0.255) | 0.121 ± 0.031 | 0.115 (0.074; 0.255) | 0.634 |
Smoothness | 731.60×10 -10 ± 254.631×10 -9 | 160.0659×10-10 (6.85×10-10; 13151.96×10-10) | 264.15142×10 -9 ± 107.91×10 -8 | 218.2458×10-10 (7.42×10-10; 53202.34×10-10) | 1648.3565×10 -10 ± 7674.272×10 -10 | 184.4799×10 -10 (6.85×10 -10; 53202.34×10 -10) | 0.528 |
Third moment | 0.001 ± 0.001 | 0.001 (-0.004; 0.004) | 0.001 ± 0.003 | 0.001 (-0.006; 0.015) | 0.001 ± 0.003 | 0.001 (-0.006; 0.015) | 0.086 |
Uniformity | 500.572 ± 364.928 | 431.151 (54.836; 1907.015) | 498.858 ± 419.333 | 316.356 (37.249; 1697.927) | 499.750 ± 387.922 | 408.917 (37.249; 1907.015) | 0.567 |
Entropy | 6.536 ± 0.294 | 6.622 (5.838; 6.941) | 6.503 ± 0.521 | 6.556 (4.888; 7.342) | 6.520 ± 0.414 | 6.593 (4.888; 7.342) | 0.946 |
Kurtosis | 5.078 ± 2.558 | 3.962 (2.759; 13.519) | 4.451 ± 2.874 | 3.677 (2.084; 13.519) | 4.777 ± 2.705 | 3.802 (2.084; 13.519) | 0.203 |
25 Percentile | 0.464 ± 0.098 | 0.447 (0.276; 0.642) | 0.503 ± 0.126 | 0.483 (0.203; 0.836) | 0.483 ± 0.113 | 0.476 (0.203; 0.836) | 0.216 c |
75 Percentile | 0.612 ± 0.112 | 0.619 (0.411; 0.830) | 0.666 ± 0.119 | 0.658 (0.411; 0.959) | 0.638 ± 0.118 | 0.634 (0.411; 0.959) | 0.105 c |
95 Percentile | 0.768 ± 0.098 | 0.804 (0.565; 0.896) | 0.807 ± 0.093 | 0.821 (0.592; 0.992) | 0.787 ± 0.097 | 0.809 (0.565; 0.992) | 0.157 c |
Table 3 shows the FOH features of the MRIs taken from the peritumoral edematous regions (edema group). The comparison showed that median (GBM-ODG vs. GBM Medians = 1.489 vs. 1.323), normal mean (GBM-ODG vs. GBM Medians = 0.758 vs. 0.633), third moment (GBM-ODG vs. GBM Medians = -0.001 vs. 0.000), 25 percentile (GBM-ODG vs. GBM Medians = 0.688 vs. 0.560), 75 percentile (GBM-ODG vs. GBM Medians = 0.832 vs. 0.720) and 95 percentile (GBM-ODG vs. GBM Medians = 0.901 vs. 0.837) were significant when comparing GBM-ODG with GBMs without ODG components. Except for the third moment, the other meaningful FOH features had higher values in the GBM-ODG category than GBM.
First-Order Histogram Features of Edema Group: Comparison of ADC Values Between GBM-ODG and GBM According to the Surgical Pathology Diagnosis. ADC Values in Total Components Also are Shown
FOH Features | ADCs a for GBM-ODG (N = 17) | ADCs a for GBM (N = 10) | ADCs a in Total (N = 27) | P-Value | |||
---|---|---|---|---|---|---|---|
Mean ± SD | Median (Minimum; Maximum) | Mean ± SD | Median (Minimum; Maximum) | Mean ± SD | Median (Minimum; Maximum) | ||
Mean | 1.424 ± 0.349 | 1.494 (0.125; 1.674) | 1.402 ± 0.269 | 1.315 (1.144; 2.076) | 1.416 ± 0.316 | 1.454 (0.125; 2.076) | 0.066 |
Maximum | 1.911 ± 0.501 | 2.018 (0.170; 2.588) | 2.205 ± 0.633 | 2.173 (1.446; 3.581) | 2.020 ± 0.560 | 2.060 (0.170; 3.581) | 0.309 |
Minimum | 0.755 ± 0.273 | 0.892 (0.079; 1.053) | 0.712 ± 0.207 | 0.733 (0.279; 1.062) | 0.739 ± 0.247 | 0.815 (0.079; 1.062) | 0.243 |
Median | 1.443 ± 0.354 b | 1.489 (0.125; 1.687) b | 1.390 ± 0.278 b | 1.323 (1.128; 2.107) b | 1.423 ± 0.324 | 1.474 (0.125; 2.107) | 0.018 b |
Normal mean | 0.750 ± 0.065 b | 0.758 (0.562; 0.841) b | 0.627 ± 0.122 b | 0.633 (0.364; 0.770) b | 0.704 ± 0.106 | 0.736 (0.364; 0.841) | 0.04 b |
SD | 0.101 ± 0.018 | 0.099 (0.073; 0.154) | 0.112 ± 0.026 | 0.109 (0.069; 0.159) | 0.105 ± 0.021 | 0.102 (0.069; 0.159) | 0.243 |
Smoothness | 37×10 -10 ± 84×10 -10 | 11×10-10 (2×10-10; 357×10-10) | 45×10 -10 ± 110×10 -10 | 9×10-10 (0; 357×10-10) | 40×10 -10 ± 93×10 -10 | 10×10-10 (0; 357×10-10) | 0.570 |
Third moment | -0.001 ± 0.002 b | -0.001 (-0.006; 0.000) b | 0.001 ± 0.002 b | 0.000 (-0.001; 0.007) b | 0.000 | 0.000 (-0.006; 0.007) | 0.003 b |
Uniformity | 2085.458 ± 971.932 | 2245.070 (270.412; 3833.182) | 2426.117 ± 1721.120 | 2005.982 (270.412; 5593.440) | 2211.628 ± 1278.602 | 2168.382 (270.412; 5593.440) | 0.824 |
Entropy | 6.519 ± 0.205 | 6.521 (6.132; 6.868) | 6.640 ± 0.262 | 6.683 (6.115; 6.971) | 6.564 ± 0.231 | 6.528 (6.115; 6.971) | 0.170 |
Kurtosis | 3.525 ± 1.064 | 3.389 (2.291; 6.949) | 3.541 ± 1.127 | 3.110 (2.700; 5.732) | 3.531 ± 1.066 | 3.243 (2.291; 6.949) | 0.675 |
25 Percentile | 0.691 ± 0.069 b | 0.688 (0.500; 0.797) b | 0.552 ± 0.137 b | 0.560 (0.254; 0.722) b | 0.639 ± 0.119 | 0.681 (0.254; 0.797) | 0.003 b |
75 Percentile | 0.820 ± 0.064 b | 0.832 (0.638; 0.900) b | 0.699 ± 0.123 b | 0.720 (0.428; 0.836) b | 0.775 ± 0.106 | 0.806 (0.428; 0.900) | 0.002 b |
95 Percentile | 0.896 ± 0.059 b | 0.901 (0.718; 0.950) b | 0.820 ± 0.076 b | 0.837 (0.694; 0.915) b | 0.868 ± 0.074 | 0.881 (0.694; 0.950) | 0.005 b |
Table 4 consists only of the results of the Edematous group, which had more significant results than the Tumor and T1W+GAD groups. Table 4 shows that some cut-off values may be necessary for differential diagnosis of glioblastoma with an oligodendroglial component from a glioblastoma tumor without such features.
Receiver Operating Characteristic Analysis Results for FOH Features of Edematous Regions Images: Bolded Rows Have Significant Results and are Essential in the Differential Diagnosis of GBM-ODG from GBM When Considering Cut-off Values a
FOH Features | AUC, Mean ± SE | 95% CI | P-Value | Criterion (Cut-off) (×10-3 mm2/s) | Sensitivity% (95%CI) | Specificity% (95% CI) |
---|---|---|---|---|---|---|
Mean | 0.718 ± 0.117 | (0.513 to 0.873) | 0.0630 | > 1.3274 | 88.24 (63.6 - 98.5) | 60 (26.2 - 87.8) |
Maximum | 0.624 ± 0.136 | (0.418, 0.801) | 0.3637 | ≤ 2.169 | 88.24 (63.6 – 98.5) | 60 (26.2 -87.8) |
Minimum | 0.641 ± 0.118 | 0.435 to 0.815 | 0.2325 | > 0.8153 | 64.71 (38.3 - 85.8) | 80 (44.4 - 97.5) |
Median | 0.776 ± 0.112 b | 0.576 to 0.913 b | 0.0134 b | > 1.3516 b | 88.24 (63.6 - 98.5) b | 70 (34.8 - 93.3) b |
Normal mean | 0.829 ± 0.0863 b | 0.636 to 0.946 b | 0.0001 b | > 0.6671 b | 94.12 (71.3 - 99.9) b | 70 (34.8 - 93.3) b |
SD | 0.635 ± 0.126 | 0.429 to 0.810 | 0.2813 | ≤ 0.1124 | 88.24 (63.6 - 98.5) | 50 (18.7 - 81.3) |
Smoothness | 0.571 ± 0.125 | 0.367 to 0.758 | 0.5713 | > 1.48629E-009 | 47.06 (23.0 - 72.2) | 80 (44.4 - 97.5) |
Third moment | 0.835 ± 0.0844 b | 0.643 to 0.949 b | 0.0001 b | ≤ -0.0001 b | 70.59 (44.0 - 89.7) b | 90 (55.5 - 99.7) b |
Uniformity | 0.524 ± 0.135 | 0.324 to 0.718 | 0.8618 | ≤ 2887.4672 | 88.24 (63.6 - 98.5) | 40 (12.2 - 73.8) |
Entropy | 0.665 ± 0.124 | 0.458 to 0.833 | 0.1835 | ≤ 6.5428 | 70.59 (44.0 - 89.7) | 70 (34.8 - 93.3) |
kurtosis | 0.547 ± 0.121 | 0.345 to 0.738 | 0.6979 | > 3.2427 | 58.82 (32.9 - 81.6) | 70 (34.8 - 93.3) |
25 Percentile | 0.829 ± 0.0864 b | 0.636 to 0.946 b | 0.0001 b | > 0.5929 b | 94.12 (71.3 - 99.9) b | 70 (34.8 - 93.3) b |
75 Percentile | 0.847 ± 0.0773 b | 0.657 to 0.956 b | < 0.0001 b | > 0.7336 b | 88.24 (63.6 - 98.5) b | 70 (34.8 - 93.3) b |
95 Percentile | 0.821 ± 0.0826 b | 0.626 to 0.940 b | 0.0001 b | > 0.8542 b | 88.24 (63.6 - 98.5) b | 70.00 (34.8 - 93.3) b |
Diagnostically valuable results include the median (AUC = 0.776; P-value = 0.0134) with cut-off >1.3516, the sensitivity = 88.24 (63.6 - 98.5) and specificity = 70 (34.8 - 93.3); normal mean (AUC = 0.829; P-value = 0.0001) with cut-off > 0.6671, the sensitivity = 94.12 (71.3 - 99.9) and specificity = 70 (34.8 - 93.3); third moment (AUC = 0.835; P-value = 0.0001) with cut-off ≤ -0.0001, the sensitivity = 70.59 (44.0 - 89.7) and specificity = 90 (55.5 - 99.7). Further, 25 percentile (AUC = 0.829; P-value = 0.0001) with the cut-off > 0.5929, the sensitivity = 94.12 (71.3 - 99.9) and specificity = 70 (34.8 - 93.3); or 75 percentile (AUC = 0.847; P-value < 0.0001) with the cut-off > 0.7336, the sensitivity = 88.24 (63.6 - 98.5) and specificity = 70 (34.8 - 93.3), and 95 percentile (AUC = 0.821; P-value = 0.0001) with cut-off > 0.8542, the sensitivity = 88.24 (63.6 - 98.5) and specificity = 70 (34.8 - 93.3), were diagnostically valuable (Figure 2).
Receiver operating characteristic (ROC) curves plotted for the edematous group (A to N). The significant results were obtained for median (D), normal mean (E), third moment (H), 25 percentile (L), 75 percentile (M), and 95 percentile (N).
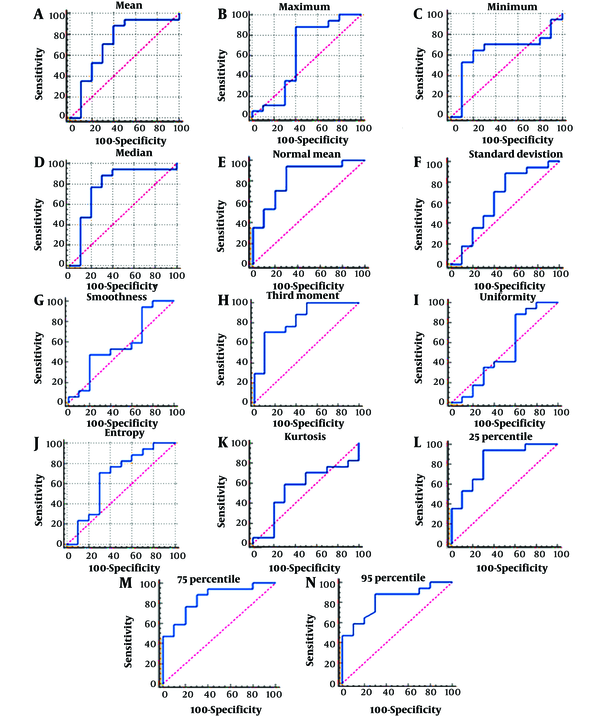
5. Discussion
Oligodendrocytes have a significant role in the rapid action potential transmission by myelination of the neuronal axons in the central nervous system (6). Glioblastomas, the most frequent glial malignancies, are highly heterogeneous phenotypically and may be presented as glial tumors or GBM with oligodendroglial features. Because of the chemo-sensitivity of GBM-ODG, differentiation of this type of tumor from GBM is essential for therapeutic purposes (13). Current diagnostics methods for differential diagnosis of brain tumors rely on surgical biopsy, a highly invasive procedure prone to errors when taking the sample (14). Recently, radiologic diagnostic methods have been prominent due to minimal invasive properties and repeatability without causing irreversible effects.
The present study showed flair or enhanced images, using MRI technique, could not differentiate between GBM-ODG and GBM alone tumors when considering ADC values after obtaining FOH features. However, some of the FOH features obtained from MRIs of edematous regions of the tumoral periphery were competent in distinguishing GBM-ODG from GBM tumors. In addition, the current study reached some cut-off points that could be of choice in clinical decisions. Other studies reported the usability of FOH features in differential diagnosis (15-21). Sometimes and after confirmatory studies, these cut-off points could be regarded as definitive biomarkers, as emphasized by other studies (2, 22).
GBM-ODG is different from GBM when exploring tumor suppressor genes and oncogenes or cytological properties, using molecular and pathological methods, respectively (13). Diagnosis methods based on molecular or cytological properties of the tissues need surgical interventions. However, the results of the current study showed that the less invasive procedure of MRI could effectively distinguish two types of malignancies with at least 70% sensitivity or specificity for FOH feature cut-off points. Lu et al. showed that peritumoral edema ADC statistics are valuable in distinguishing metastatic brain tumors from gliomas. Their results agree with the current study's findings for differentiating GBM-ODG from GBM without ODG components using MRIs from peritumoral regions (11). Also, Lu et al.'s study did not propose any cut-off point for differential diagnosis of edema or tumor types, but we reported some cut-off points, using ROC analysis.
Jenkinson et al. showed that ADC and ADC transition coefficient values of FOH features, the mean and maximum or ATC, significantly differed between oligodendroglial tumors when considering their genotypes but not subtypes or grades (8). However, Jenkinson et al.'s study did not report any cut-off value for differential diagnosis of oligodendroglial tumors, whereas they declare that the outcomes are confirmed, using the molecular method. In the current research, some proper cut-offs for discrimination of GBM-ODG from GBM tumors without ODG components were reported.
Jenkinson et al. (2010) reported that the minimum and maximum ADC values were not different when comparing the cellular density of the tumors, and oligoastrocytomas had lower minimum cell density compared to oligodendrogliomas; also, the cellularity of the tumors using microscopic evaluations was reported (7). This report agrees with the current study's results about the inability of FOH features obtained from MRIs of tumoral regions or MRIs of T1W to distinguish between GBM-ODG and GBM. Despite the effects of the tumor and T1W MRIs, peritumoral MRIs showed FOHs with somewhat valuable cut-offs with good sensitivity and specificity to the differential diagnosis of mentioned malignancies.
The current study confirms the usability of cut-off values in differentiating the tumor types. Chen et al. evaluated the diagnostic value of Minimum ADC values in predicting neuroepithelial tumor grading. They showed that minimum ADC values significantly differed between low-grade and high-grade gliomas. Also, there was a significant difference between minimum ADC values when grading was performed according to the World Health Organization (WHO) criteria. According to the WHO grading system, some cut-off values for discriminating low-grade from high-grade, G2 from G3, and G3 from G4 gliomas were reported (10).
Moreover, Fellah et al. reported that ADC values could distinguish Grade II from Grade III oligodendroglial tumors (23). The current study reported some cut-off values with enough sensitivity and specificity to differentiate GBM-ODG from GBM. However, Fellah et al.'s study showed significant results for the MRIs of tumor tissues. In contrast, the main significant developments in the current research were from the peritumoral edematous regions of the GBM-ODG or GBM tumors. Furthermore, in the present study, 6 FOH features for distinguishing GBM-ODG from GBM were shown; indeed, more diagnostic markers help more precise differential diagnosis, and this aspect of the current research potentiates the results.
Khayal et al. reported that normalized ADC values could distinguish oligodendroglioma from oligoastrocytomas with 91% sensitivity and 92% specificity (14). The results of the current study revealed that there were some FOHs for differential diagnosis of GBM-ODG from GBM with up to 99.9% sensitivity and 99.7% specificity regarding cut-off values. Khayal et al. considered the median criterion <1.8 or >1.8 as the cut-off for distinguishing between oligodendroglioma and oligoastrocytomas or mixed types (14). They relied on the median values of tumors and T1W, but we did not observe good results from these types of MRI; however, the results were considered in the MRI from peritumoral edematous regions. Then, edematous areas of tumors contain valuable and informative data about the type of tumor and contents as we differentiate GBM-ODG from GBM using such information. The logic of such a finding arises from the fact that the cellularity or behavior of tumoral content depends on the cell types that form the tumoral lesion (6, 9, 10, 14, 23, 24).
5.1. Conclusions
The most crucial information that could be conclusive in the current study included the important data obtained from MRIs of the peritumoral edematous regions, but not in tumor or T1W+GAD ones, and compassionate and specific cut-off values for differential diagnosis of GBM-ODG from GBM without ODG components. However, more studies about the importance of peritumoral edemas should be performed in future years to make evidence containing valuable information for neurology professionals, who decide to start the treatment or a neurosurgeon who is preparing to make an operation. However, a comparison between GBM with or without ODG components and other types of brain tumors should be performed to explore the potential usage of FOH statistics values in the differential diagnosis of brain tumors. This report could be considered good evidence for such types of surveys.
References
-
1.
Doskaliyev A, Yamasaki F, Ohtaki M, Kajiwara Y, Takeshima Y, Watanabe Y, et al. Lymphomas and glioblastomas: differences in the apparent diffusion coefficient evaluated with high b-value diffusion-weighted magnetic resonance imaging at 3T. Eur J Radiol. 2012;81(2):339-44. [PubMed ID: 21129872]. https://doi.org/10.1016/j.ejrad.2010.11.005.
-
2.
Aggarwal N, K. Agrawal R. First and second order statistics features for classification of magnetic resonance brain images. J Signal Inf Process. 2012;3(2):146-53. https://doi.org/10.4236/jsip.2012.32019.
-
3.
Romano A, Coppola V, Lombardi M, Lavorato L, Di Stefano D, Caroli E, et al. Predictive role of dynamic contrast enhanced T1-weighted MR sequences in pre-surgical evaluation of macroadenomas consistency. Pituitary. 2017;20(2):201-9. [PubMed ID: 27730456]. https://doi.org/10.1007/s11102-016-0760-z.
-
4.
Yrjänä SK, Tuominen H, Karttunen A, Lähdesluoma N, Heikkinen E, Koivukangas J. Low-field MR imaging of meningiomas including dynamic contrast enhancement study: evaluation of surgical and histopathologic characteristics. AJNR Am J Neuroradiol. 2006;27(10):2128-34. [PubMed ID: 17110681]. [PubMed Central ID: PMC7977226].
-
5.
Stupp R, Mason WP, van den Bent MJ, Weller M, Fisher B, Taphoorn MJ, et al. Radiotherapy plus concomitant and adjuvant temozolomide for glioblastoma. N Engl J Med. 2005;352(10):987-96. [PubMed ID: 15758009]. https://doi.org/10.1056/NEJMoa043330.
-
6.
Ettle B, Schlachetzki JCM, Winkler J. Oligodendroglia and myelin in neurodegenerative diseases: More than just bystanders? Mol Neurobiol. 2016;53(5):3046-62. [PubMed ID: 25966971]. [PubMed Central ID: PMC4902834]. https://doi.org/10.1007/s12035-015-9205-3.
-
7.
Jenkinson MD, du Plessis DG, Smith TS, Brodbelt AR, Joyce KA, Walker C. Cellularity and apparent diffusion coefficient in oligodendroglial tumours characterized by genotype. J Neurooncol. 2010;96(3):385-92. [PubMed ID: 19618117]. https://doi.org/10.1007/s11060-009-9970-9.
-
8.
Jenkinson MD, Smith TS, Brodbelt AR, Joyce KA, Warnke PC, Walker C. Apparent diffusion coefficients in oligodendroglial tumors characterized by genotype. J Magn Reson Imaging. 2007;26(6):1405-12. [PubMed ID: 17968881]. https://doi.org/10.1002/jmri.21062.
-
9.
Chen L, Liu M, Bao J, Xia Y, Zhang J, Zhang L, et al. The correlation between apparent diffusion coefficient and tumor cellularity in patients: a meta-analysis. PLoS One. 2013;8(11). e79008. [PubMed ID: 24244402]. [PubMed Central ID: PMC3823989]. https://doi.org/10.1371/journal.pone.0079008.
-
10.
Chen Z, Ma L, Lou X, Zhou Z. Diagnostic value of minimum apparent diffusion coefficient values in prediction of neuroepithelial tumor grading. J Magn Reson Imaging. 2010;31(6):1331-8. [PubMed ID: 20512884]. https://doi.org/10.1002/jmri.22175.
-
11.
Lu S, Ahn D, Johnson G, Law M, Zagzag D, Grossman RI. Diffusion-tensor MR imaging of intracranial neoplasia and associated peritumoral edema: introduction of the tumor infiltration index. Radiology. 2004;232(1):221-8. [PubMed ID: 15220505]. https://doi.org/10.1148/radiol.2321030653.
-
12.
Beig Zali S, Alinezhad F, Ranjkesh M, Daghighi MH, Poureisa M. Accuracy of apparent diffusion coefficient in differentiation of glioblastoma from metastasis. Neuroradiol J. 2021;34(3):205-12. [PubMed ID: 33417503]. [PubMed Central ID: PMC8165902]. https://doi.org/10.1177/1971400920983678.
-
13.
He J, Mokhtari K, Sanson M, Marie Y, Kujas M, Huguet S, et al. Glioblastomas with an oligodendroglial component: a pathological and molecular study. J Neuropathol Exp Neurol. 2001;60(9):863-71. [PubMed ID: 11556543]. https://doi.org/10.1093/jnen/60.9.863.
-
14.
Khayal IS, Vandenberg SR, Smith KJ, Cloyd CP, Chang SM, Cha S, et al. MRI apparent diffusion coefficient reflects histopathologic subtype, axonal disruption, and tumor fraction in diffuse-type grade II gliomas. Neuro Oncol. 2011;13(11):1192-201. [PubMed ID: 21865401]. [PubMed Central ID: PMC3199150]. https://doi.org/10.1093/neuonc/nor122.
-
15.
Avola D, Cinque L, Placidi G. Customized first and second order statistics based operators to support advanced texture analysis of MRI images. Comput Math Methods Med. 2013;2013:213901. [PubMed ID: 23840276]. [PubMed Central ID: PMC3694383]. https://doi.org/10.1155/2013/213901.
-
16.
Castellani U, Cristani M, Combi C, Murino V, Sbarbati A, Marzola P. Visual MRI: merging information visualization and non-parametric clustering techniques for MRI dataset analysis. Artif Intell Med. 2008;44(3):183-99. [PubMed ID: 18775655]. https://doi.org/10.1016/j.artmed.2008.06.006.
-
17.
Jack CR,Jr, Lowe VJ, Weigand SD, Wiste HJ, Senjem ML, Knopman DS, et al. Serial PIB and MRI in normal, mild cognitive impairment and Alzheimer's disease: implications for sequence of pathological events in Alzheimer's disease. Brain. 2009;132(Pt 5):1355-65. [PubMed ID: 19339253]. [PubMed Central ID: PMC2677798]. https://doi.org/10.1093/brain/awp062.
-
18.
Michopoulou S, Costaridou L, Vlychou M, Speller R, Todd-Pokropek A. Texture-based quantification of lumbar intervertebral disc degeneration from conventional T2-weighted MRI. Acta Radiol. 2011;52(1):91-8. [PubMed ID: 21498333]. https://doi.org/10.1258/ar.2010.100166.
-
19.
Rombouts SA, Barkhof F, Sprenger M, Valk J, Scheltens P. The functional basis of ocular dominance: functional MRI (fMRI) findings. Neurosci Lett. 1996;221(1):1-4. [PubMed ID: 9014166]. https://doi.org/10.1016/s0304-3940(96)13260-2.
-
20.
Tuncbilek N, Karakas HM, Okten OO. Dynamic contrast enhanced MRI in the differential diagnosis of soft tissue tumors. Eur J Radiol. 2005;53(3):500-5. [PubMed ID: 15741025]. https://doi.org/10.1016/j.ejrad.2004.04.012.
-
21.
Kunii N, Abe T, Kawamo M, Tanioka D, Izumiyama H, Moritani T. Rathke's cleft cysts: differentiation from other cystic lesions in the pituitary fossa by use of single-shot fast spin-echo diffusion-weighted MR imaging. Acta Neurochir (Wien). 2007;149(8):759-69. discussion 769. [PubMed ID: 17594050]. https://doi.org/10.1007/s00701-007-1234-x.
-
22.
Prasanna P, Tiwari P, Madabhushi A. Co-occurrence of local anisotropic gradient orientations (CoLIAGe): distinguishing tumor confounders and molecular subtypes on MRI. Med Image Comput Comput Assist Interv. 2014;17(Pt 3):73-80. [PubMed ID: 25320784]. https://doi.org/10.1007/978-3-319-10443-0_10.
-
23.
Fellah S, Caudal D, De Paula AM, Dory-Lautrec P, Figarella-Branger D, Chinot O, et al. Multimodal MR imaging (diffusion, perfusion, and spectroscopy): is it possible to distinguish oligodendroglial tumor grade and 1p/19q codeletion in the pretherapeutic diagnosis? AJNR Am J Neuroradiol. 2013;34(7):1326-33. [PubMed ID: 23221948]. [PubMed Central ID: PMC8051487]. https://doi.org/10.3174/ajnr.A3352.
-
24.
Koral K, Mathis D, Gimi B, Gargan L, Weprin B, Bowers DC, et al. Common pediatric cerebellar tumors: correlation between cell densities and apparent diffusion coefficient metrics. Radiology. 2013;268(2):532-7. [PubMed ID: 23564715]. https://doi.org/10.1148/radiol.13121362.