Abstract
Background:
The radiation resistance process is a major problem in radiotherapy. Proteomics is a useful method to determine the molecular mechanism of biological and medical events. Protein-protein interaction (PPI) network is a suitable method for proteomics data interpretation.Objectives:
Assessment of proteomics data about the radiation resistance process in human cell lines via PPI network analysis is the aim of this study.Methods:
Proteomic data were extracted from literature and the differentially expressed proteins (DEPs) were included in the PPI network via the STRING database by Cytoscape software. The network was analyzed and the central nodes were introduced. The central nodes were assessed via action map analysis and gene ontology enrichment.Results:
Among the 251 queried DEPs, 171 individuals were included in the PPI network. Epidermal growth factor receptor (EGFR), fibronectin (FN1), CD44 antigen (CD44), prostaglandin G/H synthase 2 (PTGS2), CD44 antigen (CD44), prostaglandin G/H synthase 2 (PTGS2), NF-kappa-B inhibitor alpha (NFKBIA), Kelch-like ECH-associated protein 1 (KEAP1), cathepsin D (CTSD), D-3-phosphoglycerate dehydrogenase (PHGD), and 5-nucleotidase (NT5E) were introduced as the critical DEPs. Eight groups of biological terms were attributed to the introduced critical DEPs. EGFR, FN1, CD44, and PTGS2 were discriminated among the critical DEPs as the key dysregulated proteins.Conclusions:
The results indicate that EGFR, FN1, CD44, and PTGS2 are the four essential proteins that are involved in radiation resistance in the radioresistant cells.Keywords
Radiotherapy Radioresistant Network Analysis Proteomics Gene Ontology
1. Background
Radiotherapy is a well-known method against cancer development. The radiation resistance process is a main problem in radiotherapy. There are many investigations about radiation resistance phenomena in the different levels of cellular, tissue, and body. It is attempted to find the molecular mechanism of radiation resistance to elevate the efficacy of radiotherapy (1, 2).
Proteomics as a high throughput method is applied to solve many problems in the medical field. The output of proteomics can be interpreted to provide a perspective of molecular events in the studied samples. Large numbers of dysregulated proteins in proteomics correspond to the targeted genes in the investigated cells, tissue, or body. Experiments are shown that among the results of a proteomics investigation, there are limited numbers of proteins that play a critical role in the evaluated diseases or conditions. These crucial proteins can be considered biomarkers (3-5).
However, fold change is an important index in proteomics, the kind of dysregulated proteins determines the biological effect of protein expression changes. PPI network analysis is a method that is applied widely to discriminate the dysregulated proteins based on the centrality properties of the proteins. There are several centrality properties in PPI network analysis such as degree value, betweenness centrality, closeness centrality, and stress, which are applied to analyze the studied networks (6-8).
Action map analysis is the other tool that can be used to detect regulatory actions between the studied proteins. Different actions such as activation, inhibition, and expression relationships between different proteins can be identified via action map analysis. Action map results are a useful tool to elucidate the important role of the studied proteins (9).
Gene ontology enrichment is the other method that can provide the related molecular function, biological processes, cellular compartments, and biochemical pathways related to the investigated proteins (10).
2. Objectives
In the present study, the introduce dysregulated proteins in the radioresistant human oesophageal adenocarcinoma cells (OE33R) versus the radiosensitive cells OE33 cells (OE33P) are extracted from literature and are analyzed via PPI network analysis, actin map evaluation, and gene ontology assessment to determine the core of the proteins, which are involved in radiation resistance in the cellular level. The results can be used to provide a new perspective on radiation resistance events in radiotherapy.
3. Methods
The data are extracted from the paper about proteomic signatures of radioresistant oesophageal adenocarcinoma, which is published by Marcone et al. (11). In the original source of data, the proteome of the generation of an isogenic cell line model of radioresistant oesophageal adenocarcinoma, the radioresistant cells (OE33R) is compared with the radiosensitive OE33 cells (OE33P). The significant differentially expressed proteins (DEPs) are selected based on a P-value < 0.05. The selected proteins were included in a protein-protein interaction (PPI) network via the STRING database by Cytoscape software. The network was analyzed by the “network analyzer” application of Cytoscape software. The main connected component of the analyzed network was assessed to find the central DEPs; 10% of nodes based on degree value, betweenness centrality, closeness centrality, and stress were selected as central nodes. The common central nodes were identified as the critical central nodes. Regulatory properties between the critical central nodes were evaluated via action map analysis and by the CluePedia application of Cytoscape software. Activation, inhibition, and expression actions were determined. The related biological terms for the critical central nodes were assessed via gene ontology analysis by the ClueGO plug-in of Cytoscape software.
4. Results
The number 251 DEPs were included in the STRING database via Cytoscape software. Among the queried DEGs, 249 individuals were recognized by the STRING database. As it is shown in Figure 1, the PPI network including 64 isolated nodes, 12 paired nodes, a tetrad component, and a main connected component of 171 nodes was constructed. The elements of the main connected component were linked by 349 edges. The main connected component was analyzed (Figure 2) and the central nodes were identified.
PPI network of the recognized DEGs related to the difference between the radiosensitive and radioresistant cells. The 249 nodes are connected by 358 edges.
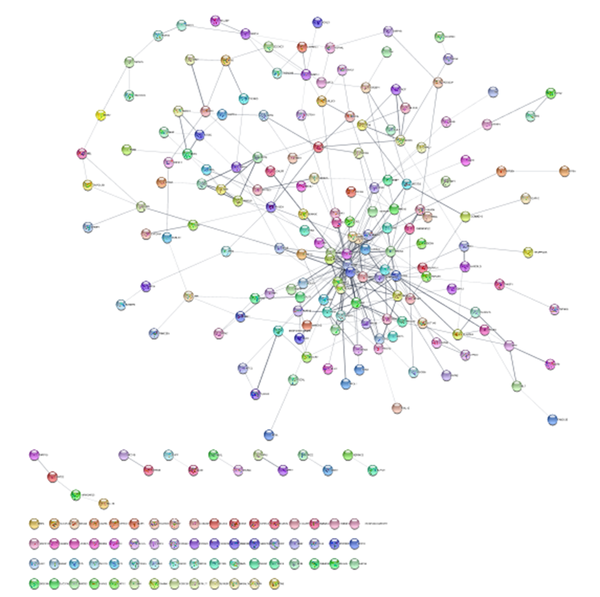
The main connected component of the PPI network of the recognized DEGs is related to the difference between the radiosensitive and radioresistant cells. The 171 nodes are connected by 349 edges. The nodes are layout based on degree value. Red to blue and small to large size refers to an increase in degree value.
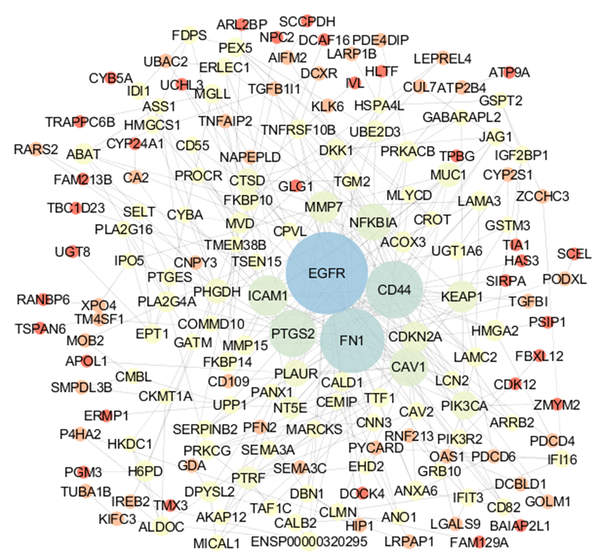
Ten percent of the nodes (17 nodes) as the central nodes based on 4 centrality parameters (degree, betweenness centrality, closeness centrality, and stress) were determined. The total central nodes were 68 nodes, including 9 nodes, which were common among 4 types of central nodes. The 9 common nodes including epidermal growth factor receptor (EGFR), fibronectin (FN1), CD44 antigen (CD44), prostaglandin G/H synthase 2 (PTGS2), CD44 antigen (CD44), prostaglandin G/H synthase 2 (PTGS2), NF-kappa-B inhibitor alpha (NFKBIA), Kelch-like ECH-associated protein 1 (KEAP1), cathepsin D (CTSD), D-3-phosphoglycerate dehydrogenase (PHGD), and 5-nucleotidase (NT5E) were identified as the critical central nodes (Table 1). The rate of expression change for the critical central nodes in the resistant samples versus control is extracted from an investigation of Marcone et al. (11) and shown in Table 2. As it is depicted in Table 2, there are 5 down-regulated and 4 up-regulated critical central DEGs. Connections between the critical central nodes are shown in Figure 3. As it is shown in this sub-network, EGFR is connected to all neighbors directly.
Common Nodes Between the Four Types of the Central Node
N | Display Name | Degree | Betweenness Centrality | Closeness Centrality | Stress |
---|---|---|---|---|---|
1 | EGFR | 44 | 0.38 | 0.429 | 25670 |
2 | FN1 | 32 | 0.235 | 0.401 | 16690 |
3 | CD44 | 27 | 0.119 | 0.376 | 9144 |
4 | PTGS2 | 21 | 0.159 | 0.373 | 13448 |
5 | NFKBIA | 15 | 0.066 | 0.373 | 6196 |
6 | KEAP1 | 12 | 0.039 | 0.331 | 4548 |
7 | CTSD | 8 | 0.052 | 0.333 | 3610 |
8 | PHGDH | 8 | 0.134 | 0.337 | 9726 |
9 | NT5E | 7 | 0.098 | 0.346 | 7236 |
Description, Fold Change, and Dis-regulation for Resistant Samples Versus Control of the Critical Central Nodes. Data are Extracted from the Document of Marcone et al. (11)
N | Gene Name | Description | Dis-regulation | Fold Change |
---|---|---|---|---|
1 | EGFR | Epidermal growth factor receptor | Down-regulation | 0.41 |
2 | FN1 | Fibronectin | Down-regulation | 0.34 |
3 | CD44 | CD44 antigen | Up-regulation | 2.19 |
4 | PTGS2 | Prostaglandin G/H synthase 2 | Up-regulation | 62.87 |
5 | NFKBIA | NF-kappa-B inhibitor alpha | Up-regulation | 6.74 |
6 | KEAP1 | Kelch-like ECH-associated protein 1 | Down-regulation | 0.43 |
7 | CTSD | Cathepsin D | Up-regulation | 2.11 |
8 | PHGDH | D-3-phosphoglycerate dehydrogenase | Down-regulation | 0.01 |
9 | NT5E | 5-nucleotidase | Down-regulation | 0.19 |
The sub-network including the 9 critical central nodes. Red to green and bigger size of nodes refer to increment of degree.
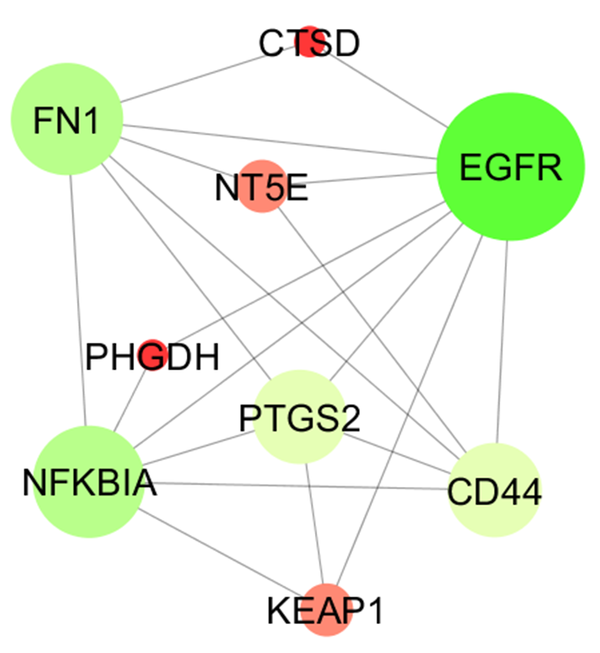
The regulatory relationship between the critical central DEPs is illustrated in Figure 4. Three actions including activation, inhibition, and expression were assessed. As it is shown in Figure 4, EGFR, FN1, CD44, PTGS2, NFKBIA, and NT5E are connected via activation and expression actions. The critical central nodes were enriched via gene ontology analysis. The identified biological terms linked to the related DEPs are shown in Figure 5. Among the queried DEPs, KEAP1 was not connected to the determined biological terms.
Action map including the 9 critical central nodes. Yellow; expression, green; activation.
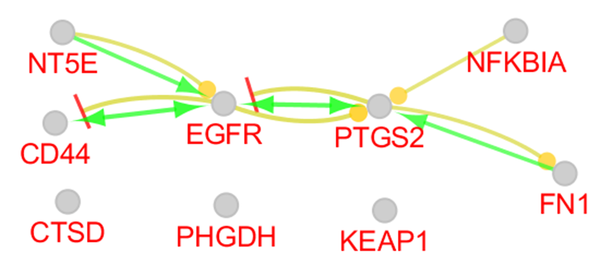
The critical central DEPs and the relative biological terms. KEAP1 was not related to the identified terms. The nodes which are related to the more biological terms are shown.
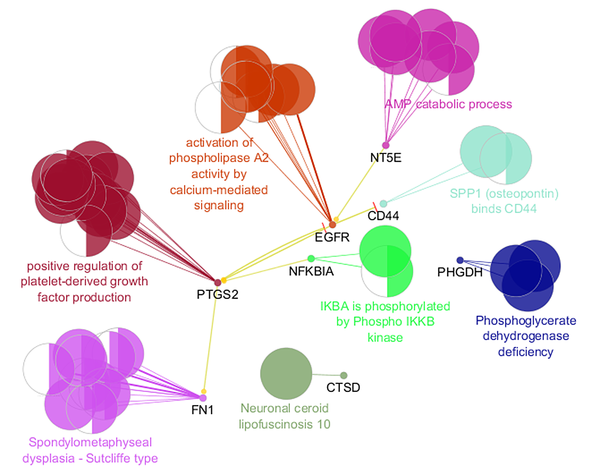
5. Discussion
There are several documents about the application of PPI network analysis of radiation effects on humans. The investigations deal with the molecular mechanism of radiation in the human body and the cultured cells (12, 13). In the original research, from which our data were extracted, alteration in 3 important systems including inflammation, metabolism-related factors, and angiogenesis is assessed in the radio-resistant human cells via proteomic and bioinformatic investigations (11). In the present study, a deep opinion about the central proteins that are involved in radio-resistance phenomena is provided.
PPI network analysis showed that the dis-regulation of EGFR, FN1, CD44, PTGS2, NFKBIA, KEAP1, CTSD, PHGDH, and NT5E is the core of molecular elements, which control radio-resistance events. As it is depicted in Table 1, EGFR is the top protein that plays a role in the analyzed network. Regarding 4 centrality parameters, EGFR is a key central DEP in the resistant cells. EGFR is a top central node and is connected to all critical central nodes, while it is down-regulated in the radio-resistant cells (Table 2 and Figure 3). Chakravarti et al. showed that up-regulation of EGFR is involved in radiation resistance in human gliomas (14). The role of EGFR in radiation resistance is pointed out in many investigations (15, 16). However, the down-regulation of EGFR is reported by the original report, which is in contrast with the literature, the prominent role of EGFR in radiation resistance is emphasized widely. The crucial role of EGFR is highlighted in the action map (Figure 4). Gene ontology analysis revealed that activation of phospholipase-A2 is related to EGFR. Alteration of phospholipase-A2 activity after radiation is investigated in several documents (17, 18).
Fibronectin is the second critical central node, which is ranked as the second crucial DEP based on all centrality parameters. PPI network analysis plus the other analysis pointed out that FN1 can be considered crucial DEP. This protein as like EGFR is down-regulated in radiation-resistant cells. Cordes et al. published research about an increment of resistance to ionizing radiation by fibronectin in human cells (19). The “Spondylometaphyseal dysplasia Sutcliffe type” group of biological terms is related to the FN1 (Figure 5). Gene ontology results indicate that “Spondylometaphyseal dysplasia Sutcliffe type” is the second group of biological terms that includes 20.83% of biological terms.
Prostaglandin G/H synthase 2 (PTGS2) is a critical DEP that is related to the largest group of biological terms. This group “Positive regulation of platelet-derived growth factor production” includes 31.25% of the biological terms. PTGS2 parallel with EGFR plays a significant role in the action map. The importance of PTGS2 in radiation resistance is highlighted by Tan et al. (20). As is shown in Table 2, the fold change of PTGS2 is 62.87 and the protein corresponds to the literature is up-regulated (20).
CD44 is the other critical protein that is up-regulated and appeared as a relatively important dysregulated protein. Zhao et al. investigated the role of CD44 in response to ionizing radiation. In this report, the up-regulation of CD44 by K-RAS in response to radiation is emphasized (21).
NF-kappa-B inhibitor alpha (NFKBIA) is an up-regulated DEP. However, it is connected to 6 critical DEPs, but it has appeared with a less important role in the other assessment such as action map analysis, gene ontology evaluation, and PPI network investigation. Approximately, all analyses indicate that the critical nodes can be divided into 2 groups: First; EGFR, FN1, CD44, and PTGS2, and second; NFKBIA, PHGDH, CTSD, KEAP1, and NT5E. The first group is involved in the radiation resistance significantly, while the second group is related weakly.
5.1. Conclusions
In conclusion, protein expression changes of EGFR, FN1, CD44, PTGS2, NFKBIA, KEAP1, CTSD, PHGDH, and NT5E are the core of radiation resistance even in human cells. More analysis revealed that the elements of the introduced core can be divided into 2 categories. The first class including 4 proteins (EGFR, FN1, CD44, and PTGS2) is the main dysregulated part of the proteome, which is involved in radiation resistance at the cellular level.
Acknowledgements
References
-
1.
Schaue D, McBride WH. Opportunities and challenges of radiotherapy for treating cancer. Nat Rev Clin Oncol. 2015;12(9):527-40. [PubMed ID: 26122185]. [PubMed Central ID: PMC8396062]. https://doi.org/10.1038/nrclinonc.2015.120.
-
2.
Olivares-Urbano MA, Grinan-Lison C, Marchal JA, Nunez MI. CSC radioresistance: A therapeutic challenge to improve radiotherapy effectiveness in cancer. Cells. 2020;9(7). [PubMed ID: 32660072]. [PubMed Central ID: PMC7407195]. https://doi.org/10.3390/cells9071651.
-
3.
Mischak H, Allmaier G, Apweiler R, Attwood T, Baumann M, Benigni A, et al. Recommendations for biomarker identification and qualification in clinical proteomics. Sci Transl Med. 2010;2(46):46ps42. [PubMed ID: 20739680]. https://doi.org/10.1126/scitranslmed.3001249.
-
4.
Zali H, Zamanian-Azodi M, Rezaei Tavirani M, Akbar-Zadeh Baghban A. Protein drug targets of Lavandula angustifolia on treatment of Rat alzheimer's disease. Iran J Pharm Res. 2015;14(1):291-302. [PubMed ID: 25561935]. [PubMed Central ID: PMC4277642].
-
5.
Vasileva D, Badawi A. C-reactive protein as a biomarker of severe H1N1 influenza. Inflamm Res. 2019;68(1):39-46. [PubMed ID: 30288556]. [PubMed Central ID: PMC6314979]. https://doi.org/10.1007/s00011-018-1188-x.
-
6.
Aguilan JT, Kulej K, Sidoli S. Guide for protein fold change and P-value calculation for non-experts in proteomics. Mol Omics. 2020;16(6):573-82. [PubMed ID: 32968743]. https://doi.org/10.1039/d0mo00087f.
-
7.
Antonov AV, Dietmann S, Rodchenkov I, Mewes HW. PPI spider: a tool for the interpretation of proteomics data in the context of protein-protein interaction networks. Proteomics. 2009;9(10):2740-9. [PubMed ID: 19405022]. https://doi.org/10.1002/pmic.200800612.
-
8.
Asadzadeh-Aghdaee H, Shahrokh S, Norouzinia M, Hosseini M, Keramatinia A, Jamalan M, et al. Introduction of inflammatory bowel disease biomarkers panel using protein-protein interaction (PPI) network analysis. Gastroenterol Hepatol Bed Bench. 2016;9(Suppl1):S8-s13. [PubMed ID: 28224022]. [PubMed Central ID: PMC5310794].
-
9.
Mansouri V, Razzaghi M, Rostami-Nejad M, Rezaei-Tavirani M, Heidari MH, Safari S, et al. Neuroprotective properties of photobiomodulation in retinal regeneration in Rats: Perspectives from interaction levels. J Lasers Med Sci. 2020;11(3):280-6. [PubMed ID: 32802288]. [PubMed Central ID: PMC7369552]. https://doi.org/10.34172/jlms.2020.47.
-
10.
Rezaei Tavirani M, Mansouri V, Rezaei Tavirani S, Hesami Tackallou S, Rostami - Nejad M. Gliosarcoma protein - protein interaction network analysis and gene ontology. Int J Cancer Manag. 2018;11(5). https://doi.org/10.5812/ijcm.65701.
-
11.
Marcone S, Buckley A, Ryan CJ, McCabe M, Lynam-Lennon N, Matallanas D, et al. Proteomic signatures of radioresistance: Alteration of inflammation, angiogenesis and metabolism-related factors in radioresistant oesophageal adenocarcinoma. Cancer Treat Res Commun. 2021;27:100376. [PubMed ID: 33882379]. https://doi.org/10.1016/j.ctarc.2021.100376.
-
12.
Abbaszadeh HA, Peyvandi AA, Sadeghi Y, Safaei A, Zamanian-Azodi M, Khoramgah MS, et al. Er:YAG laser and cyclosporin a effect on cell cycle regulation of human gingival fibroblast cells. J Lasers Med Sci. 2017;8(3):143-9. [PubMed ID: 29123635]. [PubMed Central ID: PMC5662504]. https://doi.org/10.15171/jlms.2017.26.
-
13.
Zhang J, Yang Y, Wang Y, Zhang J, Wang Z, Yin M, et al. Identification of hub genes related to the recovery phase of irradiation injury by microarray and integrated gene network analysis. PLoS One. 2011;6(9). e24680. [PubMed ID: 21931809]. [PubMed Central ID: PMC3172286]. https://doi.org/10.1371/journal.pone.0024680.
-
14.
Chakravarti A, Dicker A, Mehta M. The contribution of epidermal growth factor receptor (EGFR) signaling pathway to radioresistance in human gliomas: a review of preclinical and correlative clinical data. Int J Radiat Oncol Biol Phys. 2004;58(3):927-31. [PubMed ID: 14967452]. https://doi.org/10.1016/j.ijrobp.2003.09.092.
-
15.
Zhang M, Han N, Jiang Y, Wang J, Li G, Lv X, et al. EGFR confers radioresistance in human oropharyngeal carcinoma by activating endoplasmic reticulum stress signaling PERK-eIF2alpha-GRP94 and IRE1alpha-XBP1-GRP78. Cancer Med. 2018;7(12):6234-46. [PubMed ID: 30414263]. [PubMed Central ID: PMC6308109]. https://doi.org/10.1002/cam4.1862.
-
16.
Yang L, Lu P, Yang X, Li K, Chen X, Zhou Y, et al. Downregulation of annexin A3 promotes ionizing radiation-induced EGFR activation and nuclear translocation and confers radioresistance in nasopharyngeal carcinoma. Exp Cell Res. 2022;418(2):113292. [PubMed ID: 35850266]. https://doi.org/10.1016/j.yexcr.2022.113292.
-
17.
Yazlovitskaya EM, Linkous AG, Thotala DK, Cuneo KC, Hallahan DE. Cytosolic phospholipase A2 regulates viability of irradiated vascular endothelium. Cell Death Differ. 2008;15(10):1641-53. [PubMed ID: 18566601]. https://doi.org/10.1038/cdd.2008.93.
-
18.
Kim SR, Heo JI, Park JW, Kang CM, Kim KS. Radiation-induced lipoprotein-associated phospholipase A2 increases lysophosphatidylcholine and induces endothelial cell damage. Toxicology. 2021;458:152841. [PubMed ID: 34216699]. https://doi.org/10.1016/j.tox.2021.152841.
-
19.
Cordes N, Blaese MA, Plasswilm L, Rodemann HP, Van Beuningen D. Fibronectin and laminin increase resistance to ionizing radiation and the cytotoxic drug Ukrain in human tumour and normal cells in vitro. Int J Radiat Biol. 2003;79(9):709-20. [PubMed ID: 14703944]. https://doi.org/10.1080/09553000310001610240.
-
20.
Tan C, Liu L, Liu X, Qi L, Wang W, Zhao G, et al. Activation of PTGS2/NF-kappaB signaling pathway enhances radiation resistance of glioma. Cancer Med. 2019;8(3):1175-85. [PubMed ID: 30740906]. [PubMed Central ID: PMC6434213]. https://doi.org/10.1002/cam4.1971.
-
21.
Zhao Y, Kang JH, Yoo KC, Kang SG, Lee HJ, Lee SJ. K-RAS acts as a critical regulator of CD44 to promote the invasiveness and stemness of GBM in response to Ionizing radiation. Int J Mol Sci. 2021;22(20). [PubMed ID: 34681583]. [PubMed Central ID: PMC8539357]. https://doi.org/10.3390/ijms222010923.