Abstract
Background:
Genetic expression has been frequently considered as an efficient method for early diagnosis of cancer. In this study, we examined the simultaneous effect of 22 genes on contribution to bladder cancer.Objectives:
Since these 22 genes are known as the most important risk factors in many cancers, we aimed to investigate them as potential effective genes in bladder cancer.Methods:
The data consist of 25 patients with bladder cancer (the case group) and 23 matched healthy individuals as a control group. Univariate analysis was performed and differences between two groups were analyzed through the independent T-test. A multivariate gene expression model was implemented using the least absolute shrinkage and selection operator (LASSO) and Adaptive LASSO regression. Standard error of coefficients was obtained using the bootstrap method. We used two methods for classification and compared areas under the curve (AUC), using receiver operating characteristic (ROC) curve.Results:
Independent T-test showed that 11 genes had a significant difference between the two groups. Also multivariate analysis using the LASSO revealed that 12 genes have a significant effect on bladder cancer and adaptive lasso regression represented SDF1, CTLA-4, Her2 and IL-23 genes as the most effective genes. The AUC for LASSO and Adaptive LASSO were 0.71 and 0.89, respectively which was statistically significant (P = 0.009). Our multivariable results for SDF1, CTLA-4 and IL-23 confirm the findings of many studies in this field.Conclusions:
Among all genes were examined, SDF1, CTLA-4, Her2 and IL-23 which were selected by the two methods has the greatest contribution to bladder cancer.Keywords
1. Background
Bladder cancer is one of the most common cancers. It is the fourth most common cancer, the ninth cause of cancer death in males and the eighth most common cancer in females (1, 2). Every year 330,000 people throughout the world are diagnosed with bladder cancer (3). For the same reason in the early symptoms with many benign diseases of the urinary tract, usually the initial diagnosis of bladder cancer may be delayed, causing the progression of the disease to higher stages (4).
Although so far several tests have been designed and used based on genetic factors in early detection of cancer, determination of prognosis and treatment, in few studies the influence of genes have been considered simultaneously (5). Due to high associations between genes, genetic markers have special complexities and single gene analysis is not efficient in the diagnosis and treatment of cancers. High cost of genetic studies is another problem leading to smaller sample sizes. Therefore, using methods, which are efficient in low sample size and capable in considering simultaneous effects of different genes, seems necessary.
Recently, penalized regression, as an effective method, has been used in high dimensional and low sample size settings in many branches of science. Penalized regression is applicable even in cases where the number of variables is much more than the sample size like microarray studies. Tibshirani was the first researcher who used penalized method in cancer researches. He examined the association between the level of prostate specific antigen and a number of clinical variables (6). Zou and Hastie applied penalized method in leukemia data where they had 1000 gene expression and 38 samples and Huang et al. implemented penalized method in a breast cancer study where they had 500 genes (7).
Least absolute shrinkage and selection operator (LASSO) is one of the most famous penalized methods which were obtained by adding a function in the common estimator. This constraint in imposing a penalty causes many of the coefficients to be small and the others are absolutely zero. In 2006, zou introduced adaptive LASSO that is LASSO with weighted penalties.
2. Objectives
The aim of this study is identifying the genes which have the most significant contribution in bladder cancer using LASSO and a modified version of LASSO with weighted penalties (Adaptive LASSO) as the two most well known penalized methods.
3. Methods
Case group: all patients with bladder cancer who were referred to one of Faghihi, Namazi or Aliasghar hospitals in Shiraz city, south of Iran, during the years 2009 - 2011 and histopathologic examination had confirmed they suffered from bladder cancer. The patients undergoing surgery to remove the cancerous tumor or receiving chemotherapy or radiotherapy were excluded. None of the patients had metabolic diseases, immunological, genetic and infection during the sampling and no one received any treatment for their cancer.
Control group: clients who lived in the nursing home located in the Kholde Barin Park, Shiraz city in the years 2009 - 2011, did not have any of the following: urinary problems, a history of cancer and autoimmune disease, neither themselves nor their first degree relatives. Those with any type of disease during two weeks before the sampling day were excluded. After removal of the cases with missing values, finally the case and the control groups respectively consisted of 25 and 23 patients.
3.1. Real Time PCR
Real time PCR was applied to evaluate gene expression in these patients. For this, about 3 mL peripheral blood was taken from each patient and total RNA was extracted by TRIzol reagent (Invitrogen, USA) after RBC lysis by NH4Cl, as described by manufacturer’s protocol. DNA contamination was removed by DNase I treatment. After that, about 5 µg of total RNA was reverse transcripted into cDNA using revet Aid H minus Reverse transcriptase kit (fermentase, Lithuania) according to protocol recommended by kit. Specific primers for each gene were designed by Primer Blast online software (6). Finally, expression of each gene was determined by SYBR green I (ABI, USA) based on 2-ΔCt formula. Standard efficiency was calculated based on positive control amplification efficiency. For this purpose, the logarithmic dilutions of positive control were amplified and the acquired cycling thresholds (Ct) was utilized to plot a standard curve. Slope of standard curve was applied to the below formula and calculated efficiency of real time PCR reaction.
Efficiency = (10-1/slope -1) × 100
The calculated efficiency of all measured mRNA expressions were between 90% - 100%.
Statistical analysis was calculated by 2-ΔCt result of each patient. In order to reduce the computational complexity of the distribution of the information, in the first step suitable transformation implement and the logarithm of gene expression were considered up to six decimal places as the independent variable.
3.2. Statistical Analyses
In this study, we used the inverse LASSO coefficients for each variable as their weight in adaptive LASSO. Adaptive LASSO enjoys all the advantages of LASSO, chooses fewer variables than LASSO and provides an interpretable model (8, 9). In order to compare two methods, classification was performed and areas under the curve (AUC) in receiver operating characteristic (ROC) curve were calculated for both models. All the statistical analyses were performed via SPSS 18.0, MedCalc 14.0 and parcor package in R 3.0.3 software.
4. Results
In this study, 25 patients with bladder cancer as the experimental group and 23 subjects in a control group were studied. Descriptive statistics of the variables is shown in Table 1 and differences between the two groups were analyzed using independent T-test.
Comparison of Mean Logarithm of the Genes Expression in Two Groups
Gene | Case Group (N = 25) | Control Group (N = 23) | P Value | ||
---|---|---|---|---|---|
Mean | Std.Error | Mean | Std.Error | ||
CXCR4 | -0.506 | 0.859 | -0.541 | 0.694 | 0.88 |
OCT-4 | -1.671 | 1.37 | -2.634 | 0.771 | 0.004 |
SDF-1 | -4.016 | 1.106 | -7.968 | 2.359 | < 0.001 |
BCL2 | -1.985 | 0.725 | -2.751 | 0.866 | 0.003 |
TP53 | -1.197 | 0.859 | -1.569 | 0.604 | 0.088 |
Fas | -1.605 | 0.507 | -1.757 | 0.721 | 0.400 |
CTLA-4 | -2.391 | 0.572 | -3.307 | 0.815 | < 0.001 |
Foxp3 | -2.581 | 0.598 | -3.249 | 0.665 | < 0.001 |
CXCR3 | -1.527 | 1.451 | -1.058 | 1.399 | 0.261 |
E-Cadherin | -3.68 | 1.314 | -2.907 | 1.693 | 0.082 |
Her2 | -2.227 | 1.089 | -1.294 | 1.49 | 0.016 |
IFN γ | -2.142 | 1.54 | -2.941 | 1.611 | 0.086 |
IP10 | -2.651 | 1.456 | -2.643 | 1.624 | 0.987 |
IL12 A | -3.207 | 1.041 | -2.381 | 1.079 | 0.01 |
IL12 B | -2.913 | 1.365 | -3.332 | 1.895 | 0.387 |
MDM2 | -2.63 | 0.637 | -2.308 | 1.323 | 0.282 |
Survivin | -3.488 | 1.342 | -2.356 | 2.251 | 0.044 |
IL-23 | -1.637 | 0.941 | -3.899 | 2.373 | < 0.001 |
IL-27 | -3.363 | 0.808 | -5.683 | 1.906 | < 0.001 |
IL-6 | -2.778 | 0.882 | -2.575 | 1.423 | 0.559 |
TGFβ | -3.918 | 1.546 | -1.065 | 2.633 | < 0.001 |
IL-17 | -3.49 | 1.094 | -3.344 | 1.489 | 0.699 |
With the matrix X which includes all 22 independent variables (gene expression) for 48 subjects under the study and matrix Y which represents the membership of case or the control group, fitting the LASSO regression model and inverse coefficients of each variable were used as the weight in the adaptive LASSO method. Standard error of coefficients was obtained using the bootstrap method which was repeated 500 times. Table 2 presents the results of fitting the two models. As can be seen, the LASSO model estimates zero coefficients of 10 variables, which were removed from the model. Four variables had coefficients larger than 0.1 whereas 8 variables had coefficients smaller than 0.1, they remained in the model. However, LASSO method eliminates a number of redundant variables. It seems that it is unable to remove all the redundant variables.
Results of Fitting LASSO and Adaptive-LASSO Models
Variable | LASSO | Adaptive LASSO | ||
---|---|---|---|---|
Coefficient | MSE | Coefficient | MSE | |
CXCR4 | -0.01 | 0.064 | 0 | 0.035 |
OCT4 | 0.022 | 0.166 | 0 | 0.181 |
SDF1 | 0.234 | 0.046 | 0.27 | 0.053 |
BCL2 | 0 | 0.084 | 0 | 0.07 |
P53 | -0.067 | 0.211 | 0 | 0.233 |
Fas | -0.043 | 0.138 | 0 | 0.124 |
CTLA-4 | 0.142 | 0.124 | 0.114 | 0.131 |
Foxp3 | 0 | 0.104 | 0 | 0.076 |
CXCR3 | 0 | 0.078 | 0 | 0.054 |
E-Cadherin | -0.032 | 0.106 | 0 | 0.11 |
Her2 | -0.109 | 0.189 | -0.075 | 0.187 |
IFN γ | 0 | 0.041 | 0 | 0.028 |
IP10 | 0 | 0.053 | 0 | 0.008 |
IL12 A | -0.04 | 0.116 | 0 | 0.068 |
IL12 B | 0.09 | 0.122 | 0 | 0.147 |
MDM2 | 0 | 0.054 | 0 | 0.035 |
Survivin | 0 | 0.083 | 0 | 0.05 |
IL-23 | 0.12 | 0.076 | 0.099 | 0.062 |
IL-27 | 0.031 | 0.077 | 0 | 0.067 |
IL-6 | 0 | 0.081 | 0 | 0.062 |
TGFβ | 0 | 0.03 | 0 | 0.023 |
IL-17 | 0 | 0.055 | 0 | 0.054 |
In contrast, Adaptive LASSO with eliminated 18 variables defines only four genes, i.e. SDF1, CTLA-4, Her2, and IL-23, as the variables which have contributed in bladder cancer and can affect the risk of developing this disease. Small values of the standard errors of the coefficients indicate that the model has a very high level of accuracy. In addition, due to the elimination of 18 ineffective variables, Adaptive LASSO technique has a good interpreting ability. The ROC curve revealed that the AUC for LASSO and Adaptive LASSO were 0.71 and 0.89 respectively (Figure 1) which was statistically significant (P = 0.009).
Area Under the ROC Curve for LASSO and Adaptive LASSO
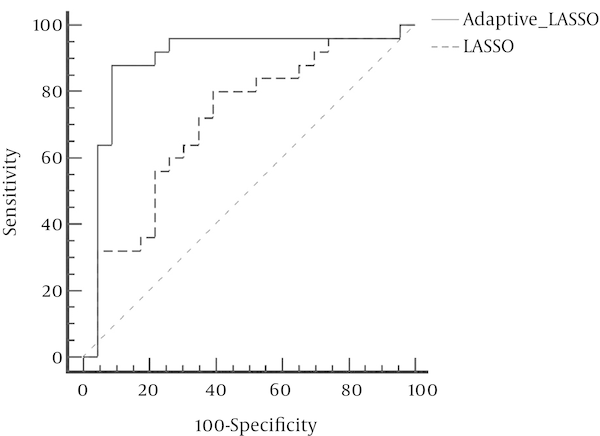
5. Discussion
To the best of our knowledge, this study is the first in evaluating the simultaneous effect of expression of this 22 genes that had an important role in many cancers at the same time. The results indicate that the expression of SDF1, CTLA-4, Her2 and IL-23 has the greatest effect on bladder cancer.
Variables that are introduced to adaptive LASSO method as genes associated with bladder cancer confirm the results of many studies in this field. Several studies on SDF1expression of genes involve metastasis and cell movement. Gosalbez et.al showed that the amount of mRNA (gene expression) has a significant increase in bladder cancer tissues compared to normal bladder tissue. They also reported that the expression of SDF1 in metastatic cancer cells and cancer-related mortality rates were higher (10). Over-expression of CTLA-4 gene in the body causes cancer cells to escape the immune system without any problems and continue to grow and reproduce, and gene expression of IL-23 coincides with the induction of inflammation that contributes to better growth of cancer cells (7, 11-13). Although the results obtained for these three genes are consistent with univariate studies, this does not happen for Her2 (14, 15). It is noteworthy that most of the studies on the relationship between genes expression and cancer carried out on any gene analyzed genes expression separately. Nevertheless, the correlation between the expressions of different genes is obvious. In this study, we considered the effect of 22 common genes expression which are known as risk factors in most cancers on the risk of bladder cancer. Among all genes examined above, SDF1, CTLA-4, Her2 and IL-23 which were selected by the two methods have the greatest effects on bladder cancer.
However, in this study, the patients’ data with bladder cancer who referred to hospitals in Shiraz city as the center in the Southern Iran, were used. Due to missing information on some genes, many of these patients were excluded. Another limitation of this study is that it was done only on men. Although the study could be a first step toward early, easy, safe and secure diagnosis of bladder cancer, these results could not be considered conclusive and larger multicenter studies in different parts for greater generalizability of results and achieving a larger sample size are necessary.
This study once again indicated the superiority of penalized methods compared to conventional ones in dealing with data of high dimension and low sample size.
Acknowledgements
References
-
1.
Andrew AS, Gui J, Sanderson AC, Mason RA, Morlock EV, Schned AR, et al. Bladder cancer SNP panel predicts susceptibility and survival. Hum Genet. 2009;125(5-6):527-39. [PubMed ID: 19252927]. https://doi.org/10.1007/s00439-009-0645-6.
-
2.
Mohammad-Beigi A, Rezaeeianzadeh A, Tabbatabaei HR. Application of life table in survival analysis of patients with bladder cancer. Zahedan J Res Med Sci. 2011;13(3):25-9.
-
3.
Sanderson S, Salanti G, Higgins J. Joint effects of the N-acetyltransferase 1 and 2 (NAT1 and NAT2) genes and smoking on bladder carcinogenesis: a literature-based systematic HuGE review and evidence synthesis. Am J Epidemiol. 2007;166(7):741-51. [PubMed ID: 17675654]. https://doi.org/10.1093/aje/kwm167.
-
4.
Wallace DM, Raghavan D, Kelly KA, Sandeman TF, Conn IG, Teriana N, et al. Neo-adjuvant (pre-emptive) cisplatin therapy in invasive transitional cell carcinoma of the bladder. Br J Urol. 1991;67(6):608-15. [PubMed ID: 2070206].
-
5.
Harris L, Fritsche H, Mennel R, Norton L, Ravdin P, Taube S, et al. American Society of Clinical Oncology 2007 update of recommendations for the use of tumor markers in breast cancer. J Clin Oncol. 2007;25(33):5287-312. [PubMed ID: 17954709]. https://doi.org/10.1200/JCO.2007.14.2364.
-
6.
Ye J, Coulouris G, Zaretskaya I, Cutcutache I, Rozen S, Madden TL. Primer-BLAST: a tool to design target-specific primers for polymerase chain reaction. BMC Bioinformatics. 2012;13:134. [PubMed ID: 22708584]. https://doi.org/10.1186/1471-2105-13-134.
-
7.
Langowski JL, Zhang X, Wu L, Mattson JD, Chen T, Smith K, et al. IL-23 promotes tumour incidence and growth. Nature. 2006;442(7101):461-5. [PubMed ID: 16688182]. https://doi.org/10.1038/nature04808.
-
8.
Huang J, Ma S, Zhang CH. Adaptive Lasso for sparse high-dimensional regression models. Statistica Sinica. 2008;18(4):1603.
-
9.
Huang J, Ma S, Zhang CH. The iterated lasso for high-dimensional logistic regression. The University of Iowa Department of Statistical and Actuarial Science Technical Report. 2008;(392).
-
10.
Gosalbez M, Hupe MC, Lokeshwar SD, Yates TJ, Shields J, Veerapen MK, et al. Differential expression of SDF-1 isoforms in bladder cancer. J Urol. 2014;191(6):1899-905. [PubMed ID: 24291546]. https://doi.org/10.1016/j.juro.2013.11.053.
-
11.
Chambers CA, Kuhns MS, Egen JG, Allison JP. CTLA-4-mediated inhibition in regulation of T cell responses: mechanisms and manipulation in tumor immunotherapy. Annu Rev Immunol. 2001;19:565-94. [PubMed ID: 11244047]. https://doi.org/10.1146/annurev.immunol.19.1.565.
-
12.
Langrish CL, Chen Y, Blumenschein WM, Mattson J, Basham B, Sedgwick JD, et al. IL-23 drives a pathogenic T cell population that induces autoimmune inflammation. J Exp Med. 2005;201(2):233-40. [PubMed ID: 15657292]. https://doi.org/10.1084/jem.20041257.
-
13.
Tivol EA, Borriello F, Schweitzer AN, Lynch WP, Bluestone JA, Sharpe AH. Loss of CTLA-4 leads to massive lymphoproliferation and fatal multiorgan tissue destruction, revealing a critical negative regulatory role of CTLA-4. Immunity. 1995;3(5):541-7. [PubMed ID: 7584144].
-
14.
Lae M, Couturier J, Oudard S, Radvanyi F, Beuzeboc P, Vieillefond A. Assessing HER2 gene amplification as a potential target for therapy in invasive urothelial bladder cancer with a standardized methodology: results in 1005 patients. Ann Oncol. 2010;21(4):815-9. [PubMed ID: 19889613]. https://doi.org/10.1093/annonc/mdp488.
-
15.
Tetu B, Fradet Y, Allard P, Veilleux C, Roberge N, Bernard P. Prevalence and clinical significance of HER/2neu, p53 and Rb expression in primary superficial bladder cancer. J Urol. 1996;155(5):1784-8. [PubMed ID: 8627884].