Abstract
Background:
Asparginase is known to be one of the most important bedrocks of acute lymphoblastic leukemia (ALL) treatment in almost all pediatric regimens in treatment protocols. Escherichia coli L-Asparginase (EC 3.5.1.1) is one of the most common resources to produce this enzyme. One of the affordable methods to overcome the side effects of drug is utilizing bioinformatic tools in the form of In silico study. In this study we designed a new structure of L-Asparginase to decrease its toxicity, reduce some side effects and increase the stability.Methods:
We used some bioinformatics software and servers like Toxin red, Popmusic, kobami and I-TASSER server to reduce toxicity level of enzyme, and to increase stability and enzyme half-life.Results:
We obtained 6 protein sequences in which the best was Mut 6 with four changes in structure: L23G, K129L, S263C and R291F. In contrast to the wild type, the new predicted protein is not toxic and has 25 hours more half-life and 600 kcal/mol more stable with no significant change in protein secondary, tertiary structure, antigenicity and allergenicity.Conclusions:
Finally, sequence number 6 was the only sequence with all distinct characteristics: non-toxic, more stability and more half life.Keywords
L-Asparginase Bioinformatics Acute Lymphoblastic Leukemia Toxicity Stability
1. Introduction
Acute lymphoblastic leukemia (ALL) cancer is an uncontrolled division of immature B-cells, which are called Lymphoblasts, in human bone marrow (1). Different mild and offensive kinds of treatments are advised for ALL such as stem cell and bone marrow transplantation, chemo and radiotherapy and treatments with steroids. Experiments have shown that chemotherapy is regarded as the best method of treatment for ALL. In most cases, treatment of this disease has three phases (Table 1). Prescriptions usually contain a list of drugs, which include: methotrexate, hydrocortisone, prednisolone, dexamethasone, cyclophosphamide, vincristine, daunorubicin, cytarabine, thioguanine, etoposide, mercaptopurine and Asparginse (2).
Three Phases of Treatment and Different Prescriptions for All
Phase | Description | Treatment Protocol | Ref. |
---|---|---|---|
Remission Induction | The main goal of this phase is to kill cancer cells and improve the patient’s condition. CNS prophylaxis starts at this point and continues to phase 2 | Asparginse (better tolerance in children) + Prednisolone + Dexamethasone + Vincristine + Danorubicine (In adults ALL) | (3) |
Consolidation/Intensification | At this phase, a high volume of multiple intravenous infusions of anti-tumors is used to reduce the tumor volume | Vincristine + Danorubicine + Etoposide +6-Thioguanin + MercaptopurineFor CNS Protection: Methotrexate or cytarabine (intrathecal), which is sometimes combined with Kranyvaspynal radiotherapy For recurrent CNS: Intrathecal infusion of Hydrocortisone + Methotrexate + Cytarabine | |
Maintenance therapy | The goal is to kill any remaining cancer cells. Despite the low number, they can cause relapses. | The daily oral uptake of Mercaptopurine + oral uptake of Methotrexate once a week + Once monthly 5-day course of intravenous Vincristine + Oral administration of corticosteroids (The duration of this phase of treatment for adults is 3 and for children is 2 years) |
Asparginase (EC 3.5.1.1) is known to be one of the most important bed rocks of ALL treatment in almost all pediatric regimens of youth and adults’ treatment protocols (4). Various prokaryotic and eukaryotic resources that are able to provide this enzyme include different kinds of Bacteria, Algae, Fungi (4) and Yeasts play an important role in amino acids metabolism, and utilization and maintaining nitrogen balance within cells. By the action of this enzyme, asparagines hydrolyzed to aspartate. Then there are various pathways possible for Aspartate (5). For example, transamination of aspartate into oxaloacetate (one of critical compound of Tricarboxylic acid cycle (TCA) or conversion into fumarate during the urea cycle (6). E. coli possesses two kinds of this enzyme: Type I, which is the cytoplasmic form and is encoded by ansA; and Type II, which is the periplasmic form and is encoded by ansB (4). Type I is expressed when asparagines is the sole source of nitrogen, but type II expresses under nutrient depletion in anaerobic conditions regulated by cAMP and oxygen-sensing regulator of fumarate and nitrate reduction (FNR) protein. Type II is the enzyme which is used as a therapeutic agent in ALL while Type I has no therapeutic activity (7).
According to the source of the enzyme (E. coli or Erwinia asparaginase), various side effects of the drug could be considered as: allergic or hypersensitivity reactions, Anaphylaxis, coagulation, abnormalities, neurotoxicity, convulsions, Pancreatitis, diabetes requiring insulin, liver toxicity, infection and death (8). On the other hand, in its native form and without any protective treatment, the enzyme has been shown low in vivo half-life when it is introduced into the body (9). Since reducing the side effects of the enzyme and increasing its stability in in vivo systems are two important achievements as therapeutic aims, bioinformatic study and in silico study seem to be nice tricks to predict and estimate before starting the work (10).
Bioinformatics makes the rapid organization and analysis of biological data. It is a branch of science utilizing different techniques like computer science, applied mathematics, statistics, informatics, chemistry, artificial intelligence and biochemistry to design an algorithm as a tool or software for biological problem solving usually on molecular levels (11, 12). With Bioinformatics we can guess and estimate biomolecules’ compounds behavior either in in vitro or in vivo condition and promote the therapeutic ability of bio-pharmaceutical compounds like enzymes. It is more affordable and time-saving to have the results in a Bioinformatics study. The main aim of this study is predicting a molecular solution to decrease enzyme’s toxicity, reduce some side effects and increase the enzyme’s stability during the Bioinformatics job by its amino acids manipulation to yield better levels of the therapeutic drug.
2. Methods
2.1. Sequence Primary Data Analysis
Related sequence of Escherichia coli L-asparaginase (isozyme II) was obtained from the national centre for biotechnology information (13). Multiple sequence alignments were accomplished using ClustalW2 software of the European Bioinformatics Institute website (14). The functional information of protein sequence was achieved from the Protein Knowledgebase (15).
2.2. Protein Toxicity Analysis and Evaluation of Its Stability
Predicting and designing the least toxic peptide of L-Asparginase protein, was carried out with ToxinPred which is a developer in silico method, to predict and design toxic/non-toxic peptides (16). PoPMuSiC (prediction of protein mutant stability changes) program was used in order for L-asparaginase stability improvement. This program evaluates the stability changes under single-site mutations and gives a report containing a list of the most stabilizing or destabilizing mutations (17).
2.3. Bioinformatics Analysis of the Wild Type and Mutant Protein
The secondary structure of the wild type and 6 mutant proteins, which are probably non-toxic and more stable than the wild type, were analyzed by GOR 4 server (18). 3D protein structures of these genes were predicted by I-TASSER server (19). Accelrys Discovery Studio 2.5 software was used to visualize the modeled 3D structures. Finally the physico-chemical parameters such as: molecular weight, isoelectric point (pI), half-life, total number of positive and negative residues, aliphatic and hydrophobic indices were computed using the Expasy’s ProtParam (20).
2.4. Predicting Antigens and Allergens
Although there is no perfect method for antigenic peptide prediction, there are several guidelines which could be followed to determine how many peptide fragments of a protein are possibly antigenic, for example, antigenic peptides contain both hydrophilic and hydrophobic residues and glycoproteins of cell surface eliminate from primary peptides which contain consensus sites for N-glycosylation (21). Antigens prediction of all proteins was performed by the VaxiJen server (22). Immunoglobulin E (IgE) plays an essential role in type I hyperreactive reactions and an effective role in allergic conditions (23); therefore, prediction of allergens based on similarity of known IgE epitopes with any region of protein was analyzed by the AlgPred server (24).
2.5. Evaluation of Predicting Structure
The energy minimization of wild type protein and all mutants were calculated by KoBamin (knowledge-based potential refinement for proteins) server (25). Additionally, the structural stability of the proteins was confirmed by Ramachandran plot.
2.6. Prediction of B-Cell Epitopes
For prediction of B-cell epitopes, BCPred software was used to determine the continuous B cell epitope based on single characters, including antigenicity, hydrophilicity, flexibility, polarity, exposed surface and accessibility (26). Also, the conformational epitopes for B cells were predicted by the Discotope server (27).
3. Results
3.1. Sequence Primary Data Analysis
To have a stable enzyme, we have considered some parameters like mutation effect on different regions of protein sequence at the beginning of the survey. All the related protein sequences of Escherichia coli L-asparaginase (isozyme II) were compared by ClustalW2 software. The result showed some conflict in the protein sequences (Table 2). The protein’s structural-functional information was obtained from the Protein Knowledgebase (Table 3).
List of Conflict’s Position in Protein Sequence Obtained from Clustalw2 Analysis
Position | Sequence Conflict |
---|---|
49 | V → A |
86 | N → D |
206 | N → D |
268 | N → D |
274 | S → T |
284 | T → N |
314 | V → I |
Protein’s Information Based on Protein Knowledgebase
Properties | Description |
---|---|
Protein name | L-asparginase 2 |
EC number | 3.5.1.1 |
Gene name | ansB |
Locus | B2957JW2924 |
Organism | E. coli K12 |
Sequence length | 348AA |
Catalytic activity | Asn + H2O = L-aspartate + NH3 |
Subunit structure | Homotetramer |
Subcellular location | Periplasm |
Molecular function | Hydrolase |
Signal peptide | 1 - 22 |
Chain | 23 - 148 |
Substrate binding | 80 - 81, 111 - 112 |
Active site | 34 |
Disulfide bond | 99 ↔ 127 |
Mass | 36,851 |
Entry | P00805 |
3.2. Protein Toxicity Analysis and Evaluation of Its Stability
ToxinPred software was used to predict the protein’s toxicity for designing the least toxic peptides. This software is an in silico model for predicting toxicity of peptides and proteins that were described by Cho et al. (28). This software is based on machine learning technique and quantitative matrix using various properties of peptides for predicting their toxicity. The protein scanning module of ToxinPred was used in order to carry out toxic and non-toxic peptid prediction. This module identified one highly toxic region in L-Asparginase protein (Table 4). Each amino acid substitution was tested one by one to reach nontoxic peptides after structural prediction (Table 5).
Finding Toxic Regions in Protein Sequence
Peptides Scanned from Original Protein | ||||
---|---|---|---|---|
Peptide Sequence | SVM Score | Prediction | Hydrophobicity | Hydrophilicity |
GTDTMEETAY | -0.64 | Non Toxic | -0.18 | 0.37 |
TDTMEETAYF | -0.60 | Non Toxic | -0.14 | 0.12 |
DTMEETAYFL | -0.51 | Non Toxic | -0.07 | -0.02 |
TMEETAYFLD | -0.77 | Non Toxic | -0.07 | -0.02 |
MEETAYFLDL | -1.13 | Non Toxic | 0.01 | -0.16 |
EETAYFLDLT | -1.05 | Non Toxic | -0.04 | -0.07 |
ETAYFLDLTV | -1.16 | Non Toxic | 0.08 | -0.52 |
TAYFLDLTVK | -1.06 | Non Toxic | 0.03 | -0.52 |
AYFLDLTVKC | -0.80 | Non Toxic | 0.05 | -0.58 |
YFLDLTVKCD | -0.46 | Non Toxic | -0.04 | -0.23 |
FLDLTVKCDK | -0.35 | Non Toxic | -0.16 | 0.30 |
LDLTVKCDKP | -0.16 | Non Toxic | -0.22 | 0.55 |
DLTVKCDKPV | -0.13 | Non Toxic | -0.22 | 0.58 |
LTVKCDKPVV | -0.24 | Non Toxic | -0.10 | 0.13 |
TVKCDKPVVM | -0.02 | Non Toxic | -0.12 | 0.18 |
VKCDKPVVMV | 0.16 | Toxic | -0.05 | 0.07 |
KCDKPVVMVG | -0.22 | Non Toxic | -0.09 | 0.22 |
CDKPVVMVGA | -0.55 | Non Toxic | 0.04 | -0.13 |
DKPVVMVGAM | -0.77 | Non Toxic | 0.07 | -0.16 |
KPVVMVGAMR | -1.27 | Non Toxic | -0.04 | -0.16 |
PVVMVGAMRP | -1.19 | Non Toxic | 0.06 | -0.46 |
Amino Acid Substitution After Structural Prediction- Amino Acid Changes in Mutants are Indicated with Red Color and Star Indicate That All Amino Acids Can Be Used
Original peptide | |||||
---|---|---|---|---|---|
Peptide Sequence | Mutation Position | SVM Score | Prediction | Hydrophobicity | Hydrophilicity |
VKCDKPVVMV | No mutation | 0.46 | Toxic | -0.05 | 0.07 |
AKCDKPVVMV | 128 | 0.13 | Toxic | -0.08 | 0.17 |
Mutant Peptide | |||||
CKCDKPVVMV | 128 | 0.10 | Toxic | -0.10 | 0.12 |
DKCDKPVVMV | 128 | 0.01 | Toxic | -0.18 | 0.52 |
EKCDKPVVMV | 128 | -0.15 | Non Toxic | -0.17 | 0.52 |
FKCDKPVVMV | 128 | -0.09 | Non Toxic | -0.05 | 0.22 |
GKCDKPVVMV | 128 | 0.13 | Toxic | -0.09 | 0.17 |
HKCDKPVVMV | 128 | -0.39 | Non Toxic | -0.15 | 0.04 |
IKCDKPVVMV | 128 | -0.06 | Non Toxic | -0.03 | 0.52 |
KKCDKPVVMV | 128 | -0.06 | Non Toxic | -0.22 | 0.04 |
LKCDKPVVMV | 128 | -0.18 | Non Toxic | -0.05 | 0.09 |
MKCDKPVVMV | 128 | -0.17 | Non Toxic | -0.08 | 0.24 |
NKCDKPVVMV | 128 | 0.12 | Toxic | -0.17 | 0.22 |
PKCDKPVVMV | 128 | 0.03 | Toxic | -0.11 | 0.24 |
QKCDKPVVMV | 128 | 0.01 | Toxic | -0.18 | 0.52 |
RKCDKPVVMV | 128 | -0.00 | Non Toxic | -0.28 | 0.25 |
SKCDKPVVMV | 128 | -0.06 | Non Toxic | -0.13 | 0.18 |
TKCDKPVVMV | 128 | -0.37 | Non Toxic | -0.12 | -0.12 |
WKCDKPVVMV | 128 | 0.06 | Toxic | -0.07 | -0.01 |
YKCDKPVVMV | 128 | 0.21 | Toxic | -0.11 | -0.28 |
VACDKPVVMV | 129 | -0.35 | Non Toxic | 0.08 | -0.33 |
VCCDKPVVMV | 129 | 1.15 | Toxic | 0.06 | 0.07 |
VDCDKPVVMV | 129 | 0.11 | Toxic | -0.01 | 0.07 |
VECDKPVVMV | 129 | 0.04 | Toxic | -0.00 | -0.48 |
VFCDKPVVMV | 129 | -0.04 | Non Toxic | 0.12 | -0.23 |
VGCDKPVVMV | 129 | -0.37 | Non Toxic | 0.07 | -0.28 |
VHCDKPVVMV | 129 | -0.16 | Non Toxic | 0.02 | -0.41 |
VKCDKPVVMV | 129 | -0.33 | Non Toxic | 0.13 | -0.41 |
VLCDKPVVMV | 129 | -0.47 | Non Toxic | 0.11 | -0.36 |
VMCDKPVVMV | 129 | -0.05 | Non Toxic | 0.08 | 0.21 |
VNCDKPVVMV | 129 | -0.24 | Non Toxic | -0.01 | -0.23 |
VPCDKPVVMV | 129 | -0.37 | Non Toxic | 0.05 | -0.21 |
VQCDKPVVMV | 129 | -0.36 | Non Toxic | -0.01 | 0.07 |
VRCDKPVVMV | 129 | -0.26 | Non Toxic | -0.12 | -0.20 |
VSCDKPVVMV | 129 | -0.41 | Non Toxic | 0.03 | -0.27 |
VTCDKPVVMV | 129 | -0.36 | Non Toxic | 0.04 | 0.38 |
VWCDKPVVMV | 129 | -0.03 | Non Toxic | 0.09 | -0.57 |
VYCDKPVVMV | 129 | -0.36 | Non Toxic | 0.06 | -0.46 |
VK*DKPVVMV | 130 | - | - | - | - |
VKC*KPVVMV | 131 | - | - | - | - |
VKCD*PVVMV | 132 | - | - | - | - |
VKCDK*VVMV | 133 | - | - | - | - |
VKCDKP*VMV | 134 | - | - | - | - |
VKCDKPV*MV | 135 | - | - | - | - |
VKCDKPVV*V | 136 | - | - | - | - |
VKCDKPVVM* | 137 | - | - | - | - |
PoPMuSiC is a program that uses database-derived potentials to predict changes in folding free energy (ΔΔGcomputed-ΔGmutant-ΔGwild-type) upon mutations (17). This software was utilized for evaluation of the protein’s mutant stability changes improvement. A list of the most stabilized and destabilized mutants yields through single-site mutations which are shown in Table 6.
Single Site Mutation Effect and Different Stabilized and Destabilized Mutants
Position of Amino Acid | Mutant | Solvent Accessibility, % | ΔΔG |
---|---|---|---|
44 | K → P | 58.02 | 1.9 6 |
64 | L → A | 0.52 | 2.49 |
72 | G → W | 32.92 | - 0.42 |
73 | E → L | 39.74 | -1.01 |
129 | K → L | 29.01 | - 0.76 |
129 | K → F | 29.01 | - 0.27 |
135 | G → Y | 00.00 | - 1.41 |
263 | S → C | 00.00 | - 1.40 |
291 | R → F | 0.20 | - 1.29 |
323 | K → W | 0.89 | - 0.88 |
341 | I → S | 0.83 | 3.39 |
3.3. Bioinformatics Analysis of the Wild Type and Mutant Protein
Following ToxinPred and PoPMuSiC analysis, 6 variants were predicted to be more stable and non-toxic than the wild type. They were selected for Bioinformatics analysis of their stability, solubility, toxicity, secondary structure, 3D structure, energy minimization and other Physico-chemical parameters (Table 7).
Bioinformatic Data of 6 Predicted Variants; Antigenicity of all proteins based on VaxiJen analysis and allergenicity of all proteins based on AlgPred analysis
Variant | Energy Minimizing, kcal/mol | Negative Allergen, % | Positive Allergen, % | Antigenicity | Half Life, h |
---|---|---|---|---|---|
Wild type | -5885.1443 | 67.96 | 85.64 | 0.5043 | 5.5 |
Mut1:K129L | -5961.0957 | 67.96 | 85.64 | 0.5174 | 5.5 |
Mut 2:K129F | -6262.4255 | 67.96 | 85.64 | 0.5213 | 5.5 |
Mut 3: K129Land G135Y | -5967.9277 | 67.96 | 85.64 | 0.5407 | 5.5 |
Mut 4: K129L and S263C | -6002.7220 | 67.96 | 85.64 | 0.5191 | 5.5 |
Mut 5: K129L , S263C and R291F | -6177.2600 | 67.96 | 85.64 | 0.5137 | 5.5 |
Mut 6: L23G, K129L , S263C and R291F | -6491.9938 | 67.96 | 85.64 | 0.5137 | 30 |
Garnier-Osguthorpe-robson (GOR) is a method based on probability parameters which are derived from empirical studies for alpha helix, beta sheet, turn and random coil of protein sequences secondary structure prediction (18). GOR 4 online program analyzes data on secondary structure of the wild type and 6 mutant proteins -which were probably non-toxic and more stable than the wild type illustrated some changes on the secondary structure of all 6 mutants rather than wild type protein.
The 3D modeled structure of wild type protein and six mutants were predicted by I-TASSER server. Iterative threading assembly refinement (I-TASSER) were produced to combine multiple pipelines of ab intio folding, atomic level function and threading refinement to predict full- length 3D structure of proteins. Wild protein and six mutant constructions were visualized with Accelrys Discovery Studio 2.5 software (Figure 1). Output data of Expasy’s ProtParam on Physico-chemical parameters are presented in Table 8.
3D Model Structure of Predicted Protein Illustrated by I-TASSER Software
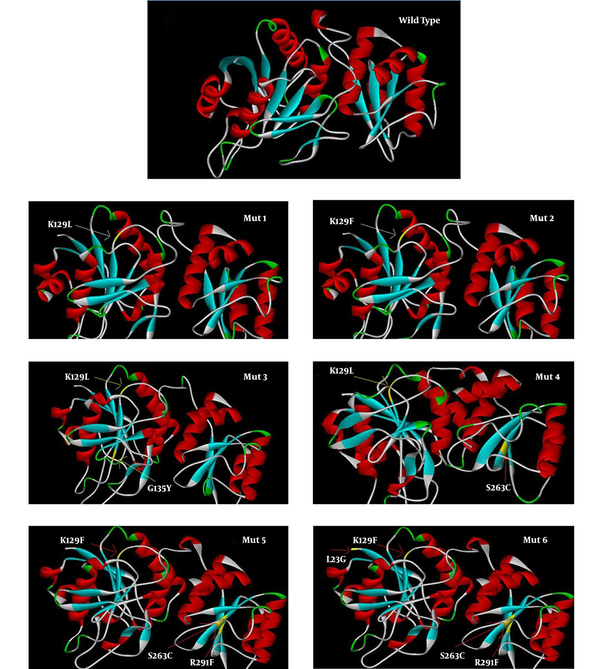
Physico-Chemical Parameters of Protein Extracted from Expasy’s ProtParam
Characteristics | Wild Type | Mut 1 | Mut 2 | Mut 3 | Mut 4 | Mut 5 | Mut 6 |
---|---|---|---|---|---|---|---|
Molecular weight | 34536.8 | 34578.9 | 34612.9 | 34685.0 | 34594.9 | 34585.9 | 34529.8 |
Isoelectric point (pI) | 5.66 | 5.43 | 5.43 | 5.43 | 5.43 | 5.24 | 5.24 |
Total number of positive residues | 33 | 33 | 33 | 33 | 33 | 33 | 33 |
Total number of negative residues | 30 | 29 | 29 | 29 | 29 | 28 | 28 |
Aliphatic indices | 85.17 | 86.10 | 84.91 | 86.10 | 86.10 | 86.10 | 84.91 |
Grand average of Hydropathicity | - 0.197 | -0.174 | -0.177 | -0.177 | -0.164 | -0.142 | -0.155 |
3.4. Predicting Antigens, Allergens and Evaluation of Predicting Structure
VaxiJen server was the first server which was designed for alignment-independent prediction of protective antigens. Using physicochemical properties of protein can classify antigens into different groups without recourse to sequence alignment. AlgPred server predicts allergens based on similarity of known epitope with any region of the protein. By using VaxiJen and AlgPred server, the antigenicity and allergenicity of all proteins were analyzed and positions were identified. Energy minimization of wild type protein and all mutants were calculated by KoBaMIN server and PDB required file for minimum free energy calculating prepared by I-TASSER server (Table 5). Ramachandran plot curve on structural stability of proteins showed in Figure 2.
Ramachandran Plot Curve on Structural Stability of Proteins
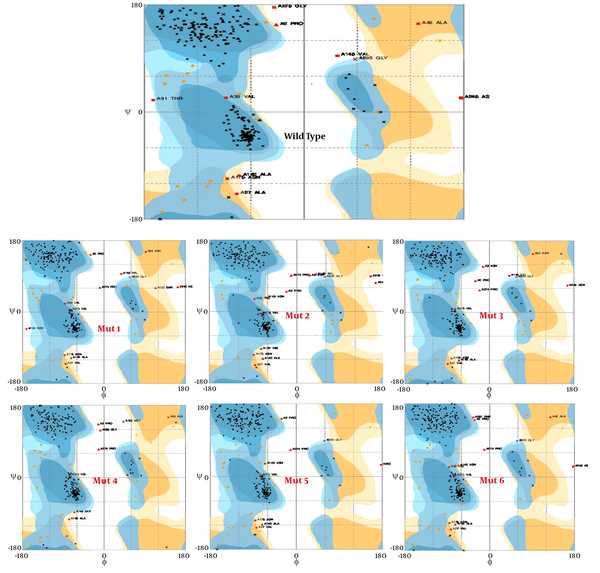
3.5. B-cell’s Epitopes Prediction
Epitope fishing (B cell epitope mapping) is a method to scan proteins for potential epitopes (29). Various factors such as plasticity, antigenicity, exterior accessibility, hydrophilicity and secondary structure were used to predict the protein epitopes. Linear and conformational B-cell epitopes of wild type and 6 mutant’s proteins were analyzed by BCPreds software (Table 9).
B-Cell Epitopes Prediction Based on BCPreds Software Analysisa
Position | Epitope | Score | |
---|---|---|---|
Wild Type | 40 | ATGGTIAGGGDSATKSNYTV | 0.999 |
128 | DKPVVMVGAMRPSTSMSADG | 0.987 | |
183 | TKTNTTDVATFKSVNYGPLG | 0.948 | |
205 | HNGKIDYQRTPARKHTSDTP | 0.923 | |
231 | NELPKVGIVYNYANASDLPA | 0.913 | |
Mutant | |||
1 | 125 | VKCDLPVVMVGAMRPSTSM | 0.978 |
3 | 126 | KCDLPVVMVYAMRPSTSM | 0.948 |
5 | 125 | VKCDLPVVMVGAMRPSTSMS | 0.978 |
2 | 128 | DFPVVMVGAMRPSTSMSADG | 0.966 |
4 | 125 | VKCDLPVVMVGAMRPSTSMS | 0.978 |
6 | 125 | VKCDLPVVMVGAMRPSTSMS | 0.978 |
4. Discussion
The drug made by E. coli L-asparginase II is used as a therapeutic agent in ALL which indicates the importance of these enzymes. Researchers have been studying it intensely for a long time. Some of these studies are about enzyme structure and some of them are about its potency as an antitumor agent. For example, Swain et al. have presented the crystal structure of L-asparginase (4). Barnes et al. have studied the physiology of enzyme synthesis in recombinant E. coli (30). Aghaeepoor et al. showed that the E. coli-drived asparginase production could be increased significantly by manipulating fermentation parameters and by applying innovative purification processes (31). Guo et al. have compared the antitumor activity and the effect of recombinant enzyme both in Vitro and Vivo (32). But this drug has some essential problems such as toxicity and low half-life. The previous studies show that up to 30% of patients experience a hypersensitivity reaction to E. coli L-Asparginase (33). To fix the low half-life problem, scientists like Inada utilizes PolyEthylen Glycol or its derivatives. He has reported a distinct reduction in immunogenicity and clearance rate of E. coli L-Asparginase due to its modification with monomethoxypolyethylen glycol (PEG) (34) and Hernandez et al. introduced E. coli PEG-L-Asparginase as a potent therapeutic approach in ALL (35). Fu and Sakamoto has reviewed PEG-Asparginase features completely in 2007 (36). As a research on physicochemical and biological stability of E. coli L-asparginase, Soares et al. surveyed the effects of PEG attachment to the enzyme (37). And finally Alrazzak et al. indicated that IV administration of PolyEthylen Glycol of asparginase enzyme increases the incidence hypersensitivity reactions compared with IM administration (38).
There is no expanded study of the reduction of the drug’s toxicity without any vital change in protein structure and activity by means of Bioinformatics. Regarding the growth of Bioinformatics and its help to the pharmacology science, we have decided to use this new branch of science in order to decrease the toxicity at first, and then to increase available drug’s half-life and stability. It is very affordable because it reduces the costs of study and produces new variants of drug and most importantly it helps children who suffer from cancer. For this purpose, a series of online software was applied by the study group. “ToxinPred” was being used by colleagues to predict decrement of the drug’s toxicity and “PoPMuSiC” and “kobamin” to predict enzyme’s half-life and energy minimizing. “ToxinPred” has revealed the toxic peptide sequences by its algorithms. Also, it is able to substitute amino acids to overcome toxicity problems. We have identified a high toxicity site that started in position 129 of E. coli L-asparginase as responsible for the toxic properties of the enzyme. Also, with the substitution of toxic peptide’s amino acids with the other ones we have reported the new low and nontoxic peptide sequences. Changing of amino acids in the primary sequence and creating 6 mutant protein had no significant changes in antigenicity and allergenicity of properties of protein. Here are two important points: first of all, using the highest score amino acids to overcome toxicity problems and define the set that is important for us. Secondly, selecting a series of amino acids, which increase enzyme’s stability beside toxicity decrement. Based on these two important factors, K129L and K129F have been chosen. “PoPMuSiC” software is almost similar to “ToxinPred” which has been mentioned before, and the difference is in protein stabilization prediction. The validity of the software was proved during “Bottomley” and colleagues study in 2007 (39).
K129L and S263C and K129L, S263C and R291F that in addition to stabilizing, they lead to nontoxic proteins. Due to N-terminal property of proteins, which reduce or increase their half-life, we substituted Leucine with Glycine in position 23 of proteins that increase half-life from 5.5 hours to 30 hours. After these changes it is necessary to evaluate physicochemical, secondary and tertiary structure of new protein to prove the correctness of prediction and keep the protein active site away from malformation. For this purpose, we utilized a number of software programs mentioned earlier.
4.1. Conclusions
As promising results of the analysis all predictions not only change active protein’s structure, but also enhance its half-life and stability and decrease its toxicity by the means of ΔG minimizing. Of all 6 sequences which we reached at the end of the analysis, sequences number 1 and 2 were the only produced non-toxic proteins; numbers 3, 4 and 5 produced both non-toxic and more stable protein and finally sequence number 6 was the only sequence with all distinct characteristics: non-toxic, more stability and more half life without addition of any components.
References
-
1.
Pui CH, Evans WE. Acute lymphoblastic leukemia. N Engl J Med. 1998;339(9):605-15. [PubMed ID: 9718381]. https://doi.org/10.1056/NEJM199808273390907.
-
2.
McEntee M, Silverman JA, Rassnick K, Zgola M, Chan AO, Tau PT, et al. Enhanced bioavailability of oral docetaxel by co-administration of cyclosporin A in dogs and rats. Vet Comp Oncol. 2003;1(2):105-12. [PubMed ID: 19379322]. https://doi.org/10.1046/j.1476-5829.2003.00015.x.
-
3.
Rahbar MR, Rasooli I, Mousavi Gargari SL, Amani J, Fattahian Y. In silico analysis of antibody triggering biofilm associated protein in Acinetobacter baumannii. J Theor Biol. 2010;266(2):275-90. [PubMed ID: 20600143]. https://doi.org/10.1016/j.jtbi.2010.06.014.
-
4.
Swain AL, Jaskolski M, Housset D, Rao JK, Wlodawer A. Crystal structure of Escherichia coli L-asparaginase, an enzyme used in cancer therapy. Proc Natl Acad Sci U S A. 1993;90(4):1474-8. [PubMed ID: 8434007].
-
5.
Cooney DA, Handschumacher RE. L-asparaginase and L-asparagine metabolism. Annu Rev Pharmacol. 1970;10:421-40. [PubMed ID: 4911021]. https://doi.org/10.1146/annurev.pa.10.040170.002225.
-
6.
Whitecar JJ, Bodey GP, Hill CJ, Samaan NA. Effect of L-asparaginase on carbohydrate metabolism. Metabolism. 1970;19(8):581-6. [PubMed ID: 4913910].
-
7.
Yun MK, Nourse A, White SW, Rock CO, Heath RJ. Crystal structure and allosteric regulation of the cytoplasmic Escherichia coli L-asparaginase I. J Mol Biol. 2007;369(3):794-811. [PubMed ID: 17451745]. https://doi.org/10.1016/j.jmb.2007.03.061.
-
8.
Haskell CM, Canellos GP, Leventhal BG, Carbone PP, Block JB, Serpick AA, et al. L-asparaginase: therapeutic and toxic effects in patients with neoplastic disease. N Engl J Med. 1969;281(19):1028-34. [PubMed ID: 4898857]. https://doi.org/10.1056/NEJM196911062811902.
-
9.
Capizzi RL. Asparaginase revisited. Leuk Lymphoma. 1993;10 Suppl:147-50. [PubMed ID: 8481663]. https://doi.org/10.3109/10428199309149127.
-
10.
Li LZ, Xie TH, Li HJ, Qing C, Zhang GM, Sun MS. Enhancing the thermostability of Escherichia coli l-asparaginase II by substitution with pro in predicted hydrogen-bonded turn structures. Enzyme Microb Technol. 2007;41(4):523-7. https://doi.org/10.1016/j.enzmictec.2007.04.004.
-
11.
Luscombe NM, Greenbaum D, Gerstein M. What is bioinformatics? An introduction and overview. Yearb Med Inform. 2001;(1):83-99. [PubMed ID: 27701604].
-
12.
Blundell TL, Sibanda BL, Montalvao RW, Brewerton S, Chelliah V, Worth CL, et al. Structural biology and bioinformatics in drug design: opportunities and challenges for target identification and lead discovery. Philos Trans R Soc Lond B Biol Sci. 2006;361(1467):413-23. [PubMed ID: 16524830]. https://doi.org/10.1098/rstb.2005.1800.
-
13.
Wheeler DL, Barrett T, Benson DA, Bryant SH, Canese K, Chetvernin V, et al. Database resources of the National Center for Biotechnology Information. Nucleic Acids Res. 2007;35(Database issue):D5-12. [PubMed ID: 17170002]. https://doi.org/10.1093/nar/gkl1031.
-
14.
Chenna R, Sugawara H, Koike T, Lopez R, Gibson TJ, Higgins DG, et al. Multiple sequence alignment with the Clustal series of programs. Nucleic Acids Res. 2003;31(13):3497-500. [PubMed ID: 12824352].
-
15.
Bairoch A, Apweiler R, Wu CH, Barker WC, Boeckmann B, Ferro S, et al. The Universal Protein Resource (UniProt). Nucleic Acids Res. 2005;33(Database issue):D154-9. [PubMed ID: 15608167]. https://doi.org/10.1093/nar/gki070.
-
16.
Gupta S, Kapoor P, Chaudhary K, Gautam A, Kumar R, Open Source Drug Discovery C, et al. In silico approach for predicting toxicity of peptides and proteins. PLoS One. 2013;8(9). e73957. [PubMed ID: 24058508]. https://doi.org/10.1371/journal.pone.0073957.
-
17.
Gilis D, Rooman M. PoPMuSiC, an algorithm for predicting protein mutant stability changes: application to prion proteins. Protein Eng. 2000;13(12):849-56. [PubMed ID: 11239084].
-
18.
Garnier J, Gibrat JF, Robson B. GOR method for predicting protein secondary structure from amino acid sequence. Academic Press; 1996. https://doi.org/10.1016/s0076-6879(96)66034-0.
-
19.
Du H, Brender JR, Zhang J, Zhang Y. Protein structure prediction provides comparable performance to crystallographic structures in docking-based virtual screening. Methods. 2015;71:77-84. [PubMed ID: 25220914]. https://doi.org/10.1016/j.ymeth.2014.08.017.
-
20.
Bridge A, Lane L, Stockinger H, Appel R, Xenarios I. Databases and Datasources at SIB, Swiss Institute of Bioinformatics. Oxford: Elsevier; 2014. https://doi.org/10.1016/b978-0-444-53632-7.01124-2.
-
21.
Mohabatkar H, Kar SK. Prediction of exposed domains of envelope glycoprotein in Indian HIV-1 isolates and experimental confirmation of their immunogenicity in humans. Braz J Med Biol Res. 2004;37(5):675-81. [PubMed ID: 15107929].
-
22.
Doytchinova IA, Flower DR. VaxiJen: a server for prediction of protective antigens, tumour antigens and subunit vaccines. BMC Bioinformatics. 2007;8:4. [PubMed ID: 17207271]. https://doi.org/10.1186/1471-2105-8-4.
-
23.
Gould HJ, Sutton BJ, Beavil AJ, Beavil RL, McCloskey N, Coker HA, et al. The biology of IGE and the basis of allergic disease. Annu Rev Immunol. 2003;21:579-628. [PubMed ID: 12500981]. https://doi.org/10.1146/annurev.immunol.21.120601.141103.
-
24.
Saha S, Raghava GP. AlgPred: prediction of allergenic proteins and mapping of IgE epitopes. Nucleic Acids Res. 2006;34(Web Server issue):W202-9. [PubMed ID: 16844994]. https://doi.org/10.1093/nar/gkl343.
-
25.
Rodrigues JP, Levitt M, Chopra G. KoBaMIN: a knowledge-based minimization web server for protein structure refinement. Nucleic Acids Res. 2012;40(Web Server issue):W323-8. [PubMed ID: 22564897]. https://doi.org/10.1093/nar/gks376.
-
26.
El-Manzalawy Y, Dobbs D, Honavar V. Predicting linear B-cell epitopes using string kernels. J Mol Recognit. 2008;21(4):243-55. [PubMed ID: 18496882]. https://doi.org/10.1002/jmr.893.
-
27.
Kringelum JV, Lundegaard C, Lund O, Nielsen M. Reliable B cell epitope predictions: impacts of method development and improved benchmarking. PLoS Comput Biol. 2012;8(12). e1002829. [PubMed ID: 23300419]. https://doi.org/10.1371/journal.pcbi.1002829.
-
28.
Cho A, Seife C, Lawler A, Leggett A, Dunningham J, Rau A. Robert Coontz, Ian Osborne, and Phil Szuromi Science. Science. 2005;11:865.
-
29.
De Groot AS, Berzofsky JA. From genome to vaccine--new immunoinformatics tools for vaccine design. Methods. 2004;34(4):425-8. [PubMed ID: 15542367]. https://doi.org/10.1016/j.ymeth.2004.06.004.
-
30.
Barnes WR, Vela GR, Dorn GL. Physiology of L-asparaginase synthesis in recombinants of Escherichia coli A-1. Appl Environ Microbiol. 1978;35(4):766-70. [PubMed ID: 25625].
-
31.
Aghaeepoor M, Mozafari S, Shahraki MK, Tabandeh F, Bambai B. High level of extracellular fermentation and alternative purification of Escherichia coli asparaginase II. Biharean Biol. 2011;5(2):96-101.
-
32.
Guo QL, Wu MS, Chen Z. Comparison of antitumor effect of recombinant L-asparaginase with wild type one in vitro and in vivo. Acta Pharmacol Sin. 2002;23(10):946-51. [PubMed ID: 12370101].
-
33.
Vrooman LM, Kirov ,I, Dreyer ZE, Kelly M, Hijiya N, Brown P, et al. Activity and Toxicity of Intravenous Erwinia Asparaginase Following Allergy to E. coli-Derived Asparaginase in Children and Adolescents With Acute Lymphoblastic Leukemia. Pediatr Blood Cancer. 2016;63(2):228-33. [PubMed ID: 26376459]. https://doi.org/10.1002/pbc.25757.
-
34.
Yoshimoto T, Nishimura H, Saito Y, Sakurai K, Kamisaki Y, Wada H, et al. Characterization of polyethylene glycol-modified L-asparaginase from Escherichia coli and its application to therapy of leukemia. Jpn J Cancer Res. 1986;77(12):1264-70. [PubMed ID: 3102431].
-
35.
Hernandez CP, Morrow K, Lopez-Barcons LA, Zabaleta J, Sierra R, Velasco C, et al. Pegylated arginase I: a potential therapeutic approach in T-ALL. Blood. 2010;115(25):5214-21. [PubMed ID: 20407034]. https://doi.org/10.1182/blood-2009-12-258822.
-
36.
Fu CH, Sakamoto KM. PEG-asparaginase. Expert Opin Pharmacother. 2007;8(12):1977-84. [PubMed ID: 17696798]. https://doi.org/10.1517/14656566.8.12.1977.
-
37.
Soares AL, Guimaraes GM, Polakiewicz B, de Moraes Pitombo RN, Abrahao-Neto J. Effects of polyethylene glycol attachment on physicochemical and biological stability of E. coli L-asparaginase. Int J Pharm. 2002;237(1-2):163-70. [PubMed ID: 11955814].
-
38.
Alrazzak M, Beaupin LK, Kinyoun P, Barth M. The Incidence of Hypersensitivity Reactions to Pegylated Asparaginase in Children With Acute Lymphoblastic Leukemia: A City-wide Experience. J Pediatr Hematol Oncol. 2016;38(1):e16-20. [PubMed ID: 26558809]. https://doi.org/10.1097/MPH.0000000000000465.
-
39.
Cabrita LD, Gilis D, Robertson AL, Dehouck Y, Rooman M, Bottomley SP. Enhancing the stability and solubility of TEV protease using in silico design. Protein Sci. 2007;16(11):2360-7. [PubMed ID: 17905838]. https://doi.org/10.1110/ps.072822507.