1. Background
Breast cancer is a multi-stage cancer, which is curable if diagnosed in early stages (1). In the 21st century, it seems that we are witnessing a progressive decline in deaths from the disease, especially in developed countries (2). Breast cancer is the second most common cancer worldwide and the fourth cause of death from cancer. Breast cancer is the most common cancer among women; 1.67 million new cases of cancer have been diagnosed in 2012 (3). In Iran, breast cancer is the most prevalent cancer (23% of all cancers) among women (4). Progress in the field of cancer is one of the main goals of health care programs (5). In oncology studies, classifying a quantitative prognostic variable or determining cut point is requested to categorize individuals into low-risk and high-risk classes. Therefore, homogenous groups of patients are created. Such categories are useful for treatment recommendations, clinical trial design, and treatment improvement planning (6, 7). There are multiple methods to categorize a quantitative variable or determine cut point such as using the sample quantiles (median), the optimal change point, change-point method, etc. (7, 8). Multiple studies have been conducted to determine the cut point, using change-point models. Tashima et al. (2015) conducted a study to determine ki-67 cut point in patients with breast cancer. The article aims at introducing ki-67 indicator as a more accurate diagnostic factor. In this study, comparison of P values and hazard ratios in univariate and multivariate cox proportional hazards (Cox PH) model and were employed to determine the optimal cut point (9). Mohsin et al. (2004) conducted a study in patients with breast cancer to develop and validate immune histochemical assay method.In this study, minimum P value method was used to determine the optimal cut point for positive progesterone receptor (10). Lopez et al. (2010) conducted carried out a study to update changes in colon cancer mortality and incidence. In this study, transition change-point model was used. They concluded that the incidence of colorectal cancer has increased markedly compared to the past. The increased incidence and mortality rates were in opposite direction (11). Since breast cancer is one of preventable diseases with screening (1), knowing change point, biological, and prognostic characteristics are highly regarded in a screening planning policy making (5). Therefore, in this study, change-point cure model is employed to estimate change point (cut point) in diagnosis age of breast cancer.
This model was introduced by Othus et al. in order to estimate the change point in a quantitative variable in long-term survival data (12). In this model, change point in breast cancer age is obtained by dividing likelihood function of a non-mixture cure model into two sections (prior to and after change point). Cure model is used because using standard models such as semi-parametric Cox PH models are not appropriate in survival studies if there are long-term or cured individuals. These models assume that all individuals would experience the event (13, 14).
Therefore, cure models are used in such cases. Some factors have been introduced as survival prognostic factors in breast cancer, including higher stage of disease, number of lymph nodes involved in cancer, pathology, socioeconomic status, type of treatment, etc. (15). These factors need to be taken into account in determining a prognostic model. Disease free survival (DFS) time is one of the most common criteria to evaluate the impact of various therapeutic agents in patients. DFS time is defined as duration from the onset of sickness to event (death or recurrence) (16).
This article aims at estimating the change point of cancer diagnosis age, using change point cure model with some predictive factors including level of education, estrogen receptor, lymph node involvement, etc. which are effective factors in survival. It also studies the concurrent effect of predictive factors prior to and after change point on cure rate. Determining cut point and distribution and relationship between variables and event prior to and after this point can be a valuable solution for clinicians and health policy makers to develop a cost-effective treatment guideline in patients diagnosed with breast cancer.
2. Methods
In this longitudinal study, 559 patients with breast cancer referring to breast cancer research center, Tehran, Iran from 1986 to 2006 entered the study. The patients were re-followed in 2013. Demographic information and clinical documentation were completed. Their survival was recorded through telephone conversation. The date of cancer diagnosis was considered the onset of disease. DFS time was calculated based on the telephone conversation follow-up. Change point cure model was employed to determine a cut point in age. The method used in this article is according to a new change point model introduced by Othus et al. (12). In that study, introduced likelihood function was tested by a simulation study and goodness of fit of the model was tested. Then, the model was used to determine a cut point in diagnosis age of prostate cancer (12). In exponential cure model, cut point of breast cancer diagnosis age was estimated, using some predictive factors, including level of education, lymph node involvement, tumor size, status of estrogen and progesterone receptors, type of surgery, and chemotherapy and radiotherapy, which are effective factors in survival.
2.1. Mixture Cure Model with Covariates
In mixture cure model, it is assumed that the population consists of two groups (cured and non-cured) and survival function of mixture cue model is defined based on this assumption. Survival function is as follows:

SU (t) is survival function for non-cured patients. For this model, all individuals are alive at t = 0 (S (0) = 1). At infinite, a proportion of individuals is cured (S (∞) = p). In other words, some do not experience event (here recurrence of cancer). The presence of individuals with long-term survival makes the Kaplan-Meier curves not to reach zero at the end.
It remains horizontally constant. Prior to using cure model, two pre-assumptions need to be studied (presence of cured people and sufficient length of follow up). In case of using covariates (X), logistic link function is used as follows (14, 17, 18).
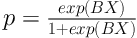
Where β Shows the effect of covariates on chance of cure. So, eβ shows odds ratio for covariate X.
2.2. Change Point Cure Model with Covariates
Mixture cure model with a change point at a quantitative variable: In this model, likelihood function of mixture cure model is divided into two sections (prior to and after change point) and change point of X quantitative variable is estimated.
In a mixture cure model with change point at a covariate variable, density function is written as follows:

Where f (t) is density function of time variable until the event before and after change point parameter, τ is change point parameter for X quantitative variable, S (t) is survival function of time variable until the event before and after change point, d is status variable, censor for each individual so that in case of event, d = 1, if censored, then, d = 0. Above function is not smooth.
Therefore, final likelihood function is as follows after smoothing (12) Figure 1.
Maximum likelihood method and numerical search methods are employed to estimate the change-point model, including β coefficient and τ change-point parameter.
2.3. Data Analysis
SPSS v.16 was employed to draw KM curve. R and Stats4 package were employed to estimate the change-point model parameters. First, the appropriateness of cure model was studied, using likelihood ratio test. Then, likelihood function was written for change-point cure model with covariates. Finally, maximum likelihood estimation was carried out, using numerical search methods.
This study was approved by ethics committee of Tarbiat Modares University.
3. Results
A total of 559 women entered the study. The mean diagnosis age of patients, who were analyzed, was reported 46.31 ± 11.17 years. Patients’ age varied between 24 and 83 years.Mean and median time of follow-up was 81.43 ± 55.72 and 68.36 months, receptively. The range of changes was 0.89 - 324. Totally, 179 (32%) deaths occurred and the rest were considered right censored.
Table 1 shows the demographic characteristics of patients who were analyzed. As it can be seen, almost 51% (287 patients) had level of education lower than diploma; 64% (358 patients) experienced lymph node involvement. The mean size of tumor was 2.17 ± 1.05 cm; 83.4% of patients (316 patients) underwent modified radical mastectomy (MRM) and the rest of patients experienced breast preservation (BP). As much as 56% (316 patients) had positive estrogen receptor. The mean tumor size, who underwent MRM, was 2.26 ± 1.09 cm. It was reported 1.71 ± 0.73 in women with breast preservation.
Characteristics of Patients | No. (%) |
---|---|
Education | |
Lower than diploma | 287 (51) |
Diploma and higher | 272 (49) |
Estrogen receptor | |
Yes | 316 (56) |
No | 243 (44) |
Surgery type | |
Modified Radical Mastectomy | 466 (83.4) |
Breast preservation | 93 (16.60) |
Lymph node involvement | |
Yes | 358 (64) |
No | 201 (36) |
Demographic, Clinical, and Health Characteristics of Patient
Figure 2 shows Kaplan-Meier curve. As it can be seen in the curve, the graph becomes flat after 17 years. It did not reach zero, though. Almost 40% of patients survived until the end of study. The presence of cured people and sufficient length of follow-up were studied to use cure model. Generally, 6 to 17 years follow-up simply shows that it is long enough. Likelihood ratio test for exponential model (null hypothesis model) and exponential cure model (alternative hypothesis) were employed to study the presence of cured patients. Since likelihood ratio statistics (with chi-square distribution and degree of freedom of 1) is 7 (greater than 3.8), the presence of cured patients was verified. Change point cure model was used to obtain change point in breast cancer diagnosis age.
Table 2 shows the change point model fitting results. Confidence interval for OR was calculated, using the following formula. The results indicate that breast cancer change-point age is 49.45 (± 0.64) years. In this study, lower than diploma, lack of lymph node involvement, Breast Preservation surgery, and negative estrogen receptor were considered as reference group. The results of cure change-point model indicate that the chance of cure was 50% less in younger group with lymph node than those without lymph node and this variable was not significant. However, in older group, the chance of cure declined by 61% and it was significant. In younger group, one unit of tumor size increase led to 57% decline in chance of cure. In older group, one unit in tumor size increase led to 51% decline in chance of cure and tumor size variable was significant in both age groups. Level of education, type of surgery, and estrogen receptor variables were not significant in none of age groups. In younger group, the chance of cure was 8% lower in diploma and higher level of education group than that of lower-than-diploma group. In older group, however, the chance of cure was 56% higher. In younger group, the chance of cure in MRM surgery was 1.3 times as much as BP surgery. In older group, the chance of cure was twice. In younger group, the chance of cure was 50% higher in positive estrogen receptor than negative estrogen receptor. This chance was 64% lower in older group.
Variable | OR | CI (95%) |
---|---|---|
Younger group (age ≤ 50) | ||
Hazard rate | 0.008 | (0.005, 0.011) |
Education | (0.413, 1.952) | |
Diploma and higher | 0.917 | |
Lower than diploma | Reference | |
Lymph node involvement | ||
Yes | 0.520 | (0.236, 1.145) |
No | Reference | |
Tumor size | 0.430 | (0.235, 0.787) |
Surgery type | (0.453, 3.803) | |
Modified Radical Mastectomy | 1.313 | |
Breast preservation | Reference | |
Estrogen receptor | (0.748, 3.321) | |
Yes | 1.576 | |
No | Reference | |
Older group (age > 50) | ||
Hazard rate | 0.019 | (0.012, 0.027) |
Education | 0.019 | (0.012, 0.027) |
Diploma and higher | 1.560 | (0.632, 3.853) |
Lower than diploma | Reference | |
Lymph node involvement | (0.168, 0.941) | |
Yes | 0.397 | |
No | Reference | |
Tumor size | 0.496 | (0.313, 0.785) |
Surgery type | (0.697, 6.276) | |
Modified Radical Mastectomy | 2.093 | |
Breast preservation | Reference | |
Estrogen receptor | (0.329, 1.701) | |
Yes | 0.748 | |
No | Reference | |
Change point at age of cancer diagnosis | 49.445 | (48.131, 50.759) |
Parameter Estimations and Confidence Intervals in Change Point Exponential Cure Model
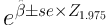
4. Discussion
This study aimed at determining the cut point of breast cancer diagnosis age with covariates. The cut point was obtained 49.45. The results also showed that tumor size increase in young group and lymph node involvement in old group declined the chance of recovery. Determining cut point in a quantitative variable or categorizing a quantitative variable is widely used in different sciences. Contal et al. (1999) employed two different methods in semi-parametric Cox survival model to determine the cut point of breast cancer diagnosis age. They obtained the 41 and 37 year-old cut point by these two methods, respectively which is inconsistent with this study (19). Different cut points have been employed in studies concerning breast cancer. For example, 50 cut point (20-22) were employed in some studies conducted in the USA and Spain, which is line with the present study. Some studies used different cut points for age (15, 23, 24). The cut points used in these studies are not consistent with this study. This difference might be associated with different statistical population and different methods to obtain cut point.
In Li Christopher et al.’s study that reviewed multiple studies concerning breast cancer, the cut point of 50 years was introduced as prognostic factor. The survival probability of patients older than 50 years is 70% to 85%, while it is 55% to 80% for patients younger than 50 years. In this study, tumor size and lymph node involvement have been suggested as prognostic survival factors in younger-than-50 age groups; however, in addition to tumor size, lymph node involvement is considered as a lower survival prognostic factor in older-than-50 age group. Individuals who receive positive estrogen and progesterone have higher chance of survival due to effectiveness of wide variety of hormonal treatments compared to those who are negative receptors (15).
In different studies, some factors are considered prognostic ones such as younger age, comorbidity, larger tumor size, negative estrogen receptor, higher number of lymph node involvement that in hazard estimation models, a significant relationship is found with relapse (15, 25). In this study, lymph node involvement in older-than-50 age group displayed a significant relationship with survival. But, this relationship was not significant in younger ages at error of 5%. In a study conducted by Wildiers et al. the probability of lymph node involvement declines until 70 years old. This probability, however, rises after 70, which is consistent with this study (26). Using different cut points and lack of relationship between survival prognostic variables and adjustment of effect of age can be associated with lack of evidence. Mamunas raised the same criticism regarding this probability (25). In this study, we obtained change point for breast cancer diagnosis age, using change point mixture cure model.
Considering the nature of long-term survival data or presence of cured patients in the study is one of the advantages of this model. We were also able to estimate and compare the covariate-related parameters affecting survival in both age categories. Determining change point is carried out, using univariate models. But, change point might also depend on other risk factors (6). For instance, family history of cancer is an important factor to develop breast cancer incidence (1). Having family history helps obtain the change point of cancer age in each group separately. Inaccessibility to some information such as family history is considered a limitation. To generalize the model, other parametric models such as Weibull and log-logistic model can be employed for fitting.
The results of the study show that the effect of age is adjusted at 50 years old in prognosis of patients with breast cancer, creating two relatively homogeneous groups. This cut point can be useful to evaluate various predictive and prognostic factors of breast cancer. The difference in the effect between tumor size and lymph node involvement in different age groups can be helpful in determining more appropriate therapeutic strategies.