Abstract
Objectives:
This study aimed to provide an overview of prediction models of undiagnosed type 2 diabetes mellitus (U-T2DM) or the incident T2DM (I-T2DM) using the transparent reporting of a multivariable prediction model for individual prognosis or diagnosis (TRIPOD) checklist and the prediction model risk of the bias assessment tool (PROBAST).Data Sources:
Both PUBMED and EMBASE databases were searched to guarantee adequate and efficient coverage.Study Selection:
Articles published between December 2011 and October 2019 were considered.Data Extraction:
For each article, information on model development requirements, discrimination measures, calibration, overall performance, clinical usefulness, overfitting, and risk of bias (ROB) was reported.Results:
The median (interquartile range; IQR) number of the 46 study populations for model development was 5711 (1971 - 27426) and 2457 (2060 - 6995) individuals for I-T2DM and U-T2DM, respectively. The most common reported predictors were age and body mass index, and only the Qrisk-2017 study included social factors (e.g., Townsend score). Univariable analysis was reported in 46% of the studies, and the variable selection procedure was not clear in 17.4% of them. Moreover, internal and external validation was reported in 43% the studies, while over 63% of them reported calibration. The median (IQR) of AUC for I-T2DM models was 0.78 (0.74 - 0.82); the corresponding value for studies derived before October 2011 was 0.80 (0.77 - 0.83). The highest discrimination index was reported for Qrisk-2017 with C-statistics of 0.89 for women and 0.87 for men. Low ROB for I-T2DM and U-T2DM was assessed at 18% and 41%, respectively.Conclusions:
Among prediction models, an intermediate to poor quality was reassessed in several aspects of model development and validation. Generally, despite its new risk factors or new methodological aspects, the newly developed model did not increase our capability in screening/predicting T2DM, mainly in the analysis part. It was due to the lack of external validation of the prediction models.Keywords
Type 2 Diabetes Prediction Models Systematic Review TRIPOD PROBAST
1. Context
Type 2 diabetes mellitus (T2DM) is a major cause of blindness, kidney failure, heart attacks, stroke, and death worldwide (1, 2). The global prevalence (95% CI) of T2DM in adults aged 20 - 79 years was estimated to be 8.8% (7.2 - 11.3%) in 2017, and it is estimated that 50% of them are unaware of their disease. This prevalence is estimated to increase by 48% in 2045. The total healthcare expenditures for diabetes care worldwide were estimated to be $727 billion in 2017 and are expected to increase by 6.7% in 2045 (2). Thus, it is essential to early identify those at high risk of T2DM.
Prediction models could be useful to estimate the probability of screening undiagnosed type 2 diabetes mellitus (U-T2DM) or predicting newly diagnosed T2DM in the future (3). Various prediction models have been developed during the past decades to predict the incident T2DM (I-T2DM). Well-known examples include the Finnish Diabetes Risk score (4), the Australian type 2 diabetes risk (5), QRISK (6), and the Framingham Offspring (FOS) risk (7). The self-assessment screening score proposed by the American diabetes association is included in the 2018 clinical guideline to detect U-T2DM (1).
A multivariable prediction model is a mathematical formula that combines several predictors to estimate individuals’ risk probability. The model-building strategy needs to be explicitly stated to improve the reporting of the prediction models. The previous review (8, 9) has shown that published papers highlight some methodological requirements. However, prediction models’ design, methods, and results have been less frequently reported. Most prediction models are rarely used because of methodological issues in model development and poor or unknown internal and external validity (8, 10).
2. Objectives
The prevalence and incidence of T2DM are increasing, and since about 50% of patients are unaware of their disease (2), prediction models could be used to lower the rate of undiagnosed diabetes. Due to the existing limitations in the prediction models’ reporting strategies, the transparent reporting of a 22-item multivariable prediction model for individual prognosis or diagnosis (TRIPOD) statement was published in 2015 (11). The risk of bias (ROB) assessment tool in line with the TRIPOD statement was proposed in 2019. Since these tools did not evaluate previous studies, we extended previous systematic reviews in the field by focusing on prediction models’ methodological aspects using the TRIPOD checklist for T2DM diagnosis or prognosis, including both previously and newly published articles.
3. Methods
3.1. Data Sources
We followed the critical appraisal and data extraction for systematic reviews of prediction modeling studies (CHARMS) standard checklist for diagnostic and prognostic prediction models, tools, or scores of T2DM (11). For avoiding duplication, only papers published between December 2011 and October 2019 were considered. Both PUBMED and EMBASE databases were searched to guarantee adequate and efficient coverage. Articles published before 2011 were addressed in previously published systematic reviews (8, 9). We included additional articles by searching references in the papers following the same search strategy.
3.2. Study Selection
Observational studies were included to predict U-T2DM or I-T2DM. We also considered studies based on the inclusion and exclusion criteria:
1) Original English articles were included.
2) Articles on gestational diabetes or type 1 DM were excluded.
3) Genetic studies, animal studies, validation studies of previously published models, studies on children or adolescents, studies with a specific population, pre-selected risk factors, and non-regression models, and articles with T2DM as a composite outcome with other outcomes (e.g., cardiovascular disease: CVD) were excluded.
This review focused on regression-based prediction models, and other prediction models such as machine learning models were excluded.
4) Editorial articles, letters, congress abstracts, clinical trials, meta-analysis, or systematic review articles were also removed.
5) The study search strategy included T2DM, undiagnosed diabetes, risk prediction, prediction models, and predictive models.
The search strategy is available in Appendix 1 in Supplementary File.
3.3. Data Extraction
Search results from different origins were combined in a single Endnote library, and duplicate articles were removed electronically and manually. Afterward, two people (S. Asgari and D. Khalili) evaluated titles and abstracts separately and marked potentially related articles for full-text reading. Disagreements were discussed with a third reviewer (F. Hadaegh). All the authors screened full-text articles. One of the reviewers (S. Asgari) extracted data. Three independent people (D. Khalili, F. Hosseinpanah, and F. Hadaegh) monitored the data collection process. Essential items extracted via a literature study included study type (case-control or cohort), country, publication year, study name, sample size, follow-up duration, participant age, and outcome definition. For model development, modeling methods (e.g., logistic regression and survival regression), variable selection methods (e.g., univariate analysis and literature review), treatment of continuous risk predictors (e.g., all categorized, all continue), treatment of missing data (e.g., imputation and complete case), risk predictors in the model, discrimination measures (e.g., sensitivity, specificity, positive or negative predictive value, Youden index, are-under-the-curve: AUC, C-statistics, and D-statistics), overall performance (e.g., Akaike information criteria: AIC and Bayesian information criteria: BIC), clinical usefulness (e.g., net benefit) and overfitting (e.g., bootstrapping) were extracted. Additionally, discrimination measurements, overall performance, and calibration of both internal and external validation were evaluated. We treated prediction models described in a single article as separate models.
3.4. Risk of Bias Assessment
The prediction studies were critically assessed by the Prediction Model Risk of Bias Assessment tool (PROBAST), which was introduced by Wolff et al. in 2019 (12). The risk of bias (ROB) tool is categorized into four domains, including participants (two questions), predictors (three questions), outcome (six questions), and analysis (nine questions). ROB was reported for each article separately to screen U-T2DM and I-T2DM. The overall judgment was performed as recommended by Wolff et al. (12). ROB was defined low if all the four domains were rated low. ROB was defined high if at least one (≥ 1) had high ROB. Also, even if all the domains were defined low, a prediction model without any external validation was judged to have high ROB. Unclear ROB was defined if at least one domain had unclear ROB and it was low risk for all the other domains. The applicability of the prediction models was also assessed, and the majority of the models regarding risk of bias.
3.5. Descriptive Analysis
We summarized the results using descriptive statistics for both model development and validation for I-T2DM. Collins et al. (8) and Noble et al. (9) considered the same characteristics for previously published reviews. The present study evaluated 18 out of the 45 studies on risk prediction (Appendix 2 in Supplementary File).
This systematic review was reported in accordance with the Preferred Reporting Items for systematic reviews and meta-analyses extension for scoping reviews (PRISMA-ScR) (13) by removing meta-analysis items. We also considered the TRIPOD guideline (14) to extract the prediction models’ required items.
4. Results
4.1. General Study Description
The search string retrieved 464 articles in PubMed and 600 articles in EMBASE. After removing duplicates, our database search yielded 755 articles. We excluded 667 articles after checking titles/abstracts and 54 articles after full-text consideration; the remaining 34 articles met the inclusion criteria. A further nine articles were also included by hand searching reference lists. In total, 24 articles on I-T2DM (15-38) and 19 articles on U-T2DM screening (39-57) published between December 2011 and October 2019 were eligible for the current review (Figure 1). For U-T2DM, two articles reported separate risk diagnosis models with different populations. Thus, our review assessed 46 risk prediction models from 43 articles.
The flowchart of study selection between November 2011 and 2019
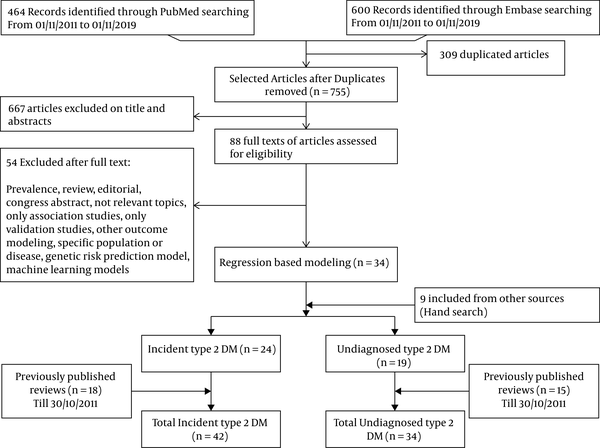
Appendices 3 and 4 show basic information of studies for I-T2DM and U-T2DM, respectively, including publication year, country, study design, study name, number of events and sample size (model development), follow-up duration, participant age, outcome definition, and the Newcastle-Ottawa scale. I-T2DM models have been developed in nine countries, while U-T2DM has been developed in 15 countries (Appendix 12 in Supplementary File). One article described the development of three risk models for U-T2DM screening using three different populations from different countries (44).
The median (interquartile range; IQR) number of the study population for model development was 5711 (1971 - 27426) and 2457 (2060 - 6995) individuals for I-T2DM and U-T2DM, respectively. The most frequent age range in the reviewed articles for both I-T2DM and U-T2DM was 40 years and older. Moreover, the median (IQR) number of the incident case of T2DM was 396 (171 - 1218) whereas the median (IQR) number of prevalent cases for U-T2DM screening was 207 (144 - 388). In 10 articles (17, 19, 20, 22, 26, 30-32, 35, 38) on I-T2DM and one article on U-T2DM (51), the study population was over 10,000 (Appendices 3 and 4 in Supplementary File).
4.2. Model Development
A summary and detailed characteristics of model development for I-T2DM are reported in Table 1 and Appendix 5 in Supplementary File, respectively. Moreover, the detailed characteristics of model development for U-T2DM screening are shown in Appendix 6 in Supplementary File.
Model Development Characteristics for the Current and Previous Reviews for incident Type 2 Diabetes Mellitus
Updated Review (Current Review = 24) | Previous Reviews Collins et al. (8) and Noble et al. (9) (Risk Prediction Modelsa = 18) | |
---|---|---|
Treatment of continuous variables | ||
All kept continuous | 4 | 3 |
All categorized | 18 | 11 |
Some continuous and some categorized | 2 | 4 |
No information | - | - |
Treatment of missing data | ||
Complete case | 13 | 4 |
Imputation | 1 | 1 |
No information | 10 | 12 |
Predictor selection | ||
Stepwise, forward, backward, automatic algorithm selection | 4 | 3 |
Univariate analysis | 7 | 2 |
Literature review | 6 | 3 |
No information | 7 | 10 |
The statistical model for prediction | ||
Logistic regression | 8 | 10 |
Cox regression | 15 | 6 |
Subdistribution hazard model | 1 | 2 |
Type of model | ||
Lab-based | 13 | 5 |
Office-based | 3 | 7 |
Both | 8 | 6 |
Sex-specific model | 2 | 4 |
Overfitting correction | 7 | 3 |
The presentation as a risk score | 19 | 16 |
4.2.1. Outcome Definition
In six of the articles, I-T2DM was defined based on fasting blood sugar (FBS), 2 hour blood sugar (2h-BS), and Hemoglobin A1c (HbA1c) (19, 20, 25, 26, 32, 36). In the remaining studies, the following compounds were considered for definition of T2DM: FBS and 2h-BS in three of the studies (18, 23, 37), FBS and HbA1c in six of the studies (21, 27-29, 33, 34), FBS in six of the studies (15, 17, 24, 30, 31, 38), HbA1c in one of the studies (16), and physician-diagnosed using electronic health records in two of the studies (22, 35). Moreover, glucose-lowering mediation as another definition for T2DM was included in 14 of the studies (15, 17, 18, 20, 24, 26, 27, 29-34, 38). Almost the same variation in definition was observed to screen U-T2DM definition (Appendix 4 in Supplementary File).
4.2.2. Treatment of Continuous Variables
The detailed information on the treatment of continuous variables for I-T2DM is reported in Appendix 5 in Supplementary File. Eighteen prediction models categorized all the continuous risk factors (15, 17, 18, 20, 23, 24, 26-30, 32-38), four risk factors (16, 22, 25, 31), and two continuous and categorical risk factor (19, 21). Considering model development for U-T2DM screening (Appendix 6 in Supplementary File), all continuous variables were categorized in 19 models (39-41, 43-54, 56, 57), and the variables kept continuous in three models (42, 55).
4.2.3. Missing Strategy
With respect to the prognostic model for I-T2DM, complete case analysis was performed on 13 of the studies (15, 18, 20, 21, 23, 24, 26-29, 32, 34, 38). Only one of the studies used multiple imputations (22). The strategy of dealing with missing values was not clear in 10 developed models (16, 17, 19, 25, 30, 31, 33, 35-37); thus, we assumed that complete case analysis was performed.
Regarding screening U-T2DM, the missing treatment strategy was not clear in nine models (44-46, 51, 54, 56, 57) (Appendix 6 in Supplementary File). Complete case analysis was performed on 12 models (16, 41, 42, 47-50, 52, 53, 55, 57), and multiple imputation was reported for one model (43).
4.2.4. Predictor Selection
Seven of the studies reported using the univariable analysis to reduce the number of risk predictors (16, 18, 20, 21, 24, 28, 30), and six of the studies included all literature-based risk factors in multivariate analysis (19, 22, 27, 29, 31, 33). Automatic selection was reported in five of the articles (32, 35-38), and no information on the model building strategy was found in seven of the articles (15, 17, 19, 23, 25-27). In the current study, the number of predictors included in the developed models ranged between 4 - 15 for I-T2DM and 3 - 10 for U-T2DM screening (excluding the article with more than 40 predictors (35)).
4.2.5. The Statistical Model for Prediction
Most prognostic models for I-T2DM were developed using Cox (n = 15) (15-20, 22, 28, 30-33, 36-38) and logistic regression (n = 8) (21, 23, 25-27, 29, 34, 35) using enter, automatic forward selection, backward elimination, or stepwise procedure. The sub-distribution hazard model was reported in one of the studies (24). As expected, all diagnostic models for U-T2DM screening used the logistic model for data analysis.
4.2.6. Overfitting in Prediction Models
For the I-T2DM model development, overfitting was controlled for seven of the studies (Table 1), and for U-T2DM, overfitting was controlled for 12 models (Appendix 6 in Supplementary File). Bootstrapping was the most used strategy to control overfitting in I-T2DM and U-T2DM.
4.2.7. Extra Information on Model Development
Thirteen of the studies generated only laboratory-based (invasive) risk prediction models (16, 17, 19, 20, 24, 28-32, 35, 37, 38) for I-T2DM, while an office-based (non-invasive) risk method using demographic and clinical measurements (e.g. sex and BMI) was reported in four of the studies (25, 27, 34). Eight of the studies reported both invasive and non-invasive prediction models (15, 18, 21-23, 26, 32, 36) (Table 2). For U-T2DM, 18 models were based solely on office-based measurements, three models were developed according to lab measurements, and only one of the studies reported both invasive and non-invasive models (Appendix 6 in Supplementary File).
Model Development and Validation Characteristics of Undiagnosed Type 2 Diabetes Mellitus (N = 19 Studies and 22 Models)
Numbers | |
---|---|
Model Performance Measures | |
Discrimination measures | |
C statistics/AUC | 22 |
D statistic | - |
Sensitivity/specificity | 19 |
Othersa | 12 |
Calibration | |
Calibration plot | 3 |
Hosmer-Lemeshow test | 7 |
Brier score | - |
Observed-predicted ratio | - |
Overfitting | 12 |
Overall performance measures: | |
R2 | - |
AIC, BIC | 2 |
Clinical usefulness | 1 |
The performance as risk score | 20 |
Model Development Measures | |
Validation | |
Apparent | 15 |
Internal validation | 8 |
External validation | 11 |
Type of model | |
Invasive | 3 |
Non-invasive | 18 |
Both | 1 |
Sex-specific model | 2 |
Treatment of missing | |
Complete case | 12 |
Imputation | 1 |
No information | 9 |
Statistical model for prediction | |
Logistic regression | 22 |
Cox regression | - |
Survival analysis | - |
Body mass index and age were the two most commonly used variables in model development regarding screening U-T2DM and predicting newly diagnosed T2DM (Figure 2). Sex was adjusted in 11 of the studies, and only two of the studies (19, 22) developed sex-specific models. For I-T2DM, the interaction between variables was checked in three of the studies (15, 22, 23). However, two of the studies (37, 52) on U-T2DM screening focused on interaction terms.
The number of model predictors for incident and undiagnosed type 2 diabetes mellitus between November 2011 and 2019. BMI, body mass index; FBS, fasting blood sugar; HbA1c, hemoglobin A1c; FHDM, family history of diabetes; WC, waist circumference; WHR, waist to height ratio; Others, gestational diabetes, C-reactive protein levels, statin, atypical antipsychotics, corticosteroids, antipsychotic, learning disability, body mass index, Townsend score, CVD, schizophrenia or bipolar affective disorder, learning disability, balanitis or vulvitis, osmotic symptoms.
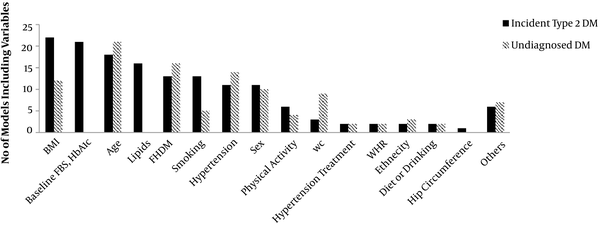
4.3. Model Validation
A summary and detailed characteristics of model validation for developing I-T2DM are reported in Table 3 and Appendix 7 in Supplementary File, respectively. Moreover, the detailed characteristics of model validation for U-T2DM screening are shown in Appendix 8 in Supplementary File.
Model Validation Characteristics for the Current and Previous Reviews for incident Type 2 DM
Updated Review (Current Review = 24) | Previous Reviews Collins et al. (8) and Noble et al. (9) (Risk Prediction Modelsa = 18) | |
---|---|---|
Validation | ||
Apparent | 10 | 11 |
Internalb | 15 | 7 |
Bootstrapping | 1 | 2 |
Random split sample | 9 | 4 |
Cross validation | 5 | 1 |
Jack-knifing | - | - |
External | 5 | 12 |
Performance measures | ||
Overall | ||
R2 | 3 | 1 |
AIC, BIC | 2 | 2 |
Brier statistics | 1 | - |
Discrimination | 25 | 18 |
AUC | 20 | 15 |
C-statistics | 8 | 2 |
D-statistics | 1 | 2 |
Calibrationc | 19 | 14 |
Calibration plot | 9 | 3 |
Hosmer-Lemeshow test | 11 | 8 |
Barrier score | - | 2 |
Observed-predicted ratio | 1 | 1 |
No information | 5 | - |
Classification | ||
NRI/IDI | 5 | 1 |
Sensitivity/specificity | 15 | 15 |
Othersd | 5 | 6 |
Clinical usefulness | 1 | - |
4.3.1. Internal and External Validation
Fifteen out of the 24 development studies for I-T2DM reported internal validation (15, 16, 20, 22-24, 26, 27, 29-32, 35, 36, 38), 9 studies reported development and validation (n = 9), cross-validation (n = 5), and bootstrapping (n = 1). Five of the studies conducted external validation (18, 19, 21, 34, 35) (Table 3 and Appendix 7 in Supplementary File). Eight models (40, 42, 45, 47, 50, 52, 53, 55) reported internal validation for U-T2DM screening, and external validation was performed for 11 out of the total introduced models (39-43, 46, 48, 51, 52, 54, 56) (Table 2 and Appendix 8 in Supplementary File).
4.3.2. Model Performance
With the aim of predicting newly diagnosed T2DM, all the studies reported at least one measure of predictive performance, with 20 of the studies reporting the area under the receiver curve (AUC) (15, 17, 18, 20, 21, 23, 24, 26-38), eight of the studies reporting C-statistics (15-17, 19, 24, 28, 29), and one of the studies reporting discrimination with D-statistics (15). Nineteen of the studies reported calibration, with the Hosmer-Lemeshow goodness of fit test in 11 of the studies (17, 18, 21, 23, 26, 27, 29, 31, 34, 36, 37), the observed-predicted plot in nine of the studies (16, 19, 22, 24, 26, 28, 32, 36, 38), and the observed-predicted ratio in one of the studies (30). Moreover, 15 of the studies reported classification analysis, and four of the studies reported the overall performance measure.
All the introduced models reported AUC for U-T2DM screening (39-57), 10 of the studies (39, 40, 43, 47-50, 52, 53) reported calibration, and three of the models (42, 47, 55) reported overall performance measurements. The median (IQR) value of AUC or C-statistics was 0.78 (0.74-0.82) for I-T2DM, while the median (IQR) value of AUC was 0.77 (0.74-0.81) for U-T2DM screening.
4.4. Other Considerations
4.4.1. Risk of Bias Assessment
The PROBAST recommendations for ROB assessment were presented for both I-T2DM (Appendix 9 in Supplementary File) and U-T2DM screening (Appendix 10 in Supplementary File) models. All the studies used an appropriate data source. The overall judgment of ROB assessment is shown in Figure 3. Low ROB was noted in three domains of participants, predictors, and outcomes for both I-T2DM and U-T2DM. Forty-two percent of the prediction models were observed to have high ROB for I-T2DM, which was 18.2% for U-T2DM. ROB was generally high or unclear for I-T2DM and low or unclear (82%) for U-T2DM.
The overall judgment of risk of bias (ROB) for incident and undiagnosed type 2 diabetes mellitus between November 2011 and 2019
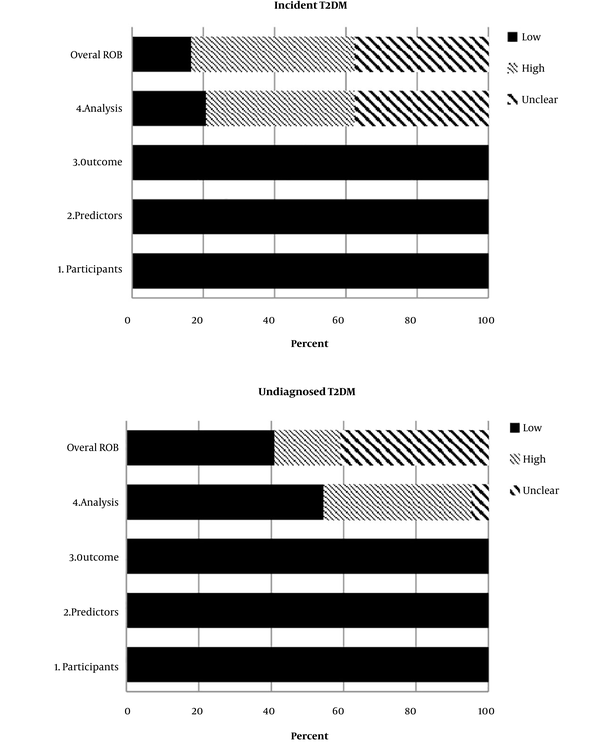
4.4.2. Citation Rate
The median duration from the publication date for the prognostic models was 3 years with the 2.35 citation rate per year. Further, the median duration from the publication date for the U-T2DM screening models was 4 years with the 2.26 citation per year (Appendix 11 in Supplementary File).
5. Discussion
To the best of our knowledge, this was the first systematic review to report requirements for major prediction models to predict I-T2DM or screen U-T2DM using the TRIPOD and PROBAST checklist. Our systematic review yielded 45 published studies between December 2011 and October 2019 reporting all aspects of developing and validating prediction models according to the CHARMS checklist. According to the PROBAST assessment tool introduced based on the TRIPOD statement, the majority of the prediction models were observed to have high or unclear risk for I-T2DM but low or unclear risk for U-T2DM.
5.1. Study Design for Model Development
A variable selection strategy is a challenging part of prediction modeling. Several approaches are recommended, including pre-specified literature-based variable selection, univariable analysis, and automatic variable selection (forward selection, backward elimination, or stepwise). In our review, univariable analysis (29%) was the most commonly used method to build a statistical model. However, in the previously published reviews, literature-based and automatic variable selection approaches were the most reported ones (16.7%). Thirty-two percent of the studies in our review (55.5% of the previously published reviews) failed to report any information regarding variable selection strategies.
One of the problems in developing multivariable prediction models is to treat continuous variables and examine whether they are categorized or kept continuous. With categorizing continuous variables, important information might be lost, and we may lose power to detect real association (3). There is a firm opinion that continuous variables should be kept continuous, and in case of a non-linear association, other statistical methods (e.g., splines) are recommended (58). Nevertheless, researchers prefer to categorize continuous variables because it is more applicable in clinical decision-making (59). In our review, 75% of the studies on I-T2DMcategorized all variables and; In the previously published articles 61% of articles categorized all variables.
5.2. Missing Data Strategy
Missing data is a serious problem in epidemiological and clinical studies as it can reduce statistical power and efficiency. A common way to manage missing data is to use listwise methods, also known as complete case analysis. Although this strategy is straightforward and easy to use, it decreases statistical analysis power and thus it is not recommended. Multiple imputation (MI) is a superior approach to minimize the missing information effect. MI can increase study precision and result in robust statistics (60). Single imputation (SI) may be a good alternative for prediction models despite its limitations, such as uncertainty underestimation. Since point estimation, and not variability, is our primary interest in the prediction models, statisticians advise SI because it is easy to implement and since a score based on rounded coefficients gives almost the same result as MI (3). As acknowledged by Steyerberg (3) “MI may, therefore, have only minor advantages over SI for model prediction” (2009, clinical prediction models, Part III, section 7, page 133). In our review, 54% of the studies (44% of the previously published reviews) followed complete case analysis and only one of the studies reported MI. However, the method used to resolve the missing data issue was not reported for I-T2DM in 42% of the studies; this was 66.7% of the previously published reviews.
5.3. Statistical Models
Multivariable regression models such as logistic regression or Cox proportional hazards regression commonly use statistical methods for deriving prediction models. We used the same strategy in our study with the difference that researchers have recently paid attention to family regression survival. Each of these statistical approaches has its own assumptions and limitations that may reduce generalizability. The usual approach in driving prediction models is to use all available data and population risk factors to compute risk scores using only one measurement, known as “global predictive models”. Patient-specific predictive models, introduced as “personalized prediction models”, are an alternative approach that use each individual’s dynamic information to derive more relevant models. In recent years, time-varying regression models are becoming more common (61-63).
5.4. Overfitting in Model Development
Both model and parameter uncertainty result in occurring overfitting, indicating that the prediction models are not valid for the new society. Bootstrapping is recommended by using a rule of thumb of 10 cases per predictor or reporting optimism-corrected performance (3). Of the studies included in this review, 29% had overfitting correction, while this rate was 16.7% in the previously published articles for I-T2DM.
5.5. Model Performance
The next crucial step after model development is to quantify model performance. There are three types of performance: (1) apparent validation (using the same data set as the model developed for reporting validation); (2) internal validation such as split sampling, cross-validation, or bootstrapping methods; and (3) external validation (using completely different data). More than half of the studies in the current review for I-T2DM reported internal validation, while this rate was 38.9% in the previously published articles. In the current review, 21% of the studies reported external validation, while this rate was 48% in the previously published articles.
Reporting overall performance (e.g., AIC/BIC and R2) with discrimination ability between events and non-events (e.g., AUC, C-index, sensitivity, and specificity) is informative and somehow necessary in model evaluation. In the current and previously published reviews, all the articles reported at least one discrimination aspect. Overall performance was reported only in four of the articles for I-T2DM. Moreover, demonstrating the calibration method (e.g., the Hosmer-Lemeshow test and the calibration plot), especially for a binary outcome, is informative and shows the agreement level between observed and predicted outcomes. More than 75% of the selected articles in the current and previously published reviews reported calibration measurements for I-T2DM.
5.6. Strategies for Model Improvement
We focused on model development and validation requirements. However, some other model improvement strategies, such as improving statistical methods, considering interaction terms, and considering non-linear associations, are also recommended. Some epidemiologists advised to estimate prediction models including relevant interaction terms in addition to the main effects. A literature review may help us select the proper interaction. However, it should be noted that interaction terms in the prediction models do not necessarily increase model performance. Moreover, because of the therapeutic improvement of medicine or disease-related definition, predictors’ effect may change over time. For example, predictors’ effect for T2DM development is noted to decrease with aging. The older population is more affected by other types of disease; thus, considering “age × predictors” in the prediction models may be useful. In the current review, only one of the studies reported age interaction (22). Further biological and pre-specified relevant interactions such as ‘SEX×predictors’ are also recommended.
5.7. Sex-specific Prediction Models
Evidence shows that gender differences are important in many diseases, particularly non-communicable diseases (64, 65). According to the 2019 IDF Atlas in 2019, there were 17 million more men diagnosed as having T2DM than women (66). Of the studies included in this review, sex-specific prediction models were reported only in two (8%); this number was four (22.2%) among the previously published reviews on I-T2DM. Varieties in endocrine (e.g., biology and sex-hormones), as well as in behavioral (e.g., lifestyle and socioeconomic status), cultural, environmental, and epidemiological context, Indicates the difference between male and females. For example, overweight/obesity is the major risk factor of T2DM in both genders, with the difference that men are overweight/obese in their younger age whereas women are overweight/obese in their middle age. Also, diabetes-related comorbidities differ in men and women and require specific management strategies (65, 67, 68). A systematic review showed that microvascular complications were higher among men with T2DM, while CVD morbidity and mortality, as well as psychological problems, were higher among women with T2DM (69). Despite the importance to consider sex differences in awareness, diagnosis, treatment, prediction, and prevention strategies, few studies have focused on the issue (69). In the current study, we observed a downward trend of sex-specific models (8%) compared to the previously published articles for I-T2DM, although not significant (22.2%).
5.8. Age-specific Prediction Models
The global prevalence of T2DM is expected to rise from 9.3% to 10.2% between 2019 and 2030 (70). Even though most of this increase has been reported in the middle-aged and elderly population, several studies showed a decrease in the age of diagnosis (71-73). In the current review, the prediction models were mostly developed in the middle-aged and older population, and only two studies recruited a younger population for I-T2DM (15, 30). Previous reviews show that the early onset of T2DM is a serious concern in various ethnic groups and is strongly associated with the development of micro/macrovascular complications. A better understanding of potential risk factors and a possible disease mechanism of the early onset of T2DM in the young population could be helpful in controlling future complications of the disease on individuals and the healthcare system (73, 74).
5.9. Role of Non-traditional Risk Factors in Prediction Models
Besides biological factors, psychological disorders are also responsible for increased blood glucose. Epidemiological studies implicate that psychological factors, socioeconomic status, poverty, education level, occupational stress, and sleep disorders are related to a higher risk of T2DM (75, 76). In our review, over 90% of the studies did not use these factors, and only one of the studies used a depression score (22) and sleep apnea (35). For example, low education is related to a higher risk of diabetes among Australian women (76), while higher education increases I-T2DM among Iranian men (77). Adding psychological factors may improve the fit of models predicting or screening T2DM, as even shown in QRISK 2017 (22). Evidence supports the existence of a two-way relationship between T2DM and poverty, with T2DM increasing the risk of falling into poverty, especially in men, and poverty is associated with a higher risk of I-T2DM along with inequality of diabetes care (78, 79). However, using simple and reliable covariates is the main point of prediction models. Clinicians recommend improving these models with even subjective measurements.
Two systematic reviews (80, 81) suggested that the presence of endocrine-disrupting chemicals (EDCs) in the environment, such as bisphenol A, phthalates, and persistent organic pollutants or dioxins, may also be associated with I-T2DM. Plastic bottles, metal cans, toys, and many other manufacturer products are considered EDCs. They impair the normal activity of hormones and cause a wide range of adverse events. Several epidemiological studies evaluated the association between EDCs such as air pollution (82) and T2DM. However, the causality and a whole mixture of toxicants as well as duration of being at risk in the human study have not been demonstrated yet (80). Recently, scientists have shown that both nitrogen dioxide (NO2) as a measure of traffic-exposure and annual concentrations of particular matter < 2.5 µm (PM 2.5) as a measure of both traffic-related and transported particles, are statistically associated with a quick decline in the whole-body insulin sensitivity and a faster increase in BMI among children aged 8 - 15 years (83, 84). However, the roles of air pollution and endocrine disrupters have not been yet considered in studies including the current one, despite the high prevalence of air pollution in some countries (33-39).
5.10. Ethnicity in Prediction Models
Evidence is accumulating on the significance of specific ethnic groups at the increased risk of T2DM. According to the IDF report, the Middle East and African countries have the highest age-standardized prevalence of T2DM, and the number of people with T2DM is expected to increase by 94% and 143% between 2019 and 2045 in these regions, respectively. Globally, the lower increasing rate of prevelance is estimated in the European ethnicity by 15% (70). Several risk prediction models have been developed for U-T2DM prognosis or screening worldwide (8). However, the significance of country-based models is still controversial. In the current review, over 70% of the prediction models for I-T2DM were derived in the East Asian countries (17, 29-31, 36, 38). While in the previously published articles, more than 50% of the prediction models were developed in the American and European populations (6, 7, 85-93). By comparing the risk prediction models’ performance in the current review and the previously published articles, a similar median discrimination index (0.78 for the current review and 0.8 for the previously published reviews) with almost similar predictors was observed, irrespective of the geographical location. Our findings are supported by the studies of Tanamas (94) and Rosella et al. (95). Tanamas et al. (94) examined several T2DM prediction models in two cohort studies: AusDiab and Mauritian south population survey. The discrimination power was reported to be higher in the mixed population. They found that ethnicity did not improve model performance. Their findings are in line with the previous study (95) considering that ethnicity information did not improve the discrimination and accuracy of the prediction models. They emphasized that the similarity of ethnicity or diabetes risk could not determine the appropriate model performance in another population. This could be due to the fact that ethnicity is affected by other diabetes risk factors including a family history of diabetes, BMI, physical activity, and diet. According to the discussion above, compared to development of new models, external validation and calibration of the existing models are preferred and cost-neutral (96).
5.11. External Validation ad Recalibration on Prediction Models
To the best of our knowledge, none of the studies in the current review was externally validated in an independent study. However, some previously developed models were externally validated and recalibrated several times by independent researchers (4, 7, 91, 97). Masconi et al. (98) investigated the external validation and recalibration of diabetes risk prediction models in their systematic review of 94 articles, including 70 models and 236 validations on T2DM. The most commonly validated model for I-T2DM was FOS (7) (10.1%), followed by the San Antonio risk model (91) (9.5%). For U-T2DM screening, the Finish diabetes risk score (4) (14.8%) was the most frequently validated prediction model, followed by the Rotterdam model 1 (97) (12.5%). Recalibration was performed on 22.9% of the validation models in the validation study for I-T2DM.
5.12. Strengths and Limitations
The strength of this study is that it was reported in accordance with the PRISMA-ScR checklist. This review also included a comprehensive report of model development (e.g., the outcome definition, variable selection, statistical analysis, and treatment of continuous variables) and validation (e.g., calibration and net benefit) requirements according to the TRIPOD guideline. Study quality control and ROB assessment were also reported using the Newcastle-Ottawa scale and the PROBAST checklist. Our study is very informative since previously published articles examined in previous systematic reviews were also evaluated and compared with the currently selected articles based on the TRIPOD prediction model guideline. However, there are also some limitations. Firstly, only English articles were included and thus we may have missed some articles. Secondly, we decided to exclude Genetic risk prediction or non-regression based models (e.g., neural networks or decision tree) due to their different nature.
6. Conclusions
Among prediction models of I-T2DM progression or U-T2DM screening between December 2011 and October 2019, we observed intermediate to poor quality were assessed in several aspects of model development and validation, mainly from the analysis part. It poses the question whether we could rely on the current prediction models or we should develop new models. Another major concern is that a newly developed model can be easily disregarded if it has no added value for health policymakers or clinicians. Using pre-specific risk factors or traditional statistical approaches is similar to the existing prediction models; for example, the mean (SD) of AUC has been 0.78 (0.06) in the last twenty years. It may be required to develop personalized comprehensive prediction models by considering additional risk factors so that the prediction models’ performance could be improved more effectively. It has been shown that time-varying prediction models can outperform global models (63). External validation and recalibration could help us tailor the available prediction models to local populations, which is a better option than developing a new model.
Acknowledgements
References
-
1.
American Diabetes Association. Standards of Medical Care in Diabetes-2018 Abridged for Primary Care Providers. Clin Diabetes. 2018;36(1):14-37. [PubMed ID: 29382975]. [PubMed Central ID: PMC5775000]. https://doi.org/10.2337/cd17-0119.
-
2.
Ogurtsova K, da Rocha Fernandes JD, Huang Y, Linnenkamp U, Guariguata L, Cho NH, et al. IDF Diabetes Atlas: Global estimates for the prevalence of diabetes for 2015 and 2040. Diabetes Res Clin Pract. 2017;128:40-50. [PubMed ID: 28437734]. https://doi.org/10.1016/j.diabres.2017.03.024.
-
3.
Steyerberg EW. Clinical Prediction Models. Springer; 2009. https://doi.org/10.1007/978-0-387-77244-8.
-
4.
Lindstrom J, Tuomilehto J. The diabetes risk score: a practical tool to predict type 2 diabetes risk. Diabetes Care. 2003;26(3):725-31. [PubMed ID: 12610029]. https://doi.org/10.2337/diacare.26.3.725.
-
5.
Chen L, Magliano DJ, Balkau B, Colagiuri S, Zimmet PZ, Tonkin AM, et al. AUSDRISK: an Australian Type 2 Diabetes Risk Assessment Tool based on demographic, lifestyle and simple anthropometric measures. Med J Aust. 2010;192(4):197-202. [PubMed ID: 20170456]. https://doi.org/10.5694/j.1326-5377.2010.tb03507.x.
-
6.
Hippisley-Cox J, Coupland C, Robson J, Sheikh A, Brindle P. Predicting risk of type 2 diabetes in England and Wales: prospective derivation and validation of QDScore. BMJ. 2009;338:b880. [PubMed ID: 19297312]. [PubMed Central ID: PMC2659857]. https://doi.org/10.1136/bmj.b880.
-
7.
Wilson PW, Meigs JB, Sullivan L, Fox CS, Nathan DM, D'Agostino RB. Prediction of incident diabetes mellitus in middle-aged adults: the Framingham Offspring Study. Arch Intern Med. 2007;167(10):1068-74. [PubMed ID: 17533210]. https://doi.org/10.1001/archinte.167.10.1068.
-
8.
Collins GS, Mallett S, Omar O, Yu LM. Developing risk prediction models for type 2 diabetes: a systematic review of methodology and reporting. BMC Med. 2011;9:103. [PubMed ID: 21902820]. [PubMed Central ID: PMC3180398]. https://doi.org/10.1186/1741-7015-9-103.
-
9.
Noble D, Mathur R, Dent T, Meads C, Greenhalgh T. Risk models and scores for type 2 diabetes: systematic review. BMJ. 2011;343:d7163. [PubMed ID: 22123912]. [PubMed Central ID: PMC3225074]. https://doi.org/10.1136/bmj.d7163.
-
10.
Wareham NJ, Griffin SJ. Risk scores for predicting type 2 diabetes: comparing axes and spades. Diabetologia. 2011;54(5):994-5. [PubMed ID: 21380593]. https://doi.org/10.1007/s00125-011-2101-0.
-
11.
Moons KG, de Groot JA, Bouwmeester W, Vergouwe Y, Mallett S, Altman DG, et al. Critical appraisal and data extraction for systematic reviews of prediction modelling studies: the CHARMS checklist. PLoS Med. 2014;11(10). e1001744. [PubMed ID: 25314315]. [PubMed Central ID: PMC4196729]. https://doi.org/10.1371/journal.pmed.1001744.
-
12.
Wolff RF, Moons KGM, Riley RD, Whiting PF, Westwood M, Collins GS, et al. PROBAST: A Tool to Assess the Risk of Bias and Applicability of Prediction Model Studies. Ann Intern Med. 2019;170(1):51-8. [PubMed ID: 30596875]. https://doi.org/10.7326/M18-1376.
-
13.
Tricco AC, Lillie E, Zarin W, O'Brien KK, Colquhoun H, Levac D, et al. PRISMA Extension for Scoping Reviews (PRISMA-ScR): Checklist and Explanation. Ann Intern Med. 2018;169(7):467-73. [PubMed ID: 30178033]. https://doi.org/10.7326/M18-0850.
-
14.
Moons KG, Altman DG, Reitsma JB, Ioannidis JP, Macaskill P, Steyerberg EW, et al. Transparent Reporting of a multivariable prediction model for Individual Prognosis or Diagnosis (TRIPOD): explanation and elaboration. Ann Intern Med. 2015;162(1):W1-73. [PubMed ID: 25560730]. https://doi.org/10.7326/M14-0698.
-
15.
Arellano-Campos O, Gomez-Velasco DV, Bello-Chavolla OY, Cruz-Bautista I, Melgarejo-Hernandez MA, Munoz-Hernandez L, et al. Development and validation of a predictive model for incident type 2 diabetes in middle-aged Mexican adults: the metabolic syndrome cohort. BMC Endocr Disord. 2019;19(1):41. [PubMed ID: 31030672]. [PubMed Central ID: PMC6486953]. https://doi.org/10.1186/s12902-019-0361-8.
-
16.
Brateanu A, Barwacz T, Kou L, Wang S, Misra-Hebert AD, Hu B, et al. Determining the optimal screening interval for type 2 diabetes mellitus using a risk prediction model. PLoS One. 2017;12(11). e0187695. [PubMed ID: 29135987]. [PubMed Central ID: PMC5685604]. https://doi.org/10.1371/journal.pone.0187695.
-
17.
Chen X, Wu Z, Chen Y, Wang X, Zhu J, Wang N, et al. Risk score model of type 2 diabetes prediction for rural Chinese adults: the Rural Deqing Cohort Study. J Endocrinol Invest. 2017;40(10):1115-23. [PubMed ID: 28474301]. https://doi.org/10.1007/s40618-017-0680-4.
-
18.
Doi Y, Ninomiya T, Hata J, Hirakawa Y, Mukai N, Iwase M, et al. Two risk score models for predicting incident Type 2 diabetes in Japan. Diabet Med. 2012;29(1):107-14. [PubMed ID: 21718358]. https://doi.org/10.1111/j.1464-5491.2011.03376.x.
-
19.
Ha KH, Lee YH, Song SO, Lee JW, Kim DW, Cho KH, et al. Development and Validation of the Korean Diabetes Risk Score: A 10-Year National Cohort Study. Diabetes Metab J. 2018;42(5):402-14. [PubMed ID: 30113144]. [PubMed Central ID: PMC6202558]. https://doi.org/10.4093/dmj.2018.0014.
-
20.
Han X, Wang J, Li Y, Hu H, Li X, Yuan J, et al. Development of a new scoring system to predict 5-year incident diabetes risk in middle-aged and older Chinese. Acta Diabetol. 2018;55(1):13-9. [PubMed ID: 28918462]. https://doi.org/10.1007/s00592-017-1047-1.
-
21.
Heianza Y, Arase Y, Hsieh SD, Saito K, Tsuji H, Kodama S, et al. Development of a new scoring system for predicting the 5 year incidence of type 2 diabetes in Japan: the Toranomon Hospital Health Management Center Study 6 (TOPICS 6). Diabetologia. 2012;55(12):3213-23. [PubMed ID: 22955996]. https://doi.org/10.1007/s00125-012-2712-0.
-
22.
Hippisley-Cox J, Coupland C. Development and validation of QDiabetes-2018 risk prediction algorithm to estimate future risk of type 2 diabetes: cohort study. BMJ. 2017;359:j5019. [PubMed ID: 29158232]. [PubMed Central ID: PMC5694979]. https://doi.org/10.1136/bmj.j5019.
-
23.
Lim NK, Park SH, Choi SJ, Lee KS, Park HY. A risk score for predicting the incidence of type 2 diabetes in a middle-aged Korean cohort: the Korean genome and epidemiology study. Circ J. 2012;76(8):1904-10. [PubMed ID: 22640983]. https://doi.org/10.1253/circj.cj-11-1236.
-
24.
Liu X, Fine JP, Chen Z, Liu L, Li X, Wang A, et al. Prediction of the 20-year incidence of diabetes in older Chinese: Application of the competing risk method in a longitudinal study. Medicine (Baltimore). 2016;95(40). e5057. [PubMed ID: 27749572]. [PubMed Central ID: PMC5059075]. https://doi.org/10.1097/MD.0000000000005057.
-
25.
Moreno LM, Vergara J, Alarcon R. Predictive risk model for the diagnosis of diabetes mellitus type 2 in a follow-up study 15 years on: PRODI2 Study. Eur J Public Health. 2019;29(1):178-82. [PubMed ID: 29897477]. https://doi.org/10.1093/eurpub/cky107.
-
26.
Nanri A, Nakagawa T, Kuwahara K, Yamamoto S, Honda T, Okazaki H, et al. Development of Risk Score for Predicting 3-Year Incidence of Type 2 Diabetes: Japan Epidemiology Collaboration on Occupational Health Study. PLoS One. 2015;10(11). e0142779. [PubMed ID: 26558900]. [PubMed Central ID: PMC4641714]. https://doi.org/10.1371/journal.pone.0142779.
-
27.
Wen J, Hao J, Liang Y, Li S, Cao K, Lu X, et al. A non-invasive risk score for predicting incident diabetes among rural Chinese people: A village-based cohort study. PLoS One. 2017;12(11). e0186172. [PubMed ID: 29095851]. [PubMed Central ID: PMC5667808]. https://doi.org/10.1371/journal.pone.0186172.
-
28.
Yatsuya H, Li Y, Hirakawa Y, Ota A, Matsunaga M, Haregot HE, et al. A Point System for Predicting 10-Year Risk of Developing Type 2 Diabetes Mellitus in Japanese Men: Aichi Workers' Cohort Study. J Epidemiol. 2018;28(8):347-52. [PubMed ID: 29553059]. [PubMed Central ID: PMC6048299]. https://doi.org/10.2188/jea.JE20170048.
-
29.
Ye X, Zong G, Liu X, Liu G, Gan W, Zhu J, et al. Development of a new risk score for incident type 2 diabetes using updated diagnostic criteria in middle-aged and older chinese. PLoS One. 2014;9(5). e97042. [PubMed ID: 24819157]. [PubMed Central ID: PMC4018395]. https://doi.org/10.1371/journal.pone.0097042.
-
30.
Zhang H, Wang C, Ren Y, Wang B, Yang X, Zhao Y, et al. A risk-score model for predicting risk of type 2 diabetes mellitus in a rural Chinese adult population: A cohort study with a 6-year follow-up. Diabetes Metab Res Rev. 2017;33(7). [PubMed ID: 28608942]. https://doi.org/10.1002/dmrr.2911.
-
31.
Zhang M, Zhang H, Wang C, Ren Y, Wang B, Zhang L, et al. Development and Validation of a Risk-Score Model for Type 2 Diabetes: A Cohort Study of a Rural Adult Chinese Population. PLoS One. 2016;11(4). e0152054. [PubMed ID: 27070555]. [PubMed Central ID: PMC4829145]. https://doi.org/10.1371/journal.pone.0152054.
-
32.
Hu H, Nakagawa T, Yamamoto S, Honda T, Okazaki H, Uehara A, et al. Development and validation of risk models to predict the 7-year risk of type 2 diabetes: The Japan Epidemiology Collaboration on Occupational Health Study. J Diabetes Investig. 2018;9(5):1052-9. [PubMed ID: 29380553]. [PubMed Central ID: PMC6123034]. https://doi.org/10.1111/jdi.12809.
-
33.
Hu H, Wang J, Han X, Li Y, Miao X, Yuan J, et al. Prediction of 5-year risk of diabetes mellitus in relatively low risk middle-aged and elderly adults. Acta Diabetol. 2020;57(1):63-70. [PubMed ID: 31190268]. https://doi.org/10.1007/s00592-019-01375-w.
-
34.
Kraege V, Vollenweider P, Waeber G, Sharp SJ, Vallejo M, Infante O, et al. Development and multi-cohort validation of a clinical score for predicting type 2 diabetes mellitus. PLoS One. 2019;14(10). e0218933. [PubMed ID: 31596852]. [PubMed Central ID: PMC6785081]. https://doi.org/10.1371/journal.pone.0218933.
-
35.
McCoy RG, Nori VS, Smith SA, Hane CA. Development and Validation of HealthImpact: An Incident Diabetes Prediction Model Based on Administrative Data. Health Serv Res. 2016;51(5):1896-918. [PubMed ID: 26898782]. [PubMed Central ID: PMC5034198]. https://doi.org/10.1111/1475-6773.12461.
-
36.
Miyakoshi T, Oka R, Nakasone Y, Sato Y, Yamauchi K, Hashikura R, et al. Development of new diabetes risk scores on the basis of the current definition of diabetes in Japanese subjects [Rapid Communication]. Endocr J. 2016;63(9):857-65. [PubMed ID: 27523099]. https://doi.org/10.1507/endocrj.EJ16-0340.
-
37.
Noto D, Cefalu AB, Barbagallo CM, Falletta A, Ganci A, Sapienza M, et al. Prediction of incident type 2 diabetes mellitus based on a twenty-year follow-up of the Ventimiglia heart study. Acta Diabetol. 2012;49(2):145-51. [PubMed ID: 21698484]. https://doi.org/10.1007/s00592-011-0305-x.
-
38.
Wang A, Chen G, Su Z, Liu X, Liu X, Li H, et al. Risk scores for predicting incidence of type 2 diabetes in the Chinese population: the Kailuan prospective study. Sci Rep. 2016;6:26548. [PubMed ID: 27221651]. [PubMed Central ID: PMC4879553]. https://doi.org/10.1038/srep26548.
-
39.
Asadollahi K, Asadollahi P, Azizi M, Abangah G. A self-assessment predictive model for type 2 diabetes or impaired fasting glycaemia derived from a population-based survey. Diabetes Res Clin Pract. 2017;131:219-29. [PubMed ID: 28778049]. https://doi.org/10.1016/j.diabres.2017.07.016.
-
40.
Bernabe-Ortiz A, Smeeth L, Gilman RH, Sanchez-Abanto JR, Checkley W, Miranda JJ, et al. Development and Validation of a Simple Risk Score for Undiagnosed Type 2 Diabetes in a Resource-Constrained Setting. J Diabetes Res. 2016;2016:8790235. [PubMed ID: 27689096]. [PubMed Central ID: PMC5027039]. https://doi.org/10.1155/2016/8790235.
-
41.
Bhowmik B, Akhter A, Ali L, Ahmed T, Pathan F, Mahtab H, et al. Simple risk score to detect rural Asian Indian (Bangladeshi) adults at high risk for type 2 diabetes. J Diabetes Investig. 2015;6(6):670-7. [PubMed ID: 26543541]. [PubMed Central ID: PMC4627544]. https://doi.org/10.1111/jdi.12344.
-
42.
Felix-Martinez GJ, Godinez-Fernandez JR. Screening models for undiagnosed diabetes in Mexican adults using clinical and self-reported information. Endocrinol Diabetes Nutr. 2018;65(10):603-10. [PubMed ID: 29945768]. https://doi.org/10.1016/j.endinu.2018.04.004.
-
43.
Gray LJ, Barros H, Raposo L, Khunti K, Davies MJ, Santos AC. The development and validation of the Portuguese risk score for detecting type 2 diabetes and impaired fasting glucose. Prim Care Diabetes. 2013;7(1):11-8. [PubMed ID: 23357741]. https://doi.org/10.1016/j.pcd.2013.01.003.
-
44.
Handlos LN, Witte DR, Almdal TP, Nielsen LB, Badawi SE, Sheikh AR, et al. Risk scores for diabetes and impaired glycaemia in the Middle East and North Africa. Diabet Med. 2013;30(4):443-51. [PubMed ID: 23331167]. https://doi.org/10.1111/dme.12118.
-
45.
Katulanda P, Hill NR, Stratton I, Sheriff R, De Silva SD, Matthews DR. Development and validation of a Diabetes Risk Score for screening undiagnosed diabetes in Sri Lanka (SLDRISK). BMC Endocr Disord. 2016;16(1):42. [PubMed ID: 27456082]. [PubMed Central ID: PMC4960842]. https://doi.org/10.1186/s12902-016-0124-8.
-
46.
Lee YH, Bang H, Kim HC, Kim HM, Park SW, Kim DJ. A simple screening score for diabetes for the Korean population: development, validation, and comparison with other scores. Diabetes Care. 2012;35(8):1723-30. [PubMed ID: 22688547]. [PubMed Central ID: PMC3402268]. https://doi.org/10.2337/dc11-2347.
-
47.
Wu J, Hou X, Chen L, Chen P, Wei L, Jiang F, et al. Development and validation of a non-invasive assessment tool for screening prevalent undiagnosed diabetes in middle-aged and elderly Chinese. Prev Med. 2019;119:145-52. [PubMed ID: 30594538]. https://doi.org/10.1016/j.ypmed.2018.12.025.
-
48.
Zhou H, Li Y, Liu X, Xu F, Li L, Yang K, et al. Development and evaluation of a risk score for type 2 diabetes mellitus among middle-aged Chinese rural population based on the RuralDiab Study. Sci Rep. 2017;7:42685. [PubMed ID: 28209984]. [PubMed Central ID: PMC5314328]. https://doi.org/10.1038/srep42685.
-
49.
Barengo NC, Tamayo DC, Tono T, Tuomilehto J. A Colombian diabetes risk score for detecting undiagnosed diabetes and impaired glucose regulation. Prim Care Diabetes. 2017;11(1):86-93. [PubMed ID: 27727004]. https://doi.org/10.1016/j.pcd.2016.09.004.
-
50.
Dugee O, Janchiv O, Jousilahti P, Sakhiya A, Palam E, Nuorti JP, et al. Adapting existing diabetes risk scores for an Asian population: a risk score for detecting undiagnosed diabetes in the Mongolian population. BMC Public Health. 2015;15:938. [PubMed ID: 26395572]. [PubMed Central ID: PMC4578253]. https://doi.org/10.1186/s12889-015-2298-9.
-
51.
Heianza Y, Arase Y, Saito K, Hsieh SD, Tsuji H, Kodama S, et al. Development of a screening score for undiagnosed diabetes and its application in estimating absolute risk of future type 2 diabetes in Japan: Toranomon Hospital Health Management Center Study 10 (TOPICS 10). J Clin Endocrinol Metab. 2013;98(3):1051-60. [PubMed ID: 23393174]. https://doi.org/10.1210/jc.2012-3092.
-
52.
Li W, Xie B, Qiu S, Huang X, Chen J, Wang X, et al. Non-lab and semi-lab algorithms for screening undiagnosed diabetes: A cross-sectional study. EBioMedicine. 2018;35:307-16. [PubMed ID: 30115607]. [PubMed Central ID: PMC6154869]. https://doi.org/10.1016/j.ebiom.2018.08.009.
-
53.
Memish ZA, Chang JL, Saeedi MY, Al Hamid MA, Abid O, Ali MK. Screening for Type 2 Diabetes and Dysglycemia in Saudi Arabia: Development and Validation of Risk Scores. Diabetes Technol Ther. 2015;17(10):693-700. [PubMed ID: 26154413]. https://doi.org/10.1089/dia.2014.0267.
-
54.
Riaz M, Basit A, Hydrie MZ, Shaheen F, Hussain A, Hakeem R, et al. Risk assessment of Pakistani individuals for diabetes (RAPID). Prim Care Diabetes. 2012;6(4):297-302. [PubMed ID: 22560662]. https://doi.org/10.1016/j.pcd.2012.04.002.
-
55.
Stiglic G, Kocbek P, Cilar L, Fijacko N, Stozer A, Zaletel J, et al. Development of a screening tool using electronic health records for undiagnosed Type 2 diabetes mellitus and impaired fasting glucose detection in the Slovenian population. Diabet Med. 2018;35(5):640-9. [PubMed ID: 29460977]. https://doi.org/10.1111/dme.13605.
-
56.
Sulaiman N, Mahmoud I, Hussein A, Elbadawi S, Abusnana S, Zimmet P, et al. Diabetes risk score in the United Arab Emirates: a screening tool for the early detection of type 2 diabetes mellitus. BMJ Open Diabetes Res Care. 2018;6(1). e000489. [PubMed ID: 29629178]. [PubMed Central ID: PMC5884268]. https://doi.org/10.1136/bmjdrc-2017-000489.
-
57.
Zhang M, Lin L, Xu X, Wu X, Jin Q, Liu H. Noninvasive screening tool to detect undiagnosed diabetes among young and middle-aged people in Chinese community. Int J Diabetes Develop Countries. 2018;39(3):458-62. https://doi.org/10.1007/s13410-018-0698-y.
-
58.
Royston P, Sauerbrei W. Multivariable model-building: a pragmatic approach to regression anaylsis based on fractional polynomials for modelling continuous variables. 777. John Wiley & Sons; 2008.
-
59.
Mazumdar M, Glassman JR. Categorizing a prognostic variable: review of methods, code for easy implementation and applications to decision-making about cancer treatments. Stat Med. 2000;19(1):113-32. [PubMed ID: 10623917]. https://doi.org/10.1002/(sici)1097-0258(20000115)19:1<113::aid-sim245>3.0.co;2-o.
-
60.
Little RJ, Rubin DB. Statistical Analysis with Missing Data, Third Edition. 2019. https://doi.org/10.1002/9781119482260.
-
61.
Cowley LE, Farewell DM, Maguire S, Kemp AM. Methodological standards for the development and evaluation of clinical prediction rules: a review of the literature. Diagn Progn Res. 2019;3:16. [PubMed ID: 31463368]. [PubMed Central ID: PMC6704664]. https://doi.org/10.1186/s41512-019-0060-y.
-
62.
Rizopoulos D. Joint Models for Longitudinal and Time-to-Event Data. Chapman and Hall/CRC; 2012. https://doi.org/10.1201/b12208.
-
63.
Ng K, Sun J, Hu J, Wang F. Personalized Predictive Modeling and Risk Factor Identification using Patient Similarity. AMIA Jt Summits Transl Sci Proc. 2015;2015:132-6. [PubMed ID: 26306255]. [PubMed Central ID: PMC4525240].
-
64.
Perreault L, Ma Y, Dagogo-Jack S, Horton E, Marrero D, Crandall J, et al. Sex differences in diabetes risk and the effect of intensive lifestyle modification in the Diabetes Prevention Program. Diabetes Care. 2008;31(7):1416-21. [PubMed ID: 18356403]. [PubMed Central ID: PMC2453677]. https://doi.org/10.2337/dc07-2390.
-
65.
Kautzky-Willer A, Harreiter J, Pacini G. Sex and Gender Differences in Risk, Pathophysiology and Complications of Type 2 Diabetes Mellitus. Endocr Rev. 2016;37(3):278-316. [PubMed ID: 27159875]. [PubMed Central ID: PMC4890267]. https://doi.org/10.1210/er.2015-1137.
-
66.
Saeedi P, Petersohn I, Salpea P, Malanda B, Karuranga S, Unwin N, et al. Global and regional diabetes prevalence estimates for 2019 and projections for 2030 and 2045: Results from the International Diabetes Federation Diabetes Atlas, 9(th) edition. Diabetes Res Clin Pract. 2019;157:107843. [PubMed ID: 31518657]. https://doi.org/10.1016/j.diabres.2019.107843.
-
67.
Thorand B, Baumert J, Kolb H, Meisinger C, Chambless L, Koenig W, et al. Sex differences in the prediction of type 2 diabetes by inflammatory markers: results from the MONICA/KORA Augsburg case-cohort study, 1984-2002. Diabetes Care. 2007;30(4):854-60. [PubMed ID: 17392546]. https://doi.org/10.2337/dc06-1693.
-
68.
Onat A, Hergenc G, Keles I, Dogan Y, Turkmen S, Sansoy V. Sex difference in development of diabetes and cardiovascular disease on the way from obesity and metabolic syndrome. Metabolism. 2005;54(6):800-8. [PubMed ID: 15931618]. https://doi.org/10.1016/j.metabol.2005.01.025.
-
69.
Arnetz L, Ekberg NR, Alvarsson M. Sex differences in type 2 diabetes: focus on disease course and outcomes. Diabetes Metab Syndr Obes. 2014;7:409-20. [PubMed ID: 25258546]. [PubMed Central ID: PMC4172102]. https://doi.org/10.2147/DMSO.S51301.
-
70.
Cho NH, Shaw JE, Karuranga S, Huang Y, da Rocha Fernandes JD, Ohlrogge AW, et al. IDF Diabetes Atlas: Global estimates of diabetes prevalence for 2017 and projections for 2045. Diabetes Res Clin Pract. 2018;138:271-81. [PubMed ID: 29496507]. https://doi.org/10.1016/j.diabres.2018.02.023.
-
71.
Koopman RJ, Mainous AG, Diaz VA, Geesey ME. Changes in age at diagnosis of type 2 diabetes mellitus in the United States, 1988 to 2000. Ann Fam Med. 2005;3(1):60-3. [PubMed ID: 15671192]. [PubMed Central ID: PMC1466782]. https://doi.org/10.1370/afm.214.
-
72.
Esteghamati A, Etemad K, Koohpayehzadeh J, Abbasi M, Meysamie A, Noshad S, et al. Trends in the prevalence of diabetes and impaired fasting glucose in association with obesity in Iran: 2005-2011. Diabetes Res Clin Pract. 2014;103(2):319-27. [PubMed ID: 24447808]. https://doi.org/10.1016/j.diabres.2013.12.034.
-
73.
Song SH, Hardisty CA. Early-onset Type 2 diabetes mellitus: an increasing phenomenon of elevated cardiovascular risk. Expert Rev Cardiovasc Ther. 2008;6(3):315-22. [PubMed ID: 18327993]. https://doi.org/10.1586/14779072.6.3.315.
-
74.
Wilmot E, Idris I. Early onset type 2 diabetes: risk factors, clinical impact and management. Ther Adv Chronic Dis. 2014;5(6):234-44. [PubMed ID: 25364491]. [PubMed Central ID: PMC4205573]. https://doi.org/10.1177/2040622314548679.
-
75.
Hackett RA, Steptoe A. Type 2 diabetes mellitus and psychological stress - a modifiable risk factor. Nat Rev Endocrinol. 2017;13(9):547-60. [PubMed ID: 28664919]. https://doi.org/10.1038/nrendo.2017.64.
-
76.
Kautzky-Willer A, Dorner T, Jensby A, Rieder A. Women show a closer association between educational level and hypertension or diabetes mellitus than males: a secondary analysis from the Austrian HIS. BMC Public Health. 2012;12:392. [PubMed ID: 22646095]. [PubMed Central ID: PMC3407471]. https://doi.org/10.1186/1471-2458-12-392.
-
77.
Derakhshan A, Sardarinia M, Khalili D, Momenan AA, Azizi F, Hadaegh F. Sex specific incidence rates of type 2 diabetes and its risk factors over 9 years of follow-up: Tehran Lipid and Glucose Study. PLoS One. 2014;9(7). e102563. [PubMed ID: 25029368]. [PubMed Central ID: PMC4100911]. https://doi.org/10.1371/journal.pone.0102563.
-
78.
Hsu CC, Lee CH, Wahlqvist ML, Huang HL, Chang HY, Chen L, et al. Poverty increases type 2 diabetes incidence and inequality of care despite universal health coverage. Diabetes Care. 2012;35(11):2286-92. [PubMed ID: 22912425]. [PubMed Central ID: PMC3476930]. https://doi.org/10.2337/dc11-2052.
-
79.
Callander EJ, Schofield DJ. Type 2 diabetes mellitus and the risk of falling into poverty: an observational study. Diabetes Metab Res Rev. 2016;32(6):581-8. [PubMed ID: 26663863]. https://doi.org/10.1002/dmrr.2771.
-
80.
Alonso-Magdalena P, Quesada I, Nadal A. Endocrine disruptors in the etiology of type 2 diabetes mellitus. Nat Rev Endocrinol. 2011;7(6):346-53. [PubMed ID: 21467970]. https://doi.org/10.1038/nrendo.2011.56.
-
81.
Chevalier N, Fenichel P. Endocrine disruptors: new players in the pathophysiology of type 2 diabetes? Diabetes Metab. 2015;41(2):107-15. [PubMed ID: 25454091]. https://doi.org/10.1016/j.diabet.2014.09.005.
-
82.
Liu F, Chen G, Huo W, Wang C, Liu S, Li N, et al. Associations between long-term exposure to ambient air pollution and risk of type 2 diabetes mellitus: A systematic review and meta-analysis. Environ Pollut. 2019;252(Pt B):1235-45. [PubMed ID: 31252121]. https://doi.org/10.1016/j.envpol.2019.06.033.
-
83.
Dzhambov AM. Long-term noise exposure and the risk for type 2 diabetes: a meta-analysis. Noise Health. 2015;17(74):23-33. [PubMed ID: 25599755]. [PubMed Central ID: PMC4918642]. https://doi.org/10.4103/1463-1741.149571.
-
84.
Marshall JD, Brauer M, Frank LD. Healthy neighborhoods: walkability and air pollution. Environ Health Perspect. 2009;117(11):1752-9. [PubMed ID: 20049128]. [PubMed Central ID: PMC2801167]. https://doi.org/10.1289/ehp.0900595.
-
85.
Balkau B, Lange C, Fezeu L, Tichet J, de Lauzon-Guillain B, Czernichow S, et al. Predicting diabetes: clinical, biological, and genetic approaches: data from the Epidemiological Study on the Insulin Resistance Syndrome (DESIR). Diabetes Care. 2008;31(10):2056-61. [PubMed ID: 18689695]. [PubMed Central ID: PMC2551654]. https://doi.org/10.2337/dc08-0368.
-
86.
Kahn HS, Cheng YJ, Thompson TJ, Imperatore G, Gregg EW. Two risk-scoring systems for predicting incident diabetes mellitus in U.S. adults age 45 to 64 years. Ann Intern Med. 2009;150(11):741-51. [PubMed ID: 19487709]. https://doi.org/10.7326/0003-4819-150-11-200906020-00002.
-
87.
Kanaya AM, Wassel Fyr CL, de Rekeneire N, Shorr RI, Schwartz AV, Goodpaster BH, et al. Predicting the development of diabetes in older adults: the derivation and validation of a prediction rule. Diabetes Care. 2005;28(2):404-8. [PubMed ID: 15677800]. https://doi.org/10.2337/diacare.28.2.404.
-
88.
Rosella LC, Manuel DG, Burchill C, Stukel TA; Phiat-Dm team. A population-based risk algorithm for the development of diabetes: development and validation of the Diabetes Population Risk Tool (DPoRT). J Epidemiol Community Health. 2011;65(7):613-20. [PubMed ID: 20515896]. [PubMed Central ID: PMC3112365]. https://doi.org/10.1136/jech.2009.102244.
-
89.
Schmidt MI, Duncan BB, Bang H, Pankow JS, Ballantyne CM, Golden SH, et al. Identifying individuals at high risk for diabetes: The Atherosclerosis Risk in Communities study. Diabetes Care. 2005;28(8):2013-8. [PubMed ID: 16043747]. https://doi.org/10.2337/diacare.28.8.2013.
-
90.
Schulze MB, Hoffmann K, Boeing H, Linseisen J, Rohrmann S, Mohlig M, et al. An accurate risk score based on anthropometric, dietary, and lifestyle factors to predict the development of type 2 diabetes. Diabetes Care. 2007;30(3):510-5. [PubMed ID: 17327313]. https://doi.org/10.2337/dc06-2089.
-
91.
Stern MP, Morales PA, Valdez RA, Monterrosa A, Haffner SM, Mitchell BD, et al. Predicting diabetes. Moving beyond impaired glucose tolerance. Diabetes. 1993;42(5):706-14. [PubMed ID: 8482427]. https://doi.org/10.2337/diab.42.5.706.
-
92.
von Eckardstein A, Schulte H, Assmann G. Risk for diabetes mellitus in middle-aged Caucasian male participants of the PROCAM study: implications for the definition of impaired fasting glucose by the American Diabetes Association. Prospective Cardiovascular Munster. J Clin Endocrinol Metab. 2000;85(9):3101-8. [PubMed ID: 10999793]. https://doi.org/10.1210/jcem.85.9.6773.
-
93.
Wannamethee SG, Papacosta O, Whincup PH, Thomas MC, Carson C, Lawlor DA, et al. The potential for a two-stage diabetes risk algorithm combining non-laboratory-based scores with subsequent routine non-fasting blood tests: results from prospective studies in older men and women. Diabet Med. 2011;28(1):23-30. [PubMed ID: 21166842]. https://doi.org/10.1111/j.1464-5491.2010.03171.x.
-
94.
Tanamas SK, Magliano DJ, Balkau B, Tuomilehto J, Kowlessur S, Soderberg S, et al. The performance of diabetes risk prediction models in new populations: the role of ethnicity of the development cohort. Acta Diabetol. 2015;52(1):91-101. [PubMed ID: 24996544]. https://doi.org/10.1007/s00592-014-0607-x.
-
95.
Rosella LC, Mustard CA, Stukel TA, Corey P, Hux J, Roos L, et al. The role of ethnicity in predicting diabetes risk at the population level. Ethn Health. 2012;17(4):419-37. [PubMed ID: 22292745]. [PubMed Central ID: PMC3457038]. https://doi.org/10.1080/13557858.2012.654765.
-
96.
Damen JA, Hooft L, Schuit E, Debray TP, Collins GS, Tzoulaki I, et al. Prediction models for cardiovascular disease risk in the general population: systematic review. BMJ. 2016;353. i2416. [PubMed ID: 27184143]. [PubMed Central ID: PMC4868251]. https://doi.org/10.1136/bmj.i2416.
-
97.
Baan CA, Ruige JB, Stolk RP, Witteman JC, Dekker JM, Heine RJ, et al. Performance of a predictive model to identify undiagnosed diabetes in a health care setting. Diabetes Care. 1999;22(2):213-9. [PubMed ID: 10333936]. https://doi.org/10.2337/diacare.22.2.213.
-
98.
Masconi KL, Matsha TE, Erasmus RT, Kengne AP. Recalibration in Validation Studies of Diabetes Risk Prediction Models: A Systematic Review. Int J Stat Med Res. 2015;4(4):347-69. https://doi.org/10.6000/1929-6029.2015.04.04.5.