Abstract
Background:
US adolescent obesity rates have quadrupled over the past 3 decades. Research examining complex factors associated with obesity is limited.Objectives:
The purpose of this study was to utilize a representative sample of students (grades 6 - 8) in Tennessee to determine the co-occurrence of risk behaviors with adolescent obesity prevalence and to analyze variations by strata.Patients and Methods:
The 2010 youth risk behavior survey dataset was used to examine associations of obesity with variables related to sample demographics, risk and protective behaviors, and region. Hierarchical logistic regression analyses stratified by demographics and region were conducted to evaluate variation in obesity risk occurring on three hierarchical levels: class, school and district.Results:
The sample consisted of 60715 subjects. The overall obesity rate was 22%. High prevalence of obesity existed in males, non-white race, those ever smoked and was positively correlated with age. Across three state regions, race, gender, and specific behaviors (smoking, weight misperception, disordered eating, +3 hours TV viewing, and no sports team participation) persisted as significant predictors of adolescent obesity, although variations by region and demographics were observed. Multilevel analyses indicate that < 1%, 0 - 1.97% and 4.03 - 13.06% of the variation in obesity was associated with district, school and class differences, respectively, when stratifying the sample by demographic characteristics or region.Conclusions:
Uniform school-based prevention efforts targeting adolescent obesity risk may have limited impact if they fail to respond to geographical and demographic nuances that hierarchal modeling can detect. Study results reveal that stratified hierarchical analytic approaches to examine adolescent obesity risk have tremendous potential to elucidate significant prevention insights.Keywords
Adolescents Obesity Health Risk Behaviors Hierarchical Logistic Models Regression Analysis
1. Background
Adolescent obesity is a major public health problem with rates steadily increasing (1). Obesity rates among 12 - 19 year olds have increased from 5% in 1980 to 20.5% in 2014 (2, 3). For any children under 18 years old, if his/her body mass index (BMI) ≥ the 95th percentile then he/she will be considered as obese (1). Many physical and demographic determinants of obesity during adolescence are well documented in the literature, with significant factors being age, gender, and ethnicity (1, 4, 5). More specifically, males are at higher risk of being obese than females (18.6% vs. 15.0%) (4), and black and Hispanic adolescents have a higher prevalence than whites (5). Personal factors, such as poor nutritional choices and sedentary lifestyle, also contribute significantly to adolescent obesity (6). The health risks of obesity for adolescents are numerous and include: hypertension, metabolic syndromes, and type 2 diabetes (7, 8), as well as poor mental health and decreased academic performance (9). Despite the understanding that obesity is a multi-factorial disease, little is understood about the role that health risk behaviors, such as drug and alcohol use, as well as protective factors, such as use of seatbelts and helmets, may play in adolescent obesity.
Adolescence is a developmental stage when tobacco, alcohol, drugs and other substances are initiated (10). An adolescent’s inclination to engage in risky health behaviors results from the interaction of individual attributes, peer or social pressures, and environmental influences-which parallels obesity risk factor research findings (11). Additionally, scientists support that the co-occurrence of multiple risk behaviors increases with adolescent age (12); and that relationships between various health risk behaviors exist (13).
2. Objectives
The purpose of this study was to utilize a statewide, representative sample of students in grades 6 - 8 in Tennessee to determine the co-occurrence of health risk behaviors among adolescents by determining the impact gender, age, race, and geographic region have on the association of these behaviors with the prevalence of adolescent obesity. Variations in risk patterns were explored by strata, which provided valuable insights into the role that district, school, and class level may play in adolescent obesity using multi-level logistic regression modeling, which allowed for stratified analyses with simultaneous control of individual factors.
3. Patients and Methods
The 2010 Tennessee middle school youth risk behavior survey (YRBS) data was used for this research project. The YRBS was designed to collect information about health risk behaviors among adolescence, like unhealthy dietary consumption of food and drinks, smoking, use of tobacco products and physical inactivity (14). In spring of 2010, a statewide representative sample of students in grades 6 - 8 in Tennessee Coordinated School Health were surveyed using a modified version of the YRBS. Adolescence in selected schools voluntarily worked on an anonymous, 46-question version of the YRBS form. Both parental and child consents were obtained before the survey form was administrated. Data for this research project were obtained from 456 public primary and secondary schools for a total of 65182 (83.10%) of the eligible 78441 students in grades 6 - 8. The survey had an overall response rate of 79.1%, which was calculated as (n1 of surveyed schools/n2 of eligible schools) times (n3 of useable survey form/n4 of eligible students in surveyed schools). The sample selection for schools consisted of all standard public schools containing grades 6 - 8. The selection of specific classes in each school utilized a systematic equal probability sampling approach with a random start time.
This study was based on 64790 survey forms, 4075 survey forms were discarded due to missing data. The study sample included 60715 students (93.7% of the total sample) and statistical analyses were completed in 2017.
3.1. Description of Key Study Variables
3.1.1. Outcome-Adolescent Obesity
BMI was calculated using the following formula: (weight/height2) × 703. For each age included on the measure, corresponding BMI percentiles were recorded. Those surveyed adolescences were classified as non-obese if BMI < 95th percentile and obese if BMI ≥ 95th percentile.
3.2. Explanatory Variables by Strata
3.2.1. District Level Variables
At the district level, two variables were found to be protective, wearing a seatbelt when in a car and wearing a helmet while using a bicycle. Both of these variables are legally mandated (15, 16) and rates of seat belt use among children are much higher in states with primary enforcement laws (17). Another variable, the percent of adolescence who answered ‘yes’ when asked to verify age when purchasing cigarettes, was used as a district level item. Research has shown that enforcement of tobacco sales laws improved merchants’ compliance with the proof-of-age requirements and had an impact on reducing youth smoking by prohibiting the purchase of tobacco (18, 19). However, enforcing laws such as these does require district level resources and resource-poor areas may not have adequate funds to enforce such laws, therefore, these variables may serve as a surrogate for unmeasured district socioeconomic status.
3.2.2. School Level Variables
There were two items computed at the school level. One was the percentage of students who had ever tried smoking. Student smoking prevalence is significantly associate with the enforcement of a school’s smoking policy (20). Further, the school environment is a contributing factor to peer influences on smoking. An individual’s peer network and reflective school norms have been shown to be significantly associated with smoking (21, 22). Another item was the percentage of students who reported having attended HIV/AIDS class in school, due to its inclusion in Tennessee’s comprehensive health education program (23).
3.2.3. Class Level Variables
Two items were evaluated at the class level. One was mean number of cigarettes smoking in the last month. Several studies have suggested peer influence as being the most important and consistent factors of adolescent tobacco use (10, 20, 24, 25). The risk of an adolescent currently smoking is significantly associated with their peer network especially if one or two best friends smoke (21). The logic of classifying this variable on the class level relates to proximity of peers within same classes and recognized associations between peers and smoking status. Similarly, the percentage of adolescence in each class reporting having “ever exercised to lose weight or to keep from gaining weight” was selected as a class level risk factors. Parallel to tobacco use, physical activity and related motivations are important for crowd association and significantly influenced by people around you (13), which provides basis this item as a class level variable.
3.2.4. Individual Level Variables
Age, sex, course study performance, and three regions in Tennessee (Delta, Central, or Appalachia) (26) were all evaluated as student level items. Students also responded to a multiple choice item summarizing overall grade performance. The reported grade variable was dichotomized as mostly A’s and B’s or mostly D’s and F’s. Due to the homogeneity of the sample, race was categorized as one of two categories: (1) white, American Indian, or Asian (WIA); or (2) black/African American, Cuban, Mexican, Puerto Rican, South or Central American, or other Spanish culture or origin (BHL). In addition, adolescence were asked whether they had “ever brought with a weapon, such as a shotgun, tool for cutting, or wooden stick” and if they had “ever been in or injured in a physical fight”. Substance use at early stage (substance use at the age of 11-year-old or younger) was evaluated for cigarette smoking, alcohol consumption, and marijuana use. Individuals’ perceptions of weight was estimated by the item “what is your opinion about your body weight”. Weight misperception was evaluated by assessing concordance or discordance between self-reported BMI and perceived weight. An eating disorder was defined as a definite reply to any of the questions; ever having abstained from all food, ever taken weight-loss drugs, or ever vomited to lose weight. Individual sedentary behaviors were evaluated using the time of watching TV on a school night (< 3 hours or ≥ 3 hours) and participation on any extracurricular sports teams. For statistical analyses each questionnaire was weighted to reduce response bias. Weights were used so that weighted analysis results were representative of all adolescence in grades 6 - 8 attending public schools in Tennessee 2010 (27). Basic descriptive statistics were conducted first. Since hierarchical analysis accounts for between-context and between-individual heterogeneity, to account for the nesting of subjects within classrooms, classrooms within schools and schools within districts the weighted data were analyzed using the hierarchical logistic regression method. Prior to performing the multiple hierarchical logistic regression modeling, the univariate hierarchical logistic regression was performed first to evaluate associations between each factor and obesity respectively. Those explanatory variables univariately associated with the dependent variable obesity with an effect size of 0.10 or larger in a simple hierarchical logistic regression were included as potential predictors (data not shown). In addition, the selected and excluded variables were checked for scientific plausibility. Due to significant interactions between gender, age, race and region (data not shown), the multiple hierarchical logistic regression analyses of the association between adolescent obesity and sample demographic variables, personality behaviors, substance use and health behaviors were further stratified by gender, age, race and region. Also, for each final model we did goodness-of-fit test and collinearity diagnostics. All final models fit the data very well and no strong collinearity was detected. The software SAS was sued to perform all statistical analyses (28).
4. Results
Totally, there were 64790 adolescence in the study sample in grades 6 - 8 in the state of Tennessee. The mean age was 12.79 years and standard deviation was 1.04 years. Obesity rates across age groups remained relatively steady at around 22% of students surveyed, except those aged 11 years (26%). Of the sample 32,054 (49.60%) were females. Males were more likely to be obese than females (27.25 vs 17.25%). There was also a higher prevalence of obesity in BHL (25.01%) compared to WIA (21.64%), although the sample predominantly (79.96%) reported as WIA.
Tables 1 - 3, present the results of four-level, multilevel logistic regressions (odds ratios and 95% confidence intervals, random effect variances and standard errors at levels 4, 3 and 2), stratified by gender (Table 1), race and region (Table 2) and age (Table 3). For females (Table 1), the district, school and class intercept variances were 0.01, 0.03 and 0.26 respectively.
Expressed as intraclass correlation, these variances suggest that 7.24% of the variability in obesity was dated with between-class (level 2) differences, less than 1% was dated with between-school (level 3) or between-district (level 4). On the other hand, for males, there was less variability in obesity at each level, comparing with females. As is often the case that differences between students are most important. Overall, there was low variability in obesity dated with between-school or between-district and there was high variability in obesity dated with between-class, especially among BHL students (13.06%, Table 2) and among students aged 11 (11.97%, Table 3). Additionally, females who had ever carried a weapon or reported not receiving HIV/AIDS education in school were also more likely to be obese. Males had a significant positive association between poorer grades in school and obesity, as well as having never been in a physical fight. Both genders had significant associations between all health behaviors, smoking and obesity.
Gender Stratified Hierarchical Logistic Regression Analysis of the Association Between Adolescent Obesity and Demographics, Personality Behaviors, Substance Use and Health Behaviors Using the Youth Risk Behavior Survey (YRBS) Data about Students in Grades 6 - 8 Attending Public Schools in Tennessee 2010
Variable | OR (95% CI) | |
---|---|---|
Female (n = 26885) | Male (n = 26309) | |
Demographics | ||
Age | 0.89 (0.86 - 0.93) | 0.95 (0.92 - 0.98) |
Race (WIA vs BHL) | 1.31 (1.19 - 1.45) | 0.93 (0.85 - 1.02) |
Region (Delta vs AC) | 1.15 (0.99 - 1.34) | 1.01(0.90 - 1.14) |
Personality behaviors | ||
Letter grades (D F vs A B) | 1.14 (0.96 - 1.35) | 1.12 (0.99 - 1.28) |
Seatbelt and helmet (yes vs no) | 0.17 (0.02 - 1.39) | 0.06 (0.01 - 0.35) |
Ever carried a weapon (yes vs no) | 1.08 (0.98 - 1.18) | 0.98 (0.91 - 1.05) |
Been in a physical fight (yes vs no) | 0.94 (0.86 - 1.02) | 0.90 (0.84 - 0.97) |
Injured in a fight (yes vs no) | 0.95 (0.78 - 1.14) | 0.92 (0.81 - 1.05) |
Substance use | ||
Ever smoked (yes vs no) | 3.52 (2.06-6.03) | 1.92 (1.24 - 2.99) |
Early onset smoking (yes vs no) | 1.04 (0.90-1.20) | 1.05 (0.93 - 1.19) |
Early onset Alcohol (yes vs no) | 0.94 (0.84-1.05) | 1.05 (0.96 - 1.15) |
Early onset Marijuana (yes vs no) | 0.99 (0.78-1.27) | 1.10 (0.92 - 1.32) |
Health behaviors | ||
Weight misperception (yes vs no) | 7.24 (6.65 - 7.88) | 12.09 (11.11 - 13.15) |
Exercised/lose weight (yes vs no) | 2.15 (1.52 - 3.03) | 2.15 (1.59 - 2.90) |
Eating disorder (yes vs no) | 1.52 (1.40 - 1.65) | 1.79 (1.64 - 1.95) |
Time watching TV (≥ 3 h/d) (yes vs no) | 1.25 (1.16 - 1.35) | 1.20 (1.13 - 1.28) |
Sports team engagement (yes vs no) | 0.65 (0.60 - 0.69) | 0.77 (0.72 - 0.82) |
HIV education in school (yes vs no) | 0.74 (0.59 - 0.92) | 0.93 (0.78 - 1.11) |
Random effects | ||
Random intercept | VC (SE), a% | VC (SE), % |
Level 4 (district) | 0.01 (0.00), 0.29 | 0.00 (0.00), 0.00 |
Level 3 (school) | 0.03 (0.01), 0.84 | 0.00 (0.00), 0.00 |
Level 2 (class) | 0.26 (0.02), 7.24 | 0.21 (0.02), 6.00 |
Race & Region Stratified Hierarchical Logistic Regression Analysis of the Association Between Adolescent Obesity and Demographics, Personality Behaviors, Substance Use and Health Behaviors Using Youth Risk Behavior Survey (YRBS) Data About Students in Grades 6 - 8 Attending Public Schools in Tennessee 2010
Variable | OR (95% CI) | |||
---|---|---|---|---|
WIA (n = 44716) | BHL (n = 7269) | AC (n = 42185) | Delta (n = 10953) | |
Demographics | ||||
Age | 0.92 (0.89 - 0.95) | 0.95 (0.90 - 1.02) | - | - |
Race (WIA vs BHL) | - | - | 1.10 (1.02 - 1.18) | 1.19 (1.04 - 1.35) |
Gender (male vs female) | 1.99 (1.87 - 2.12) | 1.41 (1.25 - 1.59) | 1.89 (1.79 - 2.01) | 1.59 (1.39 - 1.82) |
Region (Delta vs. other) | 1.04 (0.93 - 1.17) | 1.25 (1.00 - 1.57) | - | - |
Personality behaviors | ||||
Letter grades (D F vs A B) | 1.19 (1.06 - 1.34) | 0.84 (0.66 - 1.07) | 1.10 (0.99 - 1.23) | 1.17 (0.87 - 1.55) |
Seatbelt and helmet (yes vs no) | 0.08 (0.01 - 0.42) | 0.37 (0.01 - 9.28) | - | - |
Ever carried a weapon (yes vs no) | 0.99 (0.93 - 1.05) | 1.10 (0.96 - 1.27) | 1.01 (0.95 - 1.07) | 1.13 (0.98 - 1.30) |
Been in a physical fight (yes vs no) | 0.92 (0.86 - 0.97) | 0.92 (0.81 - 1.04) | 0.94 (0.89 - 1.00) | 0.81 (0.70 - 0.93) |
Injured in a fight (yes vs no) | 1.00 (0.89 - 1.13) | 0.81 (0.63 - 1.06) | 0.95 (0.85 - 1.06) | 0.98 (0.74 - 1.29) |
Substance use | ||||
Ever smoked (yes vs no) | 2.70 (1.83 - 3.98) | 1.66 (0.65 - 4.22) | - | - |
Early onset smoking (yes vs no) | 1.04 (0.94 - 1.16) | 0.99 (0.80 - 1.23) | 1.03 (0.93 - 1.14) | 1.16 (0.92 - 1.46) |
Early onset Alcohol (yes vs no) | 1.00 (0.92-1.09) | 1.07 (0.92 - 1.24) | 1.03 (0.95 - 1.11) | 0.96 (0.81 - 1.14) |
Early onset Marijuana (yes vs no) | 1.06 (0.89-1.26) | 0.87 (0.65 - 1.17) | 1.08 (0.92 - 1.26) | 0.79 (0.55 - 1.14) |
Health behaviors | ||||
Weight misperception (yes vs no) | 8.97 (8.40-9.57) | 12.12 (10.42 - 14.10) | 9.35 (8.77 - 9.97) | 9.86 (8.50 - 11.45) |
Exercised/lose weight (yes vs no) | 2.31 (1.79-2.98) | 1.61 (0.91 - 2.85) | - | - |
Eating disorder (yes vs no) | 1.55 (1.45-1.66) | 1.93 (1.70 - 2.20) | 1.65 (1.55 - 1.76) | 1.52 (1.31 - 1.76) |
Time watching TV (≥ 3 h/d) (yes vs no) | 1.28 (1.21-1.36) | 1.01(0.91 - 1.13) | 1.23 (1.16 - 1.29) | 1.25 (1.11 - 1.41) |
Sports team engagement (yes vs no) | 0.71 (0.68-0.75) | 0.68 (0.61 - 0.76) | 0.69 (0.65 - 0.73) | 0.81 (0.72 - 0.92) |
HIV education in school (yes vs no) | 0.83 (0.70-0.97) | 0.99 (0.68 - 1.43) | - | - |
Random effects | ||||
Random intercept | VC (SE), a% | VC (SE), % | VC (SE), % | VC (SE), % |
Level 4 (district) | 0.01 (0.00), 0.29 | 0.01 (0.01), 0.26 | 0.02 (0.00), 0.58 | 0.00 (0.00), 0.00 |
Level 3 (school) | 0.01 (0.01), 0.29 | 0.03 (0.03), 0.78 | 0.00 (0.00), 0.00 | 0.00 (0.00), 0.00 |
Level 2 (class) | 0.14 (0.01), 4.03 | 0.50 (0.06), 13.06 | 0.15 (0.01), 4.34 | 0.00 (0.00), 0.00 |
Age Stratified Hierarchical Logistic Regression Analysis of the Association Between Adolescent Obesity and Demographics, Personality Behaviors, Substance Use and Health Behaviors Using Youth Risk Behavior Survey (YRBS) Data about Students in Grades 6 - 8 Attending Public Schools in Tennessee 2010
Variable | OR (95% CI) | |||
---|---|---|---|---|
Age 11 (n = 5183) | Age 12 (n = 15703) | Age 13 (n = 17873) | Age 14 (n = 12492) | |
Demographics | ||||
Race (WIA vs BHL) | 0.98 (0.81 - 1.19) | 0.98 (0.87 - 1.11) | 1.18 (1.05 - 1.33) | 1.28 (1.11 - 1.48) |
Gender (male vs female) | 1.61 (1.37 - 1.88) | 1.84 (1.67 - 2.03) | 1.96 (1.78 - 2.15) | 1.99 (1.77 - 2.25) |
Region (Delta vs AC) | 1.03 (0.78 - 1.35) | 1.12 (0.94 - 1.33) | 0.94 (0.80 - 1.11) | 1.21 (1.00 - 1.47) |
Personality behaviors | ||||
Letter grades (D F vs A B) | 1.31 (0.89 - 1.92) | 0.87 (0.71 - 1.07) | 1.09 (0.91 - 1.31) | 1.42 (1.17 - 1.74) |
Seatbelt and helmet (yes vs no) | 0.00 (< 0.00 - 0.26) | 0.08(0.00 - 0.90) | 0.08 (0.00 - 0.78) | 0.12 (0.00 - 1.93) |
Ever carried a weapon (yes vs no) | 0.96 (0.80 - 1.14) | 1.04 (0.94 - 1.15) | 1.00 (0.91 - 1.10) | 1.08 (0.96 - 1.22) |
Been in a physical fight (yes vs no) | 1.02 (0.87 - 1.20) | 0.92 (0.83 - 1.01) | 0.97 (0.88 - 1.06) | 0.79 (0.70 - 0.89) |
Injured in a fight (yes vs no) | 1.35 (0.93 - 1.96) | 0.97 (0.80 - 1.19) | 0.79 (0.65 - 0.95) | 1.13 (0.91 - 1.40) |
Substance use | ||||
Ever Smoked (yes vs no) | 1.26 (0.49 - 3.24) | 2.36 (1.26 - 4.43) | 1.88 (1.03 - 3.43) | 4.51 (2.18 - 9.33) |
Early onset smoking (yes vs no) | 0.75 (0.52 - 1.06) | 0.97 (0.82 - 1.16) | 1.03 (0.87 - 1.21) | 1.22 (1.01 - 1.48) |
Early onset Alcohol (yes vs no) | 1.04 (0.83 - 1.30) | 1.00 (0.88 - 1.14) | 1.07 (0.95 - 1.21) | 0.92 (0.79 - 1.07) |
Early onset Marijuana (yes vs no) | 0.74 (0.39 - 1.41) | 1.04 (0.78 - 1.38) | 1.04 (0.81 - 1.34) | 1.15 (0.87 - 1.52) |
Health behaviors | ||||
Weight misperception (yes vs no) | 9.88 (8.14 - 11.98) | 8.72 (7.82 - 9.72) | 10.5 (9.5 - 11.65) | 10.06 (8.89 - 11.38) |
Exercised to lose weight (yes vs no) | 4.40 (2.15 - 8.98) | 2.25 (1.51-3.36) | 1.87 (1.25 - 2.79) | 1.90 (1.16 - 3.10) |
Eating disorder (yes vs no) | 1.48 (1.20 - 1.82) | 1.80 (1.61 - 2.02) | 1.67 (1.50 - 1.85) | 1.39 (1.23 - 1.57) |
Time watching TV(≥ 3 h/d) (yes vs no) | 1.65 (1.43 - 1.92) | 1.30 (1.19 - 1.42) | 1.23 (1.13 - 1.34) | 1.01 (0.91 - 1.12) |
Sports Team Engagement (yes vs no) | 0.74 (0.64 - 0.86) | 0.67 (0.61 - 0.73) | 0.80 (0.74 - 0.87) | 0.61 (0.55 - 0.67) |
HIV education in school (yes vs no) | 0.91 (0.62 - 1.34) | 0.92 (0.71 - 1.19) | 0.86 (0.68 - 1.09) | 0.88 (0.66 - 1.17) |
Random effects | ||||
Random intercept | VC (SE), a% | VC (SE), % | VC (SE), % | VC (SE), % |
Level 4 (district) | 0.02 (0.02), 0.53 | 0.00 (0.00), 0.00 | 0.01 (0.01), 0.28 | 0.02 (0.01), 0.56 |
Level 3 (school) | 0.00 (0.00), 0.00 | 0.07 (0.02), 1.97 | 0.00 (0.00), 0.00 | 0.00 (0.00), 0.00 |
Level 2 (class) | 0.45 (0.06), 11.97 | 0.20 (0.03), 5.62 | 0.29 (0.03), 8.08 | 0.24 (0.03), 6.76 |
Females in the Delta region were also more likely than females in the Appalachian and Central (AC) regions to be obese (data not shown). Table 2 also presents stratification analysis by race and region. Poor grades, wearing a seatbelt or helmet, physical fight, or smoking and HIV/AIDS education in school were all significantly associated with obesity among WIA adolescents. Obese WIA adolescents were also more likely to have exercised to lose weight and spent more than 3 hours a day watching TV. BHL adolescents in the Delta region were more likely to be obese compared to BHL adolescents living in other regions. The most significant health behaviors associated with adolescent obesity in BHL were weight misperception, eating disorder, or sports team involvement. The results in AC regions do not differ significantly from those observed within the Delta region.
Results of the multilevel logistic regression analysis for adolescent obesity stratified by age are shown in Table 3 (trends are presented in Figure 1). The odds ratio for being obese among BHL increases significantly from age 11 (OR = 0.98) to age 14 (OR = 1.28). As age increases, gender takes on a more significant role in adolescent obesity with older males having a greater likelihood of obesity. Results indicate a positive association of “ever tried smoking” with increasing age, with the most drastic change at age 14 (OR = 4.51). Weight misperception, exercising to lose weight, having an eating disorder, watching TV, and sports team engagement are also associated with obesity across all age groups, however, was no observable trend. Despite having a significant association in other models, results of the multilevel analyses do not support HIV/AIDS education as significantly associated with obesity in any of the age groups. When stratified by gender and race, BHL females were more likely to be obese compared to WIA females (data not shown). When comparing white AC region females with white AC region males, we see similar patterns of association. Both obese white females and males in these regions are more likely to: earn poorer grades in school, not use a seatbelt or helmet, carry a weapon; smoke, report misperceptions of weight, have an eating disorder, report exercising to lose weight, and spend more time watching TV when compared to their non-obese counterparts. They are also less likely to participate on a sports team. The only difference between the white males and females in AC region is that the proportion having not received HIV/AIDS education in school had a significant association with obesity in females (OR = 0.69, 95% CI: 0.54 - 0.89) but not in males (data not shown).
Age trends of odds ratios between adolescent obesity and race, gender, and smoking status
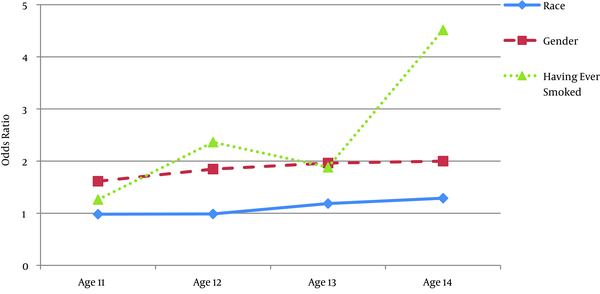
5. Discussion
Associations of health risk and protective behaviors with adolescent obesity in the State of Tennessee were examined, along with the role that the different levels of district, school, and class play in the prevalence of adolescent obesity. The use of a multilevel logistic regression model approach allowed for the identification of clusters of adolescent obesity and health risk behavior profiles that exist at district, school, classroom, and individual levels. The analyses provide important variations impacting micro areas directly which is important for prioritizing and directing intervention resources. This approach provides school systems with information at various levels and allows those systems to see how health risk and protective behaviors affect adolescent obesity, ultimately allowing for greater impact of public health interventions at all levels. Results indicate that 22.3% of the students in grades 6 - 8 surveyed were obese (BMI ≥ 95th percentile), which is significantly higher than the national proportion of 18.4% for the same age group (4). Males were more likely to be obese than females, as were BHL compared to WIA. Geographic region was associated with adolescent obesity, as students living in the Delta region had higher proportions of obesity than other regions.
Results from the multilevel logistic regression analysis demonstrated that several variables remained consistent in their significant association with adolescent obesity across all stratum. These well-established variables were smoking, weight misperception, eating disorder, time watching TV, and sports team engagement. No associations between adolescent obesity and the engagement in early substance use, such as tobacco, alcohol, or marijuana were detected. Males reported a significant association with poorer grades and obesity, whereas females not receiving HIV/AIDS education in school were more likely to be obese. This represents a noteworthy finding, while significant in some groups and not in others, may be related to characteristics of the groups, such as females being more cautious in their health risk behaviors. Additionally, a similar line of thought applies to the frequency of seat belt and helmet use. Not using a seat belt or helmet had a stronger association with obesity for males, although the association decreases in significance over time. This result is consistent with research that suggests adolescent injury-related behaviors may be associated with age and developmental stage and, as adolescents mature, early risks are replaced with other, escalating risk behaviors (12).
Study findings, when stratifying the students by age, reveal notable patterns in terms of risks behaviors and obesity. Gender and race play an increasingly important role in adolescent obesity as students age (Figure 1). These results may be explained by the influence of metabolic changes and physical growth experienced during puberty (29). Results also showed a trend across age groups for having ever tried smoking. The proportion of obese students reporting ever smoked increased from 28.76% at age 11 to 40.79% at age 16. This trend is consistent with the literature indicating that the initiation of smoking most commonly occurs among youth between the ages of 10 to 13 years (30). The relationship between ever smoked and obesity increased from age 11 to age 12, yet slightly decreased from 12 to 13, only to precipitously increase again from age 13 to 14. The drop in association could be attributed to students having tried smoking and then decided to quit, or other risk factors increasing in significance during that time. One such risk factor could be peer influence. In fact, research has shown the importance of peer group on smoking (20-25) and the sharp incline in the relationship of having ever smoked and obesity specifically among between ages 13 to 14.
5.1. Limitations
The main limitation of this study is that causality cannot be determined due to the cross-sectional study design. Further, height and weight values used to calculate BMI percentile were self-reported data, likely leading to an underestimation of obesity prevalence, thus impacting the associations found in the models. Other known risk factors for obesity, such as family income and parents’ education level, were not available for this study and could potentially influence study findings. Further, not all LEAs participated in the YRBS survey, which could mask important findings. Finally, the statistical modeling assumed homogeneity of schools and classes, which may not be accurate in the various levels of analyses conducted. Nevertheless, these findings can be used to guide the development of programs based at the district, school, class, and individual levels to target risk behaviors associated with adolescent obesity.
5.2. Conclusion
The relationship between obesity and health risk behaviors is complex. Findings from this study suggest that certain risk behaviors, such as having ever tired smoking, seat belt and helmet use, along with weight misperceptions, eating disorders, and sedentary behaviors, are associated with adolescent obesity. Future studies are necessary to understand how multiple health risk behaviors may bear a cumulative impact on the prevalence of obesity as adolescents mature. Continued research attention examining education efforts on adolescent obesity is warranted, given that educational exposure was found to be associated with obesity on the district, school, and class levels.
References
-
1.
Centers for Disease Control and Prevention. Overweight and obesity. Basics about childhood obesity. [cited June 11]. Available from: http://www.cdc.gov/obesity/childhood/basics.html.
-
2.
Centers for Disease Control and Prevention. Obesity Prevention. Healthy Schools. [cited July 24]. Available from: http://www.cdc.gov/healthyschools/obesity/facts.htm.
-
3.
Products - data briefs - number 219 - November 2015. [cited January 31]. Available from: https://www.cdc.gov/nchs/data/databriefs/db219.htm.
-
4.
Ogden CL, Carroll MD, Kit BK, Flegal KM. Prevalence of obesity in the United States, 2009-2010. NCHS Data Brief. 2012;(82):1-8. [PubMed ID: 22617494].
-
5.
Taveras EM, Gillman MW, Kleinman K, Rich-Edwards JW, Rifas-Shiman SL. Racial/ethnic differences in early-life risk factors for childhood obesity. Pediatrics. 2010;125(4):686-95. [PubMed ID: 20194284]. [PubMed Central ID: PMC3836212]. https://doi.org/10.1542/peds.2009-2100.
-
6.
Centers for Disease Control and Prevention. The obesity epidemic and united states students factsheet. [cited June 11]. Available from: http://www.cdc.gov/HealthyYouth/yrbs/pdf/us_obeisty_combo.pdf.
-
7.
Freedman DS, Mei Z, Srinivasan SR, Berenson GS, Dietz WH. Cardiovascular risk factors and excess adiposity among overweight children and adolescents: the Bogalusa Heart Study. J Pediatr. 2007;150(1):12-17 e2. [PubMed ID: 17188605]. https://doi.org/10.1016/j.jpeds.2006.08.042.
-
8.
Han JC, Lawlor DA, Kimm SY. Childhood obesity. Lancet. 2010;375(9727):1737-48. [PubMed ID: 20451244]. [PubMed Central ID: PMC3073855]. https://doi.org/10.1016/S0140-6736(10)60171-7.
-
9.
Naticchioni K. The relationship between obesity and academic achievement of school-age children [Thesis]. John Carroll University; 2013.
-
10.
Kann L, Kinchen S, Shanklin SL, Flint KH, Kawkins J, Harris WA, et al. Youth risk behavior surveillance--United States, 2013. MMWR Suppl. 2014;63(4):1-168. [PubMed ID: 24918634].
-
11.
Steinberg L. A social neuroscience perspective on adolescent risk-taking. Dev Rev. 2008;28(1):78-106. [PubMed ID: 18509515]. [PubMed Central ID: PMC2396566]. https://doi.org/10.1016/j.dr.2007.08.002.
-
12.
Aklin WM, Lejuez CW, Zvolensky MJ, Kahler CW, Gwadz M. Evaluation of behavioral measures of risk taking propensity with inner city adolescents. Behav Res Ther. 2005;43(2):215-28. [PubMed ID: 15629751]. https://doi.org/10.1016/j.brat.2003.12.007.
-
13.
Fitzgerald A, Fitzgerald N, Aherne C. Do peers matter? A review of peer and/or friends' influence on physical activity among American adolescents. J Adolesc. 2012;35(4):941-58. [PubMed ID: 22285398]. https://doi.org/10.1016/j.adolescence.2012.01.002.
-
14.
Centers for Disease Control and Prevention. 2010 youth risk behavior survey. [cited June 10]. Available from: www.cdc.gov/yrbs.
-
15.
Tennessee bicycle laws. Tennessee department of transportation. [cited June 26]. Available from: http://www.tn.gov/tdot/section/tennessee-bicycle-laws.
-
16.
Governors' highway safety association. Tennessee. [cited June 26]. Available from: http://www.ghsa.org/html/stateinfo/bystate/tn.html.
-
17.
Carpenter CS, Stehr M. The effects of mandatory seatbelt laws on seatbelt use, motor vehicle fatalities, and crash-related injuries among youths. J Health Econ. 2008;27(3):642-62. [PubMed ID: 18242744]. https://doi.org/10.1016/j.jhealeco.2007.09.010.
-
18.
Ahmad S, Billimek J. Limiting youth access to tobacco: comparing the long-term health impacts of increasing cigarette excise taxes and raising the legal smoking age to 21 in the United States. Health Policy. 2007;80(3):378-91. [PubMed ID: 16698112]. https://doi.org/10.1016/j.healthpol.2006.04.001.
-
19.
Kessel Schneider S, Buka SL, Dash K, Winickoff JP, O'Donnell L. Community reductions in youth smoking after raising the minimum tobacco sales age to 21. Tob Control. 2016;25(3):355-9. [PubMed ID: 26071428]. https://doi.org/10.1136/tobaccocontrol-2014-052207.
-
20.
Leatherdale ST, Cole A. Examining the impact of changes in school tobacco control policies and programs on current smoking and susceptibility to future smoking among youth in the first two years of the COMPASS study: looking back to move forward. Tob Induc Dis. 2015;13(1):8. [PubMed ID: 25834482]. [PubMed Central ID: PMC4381407]. https://doi.org/10.1186/s12971-015-0031-1.
-
21.
Cambron C, Kosterman R, Catalano RF, Guttmannova K, Hawkins JD. Neighborhood, family, and peer factors associated with early adolescent smoking and alcohol use. J Youth Adolesc. 2018;47(2):369-82. [PubMed ID: 28819911]. [PubMed Central ID: PMC5790639]. https://doi.org/10.1007/s10964-017-0728-y.
-
22.
Miller JW, Naimi TS, Brewer RD, Jones SE. Binge drinking and associated health risk behaviors among high school students. Pediatrics. 2007;119(1):76-85. [PubMed ID: 17200273]. https://doi.org/10.1542/peds.2006-1517.
-
23.
Tennessee Department of Education. Coordinated School Health. [cited June 23]. Available from: http://www.tn.gov/education/topic/coordinated-school-health.
-
24.
Wellman RJ, Dugas EN, Dutczak H, O'Loughlin EK, Datta GD, Lauzon B, et al. Predictors of the Onset of Cigarette Smoking: A Systematic Review of Longitudinal Population-Based Studies in Youth. Am J Prev Med. 2016;51(5):767-78. [PubMed ID: 27180028]. https://doi.org/10.1016/j.amepre.2016.04.003.
-
25.
Peretti-Watel P, Seror V, Verger P, Guignard R, Legleye S, Beck F. Smokers' risk perception, socioeconomic status and source of information on cancer. Addict Behav. 2014;39(9):1304-10. [PubMed ID: 24836161]. https://doi.org/10.1016/j.addbeh.2014.04.016.
-
26.
Appalachian Regional Commission. The Appalachian region. [cited June 23]. Available from: http://www.arc.gov/counties.
-
27.
Eaton DK, Kann L, Kinchen S, Shanklin S, Ross J, Hawkins J, et al. Youth risk behavior surveillance - United States, 2009. MMWR Surveill Summ. 2010;59(5):1-142. [PubMed ID: 20520591].
-
28.
SAS® 9.4. Cary,. NC: SAS Institute Inc; 2018.
-
29.
Jasik CB, Lustig RH. Adolescent obesity and puberty: the "perfect storm". Ann N Y Acad Sci. 2008;1135:265-79. [PubMed ID: 18574233]. https://doi.org/10.1196/annals.1429.009.
-
30.
Daw J, Margolis R, Wright L. Emerging adulthood, emergent health lifestyles: Sociodemographic determinants of trajectories of smoking, binge drinking, obesity, and sedentary behavior. J Health Soc Behav. 2017;58(2):181-97. [PubMed ID: 28661779]. [PubMed Central ID: PMC5894852]. https://doi.org/10.1177/0022146517702421.