Abstract
Background:
Tuberculosis is known as one of the most dangerous diseases caused by Mycobacterium tuberculosis. Although different strategies have been applied to prevent this disease, it is still considered a killer disease in the world.Objectives:
This project was conducted to design a novel poly-epitope vaccine based on three antigenic proteins against tuberculosis.Methods:
To design a poly-epitope vaccine, first, the antigenic proteins of Mycobacterium tuberculosis, including Dnak, FbpA, and katG were selected from the database. Then, B cell, MHCI, and MHCII epitopes of the antigenic proteins were predicted using reliable online tools. The best-predicted epitopes were applied to assemble a poly-epitope vaccine. The physicochemical features, the antigenicity of the whole vaccine, and the protein structures of the designed poly-epitope vaccine were evaluated by the most precise tools. Also, the coding DNA sequence of the vaccine was adapted for expression in the prokaryotic system, then, it was theoretically cloned in pET32a (+) vector.Results:
The results revealed that the molecular weight and length of the designed poly-epitope vaccine were 32 kDa and 308 amino acids, respectively. The protein structure results demonstrated that the designed poly-epitope vaccine contained 19.48% alpha-helix and 73.05% random coil. Also, the results showed that 92.2% of amino acid residues were located in the favored region. Finally, it was clarified that the antigenicity of the designed poly-epitope vaccine was 12333.Conclusions:
According to the results of the current project, it seems that the designed poly-epitope vaccine can be an appropriate candidate to control tuberculosis.Keywords
1. Background
Mycobacterium tuberculosis (M. tuberculosis) is an intracellular pathogen that leads to tuberculosis disease. This bacterium is also known as Koch Bacillus because it was first identified by Robert Koch (1, 2). Tuberculosis can cause intense symptoms through lung infection, such as fever, cough, and even death (3). It has been reported that the prevalence and mortality rate of this disease have a direct relationship with the AIDS pandemic so that one-third of the world population has shown an infection with M. tuberculosis (1, 2). Accordingly, appropriate vaccines should be applied to control this important disease. Although the Bacille Calmette–Guérin (BCG) vaccine has been able to prevent tuberculosis in most countries, the existence of some problems, such as diverse protection in young and adult people leads to a decrease in applying this vaccine (4-8). Consequently, the identification of an efficient strategy to prevent tuberculosis is very crucial. Poly-epitope or multi-epitope vaccine is a new generation of vaccine which uses different epitopes of the antigenic proteins instead of the whole microorganism (9). The poly-epitope vaccine is a safe and cost-effective strategy that can induce protection without diversity in different hosts (10-12). In general, engineering a poly-epitope vaccine has two important steps: (i) identification of antigenic protein, and (ii) epitope prediction (13, 14). The vaccine investigation and online information network (VIOLIN) database can be applied to identify antigenic proteins as a reliable source. This database has been developed based on literature review and consists of the most antigenic proteins of different pathogens (15, 16). Also, epitope identification is considered one of the most vital phases of poly-epitope vaccine designing. Epitopes are short amino acid sequences that can stimulate the immune system via binding to different cells (17). So far, many online tools have been developed by bioinformatics science for epitope prediction. These tools not only can predict B cell epitopes but also can identify MHCI and MHCII epitopes (18, 19). Bioinformatics which has been created based on computer, biology, and statistics sciences is extensively being applied in different areas of biology. The success of this science is related to its speed, accuracy, and affordability (20, 21). This study was conducted to design an efficient poly-epitope vaccine against M. tuberculosis infection. In this case, the most antigenic proteins of this pathogen, including, FbpA, katG, and Dnak were extracted from the VIOLIN database. Then, the best B cell, MHCI, and MHCII epitopes of the antigenic proteins were predicted by the most accurate and reliable online tools.
2. Objectives
The current project was conducted to design a novel poly-epitope vaccine against tuberculosis.
3. Methods
3.1. Amino Acid Sequence Collection
The present study collected the amino acid sequences of the FbpA, katG, and Dnak proteins from UniProt (https://www.uniprot.org/) database. The accession numbers of FbpA, katG, and Dnak proteins were P9WQP3, P9WIE5, and P9WMJ9, respectively.
3.2. MHCI and MHCII Epitopes Prediction
We used the IEBD (http://tools.iedb.org/main/tcell/) server to predict the primary MHCI and MHCII epitopes of the FbpA, katG, and Dnak proteins. We also employed HLA-A*01:01 and DRB1*01:01 alleles to predict MCHI and MHCII epitope, respectively. It is critical to note that the server was adjusted based on its default. To select final epitopes, antigenicity of the primary epitopes was investigated by VaxiJen server (http://www.ddg-pharmfac.net/vaxijen/VaxiJen/VaxiJen.html) and the epitopes with high antigenicity score were considered as the best epitopes.
3.3. B Cell Epitope Prediction
The BCPREDS server (http://ailab-projects1.ist.psu.edu:8080/bcpred/) was used to identify the primary B cell epitopes of FbpA, katG, and Dnak proteins. The prediction was performed based on the default of the server. The best B cell epitopes were selected among the primary epitopes using antigenicity score (similar to MHCI and MHCII epitopes).
3.4. Poly-Epitope Vaccine Designing
To design a novel poly-epitope vaccine, the best MHCI, MHCII and B cell epitopes of FbpA, katG and Dnak proteins were applied. The epitopes were used to construct three fragments, each of which contained special epitopes. Appropriate linkers were embedded among different epitopes and fragments to increase the efficiency of the designed poly-epitope vaccine.
3.5. Physicochemical Features and Antigenicity of the Poly-Epitope Vaccine
The ProtParam server was applied (https://web.expasy.org/protparam/) to evaluate the physicochemical features of the designed poly-epitope vaccine, including molecular weight, theoretical pI, length, instability index, aliphatic index, and grand average of hydropathicity (GRAVY). In this case, an amino acid sequence of the designed poly-epitope vaccine was submitted to the server, and the antigenicity of the designed poly-epitope vaccine was assessed by the VaxiJen server.
3.6. Secondary Structure of the Designed Poly-Epitope Vaccine
The GOR4 server (https://npsa-prabi.ibcp.fr/cgi-bin/npsa_automat.pl?page=/NPSA/npsa_gor4.html) was used for the determination of the percentage of alpha-helix, extended strand, beta-turn, and random coil of the poly-epitope vaccine. To predict secondary structure, a protein sequence of the designed poly-epitope vaccine was pasted into the server.
3.7. Tertiary Structure of the Designed Poly-Epitope Vaccine
We used the I-TASSER server (https://zhanglab.ccmb.med.umich.edu/I-TASSER/) to model the raw tertiary structure of the designed poly-epitope vaccine. In this case, an amino acid sequence of the vaccine was submitted to the server, and the raw tertiary structure was selected based on the C score. The raw tertiary structure was refined by GalaxyRefine server (http://galaxy.seoklab.org/cgi-bin/submit.cgi?type=REFINE), and the best model was chosen using an interpretation of Ramachandran plots. It is critical to note that Ramachandran plots were created by the MolProbity server (http://molprobity.biochem.duke.edu/index.php).
3.8. Codon Adaptation and in Silico Cloning
First of all, the reverse translation of the vaccine protein sequence was performed by CLC Main workbench software to adapt the coding DNA sequence of the designed poly-epitope vaccine. Then, the achieved coding DAN sequence was adapted by JCat online tool (http://www.jcat.de/Start.jsp) for the expression in the prokaryotic system. Also, NcoI and XhoI restriction enzymes were added at 5´ and 3´ terminals of the coding DNA sequence, respectively, to perform in silico cloning. The prepared insert fragment was cloned in multiple cloning site of the pET32a (+) vector. Notably, two nucleotides were added before the restriction site of the NcoI enzyme to keep the open reading frame (ORF) of the insert fragment.
4. Results
4.1. Epitope Prediction
We applied the most reliable online tools to predict the best MHCI, MHCII, and B cell epitopes of FbpA, katG, and Dnak proteins. The results revealed that 77 - 86, 595 - 607, and 338 - 352 amino acid residues of the Dnak protein were the best MHCI, B cell, and MHCII epitopes, respectively (Table 1). Moreover, the best MHCI, B cell, and MHCII epitopes of FbpA proteins were located in 278 - 287, 253 - 266, and 167 - 181 amino acid residues, respectively (Table, 1). Finally, 379 - 390, 118 - 131, and 43 - 57 amino acid residues of KatG protein were considered as the best MHCI, B cell, and MHCII epitopes of this protein (Table 1).
List of the Best Predicted B Cell, MHCI and MHCII Epitopes of the Antigenic Proteins
Antigen | Rank | Epitope Type | Sequence | Start Position | End Position | Antigenicity Score |
---|---|---|---|---|---|---|
Dnak | 1 | B cell | AAHPGGEPGGAHPG | 594 | 607 | 1.18 |
Dnak | 2 | B cell | VFDLGGGTFDVSLL | 168 | 181 | 0.92 |
Dnak | 3 | B cell | KGVNPDEVVAVGAA | 337 | 350 | 0.65 |
FbpA | 1 | B cell | YCGNGKPSDLGGNN | 253 | 266 | 1.78 |
FbpA | 2 | B cell | LGATPNTGPAPQGA | 325 | 338 | 0.81 |
FbpA | 3 | B cell | GGGHNGVFDFPDSG | 290 | 303 | 0.71 |
katG | 1 | B cell | GRGGAGGGMQRFAP | 118 | 131 | 2.46 |
katG | 2 | B cell | HGAGPADLVGPEPE | 276 | 289 | 1.14 |
katG | 3 | B cell | PFTPGRTDASQEQT | 566 | 579 | 0.88 |
Dnak | 1 | MHCI | WSIEIDGKKY | 77 | 86 | 2.34 |
Dnak | 2 | MHCI | TADDNQPSVQIQVY | 402 | 415 | 1.36 |
Dnak | 3 | MHCI | EADVRNQAETLVY | 506 | 518 | 0.73 |
FbpA | 1 | MHCI | TSNIKFQDAY | 278 | 287 | 1.43 |
FbpA | 2 | MHCI | DSGTHSWEY | 301 | 309 | 1.05 |
FbpA | 3 | MHCI | SSALTLAIY | 173 | 181 | 0.99 |
katG | 1 | MHCI | ATDLSLRVDPIY | 379 | 390 | 1.63 |
katG | 2 | MHCI | QTDVESFAV | 578 | 586 | 1.03 |
katG | 3 | MHCI | VADPMGAAFDY | 54 | 64 | 0.52 |
Dnak | 1 | MHCII | GVNPDEVVAVGAALQ | 338 | 352 | 0.71 |
Dnak | 2 | MHCII | QAIYEAAQAASQATG | 579 | 593 | 0.51 |
Dnak | 3 | MHCII | PDEVVAVGAALQAGV | 341 | 355 | 0.41 |
FbpA | 1 | MHCII | GLSMAASSALTLAIY | 167 | 181 | 0.89 |
FbpA | 2 | MHCII | LPVEYLQVPSPSMGR | 49 | 63 | 0.79 |
FbpA | 3 | MHCII | ANSPALYLLDGLRAQ | 74 | 88 | 0.44 |
katG | 1 | MHCII | LNLKVLHQNPAVADP | 43 | 57 | 1.18 |
katG | 2 | MHCII | GPLFIRMAWHAAGTY | 99 | 113 | 0.86 |
katG | 3 | MHCII | KANLLTLSAPEMTVL | 613 | 627 | 0.45 |
4.2. Poly-Epitope Vaccine Designing
The designed poly-epitope vaccine from N-terminal to C-terminal contained MHCI, B cell, and MHCII fragments, respectively. In fact, these fragments were made using the first rank MHCI, B cell, and MHCII epitopes of Dnak FbpA, and katG proteins reported in Table 1. Each epitope was repeated twice in each fragment to increase the antigenicity score of the designed poly-epitope vaccine. The KPKP linker was embedded among different epitopes of each fragment, whereas the EAAAK linker was intercalated among different fragments (Figure 1A).
(A) Construct of the designed poly-epitope vaccine, MHCI, B cell, and MHCII fragments have been shown with green, gray and blue colors, respectively. (B) Secondary structure of the designed poly-epitope vaccine, different regions have been shown with different colors.
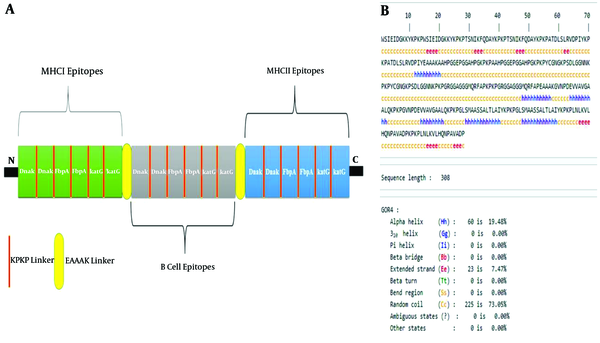
4.3. Physicochemical Features and Antigenicity of the Poly-Epitope Vaccine
The results of the physicochemical analysis revealed that the molecular weight of the designed poly-epitope vaccine was 32 kDa, and this vaccine had 308 amino acids in length. Also, the results showed that theoretical pI, instability index, aliphatic index, and GRAVY of the designed poly-epitope vaccine were 9.91, 15.95, 63.51, and -0.695, respectively. The result of the antigenicity demonstrated that the antigenicity score of the designed poly-epitope vaccine was 1.21.
4.4. Secondary Structure of the Designed Poly-Epitope Vaccine
For the secondary structure of the designed poly-epitope vaccine, we used the GOR4 server. The results of this server clarified that the protein sequence of the vaccine contained 19.48% alpha-helix, 7.470% extended strand 0.0% beta-turn, and 73.05% random coil (Figure 1B).
4.5. Tertiary Structure of the Designed Poly-Epitope Vaccine
As noted, to model the primary tertiary structure of the designed poly-epitope vaccine I-TASSER server was applied, C score of this model was -2.46 (Figure 2A). The refinement of the designed poly-epitope vaccine was conducted by the GalaxyRefine server. The results showed that in the primary model, 55.2% of the amino acid residues were located in the favored region, but in the refined model, 92.2% of amino acid residues were in the favored region (Figure 2B).
(A) Cartoon (left- hand) and sphere (right-hand) models of the designed poly-epitope vaccine which were visualized by PyMol software. (B) Ramachandran plots of the raw (1) and the refined (2) models of the vaccine. Compared with the primary model, in the refined model most amino acid residues have been located in favored region.
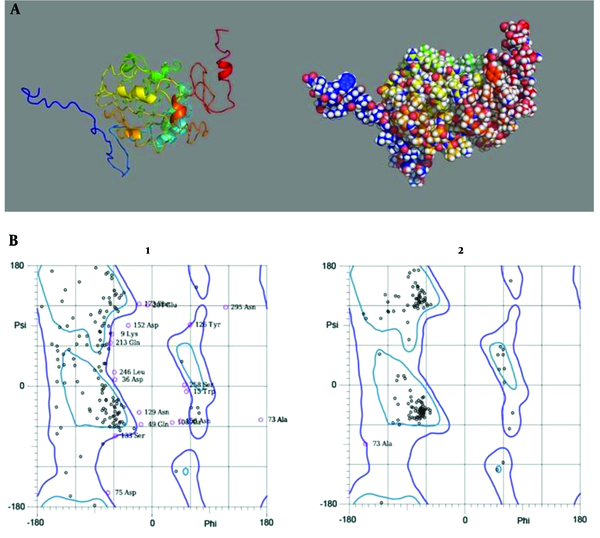
4.6. Codon Adaptation and in Silico Cloning
The results of codon adaptation showed that the codon adaptation index (CAI) and GC content of the coding DNA sequence reached 1 and 54.32%, respectively (Figure 3). Moreover, the results of in silico cloning revealed that the coding DNA sequence of the designed poly-epitope vaccine was successfully cloned in pET32a (+) vector (Figure 4).
Codon Adaptation plot of the vaccine coding DNA sequence before (left-hand) and after (right-hand) codon adaptation.
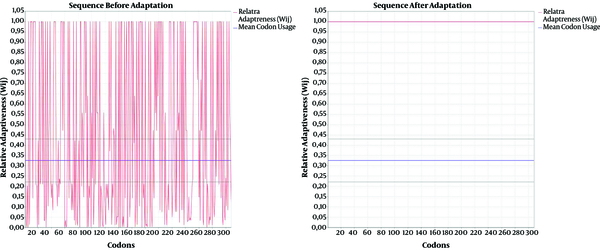
In silico cloning of coding DNA sequence of the designed poly-epitope vaccine in pET32a (+) vector. (A) Digested fragment, (B) pET32a (+) along with inserted gene (blue region).
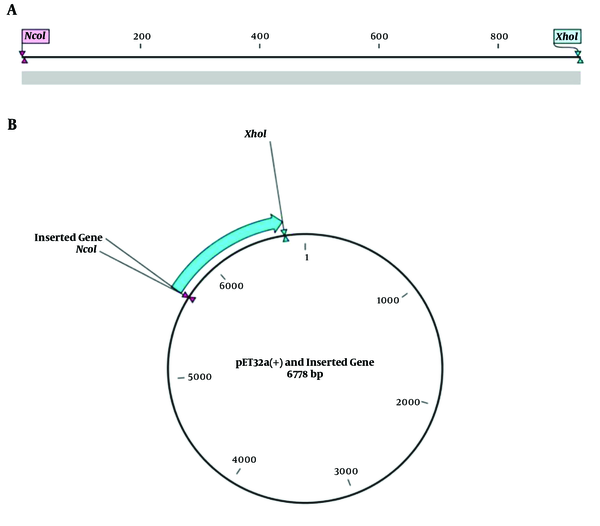
5. Discussion
Immunoinformatics science has recently been considered as one of the most powerful tools used for simulation, prediction, and analysis of the immune system (22). Vaccine development based on genomic and proteomic data is the most critical application of the immunoinformatics approach. Many studies have used this strategy for vaccine designing against different infectious diseases (15, 16, 23). In the current project, we used this strategy to design an efficient poly-epitope vaccine against M. tuberculosis infection. In this case, initially, the most critical antigenic proteins of M. tuberculosis, including FbpA, katG, and Dnak were selected from the VIOLIN database and were used for B cell and T cell epitopes prediction. Low immunogenicity is known as one of the biggest weaknesses of the poly-epitope vaccines, as a consequence to increase antigenicity of these vaccines, some strategies should be considered (24). In the current project, we employed two strategies to increase the antigenicity of the designed poly-epitope vaccine. First, the best B cell and T cell epitopes of FbpA, katG, and Dnak proteins were filtered among primary epitopes based on their antigenicity score. The epitopes with the highest antigenicity were considered as the best epitopes. Second, the rank-one epitopes, which had the highest antigenicity score, were repeated in the designed poly-epitope vaccine. The poly-epitope vaccine, which contains both B cell and T cell epitopes can not only stimulate humoral immunity but also trigger cellular immunity. In view of our designed poly-epitope vaccine consisting of B cell and T cell epitopes, it will be able to stimulate strong immune responses. The selection of appropriate linkers can maintain vaccine functionality. In this study, we used two prominent linkers (EAAAK and KPKP). To evaluate the efficiency of the designed poly-epitope vaccine, its different physicochemical features, including molecular weight, theoretical pI, length, instability index, aliphatic index, and grand GRAVY were assessed. The results of the physicochemical evaluation showed that the molecular weight of the designed poly-epitope vaccine was 32 kDa. It has been revealed that a protein with a molecular weight less than 10 kDa is deleted from the body using the renal system, whereas a protein with a molecular weight of more than 10 kDa can escape from this system (25). Hence, it can be claimed that molecular weight has a direct association with the half-life of a protein. The stability of a protein is investigated by its instability index. In general, stable proteins can keep their folding in different conditions. While, instability index is > 40, instability of the protein is confirmed (26-28). In the next steps, the secondary and tertiary structures of the designed poly-epitope vaccine were examined. The results of the secondary structure demonstrated that our designed vaccine contained 73.05% random coil. It has been reported that the random coil of a protein is related to the exposure rate of regions that are on the protein surface. Hence, it seems that the majority of epitopes that were intercalated at the designed poly-epitope vaccine can be identified by the immune system of the host. Also, the results of the tertiary structure revealed that the designed poly-epitope vaccine was well modeled (the C score of the model was -2.46). The results of refinement showed that more than 90% of the vaccine amino acid residues were in the favored region and 98% of amino acid residues were in the allowed region. Finally, the results showed that the codon adapted sequence of the designed poly-epitope vaccine was successfully cloned in pET32a (+) vector for expression in a prokaryotic system such as E. coli.
5.1. Conclusion
The current project identified the best B cell and T cell epitopes of three antigenic proteins of M. tuberculosis to design a novel poly-epitope vaccine. It assessed different physicochemical, protein structure features, codon adaptation, and in silico cloning of the designed poly-epitope vaccine via the most reliable tools. According to the results, the designed vaccine can be an appropriate candidate to prevent M. tuberculosis infection. It must be mentioned that these results need to be experimentally confirmed.
References
-
1.
Fogel N. Tuberculosis: a disease without boundaries. Tuberculosis (Edinb). 2015;95(5):527-31. [PubMed ID: 26198113]. https://doi.org/10.1016/j.tube.2015.05.017.
-
2.
Keshavjee S, Farmer PE. Tuberculosis, drug resistance, and the history of modern medicine. N Engl J Med. 2012;367(10):931-6. [PubMed ID: 22931261]. https://doi.org/10.1056/NEJMra1205429.
-
3.
Comas I, Gagneux S. The past and future of tuberculosis research. PLoS Pathog. 2009;5(10). e1000600. [PubMed ID: 19855821]. [PubMed Central ID: PMC2745564]. https://doi.org/10.1371/journal.ppat.1000600.
-
4.
Colditz GA, Berkey CS, Mosteller F, Brewer TF, Wilson ME, Burdick E, et al. The efficacy of bacillus Calmette-Guerin vaccination of newborns and infants in the prevention of tuberculosis: meta-analyses of the published literature. Pediatrics. 1995;96(1 Pt 1):29-35. [PubMed ID: 7596718].
-
5.
Davenne T, McShane H. Why don't we have an effective tuberculosis vaccine yet? Expert Rev Vaccines. 2016;15(8):1009-13. [PubMed ID: 27010255]. [PubMed Central ID: PMC4950406]. https://doi.org/10.1586/14760584.2016.1170599.
-
6.
Fine PE. Variation in protection by BCG: implications of and for heterologous immunity. Lancet. 1995;346(8986):1339-45. [PubMed ID: 7475776]. https://doi.org/10.1016/s0140-6736(95)92348-9.
-
7.
Rodrigues LC, Diwan VK, Wheeler JG. Protective effect of BCG against tuberculous meningitis and miliary tuberculosis: a meta-analysis. Int J Epidemiol. 1993;22(6):1154-8. [PubMed ID: 8144299]. https://doi.org/10.1093/ije/22.6.1154.
-
8.
Trunz BB, Fine P, Dye C. Effect of BCG vaccination on childhood tuberculous meningitis and miliary tuberculosis worldwide: a meta-analysis and assessment of cost-effectiveness. Lancet. 2006;367(9517):1173-80. [PubMed ID: 16616560]. https://doi.org/10.1016/S0140-6736(06)68507-3.
-
9.
Zhang L. Multi-epitope vaccines: a promising strategy against tumors and viral infections. Cell Mol Immunol. 2018;15(2):182-4. [PubMed ID: 28890542]. [PubMed Central ID: PMC5811687]. https://doi.org/10.1038/cmi.2017.92.
-
10.
Naz A, Shahid F, Butt TT, Awan FM, Ali A, Malik A. Designing multi-epitope vaccines to combat emerging coronavirus disease 2019 (COVID-19) by employing immuno-informatics approach. Front Immunol. 2020;11:1663. [PubMed ID: 32754160]. [PubMed Central ID: PMC7365865]. https://doi.org/10.3389/fimmu.2020.01663.
-
11.
Oyarzun P, Ellis JJ, Gonzalez-Galarza FF, Jones AR, Middleton D, Boden M, et al. A bioinformatics tool for epitope-based vaccine design that accounts for human ethnic diversity: application to emerging infectious diseases. Vaccine. 2015;33(10):1267-73. [PubMed ID: 25629524]. https://doi.org/10.1016/j.vaccine.2015.01.040.
-
12.
Patronov A, Doytchinova I. T-cell epitope vaccine design by immunoinformatics. Open Biol. 2013;3(1):120139. [PubMed ID: 23303307]. [PubMed Central ID: PMC3603454]. https://doi.org/10.1098/rsob.120139.
-
13.
Cherryholmes GA, Stanton SE, Disis ML. Current methods of epitope identification for cancer vaccine design. Vaccine. 2015;33(51):7408-14. [PubMed ID: 26238725]. https://doi.org/10.1016/j.vaccine.2015.06.116.
-
14.
Yin D, Li L, Song X, Li H, Wang J, Ju W, et al. A novel multi-epitope recombined protein for diagnosis of human brucellosis. BMC Infect Dis. 2016;16:219. [PubMed ID: 27206475]. [PubMed Central ID: PMC4875615]. https://doi.org/10.1186/s12879-016-1552-9.
-
15.
Jaydari A, Nazifi N, Forouharmehr A. Computational design of a novel multi-epitope vaccine against Coxiella burnetii. Hum Immunol. 2020;81(10-11):596-605. [PubMed ID: 32718721]. https://doi.org/10.1016/j.humimm.2020.05.010.
-
16.
Shams N, Shakarami Gandabeh Z, Nazifi N, Forouharmehr A, Jaydari A, Rashidian E. Computational design of different epitope-based vaccines against Salmonella typhi. Int J Pept Res Ther. 2019;26(3):1527-39. https://doi.org/10.1007/s10989-019-09959-4.
-
17.
Nazifi N, Mousavi SM, Moradi S, Jaydari A, Jahandar MH, Forouharmehr A. In silico B cell and T cell epitopes evaluation of lipL32 and OmpL1 proteins for designing a recombinant multi-epitope vaccine against leptospirosis. Int J Infect Dis. 2018;5(2). https://doi.org/10.5812/iji.63255.
-
18.
Soria-Guerra RE, Nieto-Gomez R, Govea-Alonso DO, Rosales-Mendoza S. An overview of bioinformatics tools for epitope prediction: implications on vaccine development. J Biomed Inform. 2015;53:405-14. [PubMed ID: 25464113]. https://doi.org/10.1016/j.jbi.2014.11.003.
-
19.
Sun P, Ju H, Liu Z, Ning Q, Zhang J, Zhao X, et al. Bioinformatics resources and tools for conformational B-cell epitope prediction. Comput Math Methods Med. 2013;2013:943636. [PubMed ID: 23970944]. [PubMed Central ID: PMC3736542]. https://doi.org/10.1155/2013/943636.
-
20.
Andreini C, Bertini I, Rosato A. Metalloproteomes: a bioinformatic approach. Acc Chem Res. 2009;42(10):1471-9. [PubMed ID: 19697929]. https://doi.org/10.1021/ar900015x.
-
21.
Leipzig J. A review of bioinformatic pipeline frameworks. Brief Bioinform. 2017;18(3):530-6. [PubMed ID: 27013646]. [PubMed Central ID: PMC5429012]. https://doi.org/10.1093/bib/bbw020.
-
22.
Petrovsky N, Brusic V. Computational immunology: The coming of age. Immunol Cell Biol. 2002;80(3):248-54. [PubMed ID: 12067412]. https://doi.org/10.1046/j.1440-1711.2002.01093.x.
-
23.
Rashidian E, Gandabeh ZS, Forouharmehr A, Nazifi N, Shams N, Jaydari A. Immunoinformatics approach to engineer a potent poly-epitope fusion protein vaccine against Coxiella burnetii. Int J Pept Res Ther. 2020;26(4):2191-201. https://doi.org/10.1007/s10989-019-10013-6.
-
24.
Goumari MM, Farhani I, Nezafat N, Mahmoodi S. Multi-epitope vaccines (MEVS), as a novel strategy against infectious diseases. Current Proteomics. 2020;17(5):354-64. https://doi.org/10.2174/1570164617666190919120140.
-
25.
Jaydari A, Forouharmehr A, Nazifi N. Determination of immunodominant scaffolds of Com1 and OmpH antigens of Coxiella burnetii. Microb Pathog. 2019;126:298-309. [PubMed ID: 30447420]. https://doi.org/10.1016/j.micpath.2018.11.012.
-
26.
Forouharmehr A, Nassiri M, Ghovvati S, Javadmanesh A. Evaluation of different signal peptides for secretory production of recombinant bovine pancreatic ribonuclease a in gram negative bacterial system: An in silico study. Current Proteomics. 2018;15(1). https://doi.org/10.2174/1570164614666170725144424.
-
27.
Ghovvati S, Pezeshkian Z, Mirhoseini SZ. In silico analysis of different signal peptides to discover a panel of appropriate signal peptides for secretory production of Interferon-beta 1b in Escherichia coli. Acta Biochim Pol. 2018;65(4):521-34. [PubMed ID: 30378597]. https://doi.org/10.18388/abp.2018_2351.
-
28.
Vahedi F, Nassiri M, Ghovvati S, Javadmanesh A. Evaluation of different signal peptides using bioinformatics tools to express recombinant erythropoietin in mammalian cells. International Journal of Peptide Research and Therapeutics. 2018;25(3):989-95. https://doi.org/10.1007/s10989-018-9746-1.