Abstract
Background:
Suicide risk factors can be used to develop tools for suicide attempt prediction and prevention.Objectives:
We aimed to design a model to evaluate the risk of suicide related to socio-economic, demographic, health, and drug dependency factors.Methods:
This case-control study was conducted in a 15-65-year-old population of Golestan province, Iran. The case group included 414 individuals with a history of suicide in 2019, and the control group had 408 individuals without suicide attempts. Demographic, psychosocial health, and drug dependency data were collected. Modeling was carried out using multivariate logistic regression. The performance of suicide-predicting models was assessed, and a nomogram for the probability of suicide was drawn.Results:
A multivariate logistic regression model with age, gender, education level, mother's education level, marital status, life satisfaction, membership in cyberspace, sleep disorders, alcohol abuse, having suicidal thoughts, the interaction of gender with life satisfaction, and the interaction of gender with mother's education level was the best predicting model of suicide attempt (AUC = 0.934, CI: 0.91 - 0.95). The variables of father's education level, occupation, job satisfaction, household size, financial status, regular exercise, guardianship status, history of self-harm, history of suicide attempt in the family, smoking and drug abuse had no significant relationship with suicide attempt.5.1. Conclusions:
The results suggest that designed models can help mental health service providers to identify high-risk individuals early. So we can better manage suicide and reduce its economic, social, and health burdens.Keywords
1. Background
Suicide is a significant health and social concern worldwide (1), and its prevention is a priority for mental health officials (2). It is a complex multi-causal behavior affected by various individual, familial, social, economic, and cultural factors (3). WHO reports over 800,000 deaths due to suicide per year in the world, but the number of suicide attempts is 20 times more. Estimates show that every two seconds, one person commits suicide worldwide, and one person dies due to suicide every 40 seconds (1). The suicide rate is higher in developed countries, but almost 75% of suicide cases occur in low-to-middle-income countries (4). In Iran, suicide rates are lower than in Western countries but higher than in Eastern Mediterranean and the Middle East (5).
Contrary to world statistics, data obtained in Iran indicate increasing rates of suicide death among women and girls and an increasing suicide death ratio in women than in men (6). Suicide attempts can harm families, friends, colleagues, and societies (7). A suicide attempt can severely affect at least five friends and relatives of the committer (8). The history of a suicide attempt is the most critical risk factor for suicide (9). Therefore, finding risk factors predicting suicide attempts has been favored by officials and health service providers.
It is interesting to use suicide risk factors to design risk assessment tools or predictive scales. There are few studies on suicide with such approaches. For example, Lee et al. created a suicide model in 2009 with a good predictive capability for suicide attempts in the Korean adolescent population (10). Risk-predictive composite tools with the ability to predict suicide can be utilized by a wide range of general practitioners and psychiatrists (2) because statistics show that at least 25% of people had visited a psychiatrist, and more than 25% had visited a general practitioner before committing suicide (11).
2. Objectives
This study was done to identify suicide risk factors and develop a predictive model for suicide attempts to find at-risk people early to prevent death from suicide.
3. Methods
3.1. Study Population
This case-control study was conducted on the population living in Golestan province, Iran (Ethics code: IR.SBMU.PHNS.REC.1399.162). The case group included 414 people with a history of suicide attempts, and the control group had 408 individuals without suicide attempts. The cases were selected by systematic random sampling among all attempted suicides in 2019 using the health system information. The controls were chosen via systematic random sampling and using the data obtained from the same system after excluding people with a history of suicide attempts. Age between 15 and 65 was the inclusion criterion.
3.2. Data Collection Method
As suicide attempt is a complex multi-causal behavior with various influential factors (1), after a comprehensive literature review, a researcher-made checklist was designed to collect data. Trained psychologists gathered data on demographics (nine variables), psychosocial health assessment (nine variables), and drug (narcotic/stimulant) dependency assessment (three variables). The criteria for drug abuse and history of sleep disorders were the participants' positive responses to questions concerning "dependence on drugs" (i.e., cigarettes, alcohol, and drugs) and "history of any sleep disorders" (Table 1). We explained to the individuals the study's objectives, sample selection, and how to share the results during a phone call. Then, an appointment was fixed for face-to-face interviews with trained psychologists. Before the interviews, written informed consent was obtained from the individuals.
Data Collection: Subjects a
Aspect | Variable |
---|---|
Demographic characteristics (9) | Age#- gender # (male, female)- Marital status# (married, single, divorced, widowed)- Individual's education level# (academic, middle or high school, elementary or illiterate)- Father's education level# (academic, middle or high school, elementary or illiterate)- Mother's education level (academic, middle or high school, elementary or illiterate)- Occupation (employee or skilled worker, laborer, housewife, other forms of employment)- Household size- Financial status# (independent or dependent on others) |
Psychosocial health characteristics (9) | Cyberspace membership † (yes or no)- Job satisfaction# (completely satisfied, moderately satisfied, and dissatisfied)- Life satisfaction# (completely satisfied, moderately satisfied, and dissatisfied)- Regular exercise† (yes, no)- Guardianship status during childhood and adolescence‡ (natural, other forms of guardianship)- History of self-harm† (positive, negative)- Sleep disorder† (positive, negative)- having suicidal thoughts† (never, sometimes, often)- Family suicide attempt history* (positive, negative) |
Drug dependency assessment (3) | Smoking tobacco † (positive, negative)- Alcohol abuse† (positive, negative)- Drug abuse† (positive, negative) |
3.3. Data Analysis Method
After the collection and cleansing of the data, the mean (standard deviation) and median (IQR) were calculated to describe the population. Then, missing data analysis was done. The pattern of missingness was arbitrary and in a random fashion. There was no variable with more than 10% missing information in the data. The expectation maximization (EM) algorithm was used to estimate the missing data. EM is one of the modern and iteration-based methods for imputing missing data. In this method, the variable with missing information is entered into the model as a dependent variable and other variables as independent variables. Then, using the independent variables, it calculates the beta coefficients needed to estimate the missing data. With the assumption that the calculated coefficients and values are not the best coefficients and values, EM repeats the imputation process with new coefficients and values until convergence is reached in the size of beta coefficients and imputed values.
For statistical analysis and modeling, the data were randomly divided into training and test groups with a ratio of 70:30. Then, variables related to suicide attempts were identified using univariate logistic regression. Multivariate logistic regression was implemented on the training group data to develop different regression models. Accuracy, sensitivity, specificity, positive predictive value (PPV), negative predictive value (NPV), and area under the curve (AUC) were used to determine the performance of the models for predicting suicide attempts. The concordance index (C-index) was used to assess the model's discrimination, and the graph was used to show the calibration of the model. Considering that the ratio of case-to-control individuals in this study was 1:1 and the actual percentage of suicide attempts in the reference community was 19 per 10000 people, the modeling of suicide attempts was also done after adjusting the constant value of the model for the actual suicide attempts in the society (12), and the nomogram of the probability of committing suicide was drawn based on the adjusted model. SPSS, Stata and R software were used for data analysis.
4. Results
This study enrolled 414 individuals with suicide attempts (cases) and 408 without suicide attempts (controls) and response rates for case and control group were 94% and 91%, respectively. There were 576 people in the training group, including 281 cases and 295 controls, and 246 people in the test group, including 133 cases and 113 controls. The average age of the subjects was 32.89 ± 12.01 years. Most people were married (52.07%) and joined a virtual social network. A minority of the people (11.8%) were illiterate or poorly educated, and the majority had secondary (45.13%) and academic (43.07%) degrees.
The case and control groups' characteristics are shown in Table 2. In univariate analysis with logistic regression, age, male gender, and membership in cyberspace were protective factors. At the same time, marital status, education level, parents' education level, job, job satisfaction, financial status, sleep disorder, alcohol abuse, history of self-injury, suicidal thoughts, history of suicide attempts in the family, and life satisfaction were the risk factors for suicide attempts. The household size, regular sports activity, guardianship status of the person during childhood and adolescence, smoking, and drug abuse had no statistically significant relationship with suicide attempts in univariate analysis.
Characteristics, Univariate and Multivariate Logistic Regression Analysis a
Characteristics (Training Set) | Case Group† | Control Group† | Unadjusted OR (95% CI) | Adjusted OR (95% CI) |
---|---|---|---|---|
Age | 29.71 (10.7) | 36.18 (12.7) | 0.95 (0.94 - 0.97)* | 0.94 (0.91 - 0.97)* |
Household size | 3.8 (10.7) | 3.85 (12.7) | 0.96 (0.84 - 1.11) | 1.02 (0.79 - 1.30) |
Gender (women) | 182 (53) | 161 (47) | 0.65 (0.47 - 0.91)* | 0.26 (0.12 - 0.58)* |
Marital status | ||||
Married | 169 (55.4) | 136 (44.6) | 1 | 1 |
Unmarried | 65 (61.7) | 105 (38.2) | 2 (1.37 - 2.95)* | 0.85 (0.38 - 1.87) |
Divorced | 18 (63.3) | 31 (36.7) | 2.14 (1.16 - 4.06)* | 1.07 (0.39 - 3.05) |
Widowed | 43 (17.3) | 9 (82.7) | 0.26 (0.12 - 0.53)* | 0.11 (0.03 - 0.35)* |
Education level | ||||
Academic | 178 (72.1) | 69 (27.9) | 1 | 1 |
Secondary | 87 (33.7) | 171 (66.3) | 3.53 (2.05 - 6.14)* | 1.63 (0.58 - 4.81 ) |
Elementary | 30 (42.3) | 41 (57.7) | 5.07 (3.48 - 7.44)* | 1.69 (0.85 - 3.38) |
Father's education level | ||||
Academic | 116 (76.8) | 35 (23.2) | 1 | 1 |
Secondary | 107 (45.3) | 129 (54.7) | 3.99 (2.55 - 6.37)* | 1.74 (0.6 - 5.32) |
Elementary | 72 (38.1) | 117 (61.9) | 5.38 (3.36 - 8.78)* | 1.62 (0.64 - 4.26) |
Mother's education level | ||||
Academic | 89 (73.6) | 32 (26.4) | 1 | 1 |
Secondary | 74 (46.0) | 87 (54) | 3.27 (1.98 - 5.49)* | 3.78 (1.28 - 10.83)* |
Elementary | 132 (44.9) | 162 (55.1) | 3.41 (2.16 - 5.49)* | 3.91 (1.05 - 13.62)* |
Occupation | ||||
Employee/technical worker | 33 (24.3) | 103(75.7) | 1 | 1 |
Laborer | 36 (66.7) | 18 (33.3) | 6.24 (3.18 - 12.66)* | 1.58 (0.51 - 5.05) |
Housewife | 90 (59.2) | 62 (40.8) | 4.53 (2.75 - 7.61)* | 0.71 (0.22 - 2.25) |
Others | 122 (52.1) | 112 (47.9) | 3.4 (2.15 - 5.49)* | 0.57 (0.22 - 1.42) |
Job satisfaction | ||||
Completely satisfied | 36 (20.) | 143 (79.9) | 1 | 1 |
Moderately satisfied | 49 (54.4) | 41 (45.6) | 4.75 (2.75 - 8.32)* | 1.45 (0.58 - 3.06) |
Dissatisfied | 196 (63.8) | 111 (36.2) | 7.01 (4.59 - 10.94)* | 1.67 (0.78 - 3.54) |
Life satisfaction | ||||
Completely satisfied | 47 (19.8) | 190 (80.2) | 1 | 1 |
Moderately satisfied | 182 (70.8) | 75 (29.2) | 9.81 (6.51 - 15.02)* | 2.26 (1.11 - 4.56)* |
Dissatisfied | 52 (63.4) | 30 (36.6) | 7 (4.07 - 12.29)* | 3.34 (1.29 - 8.89)* |
Financial status (independent) | 186 (58.7) | 131 (41.3) | 2.45 (1.75 - 3.44)* | 0.97 (0.40 - 2.11) |
Cyberspace membership (positive) | 159 (42.5) | 215 (57.5) | 0.48 (0.34 - 0.68)* | 0.23 (0.11 - 0.44)* |
Exercise | 49 (46.7) | 56 (53.3) | 0.90 (0.59 - 1.37) | 1.74 (0.96 - 3.14) |
Alcohol abuse | 52 (61.2) | 33 (38.8) | 1.8 (1.13 - 2.9)* | 4.56 (1.86 - 12.88)* |
Tobacco smoking | 93 (52.8) | 83 (47.2) | 1.26 (0.89 - 1.80) | 0.86 (0.44 - 1.66) |
Drug abuse | 36 (47.4) | 40 (52.6) | 0.94 (0.57 - 1.52) | 0.43 (0.55 - 1.13) |
Having suicidal thoughts | ||||
Never | 32 (13.3) | 208 (86.7) | 1 | 1 |
Sometimes | 108 (60.3) | 71 (39.7) | 9.89 (6.2 - 16.14)* | 5.04 (2.53 - 10.27)* |
Often | 141 (89.8) | 16 (10.2) | 57.3 (31.1 - 111.8)* | 22.01 (9.66 - 53.31)* |
Guardianship type (natural) | 49 (56.3) | 38 (43.7) | 1.43 (0.90 - 2.27) | 0.90 (0.42 - 1.92) |
Self-harm (yes) | 96 (77.4) | 28 (22.6) | 4.95 (3.16 - 7.96)* | 1.23 (0.60 - 2.60) |
Sleep disorder (yes) | 105 (65.2) | 56 (34.8) | 2.55 (1.75 - 3.73)* | 3.23 (1.66 - 6.45)* |
Family suicide attempt history (yes) | 59 (62.8) | 35 (37.2) | 1.97 (1.26 - 3.13)* | 0.74 (0.34 - 1.62) |
In multivariate analysis with logistic regression, age, gender, marital status, mother's education level, life satisfaction, membership in cyberspace, sleep disorder, alcohol abuse, and experience of suicidal thoughts were significantly related to suicide attempts. Table 2 shows each variable's odds and adjusted odds ratios based on the training group data.
Finally, 45 logistic regression models were designed to determine the best predicting model of suicide attempts with forward, backward, and stepwise methods based on p values of less than 0.2 and 0.05 and Akaike's information criterion (AIC) using demographic, psychosocial health, and drug dependency variables, their combinations, and interactions. The models with the best performance are shown in Table 3.
Predictors of Suicide Attempts Based on the Multivariate Logistic Regression Model a
Predictors | Model 1 *OR (95% CI) | Model 2 #OR (95% CI) | Model 3 †OR (95% CI) | Final Model ‡OR (95% CI) |
---|---|---|---|---|
Age | 0.94 (0.92 - 0.96) | 0.94 (0.92 - 0.96) | 0.93 (0.91 - 0.96) | 0.94 (0.91 - 0.97) |
Gender (women) | 0.65 (0.42 - 0.98) | 0.49 (0.28 - 0.84) | 0.31 (0.16 - 0.56) | 5.53 (1.16 - 26.42) |
Marital status | ||||
Married | 1 | 1 | 1 | 1 |
Unmarried | 1.22 (0.72 - 2.04) | 0.88 (0.44 - 1.70) | 0.72 (0.36 - 1.41) | 0.79 (0.38 - 1.59) |
Divorced | 2.16 (1.07 - 4.53) | 0.89 (0.35 - 2.32) | 0.89 (0.35 - 2.31) | 0.92 (0.34 - 2.52) |
Widowed | 0.34 (0.14 - 0.76) | 0.15 (0.04 - 0.42) | 0.11 (0.03 - 0.32) | 0.08 (0.02 - 0.25) |
Education level | ||||
Academic | 1 | 1 | ||
Secondary | 3.61 (1.78 - 7.41) | 1.45 (0.56 - 3.74) | ||
Elementary | 3.76 (2.46 - 6.11) | 2.02 (1.04 - 3.93) | ||
Father's education level | ||||
Academic | 1 | |||
Secondary | 4.51 (2.47 - 8.89) | |||
Elementary | 4.38 (2.55 - 7.77) | |||
Mother's education level | ||||
Academic | 1 | 1 | 1 | |
Secondary | 5.7 (2.71 - 13.99) | 2.90 (2.47 - 12.85) | 12.89 (4.21 - 42.53) | |
Elementary | 6.85 (3.18 - 15.54) | 4.73 (3.30 - 16.51) | 18.12 (5.84 - 60.64) | |
Life satisfaction | ||||
Completely satisfied | 1 | 1 | 1 | |
Moderately satisfied | 3.13 (1.69 5.77) | 3.12 (1.57 - 5.37) | 3.51 (1.56 - 8.05) | |
Dissatisfied | 4.45 (1.92 - 10.53) | 5.22 (2.01 - 11.43) | 8.85 (2.80 - 30.15) | |
Cyberspace membership (positive) | 0.26 (0.15 - 0.47) | 0.19 (0.11 - 0.37) | 0.22 (0.11 - 0.42) | |
Having suicidal thoughts | ||||
Never | 1 | 1 | 1 | |
Sometimes | 5.55 (2.96 - 10.64) | 5.68 (3.00 - 11.03) | 6.24 (3.13 - 12.57) | |
Often | 23.63 (10.99 - 53.7) | 23.51 (10.84 - 54) | 24.96 (10.84 - 61.32) | |
Sleep disorder (yes) | 2.56 (1.39 - 4.80) | 3.03 (1.61 - 5.83) | 2.96 (1.53 - 5.85) | |
Alcohol abuse | 3.81 (1.71 - 8.81) | 3.82 (1.64 - 9.17) | ||
Gender*Mother education level | ||||
Academic | 1 | |||
High school | 0.09 (0.02 - 0.48) | |||
Elementary | 0.06 (0.01 - 0.27) | |||
Gender*Life satisfaction | ||||
Completely satisfied | 1 | |||
Moderately satisfied | 0.10 (0.02 - 0.53) | |||
Dissatisfied | 0.51 (0.15 - 1.66) | |||
Nagelkerke | 0.372 | 0.644 | 0.656 | 0.680 |
AUC | 0.812 (0.77 - 0.84) | 0.918 (0.90 - 0.95) | 0.927 (0.91 - 0.95) | 0.934 (0.91 - 0.95) |
The first model predicts suicide attempts based on demographic variables, including age, gender, marital status, individual's education level, and father's education (AUC = 0.817, CI: 0.78 - 0.85). The second model predicts suicide attempts based on demographic and psychosocial health variables (AUC = 0.918, CI = 0.90 - 0.95). The third model predicts suicide attempts based on demographic, psychosocial health, and drug dependency variables (AUC = 0.927, CI = 0.91 - 0.95). The final model predicts suicide attempts based on demographic, psychosocial health, and drug dependency variables and their interactions. This model was developed using the backward method and evaluated by the AIC index. As shown in Table 3, the remaining variables in the final model were age, gender, marital status, individual's education level, mother's education level, life satisfaction, membership in cyberspace, the experience of suicidal thoughts, sleep disorders, alcohol abuse, and the interaction of gender with mother's education level and life satisfaction (AUC = 0.931, CI: 0.91 - 0.95).
Models with higher sensitivity are more capable of identifying individuals with a higher probability of suicide. Therefore, the models with the highest sensitivity and at least 80% specificity were used to determine the cut-off points. With a cut-off point of 0.012, the prediction accuracy of the final model with the data of the training and test groups was 87% and 88%, respectively. The sensitivity, specificity, PPV, and NPV with the training group data were 89%, 85%, 85%, and 89%, respectively. The performance of other models in predicting suicide is shown in Table 4.
Performance of Multivariate Logistic Regression Model on Training and Testing Datasets
Indicator | Model 1 Training (Test) Set | Model 2 Training (Test) Set | Model 3 Training (Test) Set | Final Model Training (Test) Set |
---|---|---|---|---|
Accuracy | 0.75 (0.78) | 0.86 (0.87) | 0.86 (0.88) | 0.87 (0.88) |
Sensitivity | 0.87 (0.85) | 0.86 (0.81) | 0.85 (0.89) | 0.89 (0.88) |
Specificity | 0.64 (0.71) | 0.85 (0.95) | 0.87 (0.86) | 0.85 (0.88) |
PPV | 0.69 (0.77) | 0.85 (0.95) | 0.87 (0.88) | 0.85 (0.90) |
NPV | 0.83 (0.80) | 0.87 (0.81) | 0.86 (0.87) | 0.89 (0.86) |
Test set AUC (95% CI) | 0.84 (0.79 - 0.89) | 0.91 (0.89 - 0.96) | 0.92 (0.89 - 0.96) | 0.92 (0.89 - 0.96) |
The rate of suicide in the reference population was 19 per 10000 people, and in the present study, it was 50.3% (ratio of 1:1 cases and controls). As the prevalence of a disease in the community affects the PPV and NPV, the performance of the final model was also checked with a similar approach after adjusting the constant value of the model. With a cut-off point of 0.012, the prediction accuracy of the final model with the training group data was 87%. Sensitivity, specificity, PPV, and NPV for the training group data were 89%, 83%, 83%, and 87%, respectively.
A nomogram predicting the probability of suicide attempts was designed (after adjusting the constant value of the model for actual suicide attempts in the reference population) based on age, gender, marital status, individual's education level, mother's education level, life satisfaction, membership in cyberspace, the experience of suicidal thoughts, sleep disorder, and alcohol abuse (Figure 1). The nomogram can graphically display the predicted probabilities on a scale of 0-100. The score of each variable can be determined by drawing a vertical line from the variable to the score axis. By adding the scores of different variables and placing them on the axis of the total score, it is possible to estimate the probability of committing suicide for a person (13). As seen in Figure 1, the lowest (0.001) and highest (0.99) chances of suicide were predicted for the individuals with scores of 73 and 347, respectively.
Nomogram for predicting suicide attempts. The following are the codes assigned to categorical variables: Education level (academic = 0, high school = 1, elementary = 2), sleep disorder (no = 0, yes = 1), alcohol abuse (no = 0, yes = 1), life satisfaction (completely satisfied= 0, moderately satisfied = 1, dissatisfied = 2), sex (female = 0, male = 1), marital status (married = 0, unmarried = 1, divorced = 2, widowed = 3), mother's education level (academic = 0, high school = 1, elementary school = 2), cyberspace membership (no = 0, yes = 1), having suicidal thoughts (never = 0, sometimes = 1, usually = 2)
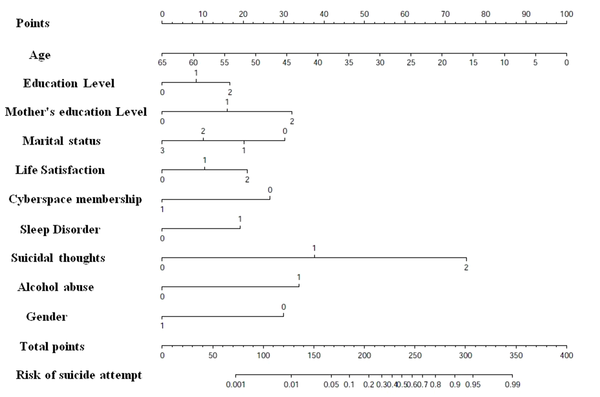
The validation of the nomogram model is usually done by discrimination and calibration. Discrimination is the ability of the model to classify patients with different outcomes. The difference between the predicted and actual values determines calibration (14). The high value of the coordination index (C-index = 0.911, se = 0.01) showed that the nomogram had excellent discrimination. Figure 2 shows the calibration plot of the nomogram model, which was made by the Bootstrap validation method. The predicted probabilities from the nomogram and the actual probabilities from the training group data had good convergence, indicating the model's optimal calibration.
Nomogram calibration curves for suicide attempt
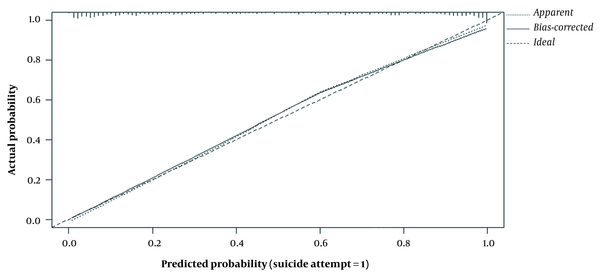
5. Discussion
In this study, we designed four predictive models using demographic, psychosocial health, and drug dependency variables. All four models could distinguish people with suicide attempts from the control group (AUC of model 1 = 0.817, AUC of model 2 = 0.918, AUC of model 3 = 0.927, and AUC of the final model = 0.931). The best model (final model) was created from multivariate logistic regression with 12 significant predictors, including age, gender, marital status, education level, mother's education level, life satisfaction, membership in cyberspace, sleep disorders, alcohol abuse, experience of suicidal thoughts, and the interactions of gender with mother's education level and life satisfaction.
Performance indicators of the final model, including accuracy, sensitivity, specificity, PPV, NPV, and AUC, were 0.87 (0.88), 0.89 (0.88), 0.85 (0.88), 0.85 (0.90), 0.89 (0.86), and 93 (92.8) percent with the training group data (test group), respectively, showing the capability of the regression model for distinguishing individuals with a suicide attempt from the control group and the ability of the model in predicting suicide attempts.
In line with the present study, Lyu and Zhang using multivariate logistic regression, showed that mental problems, low education level, low health level, aspiration strain, hopelessness, impulsivity, and depression were risk factors for suicide attempts. At the same time, social support, coping skills, and a healthy community were protective factors against suicide attempts in adolescents. The predictive model of this study was built with an artificial neural network whose performance indicators showed a sensitivity of 67.6%, specificity of 93.9%, PPV of 86.0%, NPV of 84.1%, and total coincidence rate of 84.6%, showing that the model had good efficiency (15). Lee et al. modeled the role of demographic variables, high-risk behaviors, and psychological variables in the suicide attempt of Korean adolescents using multivariate logistic regression. In the final model, age, eating breakfast, and daily sleep were protective factors, while female gender, violence experience, stress, feeling sadness, smoking and alcohol abuse, low level of perceived health, low level of education, the poor economic condition of the family, and living with non-biological parents were the risk factors for committing suicide (AUC: 0.85). Also, sadness, the experience of violence, and perceived stress were the most important variables related to suicide attempts in this model (AUC: 0.82) (10). The parsimonious principle was considered in the modeling in the present study, and the models with the minimum number of predictors had excellent performance, so they can be used as screening tools in the general population.
Nomograms can display the diagnostic power of various factors visually. Based on the designed nomogram (Figure 1), age and experience of suicidal thoughts were the strongest, and education level was the weakest diagnostic factor of the study to predict suicide attempts. The coordination index (C-Index = 0.9108) and the calibration plot (Figure 2) showed that the model had good calibration and discrimination. Considering that the predictor variables of the model are available and can be easily measured, this nomogram can be used by non-specialists and at the basic levels of healthcare services and even other fields. For example, based on the nomogram of the present study, the probability of a suicide attempt is > 99% for a 20-year-old married woman who is dissatisfied with her life, does not participate in cyberspace, usually has suicidal thoughts, suffers from sleep disorders and alcohol abuse, and herself and her mother have primary education. On the other hand, the probability of suicide is less than 1% for a 20-year-old single woman, satisfied with life, without suicidal thoughts, with cyberspace membership, academic education for herself and her mother, and not suffering from sleep disorders and alcohol abuse.
The risk predicted by the predictive models and nomogram of the present study can help early identify individuals at high risk for suicide attempts. It can lead to more effective preventive interventions and better management of suicide, reducing the economic, social, and health burden caused by suicide. This study was conducted as a case-control one. Therefore, it has the limitations of retrospective observational studies, such as being prone to some biases, including selection biases. Some variables were collected via a self-report method, so there is the potential for recall bias or underreporting, although we removed potential bias sources when designing and implementing the study.
Conclusions
Our study shown that compared to the control group, the suicide attempters were younger in terms of age. People whose mothers were less literate, those who used to abuse alcohol, people with suicidal thoughts, and individuals with sleep disorders had a higher chance of committing suicide. Also males, those who were members of cyberspace, people who were satisfied with their lives, and widowed ones (in comparison with single/married and divorced people) had a lower chance of committing suicide. The categories in which cases and controls did not differ were family size, father's education level, job and job satisfaction, financial status, exercise, drug abuse and smoking, type of guardianship, history of self-harm and history of suicide in the family. Finally This study suggests that the designed models performed well in predicting suicide attempts. Therefore, it can be used for early identification of people at risk of suicide and reducing the burden caused by suicide.
Acknowledgements
References
-
1.
Jarema M. Are we able to evaluate suicide risk? Psychiatr Pol. 2018;52(1):7-19. [PubMed ID: 29704410]. https://doi.org/10.12740/PP/74161.
-
2.
Mulder R, Newton-Howes G, Coid JW. The futility of risk prediction in psychiatry. Br J Psychiatry. 2016;209(4):271-2. [PubMed ID: 27698212]. https://doi.org/10.1192/bjp.bp.116.184960.
-
3.
Malhi GS, Outhred T, Das P, Morris G, Hamilton A, Mannie Z. Modeling suicide in bipolar disorders. Bipolar Disord. 2018;20(4):334-48. [PubMed ID: 29457330]. https://doi.org/10.1111/bdi.12622.
-
4.
Bantjes J, Tomlinson M, Weiss RE, Yen PK, Goldstone D, Stewart J, et al. Non-fatal suicidal behaviour, depression and poverty among young men living in low-resource communities in South Africa. BMC Public Health. 2018;18(1):1195. [PubMed ID: 30348143]. [PubMed Central ID: PMC6198370]. https://doi.org/10.1186/s12889-018-6104-3.
-
5.
Suicide prevention. World Health Organization; 2019. Available from: https://www.who.int/health-topics/suicide#tab=tab_2.
-
6.
Mohammad-Khani P, Mohammadi M, Rezaee D, Dogaheh E, Nazari M. [Epidemiology of suicide thoughts and suicide attempts in young girl at high-risk province of Iran]. Social Welfare. 2004;4(14):162-4. Persian.
-
7.
Suicide prevention (overview). World Health Organization; 2019. Available from: https://www.who.int/health-topics/suicide#tab=tab_1.
-
8.
Hatami H, Eftekhar Ardebili H, Majlesi F, Seyyed Nozadi M, Parizadeh MJ. Mental Health. Text book of public health. 4th ed. Tehran, Iran: Arjomand Publication; 2019. 3203 p. Persian.
-
9.
Lyu J, Wang Y, Shi H, Zhang J. Early warnings for suicide attempt among Chinese rural population. J Affect Disord. 2018;238:353-8. [PubMed ID: 29908474]. https://doi.org/10.1016/j.jad.2018.06.009.
-
10.
Lee J, Jang H, Kim J, Min S. Development of a suicide index model in general adolescents using the South Korea 2012-2016 national representative survey data. Sci Rep. 2019;9(1):1846. [PubMed ID: 30755681]. [PubMed Central ID: PMC6372692]. https://doi.org/10.1038/s41598-019-38886-z.
-
11.
Maimon O, Rokach L. Data mining and knowledge discovery handbook. 2nd ed. New York, USA: Springer; 2010.
-
12.
Huang Y, Pepe MS. Assessing risk prediction models in case-control studies using semiparametric and nonparametric methods. Stat Med. 2010;29(13):1391-410. [PubMed ID: 20527013]. [PubMed Central ID: PMC3045657]. https://doi.org/10.1002/sim.3876.
-
13.
Iasonos A, Schrag D, Raj GV, Panageas KS. How to build and interpret a nomogram for cancer prognosis. J Clin Oncol. 2008;26(8):1364-70. [PubMed ID: 18323559]. https://doi.org/10.1200/JCO.2007.12.9791.
-
14.
Shipe ME, Deppen SA, Farjah F, Grogan EL. Developing prediction models for clinical use using logistic regression: an overview. J Thorac Dis. 2019;11(Suppl 4):S574-84. [PubMed ID: 31032076]. [PubMed Central ID: PMC6465431]. https://doi.org/10.21037/jtd.2019.01.25.
-
15.
Lyu J, Zhang J. BP neural network prediction model for suicide attempt among Chinese rural residents. J Affect Disord. 2019;246:465-73. [PubMed ID: 30599370]. [PubMed Central ID: PMC6430644]. https://doi.org/10.1016/j.jad.2018.12.111.