Abstract
Background:
Methadone maintenance treatment (MMT) has been a cornerstone in heroin addiction management. However, its efficacy varies among individuals. The complex interplay of genetic backgrounds and demographic data could influence the response to MMT in heroin addiction. No previous adoption study has aimed to merge these findings into a potential pre-treatment screening tool.Objectives:
This study aimed to investigate the combined influence of dopamine and opioid receptors and receptor endocytosis machinery genes, individual genetic backgrounds, and demographic data on the response to MMT in patients with heroin addiction.Methods:
We enrolled 80 heroin addicts receiving MMT for 3 months alongside 80 healthy individuals in a comparative study. The approach utilized multinomial, linear, and binary logistic regression analyses to investigate the interplay of genetic factors (DRD1-5, opioid receptors [µ1, δ1, and κ1], DNM1L, RAB22A, and COMT), demographic independent variables, including, family history, heroin duration, age onset, heroin dose, and methadone dose, and clinical markers Subjective Opiate Withdrawal Scale (SOWS) with compliance with MMT protocols.Results:
Results revealed that a positive family history and a higher level of heroin dose significantly predicted poor compliance to MMT. Additionally, the patients with lower expression levels of DRD2 and higher expression levels of DNM1L and COMT genes were at higher risk for poor compliance with the treatment.Conclusions:
By utilizing a comprehensive dataset of gene expression profiles and demographic and clinical parameters, this study developed a regression model predicting resistance or response to methadone. This innovative approach seeks to bridge the gap between pharmacogenomics and clinical practice and offer a potential pre-treatment screening tool for personalized MMT strategies in opioid addiction management. The obtained findings hold intriguing promise for future research, potentially unlocking deeper insights into the underlying risk factors of addiction.Keywords
Compliance Prediction Methadone Maintenance Treatment (MMT) Personalized Medicine Regression Analysis
1. Background
Heroin addiction presents a significant public health challenge. Methadone maintenance treatment (MMT) is the primary treatment option. However, MMT's effectiveness varies due to genetic and environmental factors. Some patients experience treatment failure, relapse, or developing tolerance (1). The complexity of heroin addiction's neurobiology involves various neurotransmitter systems, notably the dopaminergic and opioidergic systems (2).
Dopamine receptors (DRs) (DRD1, DRD2, DRD3, DRD4, and DRD5) and the COMT enzyme, which degrades dopamine, play crucial roles in addiction. Opioid receptors (ORs) (OPRM1, OPRD1, and OPRK1) are directly impacted by heroin, affecting mood regulation and stress responses (3).
Brain-derived neurotrophic factor (BDNF), vital for neuronal growth and synaptic plasticity, experiences changes in heroin users, influencing reward pathways and cravings (4). Additionally, RAB22A and DNM1L are involved in cellular processes, such as trafficking and mitochondrial fission, respectively, which can indirectly relate to the neural adaptations observed in addiction (5).
Methadone, by action on mu-opioid receptors, modulates dopamine release more consistently than heroin, thereby impacting DRs and contributing to long-term adaptive changes in the brain. These changes can influence MMT outcomes, including mood disturbances and the severity of withdrawal symptoms, as quantified by tools, such as the Subjective Opiate Withdrawal Scale (SOWS) (6).
The primary issue that remains unresolved in the treatment of heroin addiction through MMT is its varied effectiveness, largely influenced by genetic and environmental factors (1). Given the complexities of heroin addiction, combined with the existing knowledge gap, there is a clear need for an initial screening panel before initiating MMT.
2. Objectives
The present study delved into how a combination of specific genes and demographic variables could potentially influence and predict an individual's level of compliance with MMT for heroin addiction. The ultimate goal is to develop a model that can forecast a patient's likelihood of successfully adhering to MMT. To explore these questions, an adoption design study was proposed by identifying potential biomarkers using non-invasive techniques, specifically through peripheral blood sampling. Initially, the focus was on identifying any changes in the gene expression profiles related to addiction pathogenesis pathways during MMT. Subsequently, the study examined a correlation between genetic profiles and environmental risk factors, in addition to their interactions in response to methadone. It is hypothesized that by identifying key genetic and personal factors, a pre-treatment screening tool can be created. This tool would enable a more tailored approach to MMT, aligning treatment strategies more closely with each patient's unique genetic profile and personal setting, thereby enhancing the overall effectiveness of heroin addiction management.
3. Methods
3.1. Sample Study
This cross-sectional study was conducted on 80 heroin users and 80 age- and gender-matched healthy controls between 2019 and 2020 to assess the study objectives. The group of genes that display the most powerful evidence for positive correlation with addiction pathogenesis was selected based on preceding findings by others and previous work of our group (5, 7, 8).
The first group of patients comprised compliance to treatment with a methadone daily dose of 40 mg/d or greater to 80 mg/d or less (good compliance [GC] to MMT, n = 58). The second group of patients comprised poor compliance (PC) to treatment with a methadone daily dose of 120 mg/d or greater and who were receiving no co-medications known to be inducers of methadone metabolism (PC to MMT, n = 22). Most of the sociodemographic and clinical data were collected from the clinical records (Appendix 1 in the Supplementary File provides sample classification methodology and inclusion criteria, Appendix 2 in the Supplementary File, demographic table).
3.2. Gene Expression
Primers were designed using Primer Express software (http://www.ncbi.nlm.ih.gov/tools/primer-blast/) (Appendix 3 in the Supplementary File). In this study, 5 mL of blood was used for peripheral blood mononuclear cells (PBMC) separation using Ficoll-Hypaque (Pharmacia, Sweden). The lymphocytes' messenger ribonucleic acid (mRNA) was extracted using a Roche RNA mini kit (Germany), and complementary deoxyribonucleic acid (cDNA) was synthesized by Fermentas cDNA synthesis kit (Germany). Real-time polymerase chain reaction (PCR) was performed using a Techne Flexigene PCR Cycler (USA) and LightCycler® FastStart DNA MasterPLUS SYBR Green I kit (Roche, Germany).
3.3. Statistical Analysis
Real-time PCR data were analyzed using LinRegPCR (version 2021.2) and normalized against the β-actin gene. Unpaired t-test (P < 0.05) and graphical representations were performed in GraphPad Prism 10.
Data analysis was conducted in SPSS-27 using the Kolmogorov-Smirnov test, Wilcoxon’s test, Levene's test, and effect size (Cohen's d, Hedges' g, Glass’s Δ). Linear, hierarchical, and logistic regressions were employed for predictive modeling. Multivariate logistic regression with backward elimination identified variables associated with MMT compliance, used for propensity matching. The predictive power of biomarkers was assessed via receiver operating characteristic (ROC) curves and area under the curve (AUC) evaluation (P < 0.05), and the Hosmer-Lemeshow test verified model accuracy.
4. Results
4.1. Gene Expression Associated with Heroin Addiction
The Mann-Whitney U test between heroin-addicted and controls revealed a marked decrease in OPRM1 in the addicted group (P < 0.0016, Fc: -9.37). Nevertheless, DNM1L and RAB22A showed increased expression, with DNM1L (P < 0.0001, Fc 3.72) and RAB22A (P < 0.0001, Fc 3.22).
The unpaired t-test in DRs (DRD1-DRD5) revealed that DRD1 increased 9.54-fold; however, DRD2 decreased by -27.24-fold in the addicted group (P < 0.0001). The COMT gene expression increased 6.12-fold, and ORs (OPRD1 and OPRK1) decreased (Fc -7.09 and -6.06, respectively, P < 0.0001). Additionally, BDNF gene expression significantly decreased in the addicted group (Fc -10.7, P < 0.0054) (Appendix 4 in the Supplementary File) (Figure 1).
The bar chart presents the relative gene expression levels of heroin addicts (n = 80) and control (n = 80). Error bars represent the standard error of SEM. *P < 0.05, **P < 0.01, ***P < 0.001. Data are normalized to β-actin.
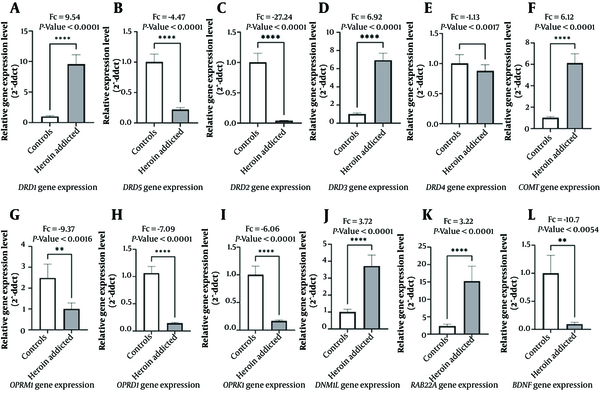
4.2. Gene Expression in MMT
In the GC-MMT, DRD3, COMT, and RAB22A gene expression decreased (Fc -5.85, -5.8, and -1.61 respectively). In contrast, the OPRM1, OPRD1, OPRK1, DRD2, BDNF, and DRD5 genes increased, (Fc 5.27, 2.73, 2.73, 5.46, 4.71, and 2.73 respectively). The aforementioned findings underscore the varied impact of methadone treatment compliance on different gene expressions in addiction (Appendix 5 in the Supplementary File, Figure 2).
Differential gene expression levels in heroin addicts under MMT (3 months). Good compliance to MMT (n = 58) and poor compliance to MMT (n = 22). Error bars denote the SEM. * P < 0.05, ** P < 0.01, *** P < 0.001, **** P < 0.0001.
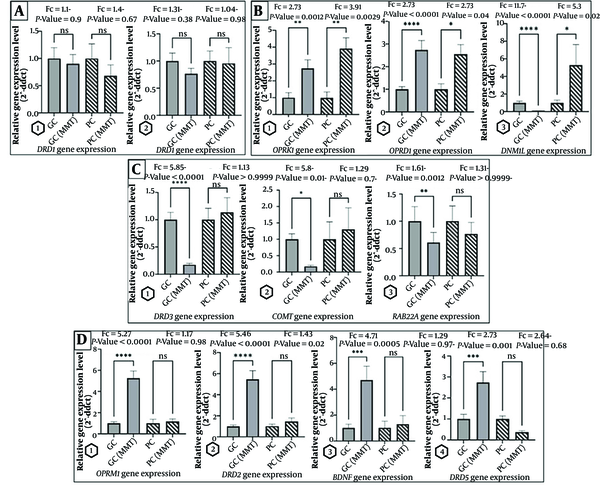
4.3. Correlation of Gene Expression with Methadone Dose
OPRM1 exhibited a significant positive correlation (r = 0.540, P < 0.0001), and RAB22A showed a significant negative correlation (r = -0.614, P < 0.0001) with methadone dose, particularly at higher doses.
Moreover, Spearman correlation analysis showed strong negative correlations for COMT (r = -0.596, P < 0.0001) and DNM1L (r = -0.761, P < 0.0001) with methadone dose, especially significant in higher dose ranges. DRD2 and DRD5 demonstrated significant positive correlations (DRD2: r = 0.531, P < 0.0001; DRD5: r = 0.619, P < 0.0001), mainly in higher doses. DRD3 revealed a strong negative correlation in higher doses (r = -0.666, P < 0.0001) (Appendix 6 in the Supplementary File) (Figure 3A-G).
(A-G) Scatter plots illustrate the significant correlations between the normalized gene expression levels (ΔCT) and the range of methadone doses administered during MMT. (H) The scatter plot depicts the predicted probabilities of patient compliance to methadone treatment against the actual observed outcomes. Predicted probabilities were derived from a logistic regression model, with individual observations represented by symbols. (I) The ROC curve graph evaluates the diagnostic ability of five genetic markers to predict methadone treatment compliance. (J) This logistic plot depicts the relationship between the probability of the predicted outcome and the values of independent genetic variables. (K) The matrix displays the pairwise Pearson correlation coefficients (R2 values) among eight genes studied in the context of methadone treatment response. (L) This bar graph depicts the relative gene expression levels associated with two categories of the subjective SOWS scores, comparing low (0 - 10) to high (20 - 30) withdrawal symptom scores. Statistical significance determined by the least significant difference (LSD) test is denoted above the bars: ** P < 0.01, * P < 0.05, ns indicates not significant.
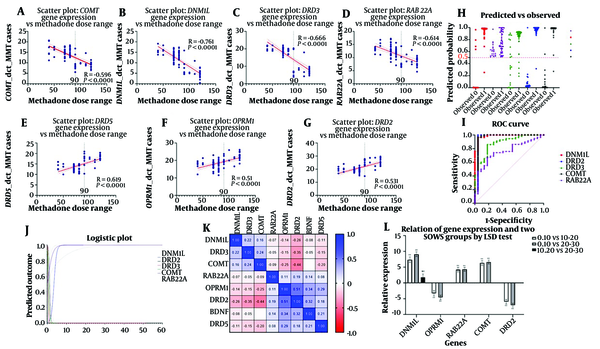
4.4. Predictive Validity of Genetic Markers in Compliance to Methadone
For each of the DNM1L, DRD3, COMT, RAB22A, OPRM1, DRD2, BDNF, and DRD5 gene expressions, a separate simple binary logistic regression was conducted where the predictor was the gene expression, and the outcome was compliance to methadone. In a binary logistic regression analysis, the increased DNM1L expression was significantly associated with PC (93.75% model accuracy); however, higher DRD2 expression correlated with GC (97.50% model accuracy). The COMT gene expression showed a substantial influence on compliance, with 96.25% predictive accuracy and a significant odds ratio (Appendix 7 in the Supplementary File). The scatter plot (Figure 3H), ROC curve (Figure 3I), logistic plot (Figure 3J), and correlation matrix (Figure 3K) highlighted the significant results.
Moderate correlations were observed between OPRM1 and DRD2, suggesting potential multicollinearity issues in regression models. To mitigate this, genes were carefully selected based on their biological relevance and clinical significance.
Differential gene expression profile between GC and PC implies a genetic predisposition influencing MMT efficacy, especially in higher dose ranges. This study also extended to demographic factors using analysis of variance (ANOVA) tests, emphasizing the importance of considering both genetic and demographic influences on MMT's effectiveness.
4.5. Gene Variations and Demographic Influences on MMT
The study evaluated compliance levels with MMT based on clinical guidelines for withdrawal management and considered various independent variables, such as age, addiction history, heroin use duration, and SOWS scores.
The analysis of variance analysis revealed significant gene expression differences related to SOWS scores, particularly in DNM1L and OPRM1, indicating varying gene expression with withdrawal severity (Appendix 8 in the Supplementary File, Figure 3L). Effect sizes highlighted strong associations for DNM1L and DRD3 (Appendix 9 in the Supplementary File).
Further ANOVA tests examined gene expression against heroin use duration, showing significant impacts on genes, such as DRD2. This analysis revealed varying effect sizes, suggesting differential gene expression impacts based on heroin dose (Appendix 10 in the Supplementary File).
This study also investigated the relationship between heroin use onset and gene expression (Appendix 11 in the Supplementary File). Significant differences were observed for DNM1L, OPRM1, and DRD2, indicating a strong link between the age of onset and gene expression.
Lastly, this study employed hierarchical logistic regression, focusing on genetic and demographic predictors for non-compliance with MMT, setting the stage for more detailed multiple logistic regression analyses. The binary logistic regression model's validity was confirmed by Omnibus Tests of Model Coefficients (P < 0.0001), and Hosmer-Lemeshow test (chi-square = 0.000, P = 1.000).
Multiple logistic regression demonstrated excellent performance, with an area under the ROC curve of 0.9945 (Table 1). DNM1L, COMT, DRD2, negative family history, and lower heroin doses showed positive coefficients, indicating their influence on increasing compliance likelihood.
Logistic Regression Analysis for Predicting Compliance to Methadone Maintenance Treatment (MMT)
Parameter | Estimate | Standard Error | 95% CI | Odds Ratio | 95% CI (Odds Ratio) | Z-Value | P-Value | VIF | R² with Other Variables |
---|---|---|---|---|---|---|---|---|---|
Intercept (β0) | -44.16 | 17.1 | -90.91 to -17.68 | 6.61E-20 | 3.29E-040 to 2.09E-08 | 2.582 | 0.0098 | - | - |
DNM1L a (β1) | 1.134 | 0.4383 | 0.4430 to 2.292 | 3.108 | 1.557 to 9.899 | 2.587 | 0.0097 | 9.138 | 0.8906 |
COMT a (β2) | 1.238 | 0.5059 | 0.4982 to 2.693 | 3.449 | 1.646 to 14.78 | 2.448 | 0.0144 | 6.862 | 0.8543 |
DRD2 a (β3) | 0.4542 | 0.2308 | 0.04824 to 1.051 | 1.575 | 1.049 to 2.861 | 1.968 | 0.049 | 10.53 | 0.905 |
Family history b [0] (β4) | 4.076 | 1.998 | 0.6705 to 9.184 | 58.9 | 1.955 to 9742 | 2.04 | 0.0413 | 1.864 | 0.4635 |
Heroin dose c [1] (β5) | 2.471 | 1.257 | 0.4136 to 6.229 | 11.83 | 1.512 to 507.4 | 1.966 | 0.0493 | 6.523 | 0.8467 |
Model diagnostics affirmed the robustness of the analysis, with high accuracy in classification (97.5%). The predictive formula derived from the model is as follows:
ρ = (e-44.16 + 1.134 × DNM1L + 1.238 × COMT + 0.4542 × DRD2 + 4.076 × Patients Family History [0] + 2.47 × Heroin Dose [1]) / (1 + e-44.16 + 1.134 × DNM1L + 1.238 × COMT + 0.4542 × DRD2 + 4.076 × Patients Family History [0] + 2.47 × Heroin Dose [1])
This equation estimates the probability of compliance to MMT, integrating both genetic predispositions and drug use behavior, refined for the prediction in a clinical setting.
In summary, the present study's workflow visually summarizes the research (Figure 4), presenting a clear and concise representation of the complex interplay between gene expression and compliance with MMT.
Workflow summarizing gene expression's role in MMT compliance, from analysis to predictive modeling. Created with BioRender.com.
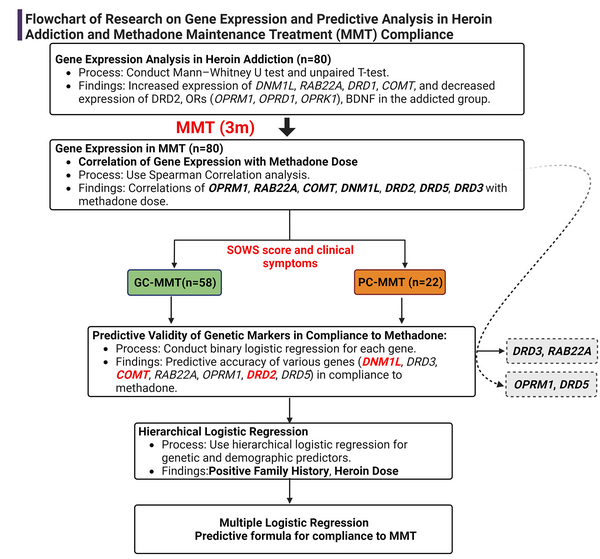
5. Discussion
Heroin addiction is a complex condition influenced by the combination of genetic elements and environmental factors (9). This study highlighted distinct variations in gene expression when analyzing the gene profile associated with heroin. Opioid receptor genes, along with DRD2, DRD5, and BDNF, showed decreased expression in heroin addiction; however, DRD1, DRD3, COMT, DNM1L, and RAB22A were upregulated. The decreased OR expression suggests reduced sensitivity, impacting pain management and addiction treatment (10). The COMT gene showed varied expression among heroin addicts. The DRD1 and DRD3 gene variations were linked to early-onset heroin dependence, indicating susceptibility to addiction (11). Previous research indicated conflicting findings regarding DRD5 and DRD4 (12). The majority of the studies focused on the brain’s expression. Therefore, quantifying DRD4 and DRD5 in peripheral blood is challenging due to low abundance, highlighting the complexities of studying these genes outside the central nervous system (CNS). The genetic susceptibility to heroin addiction was strongly associated with DRD2, evident in high expression levels in blood and particular polymorphisms (13). Studies also highlighted the influence of cocaine and morphine on DNM1L and RAB22A (5, 14); nevertheless, research on their expression in heroin addicts is missing. Additionally, alterations in BDNF expression in blood were proposed as a possible biomarker for heroin addiction treatment.
Regarding MMT, GC was associated with increased expression of ORs, BDNF, DRD2, and DRD5. Nonetheless, PC showed no significant change, except for OPRD1 and OPRK1. Research has yielded inconsistent interpretations regarding the relationship between OPRM1 expression and methadone tolerance (15), suggesting the influence of OPRM1 expression on methadone tolerance is multifaceted and might not be a direct factor.
Addiction has been shown to impact the epigenetic mechanism, such as promoter hypermethylation, leading to altered expression of ORs (16). Methadone maintenance treatment showed positive outcomes by increasing the expression of OPRM1 (17). Nevertheless, there was resistance to normalizing OPRM1 in PC-MMT.
To seek an explanation, this study examined the relationship between methadone dosage and patient categorization. Lower methadone doses consistently administered in GC led to increased OPRM1 expression, and different dosage groups (40 - 59.9 mg, 60 - 89.9 mg, and 90 mg or higher) showed varied gene expression and response patterns, indicating potential threshold effect at 90 mg where therapeutic responses change significantly, highlighting the complex nature of dose-dependent efficacy and tolerance.
The findings of the present study suggest a significant link between the age of initial heroin use, the presence of a positive family history, and OPRM1 gene expression, with early adolescence being a critical period for heroin initiation that might affect tolerance development in MMT. This finding is consistent with the higher heroin dosages observed in PC that necessitate increased methadone dosages in this subgroup. Moreover, this finding aligns with previous reports indicating that starting heroin use at an older age correlates with more effective outcomes from MMT (18), highlighting the importance of genetic predispositions in MMT outcomes.
Previous studies indicated that stressors can change BDNF expression in addiction-related brain areas (19), potentially inducing depressive symptoms in prolonged heroin use (20). Elevated BDNF levels have been observed to be linked to addictive behaviors (21). This study showed no notable change in BDNF expression in PC-MMT, contradicting prior beliefs about its link to MMT. This finding aligns with recent studies (22); however, the inconsistent results suggest that BDNF might not be a reliable biomarker for MMT efficacy.
The DRD2 gene expression remains unchanged in PC-MMT. Furthermore, lower DRD2 expression correlates with nonresponsiveness to MMT and higher prior heroin use. Methadone has been shown to increase DRD2 expression in the blood, potentially impacting the brain's reward system (23). The present study showed that higher methadone doses are associated with lower DRD2 gene expression in the PC group. These insights collectively suggest that DRD2 expression might be a pivotal factor in determining treatment resistance in opioid addiction, necessitating consideration of both genetic and environmental factors in tailoring MMT (24). Several pharmacogenetic studies have further emphasized the relationship between genetic factors and MMT response (23), highlighting DRD2's role in individual variances in pharmacotherapy response and methadone dosage requirements (25).
In addition, a significant decrease was noted in the expression of DNM1L, DRD3, COMT, and RAB22A genes in GC-MMT. On the other hand, those with PC-MMT did not exhibit substantial changes in gene expression, except for DNM1L, which intriguingly showed an increase. Additionally, changes in the DRD1 and DRD4 genes were not significant in both the GC and PC groups.
Currently, this is the first study regarding the direct connection between the RAB22A and DNM1L genes expression in peripheral blood and their implications for heroin addiction and the efficacy of methadone treatment. Notably, most research concerning RAB22A gene expression has been focused on its association with various types of cancers (26). The RAB22A gene, which is a part of the RAS oncogene family and plays a significant role in intracellular trafficking, might play a role in receptor endocytosis, contributing to resistance in addiction.
The logistic regression analysis revealed the complex interplay between genetic, environmental, and behavioral influences on MMT compliance. A significant finding is a positive coefficient for DNM1L, with an odds ratio of 3.108, underscoring its substantial role in MMT compliance. This gene, known for its involvement in mitochondrial dynamics and cellular energy regulation, has previously been linked to the therapeutic response in depression, where its upregulation was observed (27). Neurological evidence further supports a bidirectional relationship between heroin addiction and depression (28). Multiple studies suggest a connection between inflammation, mitochondrial dysfunction, increased reactive oxygen species (ROS) production, and the pathophysiology of depression (29). Additionally, the presence of depression in heroin addicts has been shown to influence methadone dosing, often requiring higher doses for stabilization in those with comorbid mood disorders (28). This is further supported by the observation that heroin-dependent individuals, once tolerant, are driven by a need to maintain homeostasis, which is linked to reduced dopamine release in key brain areas and an inability to inhibit drug-seeking behaviors (30).
As previously mentioned, another predictor was DRD2 based on the previous evidence (31) and observations of DRD2 downregulation's association with reduced MMT efficacy.
The COMT's role in breaking down dopamine has been linked to psychiatric disorders often co-existing with addiction. Higher COMT enzyme activity and reduced frontal cortical dopamine are more prevalent in heroin-dependent individuals (31). The present study further observed a connection between lower COMT expression and better MMT compliance.
The logistic model demonstrated significant associations of COMT and DRD2 with MMT response, with odds ratios of 3.449 and 1.575, respectively. In the concept of environmental and behavioral factors, the analysis reveals a pronounced effect of positive family history and higher heroin dose on response to MMT. Particularly, the high odds ratio for negative family history (58.9) highlights its substantial protective impact, potentially overshadowing other factors. Similarly, the heroin dose presents a considerable odds ratio of 11.83 for the lowest level as a protective factor and emphasizes its critical role in compliance with treatment.
The findings of the current study suggest that genetic factors significantly influence compliance with MMT, while environmental and behavioral factors, such as family history and heroin dosage, also play key roles. Individuals with a family history of addiction often show more severe opioid dependence symptoms (9). Family-related risk factors, including prenatal exposure to maternal smoking and inadequate care, lower parental education, neglect, and exposure to substance use within the family, contribute to the risk of drug abuse (32). It is believed that in the family risk factor, both the family environment and genetic predispositions might increase susceptibility to drug abuse.
Research indicates that higher methadone doses can reduce heroin use and alleviate withdrawal symptoms, suggesting their effectiveness in creating cross-tolerance to heroin (33). However, this relationship is complex (34), as higher doses might not always suppress withdrawal symptoms, particularly in patients with poor compliance to treatment. The effectiveness of methadone dosage varies based on individual responses and the importance of consistent treatment strategies.
This predictive model can guide clinicians in tailoring MMT strategies. Specifically, patients with no family history of addiction, lower heroin abuse, a lower expression level of COMT and DNM1L genes, and an increased expression level of the DRD2 gene typically show better results and adherence to MMT and might benefit from a modified approach. Nevertheless, deviations from this pattern tend to result in poorer compliance with MMT, which could lead to more side effects and less effective treatment.
Based on the current logistic regression model, this study has developed a predictive framework that can estimate an individual's likelihood of adhering to MMT, considering their genetic makeup, family history, and initial heroin dose. This area of study is still evolving, and further research is needed to fully understand these mechanisms. These insights could be instrumental in developing targeted strategies for management, prevention, or treatment in pre-selecting the most appropriate curation strategy.
4.1. Limitations and Future Directions
The current study on MMT compliance offers insights but has some limitations. The three-month follow-up might not capture long-term outcomes, and logistic regression analysis might oversimplify complex MMT compliance relationships. While significant P-values show strong associations, causality is not established, and findings might not be widely generalizable due to the specific population and moderate sample size. Additionally, gene expression measurements were performed in blood samples, not directly reflecting brain processes (35). Furthermore, more diverse research is needed for a deeper understanding and personalized treatment strategies.
4.2. Conclusions
This study showed that a positive family history, higher heroin usage, increased expression levels of COMT and DNM1L, and decreased expression of DRD2 are associated with poor compliance with MMT outcomes. These findings represent a significant step in the application of personalized medicine strategies in addiction treatment, offering a novel approach to optimizing MMT by considering individual genetic and environmental profiles.
Acknowledgements
References
-
1.
Fonseca F, Torrens M. Pharmacogenetics of Methadone Response. Mol Diagn Ther. 2018;22(1):57-78. [PubMed ID: 29168075]. https://doi.org/10.1007/s40291-017-0311-y.
-
2.
Nielsen LM, Christrup LL, Sato H, Drewes AM, Olesen AE. Genetic Influences of OPRM1, OPRD1 and COMT on Morphine Analgesia in a Multi-Modal, Multi-Tissue Human Experimental Pain Model. Basic Clin Pharmacol Toxicol. 2017;121(1):6-12. [PubMed ID: 28084056]. https://doi.org/10.1111/bcpt.12757.
-
3.
Wang SC, Chen YC, Lee CH, Cheng CM. Opioid Addiction, Genetic Susceptibility, and Medical Treatments: A Review. Int J Mol Sci. 2019;20(17). [PubMed ID: 31480739]. [PubMed Central ID: PMC6747085]. https://doi.org/10.3390/ijms20174294.
-
4.
Koo JW, Mazei-Robison MS, LaPlant Q, Egervari G, Braunscheidel KM, Adank DN, et al. Epigenetic basis of opiate suppression of Bdnf gene expression in the ventral tegmental area. Nat Neurosci. 2015;18(3):415-22. [PubMed ID: 25643298]. [PubMed Central ID: PMC4340719]. https://doi.org/10.1038/nn.3932.
-
5.
Ilkhanizadeh S, Nakhaei Sistani R, Sadeghizadeh M, Ahangari G. [Analysis of long-term treatment of Mu opioid receptors agonists effect on Dnm1L and Rab22a expression in HEK293 Flp-In MOP YFP]. Pathobiol Res. 2018;20(4):21-35. Perrsian.
-
6.
Mokri A, Noroozi A. [Protocol on management of opioid dependence with methadone]. 3rd ed. Prevention and Treatment of Substance Abuse's Office; Mental, Social, and Addiction Health Office; 2014. Persian.
-
7.
Solinas M, Belujon P, Fernagut PO, Jaber M, Thiriet N. Dopamine and addiction: what have we learned from 40 years of research. J Neural Transm (Vienna). 2019;126(4):481-516. [PubMed ID: 30569209]. https://doi.org/10.1007/s00702-018-1957-2.
-
8.
Valentino RJ, Volkow ND. Untangling the complexity of opioid receptor function. Neuropsychopharmacology. 2018;43(13):2514-20. [PubMed ID: 30250308]. [PubMed Central ID: PMC6224460]. https://doi.org/10.1038/s41386-018-0225-3.
-
9.
Alhammad M, Aljedani R, Alsaleh M, Atyia N, Alsmakh M, Alfaraj A, et al. Family, Individual, and Other Risk Factors Contributing to Risk of Substance Abuse in Young Adults: A Narrative Review. Cureus. 2022;14(12). e32316. [PubMed ID: 36505959]. [PubMed Central ID: PMC9731175]. https://doi.org/10.7759/cureus.32316.
-
10.
Pilozzi A, Carro C, Huang X. Roles of beta-Endorphin in Stress, Behavior, Neuroinflammation, and Brain Energy Metabolism. Int J Mol Sci. 2020;22(1). [PubMed ID: 33396962]. [PubMed Central ID: PMC7796446]. https://doi.org/10.3390/ijms22010338.
-
11.
Gondre-Lewis MC, Elman I, Alim T, Chapman E, Settles-Reaves B, Galvao C, et al. Frequency of the Dopamine Receptor D3 (rs6280) vs. Opioid Receptor micro1 (rs1799971) Polymorphic Risk Alleles in Patients with Opioid Use Disorder: A Preponderance of Dopaminergic Mechanisms? Biomedicines. 2022;10(4). [PubMed ID: 35453620]. [PubMed Central ID: PMC9027142]. https://doi.org/10.3390/biomedicines10040870.
-
12.
Bakar RA, Samhani I, Ahmad I, Nurul AA, Muhamad Jamil NK. D4 and D5 dopamine receptor mRNA expression in human peripheral blood lymphocytes among cooccurring opioid and amphetamine-type stimulants use disorder undergoing methadone maintenance therapy. Bangladesh J Med Sci. 2021;20(3):563-8. https://doi.org/10.3329/bjms.v20i3.52799.
-
13.
Ghaffari K, Dousti Kataj P, Torkaman-Boutorabi A, Vousooghi N. Pre-mating administration of theophylline could prevent the transgenerational effects of maternal morphine dependence on offspring anxiety behavior: The role of dopamine receptors. Pharmacol Biochem Behav. 2023;233:173660. [PubMed ID: 37852327]. https://doi.org/10.1016/j.pbb.2023.173660.
-
14.
Thangaraj A, Periyasamy P, Guo ML, Chivero ET, Callen S, Buch S. Mitigation of cocaine-mediated mitochondrial damage, defective mitophagy and microglial activation by superoxide dismutase mimetics. Autophagy. 2020;16(2):289-312. [PubMed ID: 30990365]. [PubMed Central ID: PMC6984592]. https://doi.org/10.1080/15548627.2019.1607686.
-
15.
Zahari Z, Lee CS, Ibrahim MA, Musa N, Mohd Yasin MA, Lee YY, et al. The AC/AG Diplotype for the 118A>G and IVS2 + 691G>C Polymorphisms of OPRM1 Gene is Associated with Sleep Quality Among Opioid-Dependent Patients on Methadone Maintenance Therapy. Pain Ther. 2016;5(1):43-54. [PubMed ID: 26792136]. [PubMed Central ID: PMC4912965]. https://doi.org/10.1007/s40122-016-0044-3.
-
16.
Reid KZ, Lemezis BM, Hou TC, Chen R. Epigenetic Modulation of Opioid Receptors by Drugs of Abuse. Int J Mol Sci. 2022;23(19). [PubMed ID: 36233105]. [PubMed Central ID: PMC9569510]. https://doi.org/10.3390/ijms231911804.
-
17.
Nielsen DA, Yuferov V, Hamon S, Jackson C, Ho A, Ott J, et al. Increased OPRM1 DNA methylation in lymphocytes of methadone-maintained former heroin addicts. Neuropsychopharmacology. 2009;34(4):867-73. [PubMed ID: 18650805]. [PubMed Central ID: PMC2778040]. https://doi.org/10.1038/npp.2008.108.
-
18.
Peng S, Jiang H, Du J, Lin S, Pan S, Yu S, et al. Methadone Dosage and Plasma Levels, SNPs of OPRM1 Gene and Age of First Drug Use Were Associated With Outcomes of Methadone Maintenance Treatment. Front Genet. 2018;9:450. [PubMed ID: 30420869]. [PubMed Central ID: PMC6216325]. https://doi.org/10.3389/fgene.2018.00450.
-
19.
Ravenelle R, Santolucito HB, Byrnes EM, Byrnes JJ, Donaldson ST. Housing environment modulates physiological and behavioral responses to anxiogenic stimuli in trait anxiety male rats. Neuroscience. 2014;270:76-87. [PubMed ID: 24713371]. [PubMed Central ID: PMC4047719]. https://doi.org/10.1016/j.neuroscience.2014.03.060.
-
20.
Zilkha N, Feigin E, Barnea-Ygael N, Zangen A. Induction of depressive-like effects by subchronic exposure to cocaine or heroin in laboratory rats. J Neurochem. 2014;130(4):575-82. [PubMed ID: 24798661]. https://doi.org/10.1111/jnc.12753.
-
21.
Bawor M, Dennis BB, Tan C, Pare G, Varenbut M, Daiter J, et al. Contribution of BDNF and DRD2 genetic polymorphisms to continued opioid use in patients receiving methadone treatment for opioid use disorder: an observational study. Addict Sci Clin Pract. 2015;10:19. [PubMed ID: 26437921]. [PubMed Central ID: PMC4672523]. https://doi.org/10.1186/s13722-015-0040-7.
-
22.
Hsu CW, Huang TL, Tsai MC. Decreased Level of Blood MicroRNA-133b in Men with Opioid Use Disorder on Methadone Maintenance Therapy. J Clin Med. 2019;8(8). [PubMed ID: 31349687]. [PubMed Central ID: PMC6722972]. https://doi.org/10.3390/jcm8081105.
-
23.
Levran O, Peles E, Hamon S, Randesi M, Zhao C, Zhang B, et al. Nerve growth factor beta polypeptide (NGFB) genetic variability: association with the methadone dose required for effective maintenance treatment. Pharmacogenomics J. 2012;12(4):319-27. [PubMed ID: 21358750]. [PubMed Central ID: PMC3130093]. https://doi.org/10.1038/tpj.2011.6.
-
24.
Huang MW, Chiang TA, Lo PY, Huang CS. Relationship among methadone dose, polymorphisms of dopamine D2 receptor and tri-dimensional personality questionnaire in heroin-addicted patients. Behav Brain Funct. 2016;12(1):24. [PubMed ID: 27580593]. [PubMed Central ID: PMC5007696]. https://doi.org/10.1186/s12993-016-0109-9.
-
25.
Bunten H. Exploring the role of mu opioid receptor (OPRM1) and CYP2B6 gene variations for methadone pharmacogenomics. Can these variations be used to advance toxicological interpretation post-mortem? [dissertation]. Bournemouth University; 2010.
-
26.
Luo X, Wang J, Lu J, Wang X, Miao Y, Li Q, et al. Rab22a Promotes Epithelial-Mesenchymal Transition in Papillary Thyroid Carcinoma by Activating PI3K/AKT/mTOR Signaling Pathway. Biomed Res Int. 2022;2022:1874550. [PubMed ID: 35757470]. [PubMed Central ID: PMC9217539]. https://doi.org/10.1155/2022/1874550.
-
27.
Ulrich-Merzenich G, Kelber O, Koptina A, Freischmidt A, Heilmann J, Muller J, et al. Novel neurological and immunological targets for salicylate-based phytopharmaceuticals and for the anti-depressant imipramine. Phytomedicine. 2012;19(10):930-9. [PubMed ID: 22743246]. https://doi.org/10.1016/j.phymed.2012.05.004.
-
28.
Moustafa AA, Tindle R, Cashel S, Parkes D, Mohamed E, Abo Hamza E. Bidirectional relationship between heroin addiction and depression: Behavioural and neural studies. Current Psychology. 2020;41(8):5195-211. https://doi.org/10.1007/s12144-020-01032-4.
-
29.
Khan M, Baussan Y, Hebert-Chatelain E. Connecting Dots between Mitochondrial Dysfunction and Depression. Biomolecules. 2023;13(4). [PubMed ID: 37189442]. [PubMed Central ID: PMC10135685]. https://doi.org/10.3390/biom13040695.
-
30.
Hyman SE, Malenka RC, Nestler EJ. Neural mechanisms of addiction: the role of reward-related learning and memory. Annu Rev Neurosci. 2006;29:565-98. [PubMed ID: 16776597]. https://doi.org/10.1146/annurev.neuro.29.051605.113009.
-
31.
De Jong CAJ, Schellekens AFA, Ellenbroek BA, Franke B, Verkes RJ. The course of addiction: neurobiological predictors of chronicity. Cambridge, Massachusetts: Academic Publications; 2006.
-
32.
Nawi AM, Ismail R, Ibrahim F, Hassan MR, Manaf MRA, Amit N, et al. Risk and protective factors of drug abuse among adolescents: a systematic review. BMC Public Health. 2021;21(1):2088. [PubMed ID: 34774013]. [PubMed Central ID: PMC8590764]. https://doi.org/10.1186/s12889-021-11906-2.
-
33.
Donny EC, Brasser SM, Bigelow GE, Stitzer ML, Walsh SL. Methadone doses of 100 mg or greater are more effective than lower doses at suppressing heroin self-administration in opioid-dependent volunteers. Addiction. 2005;100(10):1496-509. [PubMed ID: 16185211]. https://doi.org/10.1111/j.1360-0443.2005.01232.x.
-
34.
Donny EC, Walsh SL, Bigelow GE, Eissenberg T, Stitzer ML. High-dose methadone produces superior opioid blockade and comparable withdrawal suppression to lower doses in opioid-dependent humans. Psychopharmacology (Berl). 2002;161(2):202-12. [PubMed ID: 11981600]. https://doi.org/10.1007/s00213-002-1027-0.
-
35.
Amjadi O, Hedayatizadeh-Omran A, Zaboli E, Ghaffari-Hamedani MM, Janbabaei G, Ahangari G. Dopamine receptors gene overexpression in the microenvironment of invasive gastric cancer and its potential implications. Mol Biol Rep. 2023;50(8):6529-42. [PubMed ID: 37330941]. https://doi.org/10.1007/s11033-023-08541-y.