Abstract
Keywords
QSAR design docking drug-likeness Azetidine-2-carbonitriles P. falciparum SwissADME
Introduction
The genus Plasmodium is the causative agent of a life-threatening infection, malarial, globally established as one of the most challenging health concerns. Malarial is transmitted within humans through a bite of infected anopheles mosquitoes (1). The global malarial index shows about 228 million malarial cases yearly with 405,000 record mortalities, where the most affected are children below the ages of 5 years, constituting 585,000 (67%) of all cases (2).
Human malarial is transmitted by five species of Plasmodium, namely, Plasmodium ovale (P. ovale), Plasmodium falciparum (P. falciparum), Plasmodium vivax (P. vivax), Plasmodium malariae (P. malariae), and Plasmodium knowlesi (P. knowlesi) (3-4). The bulk of the fatalities are caused by P. falciparum, the most severe of all the species (5). P. falciparum altered the surface of red blood cells once present in the human body through interceding parasite proteins (6). The hemoglobin is ramshackle into amino acids and heme by enzymes cysteine and aspartic proteinases (7). The entire amino acid constituents are assembled into parasite proteins; although only a fraction of heme is incorporated into parasite hemoproteins, the parasite enzymes detoxified the remaining heme (8).
Efficacies of several drugs such as chloroquine, quinine, pyrimethamine, progu-anil, artemisinin, atovaquone, and mefloquine, in treating malarial has been explored. However, the resistance of the P. falciparum strain to some of these drugs has been the major problem facing the treatment of the noxious disease (9). Hence, the detection and development of new antimalarial agents targeting P. falciparum become an extremely important task to curb the accelerated escalation of this resistance. In light of this, Azetidine-2-carbonitriles reported possessing antimalarial activities (10) could provide an alternative application to the routine antimalarial drugs.
The desire to improve drugs with better antimalarial activities leads to the adoption of quantitative structure-activity relationship (QSAR) studies, an essential process in the field of drug invention and improvement due to its time and cost-effectiveness (11). QSAR is an arithmetical relationship between the structural features (biological activities) of drugs with their physicochemical properties (molecular properties). Through this, substit-utions of various groups at various positions can affect the molecular properties of the compound and hence, instrumentals in the design of antimalarial compounds of novel activities against malarial agents. Various QSAR advances are employed in the studies of biological activities of antimalarial compounds as functions of their molecular properties (12-16). This research focuses on applying QSAR techniques in determining the vital structures of Azetidine-2-carbonitriles, responsible for their antimalarial activities, and utilizing the most important molecular properties in designing derivatives of derivatives Azetidine-2-carbonitriles with enhanced activity against P. falciparum. The drug-like and SwissADME studies of the designed derivatives were conducted, followed by their molecular docking to determine their binding site and energy.
Experimental
Collection of dataset and optimization
The dataset consists of thirty-four derivatives of Azetidine-2-carbonitriles, whose chemical structures and biological activities against the Dd2 strain of P. falciparum were extracted from PubChem as presented in the literature (10). Their activities, expressed as EC50 (μM), were then converted to pEC50 by taking the negative logarithm of the EC50 (M) as indicated in Table 1. The structures of the compounds were drawn using a ChemDraw Ultra 12, and saved in *cdx format before exporting into the spartan’14 version 1.1.2 software and then optimized using DFT (DFT/B3LYP/6-31G*) in a vacuum, this is done using the initial molecular geometry (17).
Descriptors calculation
The thirty-four [34] optimized Spartan 14 structures saved as SDF format were then exported into PaDEL software where about 1,500 molecular descriptors ranging between 0-3D classes of descriptors were calculated (18).
Dataset pre-treatment and division
The dataset descriptors are treated by eliminating constant value descriptors, excessive values of coefficient of correlation, descriptors with less than 0.001 variance values. The treated data set was divided into 27 training compounds (consisting of 80% of the data set) and 7 test compounds (making up the remaining 20%) with the aid of the DatasetDivision1.2 program by employing the Kennard-Stone’s algorithm method (19).
Selection of variables and model develo-pment
Material Studio 8.0 software was employed for the development of a model connecting the biological activities of the Azetidine-2-carbonitriles to their molecular structures. The genetic function algorithm (GFA) component of the material studio was elected to carry out the model development. All possible mixtures of molecular descriptors were searched by the algorithm to produce a good model together with the use of a lack of fit function in measuring the fitness of all individual combinations (20).
Model Validation
The models were subjected to both internal and external validations, where both the leave-one-out (LOO) and leave-many-out (LMO) internal validation techniques were employed. The LOO involves casting away a molecule of the training set before developing a model with the remnant data, and the activity of the discarded compound was in turn predicted by the model, and this was performed across other compounds in the training set. The LMO involves a selection of the group of compounds to validate the developed model. The external validation entails predicting the biological activities of some dataset separated from the training set (test set) applying the model. The best predictive models were chosen based on the values of the coefficient of determination (R2), cross-validated R2 (Q2cv), and the external validated R2 (R2pred) (21). The model with the highest test set (R2pred) prediction was picked as the best model.
Descriptors variance inflation factor (VIF)
The multicollinearity of the model descriptors was investigated employing the variance inflation factor (VIF) (22). The rule of thumb for descriptors VIF (Equation 1) values was set for not greater than 10 as an omen of huge multicollinearity between descriptors (23). The VIF is obtainable by utilizing Equation 1.
(1)
Where Ri denotes the coefficient produced by regressing the descriptor xi against the other descriptors xj (j ≠ i).
Descriptors Mean effect
The mean effect refers to the measures of the descriptor’s relevancies in a generated model; it reveals the respective contributions of molecular descriptors to the selected model. The sign of the mean effect indicates the variations of its contribution as a function of descriptor values. The mean effect could be estimated with the aid of Equation 2.
(2)
where βj Conforms with the descriptor j’s coefficient, Dj conforms with each value of matrix descriptor in the training set, and m conforms with the tally of model descriptors present, and n stands for the tally of molecules used as training set (24).
Model Applicability Domain (AD)
The model limitations are defined by its biological space, which relates to the structural realm and response capacity. Interpretation of the relevant space of the model was performed using Williams’s plot. Higher leverage compounds with values exceeding the caution leverage (h*) in addition to determining standardized residual values beyond plus or minus three standard deviation units were regarded as an impairment (25).
Molecular design
The ligand-based design method was employed to design compounds possessing improved antimalarial activities. Compounds with enhanced activity are usually refined and modeled through this means by linking the empirical activities of the compounds with their molecular structures. Antimalarial derivatives possessing the highest activity will be utilized as a guide (Template) in designing theoretical compounds with elaborating activities.
Molecular docking studies
The molecular docking studies between the ligands and their protein target were carried out with the Molegro Virtual Docker (MVD). The 3D structure of the target protein was extracted from Protein Data Bank (PDB) (www.rcsb.org) and saved in PDB file format. The designed derivatives of Azetidine-2-carbonitriles were docked to a high resolution 2.4 Å crystal structure of Plasmodium falciparum dihydroorotate dehydrogenase (Pf-DHODH) (PDB ID: ITV5).
Ligand and Protein Preparation
The ligands and protein structure saved in PDB format may be lost components such as hydrogen atoms, its charges, etc. The ligands and the protein were imported into the MVD, and were prepared using the preparation wizard (capable of fixing the missing hydrogens and charges) of the MVD. The binding pocket for the interaction of the ligands and the protein target was calculated within the in-built cavity algorithm executed by the MVD software. The MVD cavity detects the algorithm and then performs the molecular docking to predict the ligand’s binding mode and the target protein in the form of a scoring function.
Docking Protocol Validation
The docking protocol was validated through the re-docking of the crystallized ligand unto the binding site of the protein receptor. The initial crystalized ligand was superimposed on the docked pose to produce the value of the root mean square deviation (RMSD). The permitted range of the RMSD value within ≤ 2.0Ǻ validates the docking protocols and confirms its usage in docking (26).
In‒silico ADME prediction
An excellent medication is not necessarily the one with the best binding interactions with the target. Hence, the need to evaluate the ligand’s pharmacokinetic properties, ADME (absorption, distribution, metabolism, and excretion), for their activity within the human system. The compounds that are likely to be taken as oral medication, should be fast and absorb completely from the gastrointestinal tract, distribute in the direction of its target, metabolize slowly, and properly dispense harmlessly. Drug failure has been associated with poor ADME properties (27). The SwissADME, an online ADME prediction tool was deployed in the present studies to predict the drug-like and the pharmacokinetic properties of the sixteen [16] designed derivatives of Azetidine-2-carbonitriles. The predictive absorption for molar refractivity (MR), skin permeability coefficients (log Kp), total polar surface area (TPSA), number of rotatable bonds (nRotB), Gastrointestinal (GI) absorption, and CYP1A2 inhibitor were evaluated besides the Lipinski’s Rule of 5 (RO5), which predicts drug-likeness of the design derivatives were also considered. Lipinski’s RO5 states that compound in excesses of 5 H-bond donors, 10 H-bond acceptors, molecular weight more than 500 Da, and the calculated Log P (MLogP) greater than 5 likely had poor absorption or permeation of the molecular entities. Hence, molecules will unlikely to become orally bioavailable as a drug if they pose properties greater than the desired number (28).
Results and Discussion
QSAR model
Several QSAR models were generated with a large value of the coefficient of determination; however, a model that is robust, efficient, and more reliable model was chosen as the best model based on the significance of its parameters since it has the largest value of R2 = 0.9465, R2Adj = 0.9304, Q2cv = of 0.8981, Q2 (L4O)cv = 0.9272, and R2ext = 0.6915. The robustness and the predictive capacity of the QSAR model were predicted through the statistical parameters. The chosen model is presented below with the names, definitions, and coefficients of the descriptors listed in Table 2.
pEC50 = 5.79415(ATSC5c) - 9.38708(MATS5e) + 12.85927(GATS8i) - 10.11181(SpMax2_Bhp) + 18.90418(PetitjeanNumber) + 1.54996(XLogP) + 18.22399
N = 27, R2 = 0.9465, R2Adj = 0.9304, Q2cv = 0.8981, Q2 (L4O)cv = 0.9272, LOF = 0.4280, R2ext = 0.6915, Next = 7
Model Validation
The high value of Q2cv (0.8981), and that of Q2 (L4O)cv = 0.9272 are indicators of good internal validations; the model was utilized externally to predict the activity of an external set which is reflected in the squared regression coefficient of the test set, R2ext (0.6915). These results are a strong indication of the exclusive (internal and external) validation of a model. The plot of predicted activity against the experimental activity revealed a cluster of data points around the legend line, as shown in Figure 1, indicating the robustness and strength of the selected model. The small difference between the experimental and predicted activity (Table 1) emphasizes the accuracy of the model. Also, the Y-randomization test carried out shows the values of R2 and Q2 obtained after 15 repetitions are far smaller than their values in the model, confirming that the model does not occur by chance.
Descriptors correlation matrix and Varia-nce inflation factor (VIF)
The low variance in the correlation matrix (Table 3) between the model’s descriptors reveals a non-mutual relationship among the descriptors, which was supported by low values of calculated descriptors VIF (< 10) as found in Table 3. Indicating that the descriptors are found to be orthogonal (22), as such the model is statistically significant.
Applicability Domain (AD) of the model
The model application limit defined by the applicability domain reflects the presents of the data sets within space, with no data point located outside the domain, as reflected in Figure 2. The threshold (h*) leverage is estimated for 0.778, beyond which the applicability of the models fails. Therefore, the whole dataset was found to possess decent leverage values and is within the model’s space, affirming the model’s predictive strength.
Interpretation and contribution of descri-ptors
The activity of the model, pEC50 = 5.79415(ATSC5c)-9.38708(MATS5e)+ 12.85927(GATS8i)- 10.11181 (SpMax2_Bhp) + 18.90418 (PetitjeanNumber) +1.54996(XLo-gP) +18.22399, is determined by the constituent descriptors ATSC5c, MATS5e, GATS8i, SpMax2_Bhp, PetitjeanNumber, and XLogP. The first descriptor, ATSC5c, which is defined as centered Broto–Moreau autocorrelation—lag 5/weighted by charges. The descriptor is related to the polarization of the molecules caused by highly electronegative elements present in a compound. The descriptor has a mean effect of MF = -0.3262 (Table 3) which indicates the activity increases with a decrease in the numeric values of the descriptors. The second descriptor, MATS5e belongs to the autocorrelation, and it describes the dependence of the compound on electronegativity (29). The autocorrelation descriptors check out the dependence of properties in one special molecule with the neighbor molecule and detect the conformity of the molecules (30). The mean effect (MF) analysis revealed the descriptor to have made MF = 0.0717 contribution. The positive sign of the MF indicates a positive contribution to the antimalarial activity. Hence, an increase in the value of the descriptor increases the antimalarial activity. The descriptor, GATS8i is a Geary autocorrelation of lag 8 weighted by first ionization potential. The 2D autocorrelation descriptors explained how the values of certain functions (topological distance) at intervals equal to the lag (atomic properties) were correlated. The analysis of the descriptors contribution yields the MF = -1.0598. The negative sign of the mean effect ensures the increase of activity with decrease descriptor values. SpMax2_Bhp is a Barysz matrix type descriptor in which the maximum absolute eigenvalue of Barysz matrix for n = 2 was weighted by polarizability (18). Analysis of the mean effect confirms SpMax2_Bhp to be the most contributive descriptor with MF = 3.3244, whose increase in numerical value increases the activity of compounds due to the positive MF. The value of shape parameter PetitjeanNumber increases when the substituents are changed from F, Cl to CF3, -OCH3 at a ring and hence increases the activity (31). The negative mean effect (MF = -0.7846) implies decreasing the descriptor values to increase the activity of the compound. The last descriptor, XlogP signifies the ratio of solute concentration in octanol & water and generally termed as octanol-water partition coefficient. The negative mean effect (MF = -0.2254) indicates decreasing the descriptor values to increase the compound activity.
Molecular design
The compound with the highest activity (pEC50 = 8.301), compound 25 presented in Figure 3, was adopted as the design template. The descriptor, SpMax2_Bhp (a descriptor in which the maximum absolute eigenvalue of Barysz matrix for n = 2 was weighted by polarizability), was established as the most influential descriptor, was employed in the design of many speculative derivatives of Azetidine-2-carbonitriles. The descriptor relates to the polarizability of a molecule, and since it has a positive mean effect, increasing the polarizability of the compounds should be able to increase the antimalarial activity. Hence, polarizability can increase through the substitution of various electron deactivating groups (F, I, Cl, SO3H, CN, NO2, etc) at different positions of the template. This lead to the design of sixteen [16] speculative derivatives of the template as depicted in Table 4. Ten of the design derivatives (D3-4, D8-13, and D15-16) have better activity than the template. The compound D13 {(2S,3S,4S)-2-cyano-3-(2’-fluoro-4’-phenoxy-[1,1’-biphenyl]-4-yl)-4-(hydroxymethyl)-N-propylazetidine-1-carboxamide}, was found to have better antimalarial activity, (pEC50 = 9.8641) than those of the design template (pIC50 = 8.301), co-designed compounds as well as the chloroquine standard (pEC50 = 6.0242) as reflected in Table 4.
Docking Protocol Validation
The validation of the docking protocols was conducted to ascertain the docking method through the determination of the deviation of the re-docking output from the original docking pose. The deviation expressed as the root mean square deviation (RMSD) value produces the RMSD value of 1.895Å. This, therefore, validate the protocols employed in the docking and can be deployed in docking the designed ligands.
Docking Analysis
The binding conformation of the design derivatives to the binding site of the target protein is discussed in the docking analysis. The structure of Plasmodium falciparum dihydroorotate dehydrogenase (Pf-DHODH) with the target site is reflected in Figure 4. Moreover, the docking result of the designed derivatives, template, and standard drug was shown in Table 5. The interactions of the ligand and the protein residues are analyzed, where hydrogen attached to either the hydroxyl or the Azetidine ring in most ligands showed H-bond interaction with Asp204 or Asp200 active site of the residues. The oxygen of the nitro in all the ligands shows H-bond interaction with either Lys305, Lys239, Lys559, Thr201, Ile206, Met536, Gly535, Asp216, or Asn195 active site residue, except in ligands D2, D3, D12, D13, D14, and D15. H-bond interaction could also be observed between the protein active site Lys239, Lys305, or Leu302 and Oxygen of N-propylacetamide of the ligands. Almost all compounds bar D1, D4, D11, D14, and D16, show H-bond interaction between the Asp200, Asp204, Ser202, Ser477, Ile218, Lys239, and Leu238 active site with methylene hydrogen of hydroxymethyl group of the compounds. Likewise, the oxygen of the hydroxyl group of the D2, D3, D14, and D15 ligands results in H-bond formation with Lys543, Lys239, Asn203, and Gly241 active sites of the protein residue. Seven of the designed derivatives, D2 (-150.8650 kcal/mol), D7 (-140.8770 kcal/mol), D9 (-177.0910 kcal/mol), D10 (-164.6990 kcal/mol), D12 (-150.2670 kcal/mol), D13 (-146.0110 kcal/mol), and D15 (-158.7300 kcal/mol), were found to possess higher binding affinity than the design template (-120.2690 kcal/mol) and the chloroquine standard (-140.3940 kcal/mol). Compound D9 was found to have the highest binding affinity (-177.0910 kcal/mol), as shown in Table 5. Hence, form better interaction than other designed derivatives as well as the standard chloroquine drug. Four H-bond in addition to several hydrophobic interactions were observed between D9 and the protein residue, two of which are conventional, between the oxygen of the nitro group of the ligand with Met536 protein residue, bond distance 2.28Å also, the interaction between the hydrogen of the methylene bridge bonded to a hydroxyl group of the ligand and Ser477 active site with bond distance 1.76Å. The other two interactions are carbon-hydrogen bonding between the oxygen of the ligand nitro group, hydrogen of N-propylacetamide with Gly535 bond distance 2.70Å, and Ala225, bond distance 2.60Å, respectively. Lastly, an unfavorable bump exists between the Asn274 residues with methylene hydrogen, which could add to the observer binding affinity. The binding modes for the best compound, D9, are presented in Figure 5. These interactions show the binding role of oxygen, hydrogen, and carbon atoms as well as their strength of inhibition.
Drug-likeness ADME predictions
The results of Lipinski’s parameters, drug-likeness as well as the in-silico ADMET screening predicted for the designed derivatives of Azetidine-2-carbonitriles were depicted in Table 6. The results show that all the designed derivatives obeyed Lipinski’s rule of five, hence possess excellent drug-like properties (32), other parameters like molar refractivity (MR), and the number of rotatable bonds (nRotB) were determined in addition to Lipinski’s parameters. Molar refractivity measures both the ease of polarization and volume of a compound; it ranges between 40 -130 (33). The rule is deployed to assess the drug-likeness of a drug candidate (34). The nRotB measures the molecular flexibility of the molecule, which should be ≤ 10. The violation of more than one rule of five by a drug candidate is a pointer to the poor oral absorption of the candidate. The great combination of membrane permeability and oral bioavailability are functions of the Log of octanol/water partition coefficient (LogP), Molecular weight (MW), and Total polar surface area (TPSA) values. In addition to the role played by hydrogen bond acceptor (HBA) and hydrogen bond donor (HBD) in determining the hydrophobicity, membrane permeability, and the bioavailability of drug candidates. The results in Table 6 indicate that all compounds are within the parameter range of MW ≤ 500 Da, LogP < 5, nHBD ≤ 5, nHBA ≤ 10, and TPSA < 140 Å2. This shows that the design derivatives are not only bioavailable, they are also membrane-permeable besides their hydrophobicity nature. The predicted ADME values (Table 6) have the skin permeability (log Kp) for the design compounds to be within -6.31 to -5.69 cm/s, lying between the acceptance range –8.0 to –1.0 cm/s (35). With the values of the nRotB ≤10, those of the MR were slightly outside the range. While most compounds showed low gastrointestinal absorption with only compounds D1-3, D13-15 that have high absorption, only a few compounds, D1, D2, D3, D14, and D15, show inhibition to CYP1A2.
Chemical structures and activities of the derivatives of Azetidine-2-carbonitriles against Chloroquine resistance strain, Dd2
Names, definitions, and coefficients of descriptors appearing in the selected model
Descriptor name | Type | Notation | Coefficient | |
---|---|---|---|---|
Constant | 18.22 | |||
1 | Centered Broto-Moreau autocorrelation - lag 5/weighted by charges | 2D-Autocorrelation | ATSC5c | 5.79 |
2 | Moran autocorrelation - lag 5/weighted by Sanderson electronegativities | 2D-Autocorrelation | MATS5e | -9.39 |
3 | Geary autocorrelation - lag 8/weighted by first ionization potential | 2D-Autocorrelation | GATS8i | 12.86 |
4 | Largest absolute eigenvalue of Barysz matrix - n 2 / weighted by relative polarizabilities | Barysz matrix | SpMax2_Bhp | -10.11 |
5 | Petitjean number | Petitjean number | PetitjeanNumber | 18.90 |
6 | XLogP | XLogP | XLogP | 1.55 |
Descriptors correlation matrix, VIF, and their Mean effect
pEC50 | ATSC5c | MATS5e | GATS8i | SpMax2_Bhp | Petitjean | XLogP | VIF | MF | |
---|---|---|---|---|---|---|---|---|---|
pEC50 | 1 | ||||||||
ATSC5c | 0.0516 | 1 | 2.3640 | -0.3262 | |||||
MATS5e | 0.0729 | 0.5890 | 1 | 3.0033 | 0.0717 | ||||
GATS8i | 0.2138 | -0.1170 | 0.3532 | 1 | 2.6423 | -1.0598 | |||
SpMax2_Bhp | 0.2163 | -0.0471 | -0.1380 | 0.2733 | 1 | 1.8832 | 3.3244 | ||
Petitjean Number | 0.3992 | 0.0425 | 0.0150 | 0.2741 | 0.1633 | 1 | 1.1472 | -0.7846 | |
XLogP | 0.7071 | -0.0473 | -0.0205 | -0.2401 | 0.3923 | -0.0038 | 1 | 1.7121 | -0.2254 |
Structures of the template, designed derivatives of Azetidine-2-carbonitriles and Chloroquine standard along with their respective activities
Docking parameters of designed derivatives of Azetidine-2-carbonitriles, template, and chloroquine standard in the active site of PfDHODH protein
Compound No. | MolDock Score (kcal/mol) | No. of H-Bonds | Amino acid involved | Atom of ligands | H-bond length (Å) |
---|---|---|---|---|---|
D1 | -128.8790 | 2 | Lys305 | O of NO2 | 2.48 |
Asp204 | H of OH | 2.14 | |||
D2 | -150.8650 | 11 | Lys543 | O of OH | 2.75 |
Lys543 | O of OH | 2.76 | |||
Ser202 | H of OH | 2.14 | |||
Leu302 | H of Amide | 2.49 | |||
Lys239 | O of N-propylacetamide | 2.71 | |||
Leu302 | N of CN | 2.65 | |||
Asp200 | H of CH2 of hydroxyl methyl | 3.00 | |||
Ser202 | H of CH2 of hydroxyl methyl | 2.64 | |||
H | O | 2.41 | |||
Asp204 | H of Azetidine ring | 2.83 | |||
Leu302 | H of N-propylacetamide | 2.89 | |||
D3 | -128.8700 | 3 | Asp200 | H of OH | 1.74 |
Lys239 | O of OH | 2.84 | |||
Asp200 | H of the CH2 of hydroxymethyl | 2.54 | |||
D4 | -133.4450 | 3 | Lys305 | O of N-propylacetamide | 2.42 |
Asp204 | H of OH | 2.13 | |||
Thr201 | O of NO2 | 2.83 | |||
D5 | -122.6040 | 5 | Lys305 | O of N-propylacetamide | 2.33 |
Asp204 | H of OH | 2.17 | |||
Thr201 | O of NO2 | 2.46 | |||
Thr201 | O of NO2 | 3.08 | |||
Asp204 | H of the CH2 of hydroxymethyl | 2.79 | |||
D6 | -139.4120 | 4 | Leu238 | H of OH | 2.46 |
Ile206 | O of NO2 | 2.67 | |||
Asp200 | H of the CH2 of hydroxymethyl | 2.10 | |||
Asp200 | H of Azetidine ring | 2.55 | |||
D7 | -140.8770 | 3 | Leu238 | H of OH | 2.38 |
Asp200 | H of the CH2 of hydroxymethyl | 2.20 | |||
Asp200 | H of Azetidine ring | 2.32 | |||
D8 | -124.5920 | 6 | Lys239 | O of NO2 | 2.46 |
Lys305 | O of N-propylacetamide | 2.69 | |||
Lys305 | O of N-propylacetamide | 2.55 | |||
Asp204 | H of OH | 1.64 | |||
H | O of OH | 3.09 | |||
Ile218 | H of the CH2 of hydroxymethyl | 2.96 | |||
D9 | -177.0910 | 4 | Met536 | O of NO2 | 2.28 |
Ser477 | H of the CH2 of hydroxymethyl | 1.76 | |||
Gly535 | O of NO2 | 2.70 | |||
Ala225 | H of N-propylacetamide | 2.60 | |||
D10 | -164.6990 | 7 | Lys559 | O of NO2 | 2.36 |
Leu238 | H of OH | 2.10 | |||
Asp200 | H of Amide | 2.02 | |||
Asp216 | O of NO2 | 2.90 | |||
Asp200 | H of the CH2 of hydroxylmethyl | 2.56 | |||
Lys239 | H of the CH2 of hydroxylmethyl | 2.96 | |||
Asp200 | H of Azetidine ring | 2.38 | |||
D11 | -125.9140 | 4 | Asn195 | O of NO2 | 2.44 |
Lys239 | O of NO2 | 1.97 | |||
Lys305 | O of N-propylacetamide | 2.35 | |||
Asp204 | H of OH | 2.20 | |||
D12 | -150.2670 | 6 | Lys305 | O of Oxydibenzene | 2.71 |
Lys239 | H of OH | 2.14 | |||
Asp200 | H of Amide | 2.08 | |||
Leu238 | H of the CH2 of hydroxymethyl | 2.88 | |||
Asp200 | H of Azetidine ring | 2.10 | |||
H | O of OH | 2.80 | |||
D13 | -146.0110 | 4 | Asp200 | H of OH | 1.75 |
Leu302 | N of CN | 2.73 | |||
Ser202 | H of the CH2 of hydroxymethyl | 2.25 | |||
Asp204 | H of Azetidine ring | 2.90 | |||
D14 | -137.2260 | 7 | Thr201 | H of OH | 1.97 |
His306 | H of Amide | 2.59 | |||
Asn203 | O of OH | 2.91 | |||
H of CH2 of hydroxymethyl | O of N-propylacetamide | 2.62 | |||
Ser202 | H of Azetidine ring | 2.88 | |||
Leu302 | O of N-propylacetamide | 2.85 | |||
Asp204 | H of a delocalized benzene ring | 2.99 | |||
D15 | -158.7300 | 6 | Leu238 | H of OH | 2.09 |
Asp200 | H of Amide | 2.14 | |||
Gly241 | O of OH | 2.43 | |||
Asp200 | H of the CH2 of hydroxymethyl | 2.45 | |||
Lys239 | H of the CH2 of hydroxymethyl | 2.89 | |||
Asp200 | H of Azetidine ring | 2.42 | |||
D16 | -134.8030 | 3 | Lys239 | O of NO2 | 1.89 |
Lys305 | O of N-propylacetamide | 2.48 | |||
Asp204 | H of OH | 2.13 | |||
Template | -120.2690 | 3 | Lys305 | O of N-propylacetamide | 2.56 |
Asp204 | H of OH | 2.17 | |||
Asp204 | H of the CH2 of hydroxymethyl | 2.97 | |||
Chloroquine | -140.3940 | 2 | His185 | N of Quinoline ring | 1.54 |
Val532 | H of amine | 2.67 |
Lipinski properties of the derivatives of Azetidine-2-carbonitriles analyzed with SwissADME
Lipinski’s parameters | MR | log Kp (cm/s) | nRotB | GI absorption | CYP1A2 inhibitor | ||||||
---|---|---|---|---|---|---|---|---|---|---|---|
S/N | MW | MLogP (<5) | nHBD (≤5) | nHBA (≤10) | TPSA (<140 Å2) | Lipinski Violation | |||||
D1 | 475.97 | 3.42 | 2 | 4 | 85.59 | 0 | 135.82 | -5.69 | 9 | High | Yes |
D2 | 475.97 | 3.42 | 2 | 4 | 85.59 | 0 | 135.82 | -5.69 | 9 | High | Yes |
D3 | 475.97 | 3.42 | 2 | 4 | 85.59 | 0 | 135.82 | -5.69 | 9 | High | Yes |
D4 | 486.52 | 2.07 | 2 | 6 | 131.41 | 0 | 139.64 | -6.31 | 10 | Low | No |
D5 | 486.52 | 2.07 | 2 | 6 | 131.41 | 0 | 139.64 | -6.31 | 10 | Low | No |
D6 | 486.52 | 2.07 | 2 | 6 | 131.41 | 0 | 139.64 | -6.31 | 10 | Low | No |
D7 | 486.52 | 2.07 | 2 | 6 | 131.41 | 0 | 139.64 | -6.31 | 10 | Low | No |
D8 | 486.52 | 2.07 | 2 | 6 | 131.41 | 0 | 139.64 | -6.31 | 10 | Low | No |
D9 | 520.96 | 2.53 | 2 | 6 | 131.41 | 1 | 144.65 | -6.08 | 10 | Low | No |
D10 | 520.96 | 2.53 | 2 | 6 | 131.41 | 1 | 144.65 | -6.08 | 10 | Low | No |
D11 | 520.96 | 2.53 | 2 | 6 | 131.41 | 1 | 144.65 | -6.08 | 10 | Low | No |
D12 | 520.96 | 2.53 | 2 | 6 | 131.41 | 1 | 144.65 | -6.08 | 10 | Low | No |
D13 | 459.51 | 3.32 | 2 | 5 | 85.59 | 0 | 130.77 | -5.96 | 9 | High | No |
D14 | 567.42 | 3.61 | 2 | 4 | 85.59 | 1 | 143.53 | -6.23 | 9 | High | Yes |
D15 | 520.42 | 3.51 | 2 | 4 | 85.59 | 1 | 138.51 | -5.91 | 9 | High | Yes |
D16 | 565.42 | 2.63 | 2 | 6 | 131.41 | 1 | 147.34 | -6.31 | 10 | Low | No |
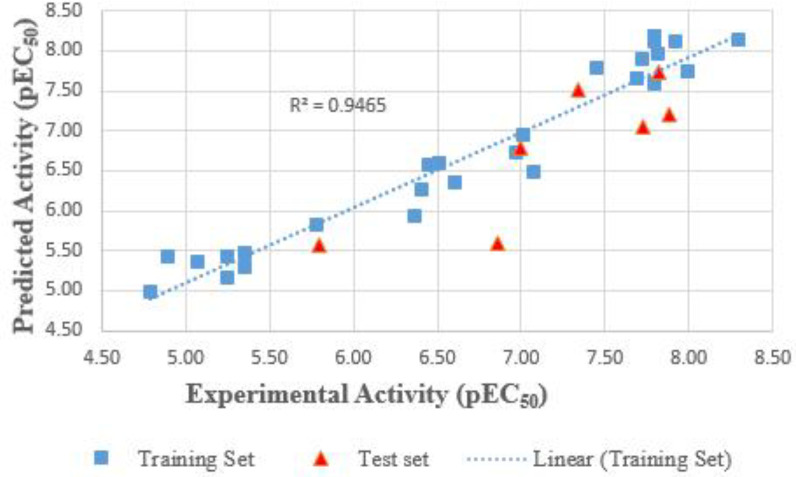
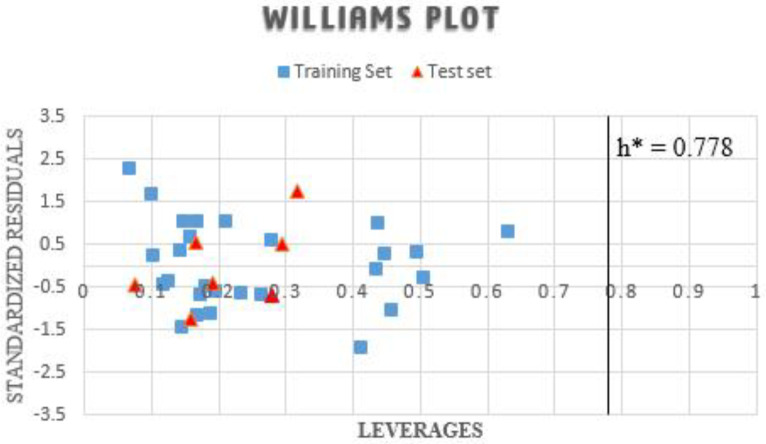
![Design template, Compound 25, (2S,3S,4S)-2-cyano-4-(hydroxymethyl)-3-(4'-phenoxy-[1,1'-biphenyl]-4-yl)-N-propylazetidine-1-carboxamide, with pEC50 = 8.301](https://services.brieflands.com/cdn/serve//315db/3ccb902241ccab5896761f9bc79c162f5cb6e28b/ijpr-20-254-g005.jpg)
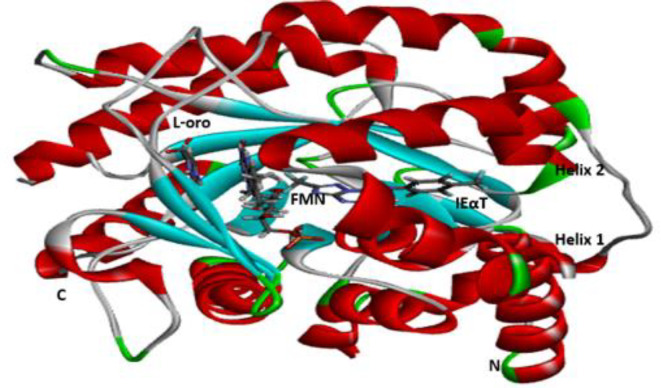
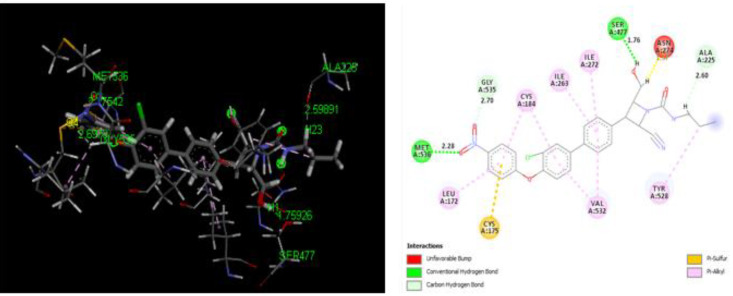
Conclusion
In this research, the ligand-base method was adopted to design sixteen (16) derivatives of Azetidine-2-carbonitriles. Ten of the designed derivatives (D3-4, D8-13, and D15-16) have better activity than the template. Molecular docking studies of the derivatives show the various interactions and the binding sites of the compounds. The compound with the highest binding energy (D9) shows its interaction with Met536, Ser477, Gly535, and Asn274 amino acid residues, which may be responsible for the high antimalarial activities. The designed compounds were found to pass all Lipinski’s RO5. The compounds were found to have their skin permeability coefficient within limits, with most of them having low gastrointestinal absorption, while compounds D1, D2, D3, D14, and D15 show inhibition to CYP1A2.
Authors’ contributions
This research involves the combined efforts of all the authors. ZY. Ibrahim and A. Uzairu, Conceived and designed the research, ZY. Ibrahim and G. Shallangwa, Performed the experiments, ZY. Ibrahim, A. Uzairu, and S. Abechi Analyzed and interpreted the data, ZY. Ibrahim, G. Shallangwa, and S. Abechi Contributed materials, analysis tools, or data, ZY. Ibrahim and S. Abechi, Wrote the manuscript. All authors read and approved the final manuscript.
Funding
The authors of this research did not receive any funding concerning this research.
Availability of data
The datasets used for analysis during these studies were included in this published study.
Ethics approval and consent to participate
This research does not require ethical approval.
Consent for publication
On behave of the authors, I hereby granted the right of this entire article content to this journal.
Declaration of Conflicting Interests
The author(s) declared no potential conflicts of interest concerning the research, authorship, and/or publication of this article.
Acknowledgements
References
-
1.
Pinheiro JC, Kiralj R, Ferreira MMC, Romero OAS. Artemisinin derivatives with antimalarial activity against Plasmodium falciparum designed with the aid of quantum chemical and partial least squares methods. QSAR Comb. Sci. 2003;22:830-42.
-
2.
-
3.
Murray MC, Perkins ME. Chapter 15. Chemotherapy of malaria. Annu. Rep. Med. Chem. 1996;31:141-50.
-
4.
Singh B, Sung LK, Matusop A, Radhakrishnan A, Shamsul SS, Cox-Singh J, Thomas A, Conway DJ. A large focus of naturally acquired Plasmodium knowlesi infections in human beings. Lancet. 2004;363:1017-24. [PubMed ID: 15051281].
-
5.
Sondo P, Derra K, Lefevre T, Diallo-Nakanabo S, Tarnagda Z, Zampa O, Kazienga A, Valea I, Sorgho H, Ouedraogo JB, Guiguemde TR, Tinto H. Genetically diverse Plasmodium falciparum infections, within-host competition and symptomatic malaria in humans. Sci. Rep. 2019;9:1-9.
-
6.
Bowman S, Lawson D, Basham D, Brown D, Chillingworth T, Churcher CM, Barrell BG. The complete nucleotide sequence of chromosome 3 of Plasmodium falciparum. Nature. 1999;400:532-8. [PubMed ID: 10448855].
-
7.
Pandey AV, Tekwani BL, Singh RL, Chauhan VS. Artemisinin, an endoperoxide antimalarial, disrupts the hemoglobin catabolism and heme detoxification systems in malarial parasite. J. Biol. Chem. 1999;274:19383-8. [PubMed ID: 10383451].
-
8.
Kamchonwongpaisan S, Samoff E, Meshnick SR. Identification of hemoglobin degradation products in Plasmodium falciparum. Mol. Biochem. Parasitol. 1997;86:179-86. [PubMed ID: 9200124].
-
9.
Calderón F, Wilson DM, Gamo FJ. Antimalarial Drug Discovery. Prog. Med. Chem. 2013:97-151. [PubMed ID: 23384667].
-
10.
Maetani M, Kato N, Jabor V, Calil FA, Nonato MC, Scherer CA, Schreiber SL. Discovery of antimalarial azetidine-2-carbonitriles that inhibit P falciparum dihydroorotate dehydrogenase. ACS Med. Chem. Lett. 2017;8:438-42. [PubMed ID: 28435533].
-
11.
Ekins S, Mestres J, Testa B. In-silico pharmacology for drug discovery: applications to targets and beyond. Br. J. Pharmacol. 2007;152:21-37. [PubMed ID: 17549046].
-
12.
Paliwal S, Sharma J, Paliwal S. Quantitative structure activity relationship analysis of bisbenzofuran cations as antimalarial agents employing multivariate statistical approach. Indian J. Chem. 2012;51:617-30.
-
13.
Santos CB, Vieira JB, Lobato CC, Hage-Melim LI, Souto RN, Lima CS, Costa EV, Brasil DS, Macêdo WJ, Carvalho JC. A SAR and QSAR study of new artemisinin compounds with antimalarial activity. Molecules. 2013;19:367-99. [PubMed ID: 24381053].
-
14.
Liu H, Qu L, Gao H, Wang J, Han L, Xiang B. Study on the quantitative structure-activity relationship of C-10 substituted artemisinin (QHS) ‘s derivatives using rough set theory. Sci. China. B Chem. 2008;51:937-45.
-
15.
Najafi A, Sobhanardakani S, Marjani M. Exploring QSAR for antimalarial activities and drug distribution within blood of a series of 4-aminoquinoline drugs using genetic-MLR. J. Chem. 2013;2013:1-12.
-
16.
Hadanu R, Mastjeh S, Mustofa M, Sholikhah EN, Wijayanti MA, Tahir I. Quantitative structure-activity relationship analysis (QSAR) of antimalarial 1,10-phenanthroline derivatives compounds. Indo. J. Chem. 2007;7:72-7.
-
17.
Adedirin O, Uzairu A, Shallangwa GA, Abechi SE. Optimization of the anticonvulsant activity of 2-acetamido-N-benzyl-2-(5-methylfuran-2-yl) acetamide using QSAR modeling and molecular docking techniques. J. Basic Appl. Sci. 2018;7:430-40.
-
18.
Yap CW. PaDEL-descriptor: An open-source software to calculate molecular descriptors and fingerprints. J. Comput. Chem. 2010;32:1466-74. [PubMed ID: 21425294].
-
19.
Abdullahi M, Uzairu A, Shallangwa GA, Mamza P, Arthur DE, Ibrahim MT. In-silico modelling studies on some C14-urea-tetrandrine derivatives as potent anti-cancer agents against prostate (PC3) cell line. J. King Saud Univ. Sci. 2020;32:770-9.
-
20.
Rogers D, Hopfinger AJ. Application of genetic function approximation to quantitative structure-activity relationships and quantitative structure-property relationships. J. Chem. Inf. Model. 1994;34:854-66.
-
21.
Golbraikh A, Tropsha A. Beware of q2! J. Mol. Graph. Model. 2002;20:269-76.
-
22.
Myers RH. Classical and modern regression application. 2nd ed. Duxbury Press, California. 1990;2:488.
-
23.
Belsley DA, Kuh E, Welsch RE. Regression diagnostics: identifying influential data and sources of collinearity. John Wiley & Sons, New York. 1980.
-
24.
Minovski N, Župerl S, Drgan V, Novič M. Assessment of applicability domain for multivariate counter-propagation artificial neural network predictive models by minimum Euclidean distance space analysis: A case study. Anal. Chim. Acta. 2013;759:28-42. [PubMed ID: 23260674].
-
25.
Netzeva TI, Worth AP, Aldenberg T, Benigni R, Cronin MT, Gramatica P, Jaworska JS, Kahn S, Klopman G, Marchant CA. Current status of methods for defining the applicability domain of (quantitative) structure-activity relationships. ATLA. 2005;33:155-73. [PubMed ID: 16180989].
-
26.
Kontoyianni M, McClellan LM, Sokol GS. Evaluation of docking performance: comparative data on docking algorithms. J. Med. Chem. 2004;47:558-65. [PubMed ID: 14736237].
-
27.
Hodgson J. ADMET--turning chemicals into drugs. Nat. biotechnol. 2001;19:722-6. [PubMed ID: 11479558].
-
28.
Ibrahim ZY, Uzairu A, Shallangwa G, Abechi S. Molecular docking studies, drug-likeness and in-silico ADMET prediction of some novel β-Amino alcohol grafted 1,4,5-trisubstituted 1,2,3-triazoles derivatives as elevators of p53 protein levels. Sci. Afr. 2020;10:e00570.
-
29.
Lee A, Mercader AG, Duchowicz PR, Castro EA, Pomilio AB. QSAR study of the DPPH radical scavenging activity of di(hetero)arylamines derivatives of benzo[b]thiophenes, halophenols and caffeic acid analogues. Chemom. Intell. Lab. Sys. 2012;116:33-40.
-
30.
Fernández M, Caballero J. QSAR modeling of matrix met-alloproteinase inhibition by N-hydroxy-a-phenylsulfonylaceta-mide derivatives. Bioorg. Med. Chem. 2007;15:6298-310. [PubMed ID: 17590339].
-
31.
Amin SA, Gayen S. Modelling the cytotoxic activity of pyrazolo-triazole hybrids using descriptors calculated from the open source tool “PaDEL-descriptor. J. Taibah Univ. Sci. 2016;10:896-905.
-
32.
Kalita J, Chetia D, Rudrapal M. Molecular Docking, Drug-likeness Studies and ADMET Prediction of Quinoline Imines for Antimalarial Activity. J. Med. Chem. Drug Des. 2019;2:1-7.
-
33.
Mishra S, Dahima R. In vitro adme studies of tug-891, a gpr-120 inhibitor using swiss adme predictor. J. Drug Deliv. Ther. 2019;9:366-9.
-
34.
Rudrapal M, Washmin Banu Z, Chetia D. Newer series of trioxane derivatives as potent antimalarial agents. Med. Chem. Res. 2017;27:653-68.
-
35.
Gaur R, Thakur JP, Yadav DK, Kapkoti DS, Verma RK, Gupta N, Khan F, Saikia D, Bhakuni RS. Synthesis, antitubercular activity, and molecular modeling studies of analogues of isoliquiritigenin and liquiritigenin, bioactive components from Glycyrrhiza glabra. Med. Chem. Res. 2015;24:3494-503.