Abstract
Keywords
COVID-19 Protease inhibitors ADMET Molecular dynamic PCA DCCM MM-PBSA
Introduction
A novel pandemic was emerged in 2019 by severe acute respiratory syndrome coronavirus 2 (SARS-CoV-2); also, this novel pandemic called COVID-19 (1, 2). SARS-CoV-2 mortality is currently computed in the range of 0.5-6% (3). Data from previous studies have indicated that older patients, especially those with underlying disease, are at higher risk in the term of Covid-19 illness and death than younger persons (4). The SARS-CoV-2 virus has a higher transmission capacity than SARS-CoV in 2003. The sudden increase in confirmed cases has made it very difficult to control the spread of COVID-19 (5). In many countries, at least 25 drugs include chloroquine, azithromycin, hydroxychloroquine, favipiravir, lopinavir-ritonavir, ribavirin, nelfinavir, Thalidomide, remdesivir, convalescent plasma, steroids, interferon, and anti–IL-6 inhibitors have been investigated for a large number of COVID-19 patients (6-10). There is currently no clinical evidence to support the effects of drugs against COVID-19 (6). Therefore, efforts are still in progress to find the best therapeutic combination for this virus.
The large genome of the coronavirus is a single-stranded positive-sense RNA (11). The gene size is in the range of 26,000 to 32,000 base; the number of open reading frames (ORFs) of the SARS-Cov-2 genome varies from 6 to 11 (12). On January 10, 2020, the complete sequence of the SARS-Cov-2 genome was released, which helped the rapid detection of the virus in patients using reverse transcriptase-polymerase chain reaction (RT-PCR) (13). The SARS-Cov-2 genomic organization consists of a 5′-untranslated region (UTR), a replicase complex (ORF1ab), a membrane protein (M) gene, an envelope protein (E) gene, a nucleocapsid protein (N) gene, a spike protein (S) gene, 3′-UTR, and several non-structural ORF (14). The major protease required for the coronavirus’s proteolytic maturation is called Mpro (3-chymotrypsin-like protease (3CLp)). This enzyme is one of the attractive and best-characterized targets for drug design because it plays an essential role in the immune regulation and cleavage of virus polyproteins (15). 3CLp is accountable for cleavage of the eleven locations, resulting in 16 non‐structural proteins (NSP) in SARS-CoV-2 (16). The 3CLp (PDB ID: 6W63) was used as a drug target in the present study.
The life cycle of the SARS-Cov-2 is as follows: The virus first attaches to the cell surface by binding to its receptor on the cell surface (ACE2) and enters the cell. After this step, the RNA is released, and a viral polyprotein is made inside the cytoplasm. This polyprotein is cleavaged by the 3CLp, and functional proteins are produced. The inhibitor designed in the present study inhibits this step of the virus life cycle (step 5 in Figure 1). Replication occurred after creating the viral protein, and the RNA and viral proteins are assembled into the new virion in the Golgi apparatus, and eventually, new virions release from the cell (Figure 1) (17, 18).
Drug discovery in the traditional procedure is a time-consuming process (more than a decade) (19), and finding an antiviral drug for COVID-19 in a short time seems to be essential. Hence, we cannot wait for the discovery of COVID-19 medicines through the traditional method. The rapid improvement of technology over the past few decades has opened many exciting ways to the scientist. One of them is computer-aided drug design (CADD), which utilizes computational software to assess the binding features of diverse compounds interacting with various targets (20). Therefore, CADD is an effective way to discover new drugs for diseases. CADD is essential to reduce laboratory costs and increase speed in drug discovery (21). The methods used in the present study include molecular docking, molecular dynamics (MD) simulations, principal component analysis (PCA), dynamical cross-correlation map (DCCM), and MM-PBSA.
Screening of approved drugs by researchers lead to recognized the HIV-1 protease inhibitor (nelfinavir) as a compound that prevented SARS-Cov-2 replication in-vitro (22). Previously, it has been demonstrated that nelfinavir is a potential inhibitor of SARS-Cov-2 protease (3CLp) based on CADD studies. Also, its antiviral activity was approved in duplicates in Vero E6 cells (23, 24). We have chosen nelfinavir as a positive control drug based on its docking energy.
Experimental
Ligands preparation
We have used a large library of natural compounds provided from the ZINC database. This purchasable library by the name of AnalytiCon Discovery NP contains over 11200 compounds. The ligand structures were minimized, and then the necessary hydrogen was added by UCSF Chimera software (25).
3CLp preparation and assign positive control
The crystal structure of SARS-Cov-2 main protease (3CLp) (PDB ID: 6W63, Resolution: 2.10 Å, R-Value Free: 0.221, R-Value Work: 0.150) was engaged for molecular modeling simulations (26). Water molecules and heteroatoms, except native ligand (non-covalent inhibitor X77), were removed, and then bond orders were defined. Hydrogen atoms were added (whole non-polar hydrogen atoms were removed) to the structure. All-atom charges were calculated by UCSF Chimera using Gasteiger–Marsili method (25, 27, 28). To relax all closed contact and prevent clashes, the structure’s minimization was accomplished by the steepest descent method implemented in GROMACS 5.1 (29). Based on the previous studies, we have chosen several drugs for positive control includes remdesivir (30), chloroquine (31), hydroxyl-chloroquine (32), nelfinavir (11), ribavirin (33), arbidol (umifenovir) (34), dihydroergotamine (35), and glecaprevir (36).
Docking study against 3CLp of SARS-Cov-2
Molecular docking of the AnalytiCon Discovery library (11200 structures) into the active site of 3CLp was carried out by AutoDock Vina (37). This software is an academic-free molecular docking and uses a Python script collection (Autodock Tools (38)) to set up docking. AutoDock Vina works based on empirical scoring functions. All default parameters were set for docking runs (37). Finally, five compounds with the lowest docking energy were selected. The compound with the lowest docking energy was picked as a positive control for comparison with designed inhibitors among the studied control drugs. The 2D diagrams of compounds were created by web-based proteins plus software (https://proteins.plus/) (39).
Computational ADME estimation
An essential aspect of drug discovery is that avoided drugs do not have good absorption, distribution, metabolism, and excretion (ADME) properties (40, 41). Unfortunately, most of the drug candidates fail in-vivo studies due to poor ADME properties. Here, the ADME of the five best ligands and positive control were computed using the SwissADME. The 3D structure of chosen and positive control in mol2 format was imported to calculate the ADME properties. The SwissADME is a web-based software that predicts some drug features. SwissADME software can examine the physicochemical properties, lipophilicity, water-solubility, pharmacokinetics, drug-likeness, and medicinal chemistry (42). The absorption (ABS) was calculated using Equation 1 (43, 44). Finally, a ligand with the best feature was selected for MD simulation.
Equation 1.
Molecular dynamic (MD) simulation
The best ligand and positive control selected after docking and ADME studies in complex with 3CLp of SARS-Cov-2 were introduced to MD simulation. All MD simulations were accomplished by GROMACS 5.1 collection with AMBER 99SB force field (29). The partial charges and topology files of ligands were computed by ACPYPE/AnteChamber (45). Each system was solvated with a TIP3P water model in a cubic box of 1 nm in periodic conditions, and related Cl− and Na+ atoms were added to neutralize the system at a 0.15 mol L-1 concentration (46). Energy minimizations were done by the steepest descent and the conjugate gradient algorithm consecutively to achieve a maximum force of less than 1000 kJ mol-1 nm-1 on every atom. A twin-range cutoff scheme was determined to appraise short-range, non-bonded interactions, with van der Waals interactions truncated at 1.4 nm and electrostatic interactions truncated at 0.9 nm. To treat the Long-range electrostatic interactions, the particle mesh Ewald (PME) method was engaged (47, 48). The temperature was set at 300 K (ref_t) using velocity rescaling with a stochastic term and coupling time constant (tau_t) of 0.1 ps (49). This thermostat is comparable with Berendsen coupling, with the same scaling using tau_t, but the stochastic term ensures that a proper canonical ensemble is produced (29). The pressure was fixed at 1.0 atm using a Parrinello-Rahman barostat with a coupling constant of 2 ps (50). All of the covalent bonds involving hydrogen atoms were constrained by a Linear Constraint Solver (LINCS) algorithm to preserve constant bond lengths (51). The number of iterations to correct for rotational lengthening in LINCS (lincs_iter) and highest order in expanding the constraint coupling matrix (lincs_order) was assigned 1 and 4, respectively. Each system was equilibrated under a constant volume NVT (constant number of particles (N), volume (V), and temperature (T)) ensemble (100 ps) and a constant pressure NPT (constant number of particles (N), pressure (P), and temperature (T)) ensemble (100 ps) (52). All MD simulations were conducted for 200 ns. The trajectories were analyzed using free VMD software (53). The “readHBmap.py” Python script, was engaged to extract the percentage occupancy of H-bonds from H-bonds.xpm generated by h_bond GROMACS command (54).
Principal component analysis (PCA)
The PCA is a method that decreases the complexity of the data and draws out of the motion in simulations that are probably significant for biological function. In the PCA, a covariance matrix was manufactured from the trajectories after removing the rotational and translational movements. The computation of the projection of eigenvalues (Eigva) and eigenvectors (Eigve) along the first two PCA was performed using gmx_covar and gmx_anaeig GROMACS tools (55, 56). The Eigva and Eigve were distinguished by diagonalizing the matrix. Eigva demonstrates the amplitude of the Eigve along with multidimensional space, while the replacement of Cα atoms along each Eigve exhibits the concerted motions of the enzyme along each direction. This procedure divided the enzyme into two conformational subspaces. The first is an essential subspace, and the second is a physically non-essential subspace (57).
Dynamical cross-correlation map (DCCM)
To evaluation of the dynamics of systems, a DCCM was created to characterize cross-correlated displacements of the backbone Cα atoms during the simulations. DCCM demonstrated the largest motions within the system. The DCCM map was calculated by Equation 2 (58, 59):
Equation 2.
Where i and j display i-th and j-th residues and 𝚫ri and 𝚫rj correspond to the replacement of i-th and j-th atom from the mean position, respectively (58, 59), the DCCM map was created by an R base analysis tool (56, 60).
Binding free energy computation
Modified Molecular Mechanics–Poisson Boltzmann Surface Area (MM-PBSA) was engaged to compute binding free energy between ligands and receptors. Recently, the MM-PBSA has been used as a scoring function in silico drug design (61, 62). In the present study, the MM-PBSA method was carried out to estimate the interaction free energy between ligands and 3CLp of SARS-Cov-2. In our study, 2,000 snapshots were extracted at every 10 ps of intervals from the last 20 ns of MD trajectories. The free energy of binding is calculated by Equation 3:
Gbinding = Gcomplex – (Gprotein + Gligand)
Equation 3.
Where Gcomplex is the total free energy of the receptor-ligand complex, and G-protein and Gligand are the total free energies of the alone protein and inhibitor in the solvent, respectively (63, 64).
Results and Discussion
Docking and ADME studies
Five combinations with the lowest docking energy were selected among the 11200 natural products library (Table 1). The studied drugs were docked inside the active site of the 3CLp protease under the same condition. Among these drugs studied, remdesivir and nelfinavir have the best docking energy, but nelfinavir is a specialized protease inhibitor of human immunodeficiency viruses (HIV) (65) and remdesivir inhibits RNA-dependent RNA polymerase (66). Therefore, nelfinavir was selected as a positive control.
The ADME properties are listed in Table 2. SwissADME has computational filters that include Lipinski, Ghose (67), Veber (68), Egan (69), Muegge rules (70). The ZINC31157475 has the best properties in these rules (Table 2). The ZINC31157475 has a molecular weight of fewer than 500 g-mol-1. Among all designed compounds, just the ZINC31157475 has a hydrogen bond (H-bond) acceptor lower than 10. All of the proposed natural products have a synthetic accessibility count of less than 10; thus, they are synthesized easily. The ZINC31157475 has the best value of synthetic accessibility. All of the proposed compounds have the proper count of predicted octanol/water partition coefficient (log Po/w), which is the classical descriptor for lipophilicity (valuable range of log Po/w is -0.4 to +5.6) (71, 72). The TPSA acceptable range is between 20 and 130 Å2. TPSA is critical in the prediction of absorption and brain access (72). All compounds except the ZINC31157475 have higher TPSA than the normal range. The ZINC31157475 (66.73%) calculated ABS is higher than all compounds listed in Table 2. The solubility of the ZINC31157475 is better than nelfinavir (log S values in the range 0 to -2 are soluble) (73). The ZINC31157475 demonstrated high gastrointestinal (GI) absorption as indicated by its high affinity for permeability glycoprotein (P-gp substrate) (Table 2). Therefore, we selected the ZINC31157475. The docking energy of the ZINC31157475 was -8.391 kcal mol-1. The docking energy of the selected positive control (nelfinavir) was -7.54 kcal mol-1. 3CLp residues interacting with ligands after docking were shown in Table 1. His41 and Glu166 residues has most interacted with the ligands (Table 1).
Evaluation of the 2D interaction of selected compounds
Residues that interacted with the ZINC31157475 and nelfinavir are listed in Table 1. Here, we demonstrated the 2D diagram of these residues at the 3CLp active site (Figure 2). Five residues have interacted with a selected compound, including Cys44, Tyr54, His163, His164, and Glu166 (Figure 2A). There are five H-bond between these residues and the selected ligand. There is no hydrophobic interaction between the active site and the ZINC31157475. As seen in Figure 2B, His41, Met49, Leu141, Glu166, Pro168 residues have interacted with nelfinavir. There are fewer H-bond (two H-bonds) in the 2D diagram of nelfinavir than the ZINC31157475. H-bonds play a vital role in the attachment of ligands in the active site (74).
Root-Mean-Square Deviation of 3CLp during the MD simulations
Root-Mean-Square Deviation (RMSD) of 3CLp-ZINC31157475, 3CLp-Nelfinavir, and un-ligated 3CLp systems during the MD simulations (200 ns) is shown in Figure 3. RMSD provides a better view of the stability of the studied system. RMSD of the un-ligated 3CLp (Red-line in Figure 3A) increases to 2.12 Å at the 3,180 ps. After that, RMSD was decreased to 1.23 Å at 10,650 ps. From 10,650 ps, an upward trend in RMSD starts and continues until 22,550 ps, at which time RMSD reaches 3.08 Å. Then, there is a downward trend until 38140 ps, which reaches 1.58 Å. In the range of 38,140-83,430 ps, the un-ligated 3CLp system reaches relative equilibrium. After this time, some drift is observed in the RMSD value, which finally reaches equilibrium after 100 ns of simulation (Figure 3A). The RMSD value of the 3CLp-Nelfinavir complex was reached 3.57 Å at 6,000 ps (Blue-line in Figure 3A). After that, an increase of RMSD value has happened until 11230 ps of the simulation. Then RMSD was increased to 3.08 Å at 26740 ps. A relative equilibrium was observed in the 3CLp-Nelfinavir system at 26,740-46,590 ps of MD simulation. The RMSD value was increased again to 3.29 Å at 48770 ps. Another drift has occurred, and the RMSD decreases to 1.72 Å at 53,110 ps. Then, slight changes in the RMSD value are observed till 100,000 ps. After this time, an upward trend in the value of RMSD is formed, which reaches 3.58 Å at 148,950 ps. Then at 160,840 ps, the RMSD value reaches 2.34 Å. Finally, the simulation was ended with an RMSD value of 3.58 Å at 200,000 ps (Figure 3A). The RMSD value of the 3CLp-ZINC31157475 complex (Black-line in Figure 3A) was increased to 3.25 Å at 22,630 ps. Then the system was reached equilibrium until 77,500 ps. After that, an increase of RMSD value was demonstrated to 3.3 Å at 79,640 ps of MD simulation. Then, after a slight decrease in the RMSD value, a proper equilibrium is observed until the end of the simulation (200 ns) (Figure 3A). The 3CLp-ZINC31157475 RMSD value is in the range of 0.80–3.45 Å, while 3CLp-nelfinavir is in the range of 0.82–4.06 for 200 ns simulation time. The average RMSD was 2.38 ± 0.4 Å (3CLp-ZINC31157475), 2.62 ± 0.43 Å (3CLp-Nelfinavir), and 2.35 ± 0.34 Å (un-ligated 3CLp). The 3CLp-ZINC31157475 RMSD value is lower than the 3CLp-Nelfinavir. Therefore, the 3CLp-ZINC31157475 was more stable than the 3CLp-Nelfinavir system (Figure 3A).
The RMSD values of the ligands were also calculated and are shown in Figure 3B. Low fluctuations and low RMSD values (less than 2 Å) indicate high ligand stability in the active site cavity (75). The average RMSD value for ZINC31157475 and Nelfinavir was 0.76 ± 0.24 and 1.76 ± 0.17, respectively. Therefore, the ZINC31157475 inhibitor is more stable inside the active site.
The radius of gyration analysis
The radius of gyration (Rg) represents the folding and unfolding of protein structure during the MD simulation. Therefore, Rg was computed to distinguish the compactness of the system over the run time (Figure 3C). Higher Rg values elucidate less compactness, with high conformational entropy, while low Rg values show more stability and compactness in the structure (76, 77). The average Rg was 21.81 ± 0.13 Å (3CLp-ZINC31157475), 21.99 ± 0.13 Å (3CLp-Nelfinavir), and 21.92 ± 0.18 Å (un-ligated 3CLp). The data manifest that all three systems were compact and well converged throughout the simulation. The average Rg in the 3CLp-ZINC31157475 complex was less than the positive control system (3CLp-Nelfinavir), which indicates the binding of ZINC31157475 leads to increases in the stability and compactness of the enzyme compared to nelfinavir.
Root-Mean-Square Fluctuation of 3CLp during the MD simulations
Root-Mean-Square Fluctuation (RMSF) analysis versus the residue number for 3CLp-ZINC31157475, 3CLp-Nelfinavir, and un-ligated 3CLp systems during the 200 ns MD simulations were calculated by using the gmx_rmsf tool, and the results are illustrated in Figure (4). The RMSF of the backbone atoms is computed to decipher the flexibility of the structure. The high value of RMSF indicates the flexible region, while the low value of RMSF indicates limited motions during MD runs (78).
Three domains organize the 3CLp: domains I and II form the antiparallel β-barrel structure, while a compact α-helical domain is formed by domain III. The active site is located between domains I and II (79). Previous studies have shown that residues His41 (catalytic residue), Phe140, Asn142, Gly143, Ser144, Cys145 (catalytic residue), Tyr161, His163, Glu166, and His172 form the major part of the active site (80). The RMSF value of His41 (as a base catalyst) in the 3CLp-ZINC31157475 and 3CLp-Nelfinavir are 0.55 Å and 0.52 Å, respectively. The flexibility of the cys145 is lower (0.7 Å) in the term of ZINC31157475 binding than nelfinavir (1.92 Å) binding. It has been indicated that ZINC31157475 binding to Cys145 was better than nelfinavir.
There are seven regions in the RMSF plot (Figure 4A) in which the flexibility of the three systems is different. The first region (Figure 4B) includes 44-64 residues that residues 44-51 have lower flexibility after binding ZINC31157475 with 3CLp than nelfinavir binding. But residues 52-64 have higher RMSF in 3CLp-ZINC31157475 than 3CLp-Nelfinavir complex. There is a small helix in this region formed by 45-50 residue (81). The next region is the connecting loop (Loop C) between domains I and II (Figure 4C), which contribute to access to the active site (82). The flexibility of 3CLp-ZINC31157475 is higher than 3CLp-Nelfinavir in loop C (Figure 4A). The next loop (Loop D) is formed by residues 153-157 (Figure 4D), and in this small loop, the RMSF of 3CLp-ZINC31157475 is lower than 3CLp-Nelfinavir. The highest RMSF value in loop D is 2.91 Å and 3.8 Å for 3CLp-ZINC31157475 and 3CLp-Nelfinavir, respectively. The β-hairpin loop (Loop E) is prepared in Figure 4E. Loop E is formed by residues 166-170 (81). The β-hairpin loop consist of Glu166, and His172 residues, which are located in the active site. The RMSF value of the Glu166 and His172 in the 3CLp-ZINC31157475 complex is 0.75 Å and 0.74 Å, respectively. Besides, the RMSF value of the Glu166 and His172 in 3CLp-Nelfinavir is 1.06 Å and 0.75 Å, respectively. The lower RMSF value of the Glu166 in 3CLp-ZINC31157475 than 3CLp-Nelfinavir indicates a better binding of the ZINC31157475 to the active site (Figure 4). Loops are one of the vital structural parts of proteins. The roles of loops include specificity, regulating enzyme catalysis, stability, flexibility, and protein-protein interactions (83). The fifth region in Figure 4A is another loop that is formed by residues 187-197 (Loop F) (Figure 4F), which helps the ligands relocate to shift closer to the β-hairpin loop (81). The loop F is more flexible in the 3CLp-ZINC31157475 than 3CLp-Nelfinavir. More flexibility in loop F can be suitable because a high rate of fluctuations in loop F can conduct the ZINC31157475 inhibitor relocation to move closer to the β-hairpin loop. Region G in the RMSF plot illustrates the loop G with the most flexibility, in which residue 222 has an RMSF value of 3.72 Å and 3.62 Å in 3CLp-ZINC31157475 and 3CLp-Nelfinavir, respectively (Figure 4G). The final region is named H, which contains a helix and was formed by residues 240-260. In this residues the RMSF value of 3CLp-Nelfinavir is lower than 3CLp-ZINC31157475 (Figures 4A and 4H). Overall, the flexibility of 3CLp-ZINC31157475 is lower than 3CLp-Nelfinavir and un-ligated 3CLp.
Hydrogen bond analysis
H-bonds play a fundamental role in many protein features that include protein folding, the binding strength of protein-ligand interaction, and the catalysis function of the enzyme (84, 85). As we have shown in Figure 5A, the highest number of H-bond was four and five for 3CLp-Nelfinavir and 3CLp-ZINC31157475 complex, respectively. The results showed that in most of the simulation time, the number of H-bond in the 3CLp-ZINC31157475 was more than the 3CLp-Nelfinavir. The number of H-bond indicates the potent inhibitory of the ZINC31157475 compared to nelfinavir. The 3D interaction between drugs and the active site of the protein is examined after extracting a snapshot from the last frame of MD simulation (Figure 5C). The 3D interaction view is indicated that ZINC31157475 and nelfinavir make three and two H-bond with the active site residues, respectively. Also, there is a hydrophobic interaction between drugs and proteins (not shown in Figure 5C). On the other hand, there are a little more intermolecular H-bonds for 3CLp-ZINC31157475 when compared to 3CLp-Nelfinavir and un-ligated 3CLp (data not shown). More intermolecular H-bonds in the 3CLp-ZINC31157475 structure might make it more stable.
High H-bonds occupancy (the ratio of the number of times that particular H-bond is present relative to the total time of the simulation) indicates the stability of the H-bond during the MD simulation (86). The occupancy of H-bonds between 3CLp and ligands is shown in Figure 5B. Based on Figure 5B, the H-bonds formed between ZINC31157475 and 3CLp are more stable (higher occupancy) than nelfinavir H-bonds during MD time.
Principal component analysis
Overall, enzymes accomplish their specific roles through collective atomic motions. Hence, a collective atomic motion of a specific enzyme is employed as a parameter to figure out the stability of the enzyme (41, 87). The effect of the overall motion of enzymes due to ligands attachment was analyzed by PCA using the construction of eigenvectors. PCA is one of the powerful methods used to determine the rigidity of each atom and large-scale motions during the MD simulation (58). Figure 6 displayed the conformational sampling of un-ligated 3CLp and ligated 3CLp in the required subspace by projecting the Cα atom along eigenvectors 1 and 2. The results showed that 3CLp bound with ZINC31157475 had a different conformational fluctuation compared to nelfinavir binding (Figure 6). All three systems have good stability, but a reduction in the occupied conformational space by the 3CLp-ZINC31157475 complex is observed, which is consistent with the stability results of the RMSD, Rg, and the intermolecular H-bonds.
Dynamic cross-correlation map
To illuminate the effect of ZINC31157475 and nelfinavir binding on the internal dynamics of 3CLp, the DCCM was constructed and is displayed in Figure 7. The DCCM analysis manifests the relevance between residues. Positive values (cyan color) illustrate residues that displace in the same direction, whiles negative values (pink color) are associated with the opposite displacement. The DCCM results indicated that ZINC31157475 and nelfinavir affect the structural remodeling of the 3CLp protease of SARS-Cov-2 as demonstrated by the change in the motions compared with the un-ligated 3CLp. Altogether, behind the ZINC31157475 and nelfinavir binding, a significant increase in the anti-correlated motions is seen for both complexes (Figure 7). The line determines the binding region of 3CLp in Figure 7A-C. The binding region included residues 20-50 and 140-190.
As seen in Figure 7, the un-ligated system showed an overall correlated motion at binding region residues highest than that of the ligated systems. There is not much difference in correlated motions in the binding region of the 3CLp-ZINC31157475 and 3CLp-Nelfinavir systems. Both studied inhibitors increase anti-correlated motions in the binding region. Overall, the binding of ZINC31157475 and nelfinavir with 3CLp construct a stable environment around the binding cavity. In domain III (indicated by the dashed line in Figure 7), the anti-correlated motions are higher in domain III of the 3CLp-ZINC31157475 system. Overall, ZINC31157475 binding resulted in more anti-correlated motions (Deeper pink color) than nelfinavir in the protein; therefore, it indicates more stability of the 3CLp-ZINC31157475 complex.
Binding free energy analysis
Finally, at the end of the MD simulation, the binding free energy of inhibitors was calculated by MM-PBSA (Table 3). Based on these results, the binding free energy of nelfinavir and ZINC31157475 were -19.54 ± 37.80 kcal mol-1 and -88.03 ± 29.84 kcal mol-1, respectively (Table 3). Therefore, the 3CLp-ZINC31157475 complex has the lowest binding free energy. As shown in Table 3, only the polar solvation energy in the 3CLp-ZINC31157475 complex is higher than that of the 3CLp-Nelfinavir. The electrostatic energy of the 3CLp-ZINC31157475 complex (-61.61 kcal mol-1) is lower than the 3CLp-Nelfinavir complex (-39.19 kcal mol-1). The MM-PBSA results listed in Table 3, which manifest the improved interactions between the ZINC31157475 and 3CLp, give hope for a strong inhibitor.
Subsequently, we probed the vital residues engaged in the receptor-ligand binding by extracting the per-residue binding free energy using MM-PBSA. The per-residue binding free energy of both studied complexes was shown in Figure 8, and residues with lower energy than -1.0 kcal mol-1 are specified in the figure. The residues involved in the 3CLp-Nelfinavir binding include the Glu14, Met49, Asp48, Glu55, Asp92, His163, Glu166, Asp176, Asp187, and Asp197. Also, binding residues of the 3CLp-ZINC31157475 include the Thr25, Leu27, Thr45, Ser46, Met49, Lys61, Cys145, His163, Met165, Glu166, Asp187, Gln189, and Asp197. It is noteworthy that the designed inhibitor (ZINC31157475) binds to Cys145 (one of the catalytic residues) with appropriate energy (-5.39 kcal mol-1), but the binding energy of nelfinavir to His41 and Cys145 is -0.25 and -0.1 kcal mol-1, respectively.
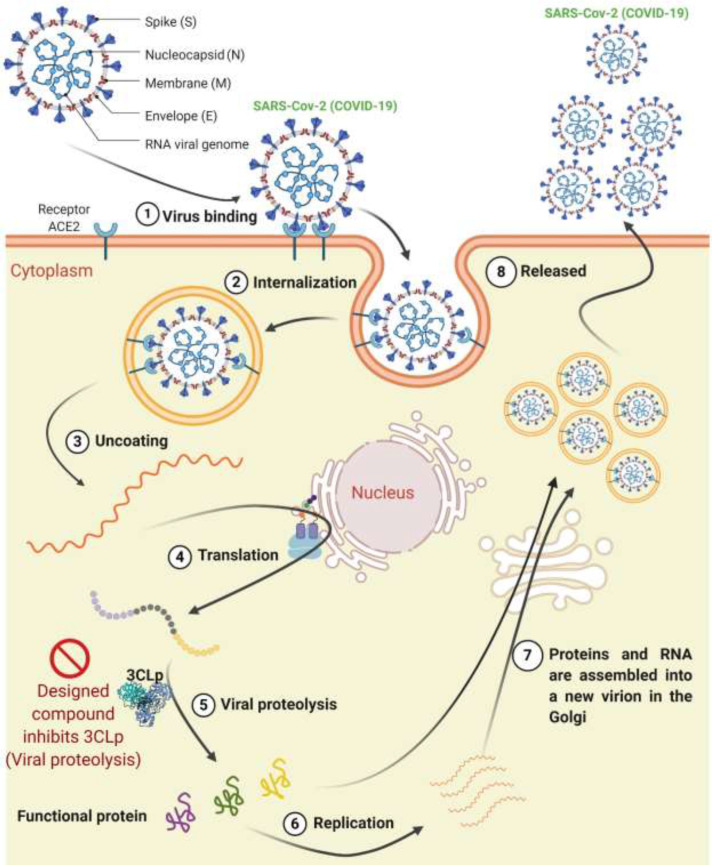
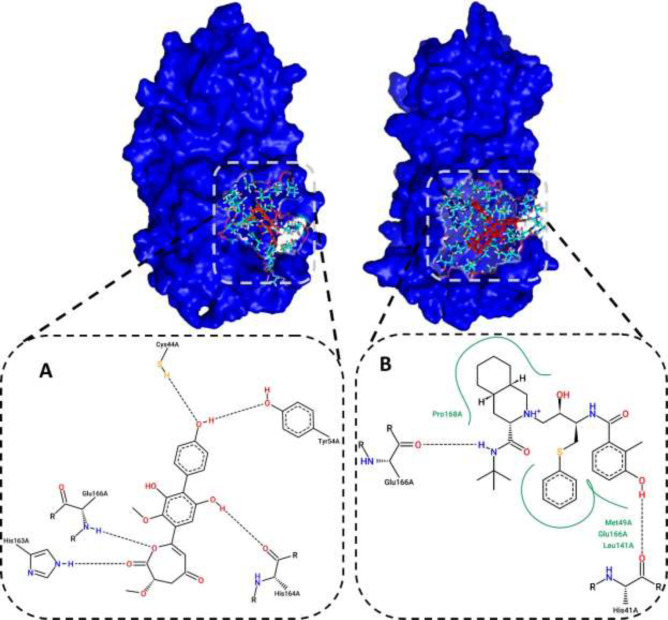
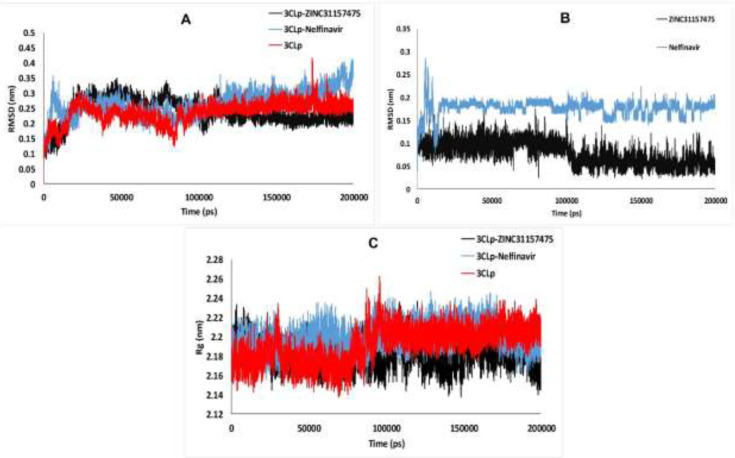
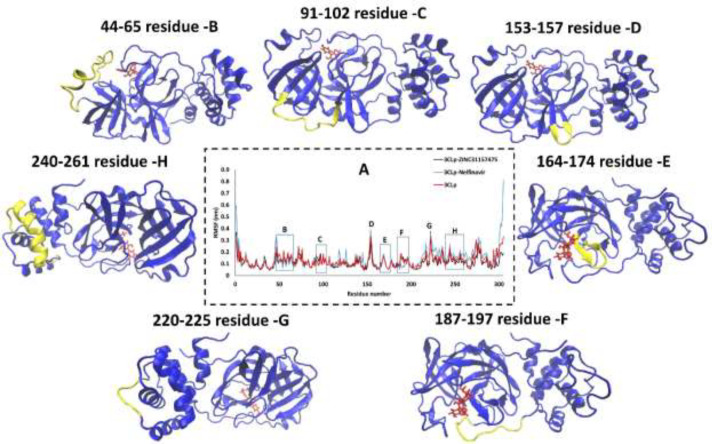
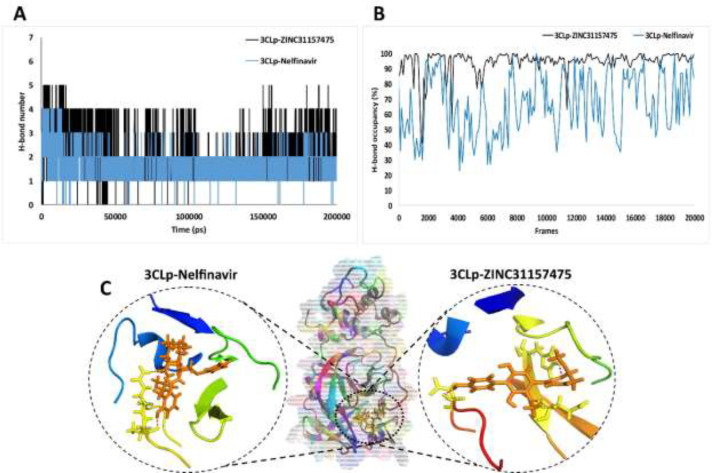
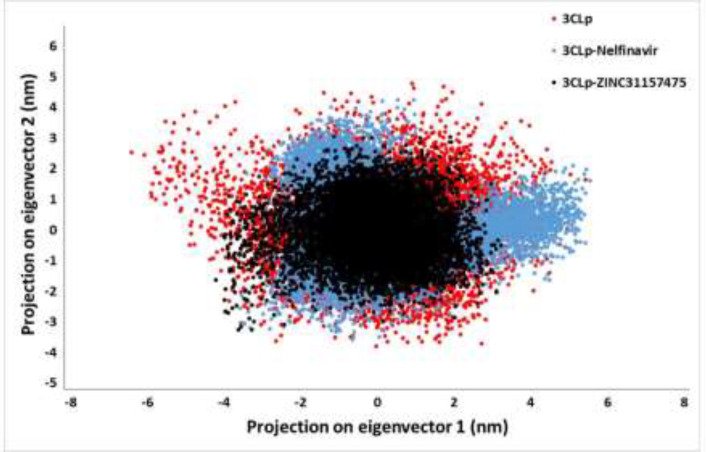
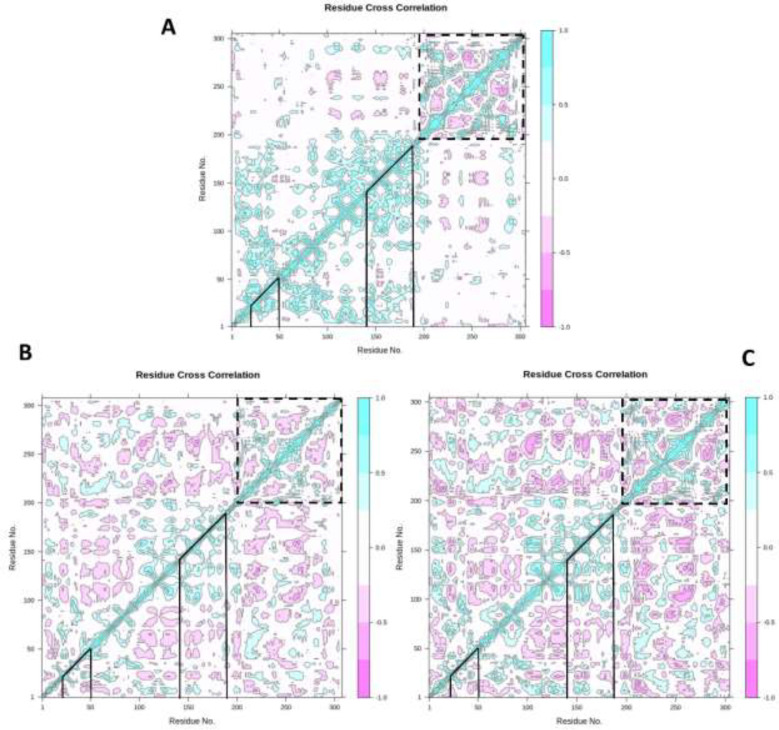
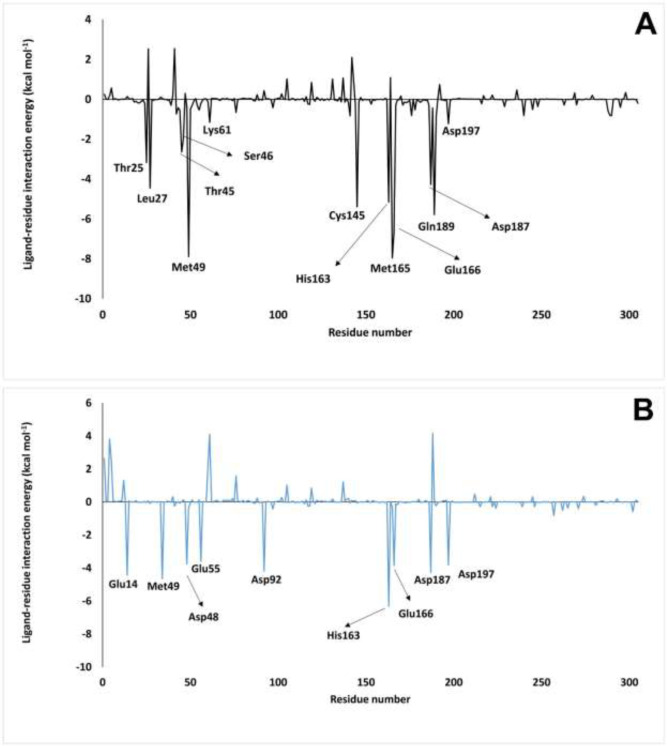
Summary of five top-ranked ligands and some drugs screened against SARS-Cov-2 3CLp with their ZINC ID, 2D structures, docking energy, and interacting residues
SwissADME results, the selected compound showed in bold
Properties | ZINC03915684 | ZINC67910750 | ZINC31157475 | ZINC77269667 | ZINC04096393 | Nelfinavir |
MWa (g mol-1) | 718.61 | 686.65 | 386.35 | 702.70 | 636.47 | 567.78 |
Log S (ESOL) mol L-1 | -6.22 | -1.85 | -3.44 | -4.12 | -3.65 | -6.36 |
Solubility (mg ml-1) | 6.02e-07 | 1.40e-02 | 3.67e-04 | 7.60e-05 | 2.24e-04 | 4.34e-07 |
Heavy atoms | 52 | 48 | 28 | 50 | 45 | 40 |
No. H-bond acceptors | 16 | 17 | 8 | 15 | 18 | 5 |
No. of rotational bonds | 14 | 14 | 4 | 16 | 10 | 12 |
Log Po/w (iLOGP)c | 1.65 | 2.50 | 2.29 | 2.77 | 1.24 | 4.24 |
Lipinski’s rule of five (violation) | 3 | 3 | 0 | 3 | 3 | 1 |
Ghose (violation) | 3 | 4 | 0 | 4 | 2 | 3 |
Veber (violation) | 2 | 2 | 0 | 2 | 1 | 1 |
Egan (violation) | 1 | 1 | 0 | 1 | 1 | 0 |
Muegge (violation) | 4 | 5 | 0 | 5 | 4 | 1 |
Bioavailability score | 0.11 | 0.11 | 0.56 | 0.17 | 0.17 | 0.55 |
Synthetic accessibility | 6.00 | 7.37 | 4.31 | 6.56 | 5.34 | 5.58 |
GId absorption | Low | Low | High | Low | Low | Low |
BBBe permeant | No | No | No | No | No | No |
P-gpf substrate | No | No | Yes | Yes | Yes | Yes |
Log Kp (skin permeation) cm s-1 | -7.86 | -12.08 | -7.36 | -9.78 | -9.93 | -5.74 |
ABS % | 13.07 | 19.09 | 66.73 | 21.67 | 1.82 | 65.11 |
TPSA (Å2) | 278.04 | 260.59 | 122.52 | 253.13 | 310.66 | 127.20 |
The energy component of 3CLp-Nelfinavir and 3CLp-ZINC31157475 complexes was calculated by MM-PBSA
3CLp- ZINC31157475 | 3CLp-Nelfinavir | Energy (kcal mol-1) |
---|---|---|
-177.32 ± 58.11 | -13.28 ± 42.88 | ∆Evdwa |
-61.61 ± 21.36 | -39.19 ± 40.22 | ∆Eelect b |
179.15 ± 52.88 | 38.32 ± 55.66 | ∆Esolv c |
-28.25 ± 6.25 | -5.39 ± 5.38 | ∆ESASA d |
-88.03 ± 29.84 | -19.54 ± 37.80 | ∆Gbinding |
Conclusion
The SARS-Cov-2 has created a pandemic, so it is important to find effective drugs against it. We also conducted this study to design a potent drug against the main protease of this virus. We proposed the ZINC31157475 (natural product) as a SARS-Cov-2 protease (3CLp) inhibitor. The ZINC31157475 binds to the active site of the 3Clp with better docking energy (-10.463 kcal mol-1) than several control drugs includes remdesivir, chloroquine, hydroxyl-chloroquine, maraviroc, nelfinavir, ribavirin, umifenovir, dihydroergotamine, and glecaprevir. The RMSD value in the designed inhibitor complex is lower than the nelfinavir bonded complex. MD data revealed that the Rg is decreased in the presence of ZINC31157475 at the 3CLp active cavity. This decrement can be the result of the increase in H-bonds in the protein after ZINC31157475 binding. The RMSF decreasing has been observed after ZINC31157475 binding in comparison with unbound 3CLp protein and 3CLp-Nelfinavir complex. The atomic motions and internal dynamics were evaluated by PCA and DCCM methods, respectively. Based on the PCA data, the three (especially 3CLp-ZINC31157475) systems have admissible stability. DCCM analysis showed that the ZINC31157475 and nelfinavir binding lead to increment in the anti-correlated motions. Increasing anti-correlated motions generate a stable environment in the active cavity. We defined the residues that have high free binding energy to the ZINC31157475 ligand. Finally, binding free energy calculated by MM-PBSA showed that ZINC31157475 has a high potential for inhibition of the 3CLp activity. However, further laboratory and clinical trials are essential to evaluate the proposed therapeutic application.
Conflicts of interest
The authors declare that they have no known competing financial interests or personal relationships that could have appeared to influence the work reported in this paper.
Authors’ contributions
Concept – Sepideh Shayan.; Design– Shahab Jamaran., Rafee Habib Askandar; Materials – Chiako Farshadfar., Noeman Ardalan.; Data Collection – Chiako Farshadfar., Noeman Ardalan.; Analysis– Sepideh Shayan., Noeman Ardalan., Shahab Jamaran.; PCA and DCCM analysis – Arian Rahimi., Azam Elahi.; Literature Search – Sepideh Shayan.; Writing – Noeman Ardalan., Sepideh Shayan.; Critical Reviews – Rafee Habib Askandar., Arian Rahimi., Noeman Ardalan., Azam Elahi., Arian Rahimi., Sepideh Shayan., Chiako Farshadfar. All authors have approved the final manuscript.
Acknowledgements
References
-
1.
Cao X. COVID-19: immunopathology and its implications for therapy. Nat. Rev. Immunol. 2020;20:269-70. [PubMed ID: 32273594].
-
2.
Jamaati H, Dastan F. Covid-19 in iran: A model for crisis management and current experience. Iran. J. Pharm. Sci. 2020;19:1-8.
-
3.
Cavasotto CN, Di Filippo JI. In silico Drug Repurposing for COVID‐19: Targeting SARS‐CoV‐2 Proteins through Docking and Consensus Ranking. Mol. Inform. 2021;40:2000115.
-
4.
COVID TC, Team R. Severe Outcomes Among Patients with Coronavirus Disease 2019 (COVID-19)-United States, February 12-March 16, 2020. Morb. Mortal. Wkly. Rep. 2020;69:343-6.
-
5.
Zheng YY, Ma YT, Zhang JY, Xie X. COVID-19 and the cardiovascular system. Nat. Rev. Cardiol. 2020;17:259-60. [PubMed ID: 32139904].
-
6.
Kalil AC. Treating COVID-19—off-label drug use, compassionate use, and randomized clinical trials during pandemics. JAMA. 2020;323:1897-8. [PubMed ID: 32208486].
-
7.
Rome BN, Avorn J. Drug evaluation during the Covid-19 pandemic. N Engl J Med. 2020;382:2282-2284. [PubMed ID: 32289216].
-
8.
Jamaati H, Dastan F, Tabarsi P, Marjani M, Saffaei A, Hashemian SM. A Fourteen-day Experience with Coronavirus Disease 2019 (COVID-19) Induced Acute Respiratory Distress Syndrome (ARDS): An Iranian Treatment Protocol. Iran. J. Pharm. Sci. 2020;19:31-6.
-
9.
Dastan F, Tabarsi P, Marjani M, Moniri A, Hashemian SM, Tavakoli-Ardakani M, Saffaei A. Thalidomide against Coronavirus Disease 2019 (COVID-19): A Medicine with a Thousand Faces. Iran. J. Pharm. Sci. 2020;19:1-2.
-
10.
Mohammadi Barzelighi H, Daraei B, Dastan F. Approaches for the Treatment of SARS-CoV-2 Infection: A Pharmacologic View and Literature Review. Iran. J. Pharm. Sci. 2020;19:258-81.
-
11.
ul Qamar MT, Alqahtani SM, Alamri MA, Chen LL. Structural basis of SARS-CoV-2 3CLpro and anti-COVID-19 drug discovery from medicinal plants. J. Pharm. Anal. 2020;10:313-319. [PubMed ID: 32296570].
-
12.
Wu A, Peng Y, Huang B, Ding X, Wang X, Niu P, Meng J, Zhu Z, Zhang Z, Wang J. Genome composition and divergence of the novel Coronavirus (2019-nCoV) originating in China. Cell host & microbe. 2020;27:325-328. [PubMed ID: 32035028].
-
13.
Zhu N, Zhang D, Wang W, Li X, Yang B, Song J, Zhao X, Huang B, Shi W, Lu R. A novel Coronavirus from patients with pneumonia in China, 2019. N Engl J Med. 2020;382:727-733. [PubMed ID: 31978945].
-
14.
Tahir ul Qamar M, Alqahtani SM, Alamri MA, Chen LL. Structural basis of SARS-CoV-2 3CLpro and anti-COVID-19 drug discovery from medicinal plants. J. Pharm. Anal. 2020;10:313-9. [PubMed ID: 32296570].
-
15.
Elmezayen AD, Al-Obaidi A, Şahin AT, Yelekçi K. Drug repurposing for Coronavirus (COVID-19): in silico screening of known drugs against Coronavirus 3CL hydrolase and protease enzymes. J. Biomol. Struct. Dyn. 2020:1-13.
-
16.
Jo S, Kim H, Kim S, Shin DH, Kim MS. Characteristics of flavonoids as potent MERS‐CoV 3C‐like protease inhibitors. Chem Biol Drug Des. 2019;94:2023-30. [PubMed ID: 31436895].
-
17.
Majumder R, Mandal M. Screening of plant-based natural compounds as a potential COVID-19 main protease inhibitor: an in silico docking and molecular dynamics simulation approach. J. Biomol. Struct. Dyn. 2020:1-16.
-
18.
Abu-Farha M, Thanaraj TA, Qaddoumi MG, Hashem A, Abubaker J, Al-Mulla F. The Role of Lipid Metabolism in COVID-19 Virus Infection and as a Drug Target. Int. J. Mol. Sci. 2020;21:3544.
-
19.
Pant S, Singh M, Ravichandiran V, Murty U, Srivastava HK. Peptide-like and small-molecule inhibitors against Covid-19. J. Biomol. Struct. Dyn. 2020:1-10.
-
20.
Kříž K, Řezáč J. Benchmarking of Semiempirical Quantum-Mechanical Methods on Systems Relevant to Computer-Aided Drug Design. J. Chem. Inf. Model. 2020;60:1453-60. [PubMed ID: 32062970].
-
21.
Wang J. Fast identification of possible drug treatment of Coronavirus disease-19 (COVID-19) through computational drug repurposing study. J. Chem. Inf. Model. 2020;60:3277-86. [PubMed ID: 32315171].
-
22.
Yamamoto N, Matsuyama S, Hoshino T, Yamamoto N. Nelfinavir inhibits replication of severe acute respiratory syndrome Coronavirus 2 in-vitro. BioRxiv. 2020.
-
23.
Xu Z, Yao H, Shen J, Wu N, Xu Y, Lu X, Li LJ. Nelfinavir is active against SARS-CoV-2 in Vero E6 cells. ChemRxiv. 2020.
-
24.
Xu Z, Peng C, Shi Y, Zhu Z, Mu K, Wang X, Zhu W. Nelfinavir was predicted to be a potential inhibitor of 2019-nCov main protease by an integrative approach combining homology modelling, molecular docking and binding free energy calculation. BioRxiv. 2020.
-
25.
Pettersen EF, Goddard TD, Huang CC, Couch GS, Greenblatt DM, Meng EC, Ferrin TE. UCSF Chimera—a visualization system for exploratory research and analysis. J. Comput. Chem. 2004;25:1605-12. [PubMed ID: 15264254].
-
26.
Mesecar A. A taxonomically-driven approach to development of potent, broad-spectrum inhibitors of Coronavirus main protease including SARS-CoV-2 (COVID-19). Be Publ. 2020.
-
27.
Gasteiger J, Marsili M. Iterative partial equalization of orbital electronegativity—a rapid access to atomic charges. Tetrahedron. 1980;36:3219-28.
-
28.
Rahimi A, Razmkhah K, Mehrnia M, Mohamadnia A, Sahebjamee H, Salehi S, Asl EA, Tahmasebi H, Shandiz SAS, Davouodbeglou F. Molecular docking and binding study of harpagoside and harpagide as novel anti-inflammatory and anti-analgesic compound from Harpagophytum procumbens based on their interactions with COX-2 enzyme. Asian Pac. J. Trop. Dis. 2016;6:227-31.
-
29.
Van Der Spoel D, Lindahl E, Hess B, Groenhof G, Mark AE, Berendsen HJ. GROMACS: fast, flexible, and free. J. Comput. Chem. 2005;26:1701-18. [PubMed ID: 16211538].
-
30.
Khan F I, Kang T, Ali H, & Lai D. Remdesivir Strongly Binds to RNA-Dependent RNA Polymerase, Membrane Protein and Main Protease of SARS-CoV-2: Indication From Molecular Modeling and Simulations. Front Pharmacol. 2021;12:1616.
-
31.
Silva Arouche Td, Reis AF, Martins AY, S Costa JF, Carvalho Junior RN, JC Neto AM. Interactions Between Remdesivir, Ribavirin, Favipiravir, Galidesivir, Hydroxychloroquine and Chloroquine with Fragment Molecular of the COVID-19 Main Protease with Inhibitor N3 Complex (PDB ID: 6LU7) Using Molecular Docking. J. Nanosci. Nanotechnol. 2020;20:7311-23. [PubMed ID: 32711596].
-
32.
Omar S, Bouziane I, Bouslama Z, Djemel A. In-silico identification of potent inhibitors of COVID-19 main protease (Mpro) and angiotensin converting enzyme 2 (ACE2) from natural products: Quercetin, hispidulin, and cirsimaritin exhibited better potential inhibition than hydroxy-chloroquine against COVID-19 main protease active site and ACE2. ChemRxiv. 2020.
-
33.
Kandeel M, Al-Nazawi M. Virtual screening and repurposing of FDA approved drugs against COVID-19 main protease. Life Sci. 2020:117627. [PubMed ID: 32251634].
-
34.
Choudhary S, Silakari O. Scaffold morphing of arbidol (umifenovir) in search of multi-targeting therapy halting the interaction of SARS-CoV-2 with ACE2 and other proteases involved in COVID-19. Virus Res. 2020:198146. [PubMed ID: 32866534].
-
35.
Gurung AB, Ali MA, Lee J, Farah MA, Al-Anazi KM. In-silico screening of FDA approved drugs reveals ergotamine and dihydroergotamine as potential Coronavirus main protease enzyme inhibitors. Saudi J. Biol. Sci. 2020;27:2674-2682. [PubMed ID: 32837219].
-
36.
Shamsi A, Mohammad T, Anwar S, AlAjmi MF, Hussain A, Rehman M, Islam A, Hassan M. Glecaprevir and Maraviroc are high-affinity inhibitors of SARS-CoV-2 main protease: possible implication in COVID-19 therapy. Biosci. Rep. 2020;40:BSR20201256. [PubMed ID: 32441299].
-
37.
Trott O, Olson AJ. AutoDock Vina: improving the speed and accuracy of docking with a new scoring function, efficient optimization, and multithreading. J. Comput. Chem. 2010;31:455-61. [PubMed ID: 19499576].
-
38.
Sanner MF. Python: a programming language for software integration and development. J Mol Graph Model. 1999;17:57-61. [PubMed ID: 10660911].
-
39.
Schöning-Stierand K, Diedrich K, Fährrolfes R, Flachsenberg F, Meyder A, Nittinger E, Steinegger R, Rarey M. ProteinsPlus: interactive analysis of protein–ligand binding interfaces. Nucleic Acids Res. 2020;48:W48-W53. [PubMed ID: 32297936].
-
40.
Lin J, Sahakian DC, De Morais S, Xu JJ, Polzer RJ, Winter SM. The role of absorption, distribution, metabolism, excretion and toxicity in drug discovery. Curr. Top. Med. Chem. 2003;3:1125-54. [PubMed ID: 12769713].
-
41.
Noorbakhsh A, Habib Askandar R, Alhagh MS, Farshadfar C, Seyedi SH, Ahmadizad M, Rahimi A, Ardalan N, Hosseininezhadian koushki E. Prevention of SARS-CoV-2 proliferation with a novel and potent main protease inhibitor by docking, ADMET, MM-PBSA, and molecular dynamics simulation. J. Theor. Comput. Chem. 2021;20:305-322.
-
42.
Daina A, Michielin O, Zoete V. SwissADME: a free web tool to evaluate pharmacokinetics, drug-likeness and medicinal chemistry friendliness of small molecules. Sci. Rep. 2017;7:42717. [PubMed ID: 28256516].
-
43.
Kumar D, Kumari K, Jayaraj A, Kumar V, Kumar RV, Dass SK, Chandra R, Singh P. Understanding the binding affinity of noscapines with protease of SARS-CoV-2 for COVID-19 using MD simulations at different temperatures. J. Biomol. Struct. Dyn. 2020:1-14.
-
44.
Alimi Livani Z, Safakish M, Hajimahdi Z, Soleymani S, Zabihollahi R, Aghasadeghi MR, Alipour E, Zarghi A. Design, Synthesis, Molecular Modeling, In Silico ADME Studies and Anti-HIV-1 Assay of New Diazocoumarin Derivatives. Iran. J. Pharm. Sci. 2018;17:65-77.
-
45.
Sousa da Silva AW, Vranken WF. ACPYPE - AnteChamber PYthon Parser interfacE. BMC Res. Notes. 2012;5:367. [PubMed ID: 22824207].
-
46.
Enríquez‐Mendiola D, Téllez‐Valencia A, Sierra‐Campos E, Campos‐Almazán M, Valdez‐Solana M, Gómez Palacio‐Gastélum M, Avitia‐Domínguez C. Kinetic and molecular dynamic studies of inhibitors of shikimate dehydrogenase from methicillin‐resistant Staphylococcus aureus. Chem Biol Drug Des. 2019;94:1504-17. [PubMed ID: 31009175].
-
47.
Darden T, York D, Pedersen L. Particle mesh Ewald: An N⋅ log (N) method for Ewald sums in large systems. J. Chem. Phys. 1993;98:10089-92.
-
48.
Essmann U, Perera L, Berkowitz ML, Darden T, Lee H, Pedersen LG. A smooth particle mesh Ewald method. J. Chem. Phys. 1995;103:8577-93.
-
49.
Bussi G, Donadio D, Parrinello M. Canonical sampling through velocity rescaling. J. Chem. Phys. 2007;126:14101. [PubMed ID: 17212484].
-
50.
Parrinello M, Rahman A. Polymorphic transitions in single crystals: A new molecular dynamics method. J. Appl. Phys. 1981;52:7182-90.
-
51.
Enayatkhani M, Hasaniazad M, Faezi S, Guklani H, Davoodian P, Ahmadi N, Einakian MA, Karmostaji A, Ahmadi K. Reverse vaccinology approach to design a novel multi-epitope vaccine candidate against COVID-19: an in silico study. J. Biomol. Struct. Dyn. 2020:1-16.
-
52.
Farshadfar C, Mollica A, Rafii F, Noorbakhsh A, Nikzad M, Seyedi SH, Abdi F, Verki SA, Mirzaie S. Novel potential inhibitor discovery against tyrosyl-tRNA synthetase from Staphylococcus aureus by virtual screening, molecular dynamics, MMPBSA and QMMM simulations. Mol Simul. 2020;46:507-20.
-
53.
Humphrey W, Dalke A, Schulten K. VMD: visual molecular dynamics. J. Mol. Graph. 1996;14:33-8. [PubMed ID: 8744570].
-
54.
Lopreiato M, Di Cristofano S, Cocchiola R, Mariano A, Guerrizio L, Scandurra R, Mosca L, Raimondo D, Scotto d’Abusco A. Biochemical and Computational Studies of the Interaction between a Glucosamine Derivative, NAPA and the IKKα Kinase. Int J Mol Sci. 2021;22:1643.
-
55.
Priya Doss CG, Chakraborty C, Chen L, Zhu H. Integrating in silico prediction methods, molecular docking, and molecular dynamics simulation to predict the impact of ALK missense mutations in structural perspective. Biomed Res. Int. 2014.
-
56.
Ardalan N, Sepahi AA, Khavari-Nejad RA. Development of Escherichia coli asparaginase II for the treatment of acute lymphocytic leukemia: In silico reduction of asparaginase II side effects by a novel mutant (V27F). Asian Pac. J. Cancer Prev. 2021;22:1137-1147. [PubMed ID: 33906306].
-
57.
Nemaysh V, Luthra PM. Computational analysis revealing that K634 and T681 mutations modulate the 3D-structure of PDGFR-β and lead to sunitinib resistance. RSC Adv. 2017;7:37612-26.
-
58.
Ndagi U, Abdullahi M, Hamza AN, Soliman ME. An analogue of a kinase inhibitor exhibits subjective characteristics that contribute to its inhibitory activities as a potential anti-cancer candidate: insights through computational biomolecular modelling of UM-164 binding with lyn protein. RSC Adv. 2020;10:145-61.
-
59.
Yesudhas D, Anwar MA, Panneerselvam S, Durai P, Shah M, Choi S. Structural mechanism behind distinct efficiency of Oct4/Sox2 proteins in differentially spaced DNA complexes. PLoS One. 2016;11:e0147240. [PubMed ID: 26790000].
-
60.
Grant BJ, Rodrigues AP, ElSawy KM, McCammon JA, Caves LS. Bio3d: an R package for the comparative analysis of protein structures. Bioinformatics. 2006;22:2695-6. [PubMed ID: 16940322].
-
61.
Kumari R, Kumar R, Consortium OSDD, Lynn A. g_mmpbsa A GROMACS tool for high-throughput MM-PBSA calculations. J. Chem. Inf. Model. 2014;54:1951-62. [PubMed ID: 24850022].
-
62.
Noorbakhsh A, Hosseininezhadian Koushki E, Farshadfar C, Ardalan N. Designing a natural inhibitor against human kynurenine aminotransferase type II and a comparison with PF-04859989: a computational effort against schizophrenia. J. Biomol. Struct. Dyn. 2021:1-14.
-
63.
Kumari R, Kumar R, Lynn A. g_mmpbsa A GROMACS Tool for High-Throughput MM-PBSA Calculations. J. Chem. Inf. Model. 2014;54:1951-62. [PubMed ID: 24850022].
-
64.
Mollica A, Mirzaie S, Costante R, Carradori S, Macedonio G, Stefanucci A, Dvoracsko S, Novellino E. Exploring the biological consequences of conformational changes in aspartame models containing constrained analogues of phenylalanine. J Enzyme Inhib Med Chem. 2016;31:953-63. [PubMed ID: 26308194].
-
65.
Gills JJ, LoPiccolo J, Tsurutani J, Shoemaker RH, Best CJ, Abu-Asab MS, Borojerdi J, Warfel NA, Gardner ER, Danish M. Nelfinavir, A lead HIV protease inhibitor, is a broad-spectrum, anticancer agent that induces endoplasmic reticulum stress, autophagy, and apoptosis in-vitro and in-vivo. Clin. Cancer Res. 2007;13:5183-94. [PubMed ID: 17785575].
-
66.
Gordon CJ, Tchesnokov EP, Feng JY, Porter DP, Götte M. The antiviral compound remdesivir potently inhibits RNA-dependent RNA polymerase from Middle East respiratory syndrome Coronavirus. Int. J. Biol. Chem. 2020;295:4773-9.
-
67.
Ghose AK, Viswanadhan VN, Wendoloski JJ. A knowledge-based approach in designing combinatorial or medicinal chemistry libraries for drug discovery A qualitative and quantitative characterization of known drug databases. J. Comb. Chem. 1999;1:55-68. [PubMed ID: 10746014].
-
68.
Veber DF, Johnson SR, Cheng H-Y, Smith BR, Ward KW, Kopple KD. Molecular properties that influence the oral bioavailability of drug candidates. J. Med. Chem. 2002;45:2615-23. [PubMed ID: 12036371].
-
69.
Egan WJ, Merz KM, Baldwin JJ. Prediction of drug absorption using multivariate statistics. J. Med. Chem. 2000;43:3867-77. [PubMed ID: 11052792].
-
70.
Muegge I, Heald SL, Brittelli D. Simple selection criteria for drug-like chemical matter. J. Med. Chem. 2001;44:1841-6. [PubMed ID: 11384230].
-
71.
Mittal L, Kumari A, Srivastava M, Singh M, Asthana S. Identification of potential molecules against COVID-19 main protease through structure-guided virtual screening approach. J. Biomol. Struct. Dyn. 2020:1-26.
-
72.
Ndombera FT. Revisiting Cheminformatics and Mechanisms of Action of Chloroquine and Hy-droxychloroquine in Targeting Covid-19. J. Bioinform. Comp. Genome. 2020;3:1-11.
-
73.
Cardoso MH, Orozco RQ, Rezende SB, Rodrigues G, Oshiro KG, Cândido ES, Franco OL. Computer-aided design of antimicrobial peptides: are we generating effective drug candidates? Front. Microbiol. 2020;10:3097.
-
74.
Schiebel J, Gaspari R, Wulsdorf T, Ngo K, Sohn C, Schrader TE, Cavalli A, Ostermann A, Heine A, Klebe G. Intriguing role of water in protein-ligand binding studied by neutron crystallography on trypsin complexes. Nat. Commun. 2018;9:1-15. [PubMed ID: 29317637].
-
75.
Sharma T, Harioudh MK, Kuldeep J, Kumar S, Banerjee D, Ghosh JK, Siddiqi MI. Identification of Potential Inhibitors of Cathepsin-B using Shape & Pharmacophore-based Virtual Screening, Molecular Docking and Explicit Water Thermodynamics. Mol. Inform. 2020;39:1900023.
-
76.
Khan SA, Zia K, Ashraf S, Uddin R, Ul-Haq Z. Identification of chymotrypsin-like protease inhibitors of SARS-CoV-2 via integrated computational approach. J. Biomol. Struct. Dyn. 2020:1-10.
-
77.
Ardalan N, Mirzaie S, Sepahi AA, Khavari-Nejad RA. Novel mutant of Escherichia coli asparaginase II to reduction of the glutaminase activity in treatment of acute lymphocytic leukemia by molecular dynamics simulations and QM-MM studies. Med. Hypotheses. 2018;112:7-17. [PubMed ID: 29447943].
-
78.
Kumar K, Anbarasu A, Ramaiah S. Molecular docking and molecular dynamics studies on β-lactamases and penicillin binding proteins. Mol. Biosyst. 2014;10:891-900. [PubMed ID: 24503740].
-
79.
Suárez D, Díaz N. SARS-CoV-2 Main Protease: A Molecular Dynamics Study. J. Chem. Inf. Model. 2020;60:5815-31. [PubMed ID: 32678588].
-
80.
Sharma P, Vijayan V, Pant P, Sharma M, Vikram N, Kaur P, Singh T, Sharma S. Identification of potential drug candidates to combat COVID-19: a structural study using the main protease (mpro) of SARS-CoV-2. J. Biomol. Struct. Dyn. 2020:1-11.
-
81.
Kneller DW, Phillips G, O’Neill HM, Jedrzejczak R, Stols L, Langan P, Joachimiak A, Coates L, Kovalevsky A. Structural plasticity of SARS-CoV-2 3CL Mpro active site cavity revealed by room temperature X-ray crystallography. Nat. Commun. 2020;11:3202. [PubMed ID: 32581217].
-
82.
Wang L, Hu W, Fan C. Structural and biochemical characterization of SADS‐CoV papain‐like protease 2. Protein Sci. 2020;29:1228-41. [PubMed ID: 32216114].
-
83.
Yu H, Yan Y, Zhang C, Dalby PA. Two strategies to engineer flexible loops for improved enzyme thermostability. Sci. Rep. 2017;7:41212. [PubMed ID: 28145457].
-
84.
Sk MF, Roy R, Kar P. Exploring the potency of currently used drugs against HIV-1 protease of subtype D variant by using multiscale simulations. J. Biomol. Struct. Dyn. 2020:1-16.
-
85.
Kumar A, Choudhir G, Shukla SK, Sharma M, Tyagi P, Bhushan A, Rathore M. Identification of phytochemical inhibitors against main protease of COVID-19 using molecular modeling approaches. J. Biomol. Struct. Dyn. 2020:1-11.
-
86.
Mahita J, Sowdhamini R. Integrative modelling of TIR domain-containing adaptor molecule inducing interferon-β (TRIF) provides insights into its autoinhibited state. Biol. Direct. 2017;12:1-15. [PubMed ID: 28095902].
-
87.
Amir M, Mohammad T, Kumar V, Alajmi MF, Rehman MT, Hussain A, Alam P, Dohare R, Islam A, Ahmad F, Hassan MI. Structural Analysis and Conformational Dynamics of STN1 Gene Mutations Involved in Coat Plus Syndrome. Front. Mol. Biosci. 2019;6:41. [PubMed ID: 31245382].