Abstract
Background:
Since the introduction of data envelopment analysis (DEA) in 1978, this method has become one of the best performance evaluation tools. Use of DEA has many advantages, as it can accommodate multiple inputs and outputs. However, there are some constraints concerning the number of variables. For instance, selective variables are commonly used to evaluate the performance of hospitals, based on the researcher’s personal preferences.Objectives:
In application of traditional DEA models in hospital comparisons, variables are always selected based on the analyst’s opinion regarding the significance or availability of data. On the other hand, there is a need to reduce the dimensions of variables. The main goal of this study was to reduce the number of input and output variables in the evaluation of military hospitals of Iran.Methods:
In this study, the Delphi technique was applied, as well as partial least squares - structural equation modeling (PLS - SEM) method.Results:
Acceptable goodness of fit was established for validity of the measurement model. The test of validity was accepted for the structural model in this study.Conclusions:
In the first step, the number of variables was reduced to 29, using the Delphi technique. In the second step, using SEM - PLS, the number of variables was reduced to half.Keywords
Delphi Technique Structural Equation Modeling Data Envelopment Analysis (DEA)
1. Background
Efficiency is a well - established concept to measure and evaluate the handling of a scarce resource. Efficiency, originally developed for economic analysis, is defined as the relationship between the input and output. High efficiency is achieved when a given output is obtained with minimum input, or maximum output is produced for a given input (1). Farrell (1957) was the first to link production functions to the measurement of technical efficiency (2, 3).
One of the most important management challenges of this century is improvement of the efficiency of healthcare (4, 5). It is generally difficult for hospitals to increase the number of beds or staffing (6). Therefore, they need to become more efficient in order to reduce costs and improve efficiency in the treatment of patients (7). Two of the most common and modern efficiency measurement techniques in healthcare are stochastic frontier analysis (SFA) and data envelopment analysis (DEA). Overall, as pointed out by Hollingsworth, the techniques used in efficiency studies of healthcare are mainly based on DEA (8).
DEA was first proposed by Charnes et al. to match the relative efficiency of peer decision - making units (DMUs) (9). Hospitals are an example of DMUs. Nunamaker and Sherman are the leading researchers performing DEA studies in healthcare. DEA was immediately recognized as a modern tool for performance measurement (10). Because of increasing cost pressure, policymakers of the military hospital sector in Iran have decided to evaluate the performance of hospitals using DEA. A valid question in the evaluation of efficiency in hospitals (health sector) is what inputs and outputs should be used to represent the production process. A large number of operational variables have been used in both categories (11). In addition, some studies have examined the impact of changing input and output specifications in hospital production efficiency models.
Magnussen measured the production efficiency of 46 Norwegian hospitals (observations in three years) using labor and capital inputs by specifying various output vectors. In addition, Wagner and Shimshak (12) asserted that “the challenge of DEA is to find a parsimonious model, using as many input and output variables as needed, but as few as possible”. A challenge in determining the appropriate input variables is that any resource that is consumed to produce a result can be considered a valid input (13).
O’Neill et al. (14) introduced the taxonomy of hospital efficiency studies, which used DEA and related techniques, and provided a summary of input and output variables in the evaluation of hospitals during 1984 - 2004. However, if there are too many variables in the comparison of hospitals, the discrimination will be low and most hospitals will be regarded as efficient; therefore, there is a need to reduce the dimensions of variables (15). In this regard, Zhang (2016) (15) reduced the number of variables in the assessment of hospital performance, using principal component analysis (PCA) and efficiency contribution measure (ECM).
DEA provides many opportunities, including collaboration opportunities for analysts and decision - makers (16). On the other hand, research in the field of management has shown that Delphi method is appropriate for promoting the contribution of researchers and practitioners to develop an understanding of multifaceted phenomena and to bridge the gap. Dalkey and colleagues at Rand Corporation originally developed the Delphi technique in the 1950’s and named it after an ancient Greek temple, where the oracles could be found (17). The Delphi method requires knowledgeable and expert contributors to individually respond to questions and submit the results to a central coordinator.
The coordinator processes the contributions, searching for central and extreme tendencies and their rationales. The results are then fed back to the respondents. Following that, the respondents are asked to resubmit their views, assisted by the input provided by the coordinator. This process continues until the coordinator sees that a consensus is reached. This technique aims to remove any possible bias when diverse groups of experts meet together. Also, in this technique, experts do not know who the other experts are during the process.
This research applies a new technique to reduce the number of variables in performance evaluation of hospitals. We used the structural equation modeling - partial least squares (SEM - PLS) to decrease the number of input and output variables. SEM - PLS was initially developed by Wold (1974, 1980, and 1982). Generally, PLS is an SEM technique based on an iterative approach, which maximizes the explained variance of endogenous constructs (18, 19). Multivariate techniques are mainly applied to expand the researchers’ explanatory ability and statistical efficiency.
The first - generation analytical techniques share a common shortcoming, i.e., each technique can examine only a single relationship at a time. SEM, an extension of several multivariate techniques, is commonly used today to examine a series of dependence relationships simultaneously. SEM is used to specify, estimate, and evaluate modes of linear models among a set of observable variables with respect to an often smaller number of unobserved variables; SEM may be applied to develop or test a theory (20).
SEM represents the hybrid of two separate statistical traditions: (a) factor analysis developed in psychology and psychometrics; and (b) simultaneous equation modeling developed mainly in econometrics. SEM allows for the evaluation of relationships among latent variables by combining the strengths of factor analysis and multiple regression analysis in a single model, which can be tested statistically. Variables can be treated as both independent and dependent in SEM. More importantly, SEM facilitates the estimation of latent variables rather than only observable variables and thereby eliminates random error. In addition, it has the advantage of yielding indices of overall fit for hypothesized models (21).
By application of SEM, we can use a reduced set of components to summarize the observed associations (18). Wold, the originator of the method, characterizes PLS - SEM as the “epoch - making innovation of the 1960’s”, which combines econometric prediction with psychometric modeling of latent variables (also referred to as constructs), determined by multiple indicators (also referred to as manifest variables) (22). In this regard, Iacobucci (23) presented an article, entitled “Everything you always wanted to know about SEM”, which fully describes assessment models in SEM - PLS.
Selection of suitable inputs and outputs is crucial for a meaningful analysis (24). Considering the power of SEM - PLS in analyzing multivariate models, flexibility of DEA, and properties of Delphi technique, this study aimed to employ these methods to select the most important variables in Iranian military hospitals.
2. Methods
2.1. Delphi Technique
The study methodology consisted of a Delphi survey using different items, which were selected after two rounds. According to Green et al. (1999), two or three rounds are preferred in the Delphi technique (25). Linstone and Turoff (26) have published an E - book on the Delphi technique, which was found to be a suitable reference for conducting the survey. We conducted the research in two parts. In the first part, using the Delphi technique, we selected the most important variables for the assessment of hospital performance. In the second part, to reduce the items, localize the research, and study the viewpoints of managers and specialists in military hospitals, variables selected in the first part were presented to the managers and experts of the selected hospitals, using a questionnaire with a five-point Likert scale.
2.2. First Section
In the first section, we used the Delphi method. We selected panel members (15 experts) with 10 years of experience in hospital management or faculty members of the university, who were knowledgeable in the field; their commitment to answering multiple rounds of questions on the same topic was essential. In the first round, a list of input and output variables used in previous research was presented to the experts. They were asked to choose the most important variables; if a new variable was to be proposed, the experts had to announce it. After collecting the experts’ opinions in the first round, the selected and newly added variables were presented to the specialists in the second round.
After receiving the experts’ feedback in the second round, we analyzed the data and selected items with consensus over 70%. In this regard, McKenna found that most statements reached consensus over 70% (27); accordingly, 29 variables were selected (Table 2). In this study, we aimed to use the specialists’ assistance in military hospitals to select the most crucial input and output variables for performance evaluation of military hospitals.
2.3. Sampling and Data Collection
In cluster sampling, a cluster refers to a group of population elements, constituting the sampling unit instead of a single element of the population (28). In the current study, the military organization was considered as a cluster with 27 hospitals. We selected a sample of hospitals (20 randomly selected hospitals) and recruited a sample of managers from these hospitals. The questionnaire was presented to 80 managers of military hospitals in Iran. The total number of usable questionnaires was 56.
Twenty items of the questionnaire were dedicated to measuring the latent variable one (input variable), and nine indicators were dedicated to measuring the latent variable two (output variable) in the measurement model. Responses to the questionnaire were used as the raw input data to estimate the construct scores as part of solving the PLS - SEM algorithm. A data matrix was prepared with the raw data by manually capturing the questionnaire responses in an Excel sheet. The questionnaire consisted of two parts. Part one consisted of demographic characteristics (Table 1).
The Demographic Characteristics of the Participants
Parameters | Percentage |
---|---|
Gender | |
Male | 89.7 |
Female | 6.9 |
Age (years) | |
31 - 41 | 1.7 |
41 - 50 | 24.1 |
≥ 51 | 70.7 |
Educational level | |
Professional degree | 74.1 |
Doctorate degree | 22.4 |
Missing systems | 3.4 |
In part two, specialists were asked to rank their insights about the importance of items in performance evaluation of hospitals using two latent variables, namely input and output variables, on a five - point Likert scale (1, not important; 2, slightly important; 3, moderately important; 4, important; 5, very important).
The Input and Output Variables Based on the Literature
Construct (latent variables) | Observable Variables (indicators) |
---|---|
Input variables (latent variable one) q1- q20 (q1, number of beds) | 1) Number of beds; 2) number of acute care beds; 3) number of ICU beds; 4) number of long - term care beds; 5) number of physicians; 6) number of surgeons; 7) number of specialists; 8) number of general practitioners; 9) number of part - time physicians; 10) number of physicians in laboratory examinations; 11) number of residence staff; 12) number of interns; 13) number of service providers (e.g., psychologists); 14) number of professional nurses; 15) number of nonprofessional nurses; 16) total cost of equipment; 17) total cost of maintenance, equipment, vehicles, and buildings; 18) number of non-physician staff; 19) number of supporting staff; 20) number of technical and technological staff |
Output variables (latent variable two) q21- q29 | 21) Number of outpatient visits; 22) number of outpatient visits plus emergency visits; 23) number of inpatients; 24) number of outpatients; 25) number of discharges; 26) number of admissions; 27) number of laboratory examinations; 28) number of inpatient surgeries; 29) number of surgeries |
2.4. Second Section: SEM - PLS
The argument for SEM - PLS as a viable methodology is gaining acceptance in many business disciplines. Overall, studies have indicated a substantial increase in the use of SEM - PLS in recent years (18). SEM - PLS is not generally a statistical technique. SEM integrates many different multivariate techniques into one model - fitting framework. It is an integration of measurement theory, factor analysis (latent variable), path analysis, regression analysis, and simultaneous equation models, which are all different techniques coming together to present SEM as the general modeling environment (29).
The SEM technique is a natural extension to factor analysis and regression. The measurement SEM is essentially a confirmatory factor analysis. The structural part of the model is similar to regression, while it is vastly more flexible in theoretical models (23). The most prominent reasons for using PLS - SEM include non-normal distribution of data, small sample size, and formative measurement constructs (18).
PLS - SEM is optimal for estimating composite models and simultaneously allows approximation of common factor models involving effect indicators (22). The goal of PLS - SEM is to generate latent variable scores, which jointly minimize the residuals of ordinary least squares (OLS) regressions in the model (i.e., maximize explanation) (22). SEM models are comprised of a measurement model, which relates variables to constructs, and a structural path model, which connects the constructs to each other (23). In this study, we started with the measurement model:
2.4.1. Measurement Model
In the SEM - PLS approach, it is a convention to depict latent variables in ovals and observable variables in rectangles. In the measurement model (also called the “outer model”), a block of directly observable indicators represents each latent variable, which is not directly observable. At this stage, each latent construct to be included in the model is identified, and the measured indicator variables are assigned to the latent construct. Although the equation can represent this identification and assignment, it is simple to describe the process with a diagram. Overall, there are three types of relationships: 1) measurement relationships between indicators/items and construct; 2) correlations among constructs; and 3) error terms for the items (20).
2.4.1.1. Structural Model: Path Analysis
In the structural model, also called the “inner model”, latent variables have predefined and theoretically/conceptually established relationships (22). This stage is critical to the development of an SEM model. It involves specifying the structural model by assigning relationships from one construct to another, based on the proposed theoretical model. The structural model specifications focus on adding single - headed directional arrows to represent the structural hypothesis in the research model (20). The relationships represent the structural model, which connects the input and output layers. This step requires the researcher to make several decisions, such as specifying the outer model in the reflective or formative manner (18, 30).
2.4.1.1.1. Reflective or Formative Constructs
The basic difference between reflective and formative constructs is that formative measures represent instances in which indicators produce the construct (i.e., arrows point from the indicators to the construct), whereas the construct creates reflective indicators (i.e., arrows point from the construct to indicators) (30). As a result, reflective indicators are interchangeable, highly correlated, and removable without changing the meaning of the construct (18). Muzamil (31) suggested an approach to distinguish reflective constructs from formative constructs. Our model is a reflective measurement model, and we focused on the evaluation of the reflective measurement model.
2.4.2. Full SEM Model: A Combined Model
The full SEM model comprised of a measurement model, which related the variables to the construct, as well as a structural model, which connected the constructs to other constructs (23).
2.4.3. Assessment Reliability
The first step is to use composite reliability to evaluate the internal consistency reliability of construct measures. Overall, composite reliability presents a more appropriate measure of internal consistency reliability (18).
2.4.4. Construct Validity
Campbell and Fiske (1959) proposed two approaches to assess the construct validity of a test: 1) convergent validity, degree of confidence that indicators properly measure a trait; and 2) discriminant validity, degree to which measures of different traits are unrelated (32).
2.4.4.1. Assessment of Convergent Validity
PLS assesses the measurement model by generating factor loadings for each indicator, which can be interpreted similar to the results produced by PCA (33). Support is provided for convergent validity when each indicator has outer loadings above 0.70, and when the average variance extracted (AVE) of each construct is 0.5 or higher.
2.4.4.2. Assessment of Discriminant Validity
Discriminant validity represents the extent to which a construct is empirically distinct from another construct; in other words, the construct measures what it is intended to measure. The heterotrait - monotrait (HTMT) ratio of correlation is a new criterion for assessing discriminant validity in PLS - SEM models (34).
2.4.4.2.1. Bootstrapping
In a nutshell, bootstrapping is a nonparametric resampling procedure, which assesses statistical variability by examining the variability of sample data rather than using parametric assumptions to evaluate the precision of estimates (19).
2.5. SRMR
Standardized root mean square residual (SRMR) is the square roots of difference between residuals of the sample covariance matrix and the hypothesized covariance model; values for SRMR range from 0 to 1.0 (< 0.08 for acceptable fitting models) (35, 36).
3. Results
3.1. Sample Size
One of the most prominent reasons for using PLS - SEM is small sample size (18). Accordingly, when other methods fail, PLS can be applied in many small sample sizes (36). A popular heuristic approach states that the minimum sample size for a PLS model should be 10 times larger than the largest number of inner model paths directed at a particular construct in the inner model (37). We had two constructs in this model (10 × 1 = 10), and the sample size was estimated at 56 (56 > 10); therefore, the sample size was sufficient according to the mentioned criterion.
3.1.1. Assessment Criteria
Table 3 presents various reliability and validity items, which need to be assessed and reported when applying a PLS - SEM approach.
The Criteria for Reliability and Validity Assessments
Assessment Criteria | |
---|---|
Reliability | |
Indicator reliability (Cronbach’s α) | preferred ≥ 0.70 (see assessment reliability section) |
Composite reliability | preferred ≥ 0.7 (see assessment reliability section) |
Validity | |
Convergent validity (AVE) | Each AVE ≥ 0.50 |
Discriminant validity | HTMTinference < 1 (see HTMT section) |
SRMR | SRMR < 0.08 |
P value and T value | A common nonparametric method with growing popularity at a hurdle rate of P < 0.05 to indicate the significance of path coefficients (Sosik, Kahai, & Piovoso, 2009, p. 19) (significant T values, 1.65 for 10%; 1.96 for 5%; and 2.58 for 1%; all two - tailed) |
3.2. Analysis
Data were analyzed using SmartPLS 3.0. Regarding the path diagram notation, the analysis started with a review of mean values, standard deviations, Cronbach’s α, and correlations among variables, followed by an assessment of measurement and structural models.
3.3. First Stage
3.3.1. Assessment of Reliability and Convergent Validity
The results of Cronbach’s α and composite reliability test show that these values are acceptable in this research (Table 4) (see criteria in Table 3).
Construct Reliability and Validity
Cronbach’s α | Composite Reliability | |
---|---|---|
Input variables | 0.9328 | 0.9404 |
Output variables | 0.8911 | 0.914 |
PLS assesses structural components by generating estimates of standardized regression coefficients for the structural paths in the model. The statistical significance of these path coefficients was evaluated using bootstrapping (38), which is a common nonparametric method with growing popularity, as well as a hurdle rate of P ≤ 0.05 to indicate the significance of path coefficients (33). Moreover, PLS assesses the measurement model by generating standardized loading factors for each indicator, which are interpretably similar to the results produced by PCA analysis (33). An example presented in Figure 1 shows an outer loading of 0.741 (> 0.70) for q1, but an outer loading of 0.594 (< 0.70) for q9. We selected indicators with outer loadings greater than 0.70 (q9 was deleted at this stage).
The Loading Factors of Variables in the First Phase (q, question)
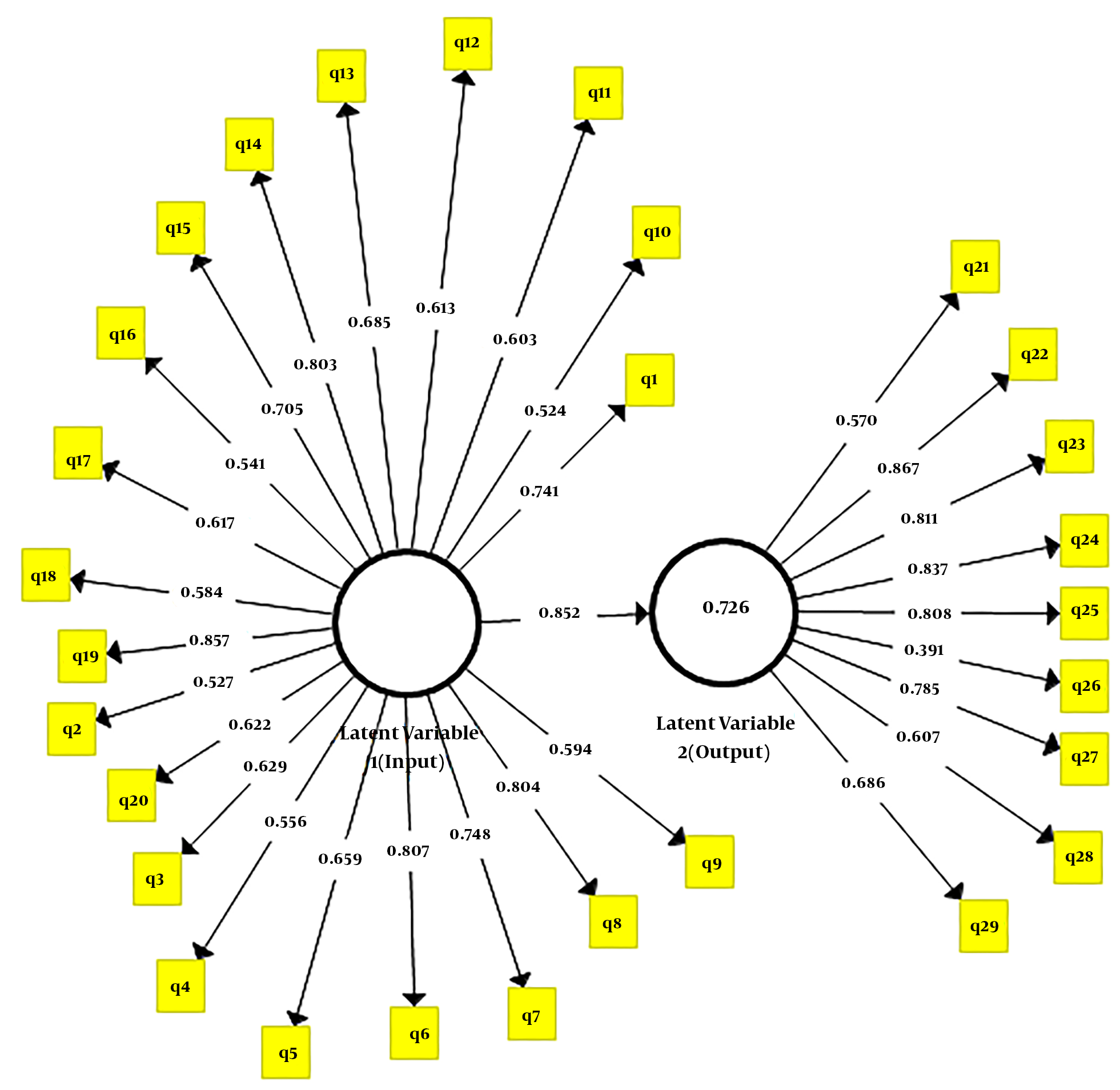
We eliminated variables with outer loadings below 0.70. Therefore, indicators of q2 - q3 - q4 - q5 - q9 - q10 - q11 - q12 - q13 - q16 - q17 - q18 - q20 input variables were removed. Also, indicators of q21 - q26 - q28 output variables were reduced. The measurement errors for undeleted items are presented in Table 5.
The Measurement Errors for Undeleted Items (e, error)
Items | eq1 | eq14 | eq15 | eq19 | eq22 | eq23 | eq24 | eq25 | eq27 | eq29 | eq6 | eq7 | eq8 |
---|---|---|---|---|---|---|---|---|---|---|---|---|---|
Measurement error | 0.427 | 0.332 | 0.434 | 0.169 | 0.225 | 0.332 | 0.277 | 0.357 | 0.372 | 0.227 | 0.253 | 0.445 | 0.275 |
Standardized Loading Factors after Reducing the Variables
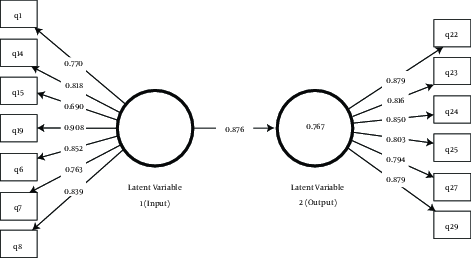
Therefore, all AVE values surpass the acceptable threshold (0.5), and convergent validity was confirmed. In Figure 2, numbers in the circle indicate the degree to which the variance of latent variable two can be explained by other latent variables (10.767).
3.4. Second stage
3.4.1. Reliability
Cronbach’s α and composite reliability exceeded 0.70 for the items.
3.4.2. Convergent validity
The AVE was equal to 0.59 for the input variables and 0.70 for the output variables.
3.5. Discriminant Validity
The HTMT approach was applied in this study to measure discriminant validity. Bootstrapping was applied to determine whether HTMT significantly diverges from one (HTMTinference). There are two hypotheses in HTMT: H0 (HTMT ≥ 1), a confidence interval of one indicates lack of discriminant validity; H1 (HTMT < 1), if the value one falls outside the interval’s range, the two constructs are empirically distinct. In this study, HTMT inference was equal to 0.948 (< 1); therefore, discriminant validity was established.
3.5.1. SRMR
At this stage, SRMR was 0.078 (< 0.080) in our model.
3.5.2. T Value
The results of bootstrapping showed a significant relationship between dependent and independent variables (T value > 1.96); the significant T value was 1.96 at 5% (two - tailed) (Figure 3).
T Values
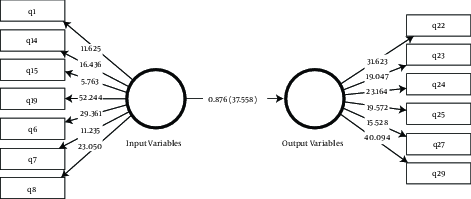
4. Discussion
The present study applied a new approach to SEM for the selection of input and output variables. The observations showed that traditional selection of input and output variables based on the researcher’s preferences is biased. Given the large number of variables for performance evaluation of hospitals in the literature, we aimed to reduce the number of variables. As discussed by O’Neill et al. (2008), based on the review of DEA applications in healthcare during 1984 - 2004, the most common number of both input and output variables is three to five. Besides, the most common input criteria include the number of fully staffed hospital beds, number of clinical staff, and labor expenses, while the most frequent output variables are the number of outpatient visits and treatment intensity.
In a study conducted by Fernandez (13) comparing 30 Florida hospitals, three input variables and two output variables were selected. In the study by Suk (38) in the East South Central region of the United States, seven inputs, as well as two outputs, were preferred. Gollhofer (39) also estimated the efficiency of 32 district hospitals with DEA and used three input variables and two output variables. Moreover, Zhang (15) employed PCA as a strategy to reduce the number of variables, which generated 18 principal output components instead of 51 original output variables.
The findings of the present research are in some way consistent with previous research. For instance, the number of beds (input variable) and outpatient visits (output variables) in our study is consistent with the study by Fernandez (13). The number of physicians (input) and inpatients (output) is consistent with the study by Suk (38), while the number of supporting staff (input) is in congruence with the study by Gollhofer (39). However, with the help of native experts, we chose variables which were not selected in previous research. In addition, with the help of specialists, we identified the most critical variables and reduced the number of variables from 29 to 13 using SEM - PLS (Table 6).
The Selected Variables
Latent Variables | Selected Indicators |
---|---|
Input variables | 1) Number of beds; 6) number of surgeons; 7) number of specialists; 8) number of general practitioners; 19) number of supporting staff; 14) number of professional nurses; 15) number of unprofessional nurses |
Output variables | 22) Number of emergency visits; 23) number of inpatients; 24) number of outpatients; 25) number of discharges; 27) number of laboratory examinations; 29) number of surgeries |
4.1. Conclusion
The present study aimed to identify the most critical input and output variables, affecting the performance evaluation of military hospitals. This investigation also aimed to reduce the number of input and output variables in performance evaluation of hospitals. The findings suggest general contributions of the model in different ways. First, SEM - PLS incorporated a plan to reduce the number of variables; accordingly, it generated 13 crucial output components instead of 29 original output variables. Second, the findings could add to the literature for reducing the number of input and output variables in the DEA context. Third, this model and the presented results could substantiate the available information by explaining how SEM - PLS should be applied.
References
-
1.
Deilmann C, Hennersdorf J, Lehmann I, Reibmann D. Data envelopment analysis of urban efficiency—Interpretative methods to make DEA a heuristic tool. Ecol Indicat. 2018;84:607-18. https://doi.org/10.1016/j.ecolind.2017.09.017.
-
2.
Farrell MJ. The Measurement of Productive Efficiency. J Roy Stat Soc. 1957;120(3):253. https://doi.org/10.2307/2343100.
-
3.
O'Neill L. Nonparametric Diagnostics in Data Envelopment Analysis with an Application to Hospital Efficiency. Pennsylvania State University; 1997.
-
4.
Sherman HD. Hospital efficiency measurement and evaluation. Empirical test of a new technique. Med Care. 1984;22(10):922-38. [PubMed ID: 6436590].
-
5.
Ozcan YA. Health Care Benchmarking and Performance Evaluation: An Assessment Using Data Envelopment Analysis. New York: Springer Science + Business Media, LLC; 2007.
-
6.
Truffer CJ, Keehan S, Smith S, Cylus J, Sisko A, Poisal JA, et al. Health spending projections through 2019: the recession's impact continues. Health Aff (Millwood). 2010;29(3):522-9. [PubMed ID: 20133357]. https://doi.org/10.1377/hlthaff.2009.1074.
-
7.
Litvak E, Bisognano M. More patients, less payment: increasing hospital efficiency in the aftermath of health reform. Health Aff (Millwood). 2011;30(1):76-80. [PubMed ID: 21209441]. https://doi.org/10.1377/hlthaff.2010.1114.
-
8.
Liu JS, Lu LYY, Lu W-M, Lin BJY. A survey of DEA applications. Omega. 2013;41(5):893-902. https://doi.org/10.1016/j.omega.2012.11.004.
-
9.
Gholami R, Anon Higon D, Emrouznejad A. Hospital performance: Efficiency or quality? Can we have both with IT? Expert Syst Appl. 2015;42(12):5390-400. https://doi.org/10.1016/j.eswa.2014.12.019.
-
10.
Emrouznejad A, Yang G. A survey and analysis of the first 40 years of scholarly literature in DEA: 1978–2016. Soc Econ Plann Sci. 2018;61:4-8. https://doi.org/10.1016/j.seps.2017.01.008.
-
11.
Gimenez V, Prieto W, Prior D, Tortosa-Ausina E. Evaluation of efficiency in Colombian hospitals: An analysis for the post-reform period. Soc Econ Plann Sci. 2018. https://doi.org/10.1016/j.seps.2018.02.002.
-
12.
Wagner JM, Shimshak DG. Stepwise selection of variables in data envelopment analysis: Procedures and managerial perspectives. Eur J Oper Res. 2007;180(1):57-67. https://doi.org/10.1016/j.ejor.2006.02.048.
-
13.
Fernandez J. Comparison of the Technical Efficiency of Tax-Supported and Nonprofit Florida Hospitals Using Data Envelopment Analysis. Walden University; 2012.
-
14.
O’Neill L, Rauner M, Heidenberger K, Kraus M. A cross-national comparison and taxonomy of DEA-based hospital efficiency studies. Soc Econ Plann Sci. 2008;42(3):158-89. https://doi.org/10.1016/j.seps.2007.03.001.
-
15.
Zhang Q. Multi-criteria Comparison of Hospital Efficiencies Using Nested Radial Data Envelopment Analysis Models. State University of New York at Binghamton, Thomas J. Watson School of Engineering and Applied Science; 2016.
-
16.
Cooper WW, Seiford LM, Tone K. Introduction to data envelopment analysis and its uses: with DEA-solver software and references. Springer Science & Business Media; 2006.
-
17.
Grisham T. The Delphi technique: a method for testing complex and multifaceted topics. Int J Manag Projects Bus. 2009;2(1):112-30. https://doi.org/10.1108/17538370910930545.
-
18.
F. Hair Jr J, Sarstedt M, Hopkins L, G. Kuppelwieser V. Partial least squares structural equation modeling (PLS-SEM). Eur Bus Rev. 2014;26(2):106-21. https://doi.org/10.1108/ebr-10-2013-0128.
-
19.
Fornell C, Bookstein FL. Two Structural Equation Models: LISREL and PLS Applied to Consumer Exit-Voice Theory. J Market Res. 1982;19(4):440. https://doi.org/10.2307/3151718.
-
20.
Bag S. A Short Review on Structural Equation Modeling: Applications and Future Research Directions. J Supply Chain Manag Syst. 2015;4(3). https://doi.org/10.21863/jscms/2015.4.3.014.
-
21.
Hays RD, Revicki D, Coyne KS. Application of structural equation modeling to health outcomes research. Eval Health Prof. 2005;28(3):295-309. [PubMed ID: 16123259]. https://doi.org/10.1177/0163278705278277.
-
22.
Richter NF, Cepeda G, Roldan JL, Ringle CM. European management research using partial least squares structural equation modeling (PLS-SEM). Eur Manag J. 2016;34(6):589-97. https://doi.org/10.1016/j.emj.2016.08.001.
-
23.
Iacobucci D. Everything you always wanted to know about SEM (structural equations modeling) but were afraid to ask. J Consum Psychol. 2009;19(4):673-80. https://doi.org/10.1016/j.jcps.2009.09.002.
-
24.
Kohl S, Schoenfelder J, Fugener A, Brunner JO. The use of Data Envelopment Analysis (DEA) in healthcare with a focus on hospitals. Health Care Manag Sci. 2018. [PubMed ID: 29478088]. https://doi.org/10.1007/s10729-018-9436-8.
-
25.
Green B, Jones M, Hughes D, Williams A. Applying the Delphi technique in a study of GPs' information requirements. Health Soc Care Community. 1999;7(3):198-205. [PubMed ID: 11560634].
-
26.
Linstone HA, Turoff M. The delphi method. Addison-Wesley Reading, MA; 1975.
-
27.
Keeney S, Hasson F, McKenna HP. A critical review of the Delphi technique as a research methodology for nursing. Int J Nurs Stud. 2001;38(2):195-200. https://doi.org/10.1016/s0020-7489(00)00044-4.
-
28.
Ahmed S. Methods in sample surveys. Johns Hopkins Bloomberg School of Public; 2009.
-
29.
Sturgis. Patrick Confirmatory Factor Analysis (Part 3 of 6). 2016, [cited 14 January]. Available from: www.youtube.com.
-
30.
Diamantopoulos A, Winklhofer HM. Index Construction with Formative Indicators: An Alternative to Scale Development. J Market Res. 2001;38(2):269-77. https://doi.org/10.1509/jmkr.38.2.269.18845.
-
31.
Muzamil. Reflective and Formative Measures. Brief Explanation. 2012, [cited 10 February]. Available from: www.youtube.com.
-
32.
Alarcon D, Sanchez JA, De Olavide U. Assessing convergent and discriminant validity in the ADHD-R IV rating scale: User-written commands for Average Variance Extracted (AVE), Composite Reliability (CR), and Heterotrait-Monotrait ratio of correlations (HTMT). Universidad Pablo de Olavide; 2015. 38 p.
-
33.
Sosik JJ, Kahai SS, Piovoso MJ. Silver Bullet or Voodoo Statistics? Group Organ Manag. 2009;34(1):5-36. https://doi.org/10.1177/1059601108329198.
-
34.
Henseler J, Ringle CM, Sarstedt M. A new criterion for assessing discriminant validity in variance-based structural equation modeling. J Acad Market Sci. 2014;43(1):115-35. https://doi.org/10.1007/s11747-014-0403-8.
-
35.
Hooper D, Coughlan J, Mullen M. Structural equation modelling: Guidelines for determining model fit. Articles. 2008;1:2.
-
36.
Hesterberg T, Moore DS, Monaghan S, Clipson A, Epstein R. Bootstrap methods and permutation tests. Intro Pract Stat. 2005;5:1-70.
-
37.
Barclay D, Higgins C, Thompson R. The Partial Least Squares (pls) Approach to Casual Modeling: Personal Computer Adoption Ans Use as an Illustration. Tech Stud. 1995.
-
38.
Suk YK. Measuring the impact of Total Quality Management (TQM) on efficiency using data envelopment analysis (DEA) in the hospital industry: The case of the East South Central region of the United States. The University of Mississippi; 1999.
-
39.
Gollhofer RE. Measuring the impact of process improvement programs on the performance of hospitals in Mid-Atlantic region using data envelopment analysis. Capella University; 2016.