Abstract
Background:
Alzheimer’s disease (AD), the most typical type of dementia and memory loss, is a complicated and progressive neurodegenerative disorder. Due to the multi-factorial etiology of AD, the multi-target-directed ligand (MTDL) approach can be a potential method in seeking new drug candidates for this disease.Methods:
In this study, over 200 tacrine-naphtoquinone hybrids have been designed and their drug-likeness, molecular docking, and descriptor analysis were conducted to find out a drug candidate with less toxicity and better binding affinity than tacrine. The Docking analysis was conducted using human acetylcholineesterase (1ACJ), human butyrylcholineesterase (4BDS), and β-secretase (BACE1) (1w51) enzymes using Autodock 4.2 and Vina.Results:
Promising results were obtained on the types of interactions. Based on molecular docking on 3 targets as well as protein ligand interaction fingerprint (PLIF) studies, the compounds with better results were introduced as good candidates for synthesis. The validity of docking protocols was confirmed using a set of familiar active ligands and decoys on these targets by means of 2 known statistical metrics such as the receiver-operating characteristic (ROC) and Enrichment Factor (EF).Conclusion:
Structure activity relationship (SAR) studies, in these class of compounds, show that the hydroxyethylamine, as a linker, is an essential group to improving binding site to AChE and BACE-1 targets.Keywords
Multi-Target-Directed Ligand Drug-Likeness Molecular Docking PLIF Studies Alzheimer’s Disease
1. Background
Alzheimer disease (AD) is a complex neurodegenerative process occurring in the central nervous system (CNS). It is normally characterized by masses of β-amyloid (Aβ) and neurofibrillary tangles, inflammatory intermediates, reactive oxygen species (ROS), loss of synapses, and death of cells, especially cholinergic neurons (1, 2).
Most therapeutic treatment for AD are drugs that are intended to restrain enzymes acetylcholineesterase (AChE) and butyrylcholineesterase (BuChE); by doing so they would increase acetylcholine concentration in cholinergic synaptic clefts (3, 4). In addition, lowering the concentration of Aβ peptide in the brain seems to be a rational therapeutic approach for treating AD (5). This can be achieved by diminishing the production of Aβ through inhibition of β-secretase (BACE-1) (6, 7).
Tacrine is an effective inhibitor of both AChE and BuChE, however, it has a high incidence of side-effects and toxicity (8). Accordingly, the development of tacrine-based dimers and hybrids with improved pharmacological properties as well as mitigated side effects has been the hub of attention in recent years (8-10).
The potential of a naphthoquinone substructure in the chemical space related to neurodegenerative diseases such as Alzheimer’s were proved in recent works (11, 12). Naphtoquinone as an anti-amyloid preferential motif, interact with several amyloid proteins, owing to its capability to form favorable π-stacking and hydrogen bond interactions. Naphthoquinone has anti-cholinesterase activity, displays anti-aggregating features, and allows recognition of Trp286 of the peripheral anionic site (PAS) of the human acetylcholinesterase (hAChE) (11). This site of AChE lies at the entrance to the active site pharynx. It is composed of 5 residues (Tyr 72, Asp74, Tyr124, Trp286, and Tyr 341) as well as a number of surface loops, conferring a high degree of conformational flexibility on the area (12).
Due to its multifunctional nature of AD, the development of a ground-breaking drug design strategy centered on multi-target-directed-ligands (MTDLs) is of a paramount import. The multi-target approach has been put forth as principally suitable to fight the heterogeneity of AD. Much recently, the MTDL notion has been exploited to design different ligands achieving different biological targets (13, 14). Molecular hybridization (MH) are normally used to produce MTDLs (15).
Based on the familiar structure of AChE, BuChE, and BACE-1, it was decided to bond the tacrine and naphtoquinone fragments using hydroxyethylamine (HEA) as a connector (16, 17). The HEA core has been utilized successfully for a number of aspartyl proteases, like BACE1.
Docking is mostly applied to predict the binding orientation of a small molecule to their receptors so that the biological affinity and activity of the small molecule drug candidates could possibly be predicted. As a result, docking plays an essential role in drug design. Given the biological and pharmaceutical importance of molecular docking, significant efforts have been exercised to improve the methods used to predict docking (18, 19).
Thus, a library with more than 200 tacrine-naphtoquinone analogues has been devised by means of MTDLs strategy. Their drug-likeness, molecular docking, descriptor analysis, and protein-ligand interaction fingerprints (PLIFs) were carried out to find out a drug candidate with better binding affinity and less toxicity than tacrine. The validity of the docking protocol was also explored using a group of known active ligands and decoys.
2. Methods
2.1. Preparation of the Structures
The 3 dimensional crystal structure of AChE (PDB ID: 1ACJ), BuChE (4BDS), and BACE-1 (1W51) were taken from a protein data bank (20). Water and co-crystal ligand molecules were eliminated from the structures and the PDBs were improved with regards to missing atom types by modeller 9.12 (21). Next, an in house application (MODELFACE) was employed to generate python script and running modeller software. Afterwards, the enzymes were converted to PDBQT and gasteiger partial charges were appended using MGLTOOLS 1.5.6 (22).
2.2. Designing of the Ligands
Based on Scheme 1, more than 200 ligands were designed using MTDLs strategy. The tacrine fragment was selected for its inhibition of AChE and BuChE. The naphtoquinone scaffold was chosen for its anti-cholinesterase, anti-aggregating features, and BACE-1 inhibitory activities. Based on the information acquired from the literature survey, the HEA linker was selected to have BACE-1 inhibitory activities (Figure 1).
Design of Tacrine-Naphtoquinone Derivatives Using MTDLs Strategy
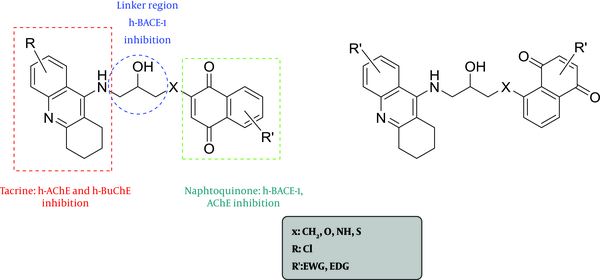
2.3. Optimization of the Ligands
ChemBioDraw ultra v.13 software (Cambridge Software) was used to sketch the 2 dimensional structures of the ligands. Then, the ligands were exposed to minimization procedures by means of an in house TCL script using Hyperchem (Version 8, Hypercube Inc., Gainesville, FL, USA). Each ligand was optimized using the molecular mechanics method (MM+) followed by quantum-based semi-empirical method (AM1) utilizing HyperChem 8. From then on, the output structures were altered to PDBQT via MGLtools 1.5.6 for the docking procedure.
2.4. Drug-Likeness Analysis
Drug-likeness rules are a set of principles for the structural properties of compounds. It is used to quickly calculate drug-like properties of a molecule. Using DruLiTo (23), an open source virtual screening tool, as shown in Table 1, drug-likeness descriptors such as molecular weight (MW), logP, AlogP, H-bond acceptor (HBA), H-bond donor (HBD), total polar surface area (TPSA), atom molar refractivity(AMR), number of rotatable bond (nRB), number of Atom, rotatable bond count (RC), number of rigid bond (nRigidB), nAtom Ring, and nHB (number of Hydrogen Bond) for all of the ligands were calculated. DruLiTo calculations are dependent on various drug-likeness rules namely Lipinski's rule, Veber rule, Ghosefilter, BBB rule, CMC-50 like rule, and quantitative estimate of drug-likeness (QED). Those compounds. which successfully passed the drug-likeness filter, were subjected to docking studies.
Drug-Likeness Descriptors Calculated by DruLiTo Application
No. | MW | logP | AlogP | HBA | HBD | TPSA | AMR | nRB | nAtom | RC | nRigidB | nAromRing | nHB |
---|---|---|---|---|---|---|---|---|---|---|---|---|---|
1 | 419.98 | 0.931 | -1.224 | 7 | 0 | 55.73 | 130.02 | 6 | 33 | 5 | 31 | 3 | 7 |
2 | 415.99 | 1.06 | -1.056 | 6 | 0 | 46.5 | 130.92 | 6 | 33 | 5 | 31 | 3 | 6 |
3 | 454.93 | 2.403 | 0.559 | 5 | 0 | 71.8 | 139.38 | 6 | 33 | 5 | 31 | 3 | 5 |
4 | 438.95 | 1.589 | -0.292 | 6 | 0 | 55.73 | 133.13 | 6 | 33 | 5 | 31 | 3 | 6 |
5 | 438.95 | 1.589 | -0.292 | 6 | 0 | 55.73 | 133.13 | 6 | 33 | 5 | 31 | 3 | 6 |
6 | 436.96 | 1.32 | -0.559 | 6 | 0 | 46.5 | 134.81 | 6 | 33 | 5 | 31 | 3 | 6 |
7 | 471.93 | 1.897 | -0.083 | 6 | 0 | 46.5 | 139.6 | 6 | 34 | 5 | 32 | 3 | 6 |
8 | 489.9 | 2.558 | 0.928 | 5 | 0 | 71.8 | 144.1 | 6 | 34 | 5 | 32 | 3 | 5 |
9 | 454.95 | 1.297 | -0.856 | 7 | 0 | 55.73 | 134.74 | 6 | 34 | 5 | 32 | 3 | 7 |
10 | 450.95 | 1.426 | -0.687 | 6 | 0 | 46.5 | 135.63 | 6 | 34 | 5 | 32 | 3 | 6 |
11 | 470.93 | 1.689 | -0.111 | 6 | 0 | 71.8 | 140.91 | 6 | 34 | 5 | 32 | 3 | 6 |
12 | 471.93 | 2.537 | -0.083 | 6 | 0 | 46.5 | 139.6 | 6 | 34 | 5 | 32 | 3 | 6 |
13 | 473.92 | 1.955 | 0.076 | 6 | 0 | 55.73 | 137.85 | 6 | 34 | 5 | 32 | 3 | 6 |
14 | 469.93 | 2.506 | 0.244 | 5 | 0 | 46.5 | 138.75 | 6 | 34 | 5 | 32 | 3 | 5 |
15 | 471.93 | 1.686 | -0.19 | 6 | 0 | 46.5 | 139.53 | 6 | 34 | 5 | 32 | 3 | 6 |
16 | 504.9 | 3.083 | 0.72 | 5 | 0 | 46.5 | 143.54 | 6 | 35 | 5 | 33 | 3 | 5 |
17 | 506.9 | 2.263 | 0.285 | 6 | 0 | 46.5 | 144.32 | 6 | 35 | 5 | 33 | 3 | 6 |
18 | 487.93 | 2.034 | -0.753 | 7 | 0 | 46.5 | 141.13 | 6 | 35 | 5 | 33 | 3 | 7 |
19 | 505.9 | 2.266 | 0.258 | 6 | 0 | 71.8 | 145.63 | 6 | 35 | 5 | 33 | 3 | 6 |
20 | 508.89 | 2.954 | 0.552 | 6 | 0 | 55.73 | 142.64 | 6 | 35 | 5 | 33 | 3 | 6 |
2.5. Docking Procedure
To attain docking simulations, an in house batch script (DOCKFACE) (24, 25) was utilized for the automatic running of Autodock 4.2 and auto-dock Vina in a parallel mode. Genetic algorithm search method was applied to establish the best pose of each ligand in the active site of the target enzymes in all the experiments. Having rendered the center of the ligand to a certain position within the receptor active site and making a series of rotamers, random orientations of the conformations were generated. This process was cyclically repeated to the point that the desired number of low-energy orientations was obtained. The dockings were carried out on flexible ligands and rigid receptors. For the Lamarckian GA method: 100 No. of GA run, 27,000 maximum generations; 2,500,000 maximum No. of evaluations, 150 population size, mutation rate of 0.02; and a crossover rate of 0.8 were used. A grid box of 50 × 50 × 50 points in x, y, and z direction with a grid spacing of 0.375 Å was built for all targets. Center of grid box in x, y, and z was 4.4, 69.9, and 65.8 for AChE, 55.7, 46.5. and 81.1 for BuChE 63, -3.7, and 75 for BACE-1.
2.6. Analysis of Docking Results
Right after finishing the docking process, the analysis of the protein-ligand complex was carried out so as to realize the type of interactions. Top ranked binding energies (kcal/mol) in the AutoDock dlg output file were taken as a response in each run.
Autodock Vina is a substitute for Autodock 4.2 and has a new scholarly, statistical scoring function rather than the semi-empirical force field of Autodock4.2. Due to great prediction accuracy and speed over Autodock4.2, Vina results were selected as the best docking binding energies. Docking results were corroborated almost by high cluster populations. The best docking result in each case was considered to be the conformation with the lowest binding energy. Figure 2 reveals the ligands with the best docking results regarding their binding free energy to the receptors.
Compounds with Best Docking Binding Scores (Part I).
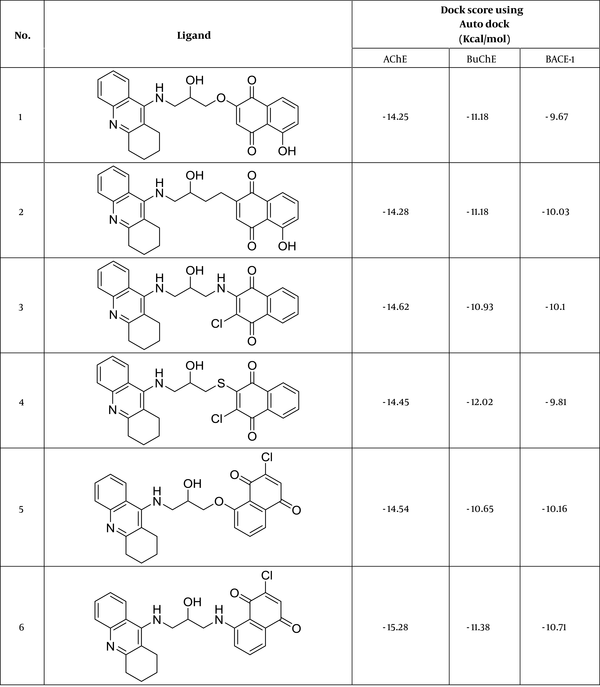
2.7. Protein Ligand Interaction Fingerprint (PLIF)
The poses of docking were extracted from dlg files so that PLIF studies on docking results could be performed by means of preAuposSOM application (26). The resulted pdbqt’s and the receptor were converted to mol2 by means of a batch script using Open Babel 2.3.1. The resulted mol2 files were subjected to AuposSOM 2.1 web server (27). Two training phases with 1000 iterations were set in the self-regulating map settings of AuposSOM conf files. Other parameters of the software were retained as default. The produced files were subjected to Dendroscope 3.2.10 (28, 29) for revelation of the results. Dendroscope is a phylogenetic relationship software that is capable of visualizing rooted phylogenetic trees and networks efficiently.
3. Results and Discussion
Cluster analysis was performed on the docked results using a root mean square deviation (RMSD) tolerance of 2 Å. For the internal validation phase, co-crystal ligand (tacrine) inside the pdb file of AChE (1ACJ) and BuChE (4BDS) was extracted using a viewer and treated the same as other ligands. All the docking results produced RMSD values below 2 Å. For more reliable docking results, 2 other metrics were used to assess the validity of docking.
A series of 106 AChE inhibitors, 161 BACE-1 inhibitors, and 42 BuChE inhibitors were retrieved from the ChEMBL database in the SMILES format to calculate the validity of the docking process (30-32). Iterative runs of open babel 2.3.2 through a shell script offered the main 3D generation of the structures as mol2 format. In order to use this metric in a virtual screening (VS) study, the ligands must be initially divided into 2 subsets of actives and decoys based on their experimental activities. Next, these ligands and decoys were docked by our set up docking procedure. The use of ROC in computational medicinal chemistry was extensively employed as a practical metric to evaluate the validity of docking scores in VS studies. It is at this phase when for all docking scores, ROC plots are subsequently being obtained by plotting (Se) versus (1-Sp). The area under the curve for ROC plots is computed by the trapezoidal integration method as implemented in our in house application. A high ROCAUC value suggests that the docking protocol is more precise to differentiate between active ligands and decoys. Enrichment Factor is another tool to evaluate the efficiency of the docking protocol in VS studies. In comparison to ROC plot, EFmax factor is strongly hinged on the number of actives in a data set. Since ROC values do not depend to the number of actives and decoys, they are more valuable in making decisions about the validity of the methods than EFmax analysis. The plots and results of ROC and EFmax provided for BACE-1 are demonstrated in Figure 3. To avoid lengthening the manuscript, other plots of ROC and EFmax are appended in the supplementary section.
Compounds with Best Docking Binding Scores (Part II).
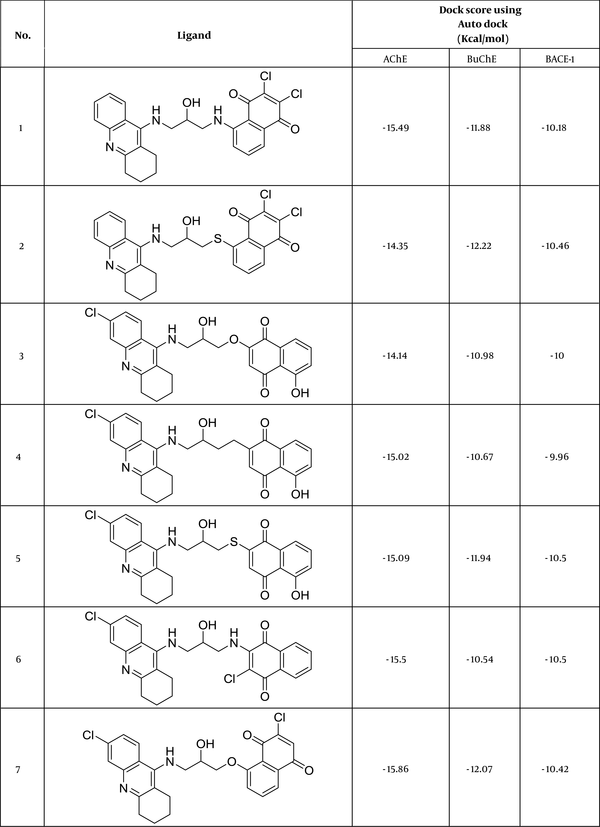
As another reliable analysis technique, Protein ligand interaction fingerprint (PLIF) was used mainly in computational chemistry studies. PLIF is another interpretation on docking results. This method allows for studying the impact of different starting states of the structures on generated poses as well as their parallel vector of contacts towards the receptor during the docking procedure (33). As it was described earlier, to calculate their contact vectors within the receptor binding cavity, all generated poses of ligands and the tacrine were exposed to AuposSOM 2.1. In this method, the contacts between the ligands and the receptor include hydrophobic, hydrogen bonding, and coulombic interactions. The resulted vectors of contacts were later analyzed using the self-organizing map as implemented in the AuposSOM software. The outcome of self-organizing map is a classification pattern for ligands. As it was shown in Figure 4, tacrine with ligand numbers 3, 7, 12, 14, 17, and 18 are in the same subgroup. These compounds can be suitable candidates for synthesis, as it is evident, compounds in the same subgroup may show a similar behavior.
Compounds with Best Docking Binding Scores Part (III)
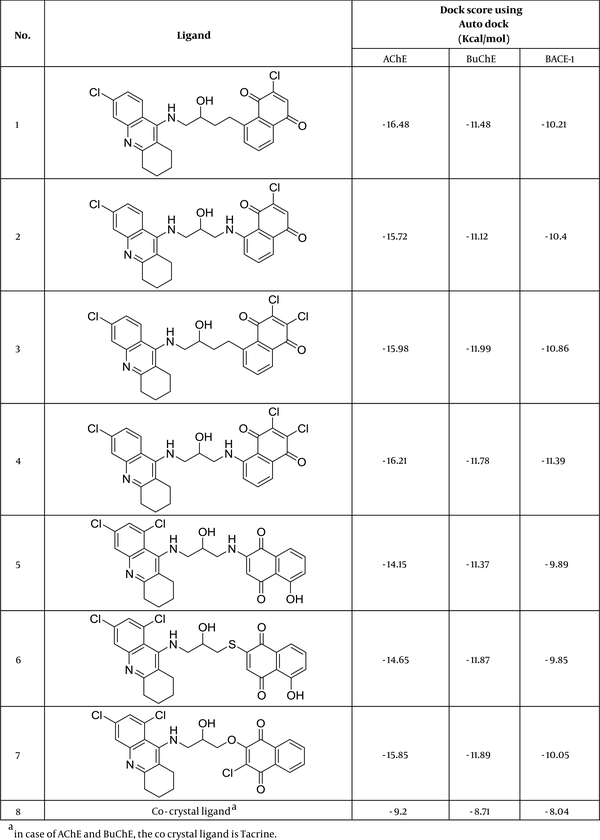
Binding interactions between docked potent agents and the targets were analyzed using Autodock tools program (ADT, Version 1.5.6) and PLIP (fully automated protein–ligand interaction profiler) (34). As it can be seen in Figure 4A, 3 types of interactions including hydrogen bond, π-Stacking, and hydrophobic are existed between compound 14 and AChE active site. A hydrogen bond interaction exists between NH of tacrine moiety in this compound with TYR331 and there also exist hydrogen bonds between carbonyl groups of quinone moiety with SER119 and GLY114. Two π-Stacking interactions also existed between phenyl and pyridine of tacrine with TRP81 and PHE327, respectively. Some hydrophobic interactions with ASP69, TRP81, TRP429, PHE327, ILE436, and TYR439 are shown in Figure 5A. Compound 17 interact with AChE receptor through different hydrogen bond and hydrophobic interactions. As it is shown in Figure 5B, hydroxyl of HEA interacts with ASN82 and NH of tacrine moiety interact with TYR118 and SER119 through hydrogen bonds. The hydrophobic reactions with TRP81, TRP429, PHE327, PHE328, and TYR331 are shown in Figure 5B.
ROC and EF Diagrams for BACE-1 Receptor
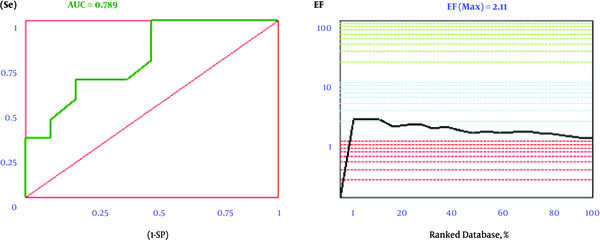
In the BuChE binding mode, Compound 14 interacts via hydrogen bonds through its hydroxyl of HEA with THR117. As it was depicted in Figure 6A, there is evidence that there are some π-Stacking interactions between phenyl and pyridine of tacrine, with TRP79 as well as some hydrophobic interactions. Compound 17 interacts via hydrogen bonds through its hydroxyl of HEA with ASN80, NH of tacrine with ASP67, NH of HEA with THR117, and carbonyl of quinone with SER195 (Figure 6B). A π-Stacking interaction with TYR329 and some hydrophobic interactions with TRP79 and PHE326 are also shown in Figure 6B.
AuposSOM Results for Poses of Docking
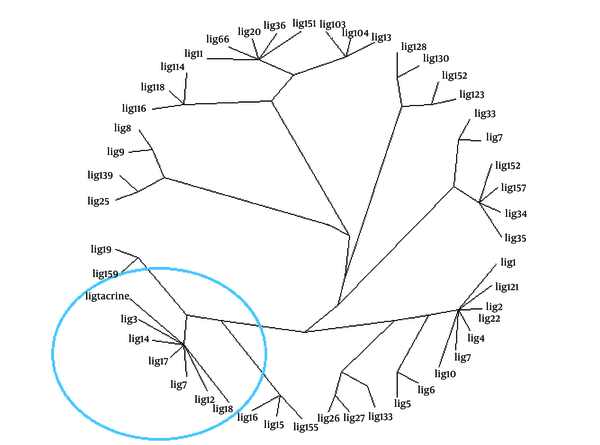
In BACE1 receptor, hydroxyl group of HEA in Compound 14 interact via hydrogen bond with ASP34. Some other hydrogen bonds exist between NH of tacrine moiety with GLN75 and carbonyl of quinone with TYR190 and LYS216. The hydrophobic interactions with LEU32, TYR73, THR74, GLN75, TRP117, and TYR190 are shown in Figures 7 - 9.
A) Compound 14, B) Compound 17, Interactions with the Residues in the Binding Site of AChE Receptor (1ACJ)
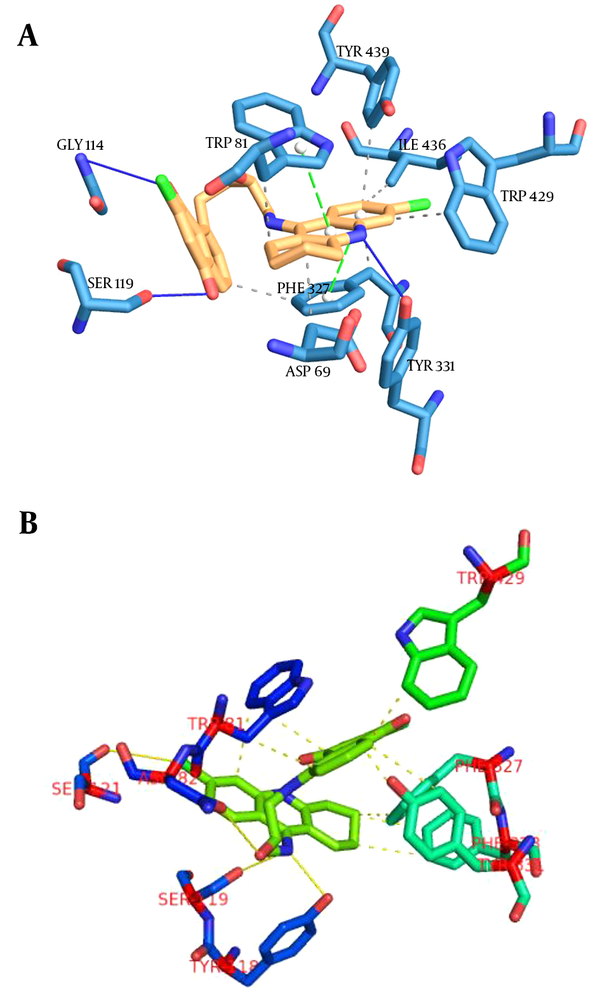
A) Compound 14, B) Compound 17, Interactions with the Residues in the Binding Site of BuChE Receptor (4bds)
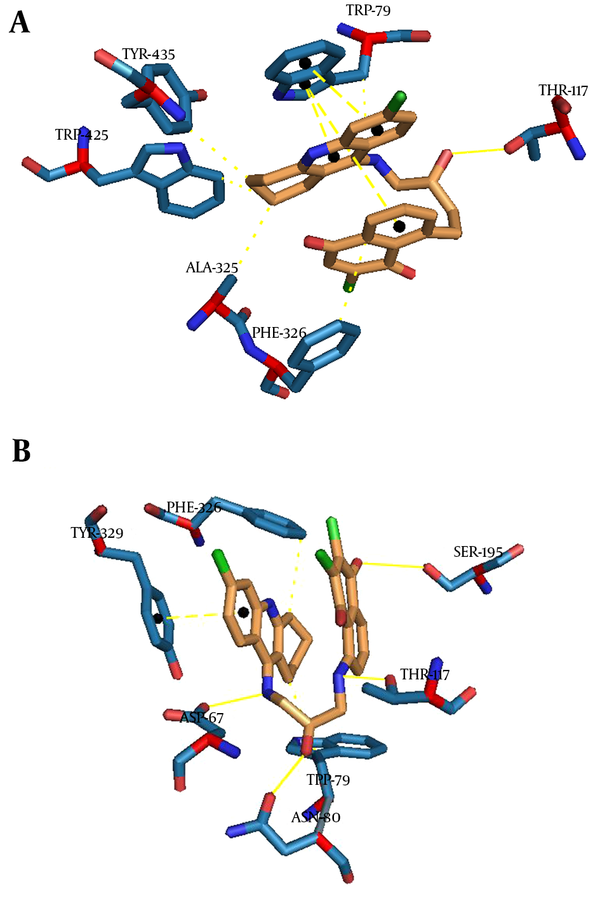
Interactions of Compound 14 with the Residues in the Binding Site of BACE-1 Receptor (1w51)
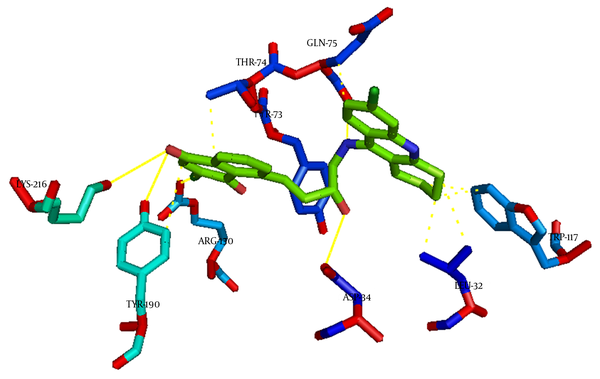
The most important functional group in this class of compounds is the hydroxyl group. Results show that the hydroxyethylamine (especially its hydroxyl group), as a linker, is an essential group to improving binding site to AChE, BuChE, and BACE-1 targets. This flexible linker could be lodged by the enzyme cavity participating in hydrogen bonding and allowing simultaneous interaction between the tacrine moiety with both the catalytic active site (CAS) and peripheral anionic site (PAS) of the AChE enzyme.
4. Conclusions
MTDL approach is a promising method in searching new drug candidates for Alzheimer’s disease. In this study, more than 200 tacrine-naphtoquinone hybrids have been designed using the MTDL strategy. The docking analyses as well as PLIF studies of our designed ligands showed that 20 ligands are efficient in their docking scores and high binding nature. Thus, these analogues are good candidates for synthesis and should be taken into account for further evaluations using in vitro and in vivo studies to develop effective multifunctional drugs for the treatment of Alzheimer’s disease.
Acknowledgements
References
-
1.
Hashimoto M, Rockenstein E, Crews L, Masliah E. Role of protein aggregation in mitochondrial dysfunction and neurodegeneration in Alzheimer's and Parkinson's diseases. Neuromolecular Med. 2003;4(1-2):21-36. [PubMed ID: 14528050]. https://doi.org/10.1385/NMM:4:1-2:21.
-
2.
Soler-Lopez M, Badiola N, Zanzoni A, Aloy P. Towards Alzheimer's root cause: ECSIT as an integrating hub between oxidative stress, inflammation and mitochondrial dysfunction. Hypothetical role of the adapter protein ECSIT in familial and sporadic Alzheimer's disease pathogenesis. Bioessays. 2012;34(7):532-41. [PubMed ID: 22513506]. https://doi.org/10.1002/bies.201100193.
-
3.
Munoz-Torrero D. Acetylcholinesterase inhibitors as disease-modifying therapies for Alzheimer's disease. Curr Med Chem. 2008;15(24):2433-55. [PubMed ID: 18855672].
-
4.
Wolfe MS. Secretase targets for Alzheimer's disease: identification and therapeutic potential. J Med Chem. 2001;44(13):2039-60. [PubMed ID: 11405641].
-
5.
Shanks M, Kivipelto M, Bullock R, Lane R. Cholinesterase inhibition: is there evidence for disease-modifying effects? Curr Med Res Opin. 2009;25(10):2439-46. [PubMed ID: 19678754]. https://doi.org/10.1185/03007990903209332.
-
6.
Chakrabarti E, Ghosh S, Sadhukhan S, Sayre L, Tochtrop GP, Smith JD. Synthesis and biological evaluation of analogues of a novel inhibitor of beta-amyloid secretion. J Med Chem. 2010;53(14):5302-19. [PubMed ID: 20568779]. https://doi.org/10.1021/jm100308g.
-
7.
Faghih Z, Fereidoonnezhad M, Tabaei SMH, Rezaei Z, Zolghadr AR. The binding of small carbazole derivative (P7C3) to protofibrils of the Alzheimer’s disease and β-secretase: Molecular dynamics simulation studies. Chem Phys. 2015;459:31-9. https://doi.org/10.1016/j.chemphys.2015.07.026.
-
8.
Hamulakova S, Janovec L, Hrabinova M, Spilovska K, Korabecny J, Kristian P, et al. Synthesis and biological evaluation of novel tacrine derivatives and tacrine-coumarin hybrids as cholinesterase inhibitors. J Med Chem. 2014;57(16):7073-84. [PubMed ID: 25089370]. https://doi.org/10.1021/jm5008648.
-
9.
Fernandez-Bachiller MI, Perez C, Monjas L, Rademann J, Rodriguez-Franco MI. New tacrine-4-oxo-4H-chromene hybrids as multifunctional agents for the treatment of Alzheimer's disease, with cholinergic, antioxidant, and beta-amyloid-reducing properties. J Med Chem. 2012;55(3):1303-17. [PubMed ID: 22243648]. https://doi.org/10.1021/jm201460y.
-
10.
Thiratmatrakul S, Yenjai C, Waiwut P, Vajragupta O, Reubroycharoen P, Tohda M, et al. Synthesis, biological evaluation and molecular modeling study of novel tacrine-carbazole hybrids as potential multifunctional agents for the treatment of Alzheimer's disease. Eur J Med Chem. 2014;75:21-30. [PubMed ID: 24508831]. https://doi.org/10.1016/j.ejmech.2014.01.020.
-
11.
Nepovimova E, Uliassi E, Korabecny J, Pena-Altamira LE, Samez S, Pesaresi A, et al. Multitarget drug design strategy: quinone-tacrine hybrids designed to block amyloid-beta aggregation and to exert anticholinesterase and antioxidant effects. J Med Chem. 2014;57(20):8576-89. [PubMed ID: 25259726]. https://doi.org/10.1021/jm5010804.
-
12.
Johnson G, Moore SW. The peripheral anionic site of acetylcholinesterase: structure, functions and potential role in rational drug design. Curr Pharm Des. 2006;12(2):217-25. [PubMed ID: 16454738].
-
13.
Hui AL, Chen Y, Zhu SJ, Gan CS, Pan J, Zhou A. Design and synthesis of tacrine-phenothiazine hybrids as multitarget drugs for Alzheimer’s disease. Med Chem Res. 2014;23(7):3546-57. https://doi.org/10.1007/s00044-014-0931-2.
-
14.
Minarini A, Milelli A, Simoni E, Rosini M, Bolognesi ML, Marchetti C, et al. Multifunctional tacrine derivatives in Alzheimer's disease. Curr Top Med Chem. 2013;13(15):1771-86. [PubMed ID: 23931443].
-
15.
Nepali K, Sharma S, Sharma M, Bedi PM, Dhar KL. Rational approaches, design strategies, structure activity relationship and mechanistic insights for anticancer hybrids. Eur J Med Chem. 2014;77:422-87. [PubMed ID: 24685980]. https://doi.org/10.1016/j.ejmech.2014.03.018.
-
16.
Digiacomo M, Chen Z, Wang S, Lapucci A, Macchia M, Yang X, et al. Synthesis and pharmacological evaluation of multifunctional tacrine derivatives against several disease pathways of AD. Bioorg Med Chem Lett. 2015;25(4):807-10. [PubMed ID: 25597007]. https://doi.org/10.1016/j.bmcl.2014.12.084.
-
17.
Kacker P, Masetti M, Mangold M, Bottegoni G, Cavalli A. Combining dyad protonation and active site plasticity in BACE-1 structure-based drug design. J Chem Inf Model. 2012;52(5):1079-85. [PubMed ID: 22313091]. https://doi.org/10.1021/ci200366z.
-
18.
Fereidoonnezhad M, Faghih Z, Mojaddami A, Tabaei SMH, Rezaei Z. Novel approach synthesis, molecular docking and cytotoxic activity evaluation of N-phenyl-2, 2-dichloroacetamide derivatives as anticancer agents. J Sci Islamic Republic Iran. 2016;27(1):39-49.
-
19.
Hamedi A, Khoshnoud MJ, Tanideh N, Abbasi F, Fereidoonnezhad M, Mehrabani D. Reproductive Toxicity of Cassia Absus Seeds in Female Rats: Possible Progesteronic Properties of Chaksine and b-Sitosterol. Pharm Chem J. 2015;49(4):268-74. https://doi.org/10.1007/s11094-015-1268-y.
-
20.
Hikisz P, Szczupak L, Koceva-Chyla A, Gu Spiel A, Oehninger L, Ott I, et al. Anticancer and Antibacterial Activity Studies of Gold(I)-Alkynyl Chromones. Molecules. 2015;20(11):19699-718. [PubMed ID: 26528965]. https://doi.org/10.3390/molecules201119647.
-
21.
Webb B, Sali A. Comparative Protein Structure Modeling Using Modeller. Current Protocols in Bioinformatics. John Wiley & Sons; 2014.
-
22.
Morris GM, Huey R, Olson AJ. Using autodock for ligand‐receptor docking. Current protocols in bioinformatics. John Wiley & Sons; 2008.
-
23.
Niper. Protocols. 2015. Available from: http://www.niper.gov.in/pi_dev_tools/DruLiToWeb/DruLiTo_Contact_us.html.
-
24.
Fereidoonnezhad M, Faghih Z, Mojaddami A, Sakhteman A, Rezaei Z. A comparative docking studies of dichloroacetate analogues on four isozymes of pyruvate dehydrogenase kinase in humans. Indian J Pharm Edu Res. 2016;50(2):S32-8.
-
25.
Fereidoonnezhad M, Faghih Z, Jokar E, Mojaddami A, Rezaei Z, Khoshneviszadeh M. QSAR, Molecular Docking and protein ligand interaction fingerprint studies of N-phenyl dichloroacetamide derivatives as anticancer agents. Trends Pharm Sci. 2016;2(2).
-
26.
Sakhteman A. PreAuposSOM. 1015. Available from: https://www.biomedicale.univ-paris5.fr/aupossom/.
-
27.
Mantsyzov AB, Bouvier G, Evrard-Todeschi N, Bertho G. Contact-based ligand-clustering approach for the identification of active compounds in virtual screening. Adv Appl Bioinform Chem. 2012;5:61-79. [PubMed ID: 23055752]. https://doi.org/10.2147/AABC.S30881.
-
28.
Huson DH, Scornavacca C. Dendroscope 3: an interactive tool for rooted phylogenetic trees and networks. Syst Biol. 2012;61(6):1061-7. [PubMed ID: 22780991]. https://doi.org/10.1093/sysbio/sys062.
-
29.
Huson DH, Richter DC, Rausch C, Dezulian T, Franz M, Rupp R. Dendroscope: An interactive viewer for large phylogenetic trees. BMC Bioinformatics. 2007;8:460. [PubMed ID: 18034891]. https://doi.org/10.1186/1471-2105-8-460.
-
30.
Gaulton A, Bellis LJ, Bento AP, Chambers J, Davies M, Hersey A, et al. ChEMBL: a large-scale bioactivity database for drug discovery. Nucleic Acids Res. 2012;40(Database issue):D1100-7. [PubMed ID: 21948594]. https://doi.org/10.1093/nar/gkr777.
-
31.
Wassermann AM, Bajorath J. BindingDB and ChEMBL: online compound databases for drug discovery. Expert Opin Drug Discov. 2011;6(7):683-7. [PubMed ID: 22650976]. https://doi.org/10.1517/17460441.2011.579100.
-
32.
Willighagen EL, Waagmeester A, Spjuth O, Ansell P, Williams AJ, Tkachenko V, et al. The ChEMBL database as linked open data. J Cheminform. 2013;5(1):23. [PubMed ID: 23657106]. https://doi.org/10.1186/1758-2946-5-23.
-
33.
Huang Q, Jin H, Liu Q, Wu Q, Kang H, Cao Z, et al. Proteochemometric modeling of the bioactivity spectra of HIV-1 protease inhibitors by introducing protein-ligand interaction fingerprint. PLoS One. 2012;7(7). e41698. [PubMed ID: 22848570]. https://doi.org/10.1371/journal.pone.0041698.
-
34.
Salentin S, Schreiber S, Haupt VJ, Adasme MF, Schroeder M. PLIP: fully automated protein-ligand interaction profiler. Nucleic Acids Res. 2015;43(W1):W443-7. [PubMed ID: 25873628]. https://doi.org/10.1093/nar/gkv315.