Abstract
Background:
Vulvovaginal candidiasis (VVC) is a common fungal infection caused by Candida species in the female genital tract.Objectives:
This study attempts to predict predisposition to VVC related to risk factors and clinical symptoms among vaginitis cases using the artificial neural network (ANN) model.Methods:
This cross-sectional study was performed on 250 women referred to gynecology clinics in Birjand, Iran. A questionnaire was used to record participants' demographic information. Swabs were used for wet mounts and culture. Candida species were identified by morphological and physiological methods. The performance of the optimal neural network model was assessed by the sensitivity, specificity, and accuracy area under the ROC curve (AUC). Descriptive statistics were used for the statistical description of data, and chi-square test, t-test, and ANN analysis using SPSS application tools (Statistical Product and Service Solutions) version 22 software at 0.05 significant level.Results:
The prevalence of vulvovaginal candidiasis was 41.0%, and Candida albicans was the most frequently identified species (55.9%). The descriptive statistics (chi-square test and t-test) revealed no significant difference between the frequencies of Candida infection with demographic factors and clinical presentations. However, factors such as abortion history, number of sexual intercourse, dyspareunia, education, natural vaginal delivery (NVD), and lower abdominal pain included in our ANN model had significant differences (P < 0.05).Conclusions:
The result of the ANN model revealed that using demographic factors and clinical symptoms can predict VVC infection. Therefore, this model can identify the effect of the clinical presentations and symptoms of infection.Keywords
Artificial Neural Network Vulvovaginal Candidiasis Risk-factors Clinical Symptoms Statistical Model
1. Background
Vulvovaginal candidiasis (VVC) is a worldwide concern due to consequences related to reproductive health, treatment cost, sexually transmitted diseases (STD), and ascending genital tract infection (1). Candida species, especially Candida albicans, are the major causes of VVC (2). The factors predisposing to VVC include: broad-spectrum antibiotics use, oral contraceptives (OCP), diabetes mellitus (DM), sexual activity, pregnancy, hormonal disorders, and particular immunological defects (3, 4). Besides, recurrent VVC has demonstrated that 75% of women experience this infection at least once during their reproductive age, and 15% suffer recurrent vulvovaginal candidiasis (RVVC) (1, 5).
Although the latest studies have demonstrated that this disease can harm society, in most developing countries, VVC has received little attention and is considered a trivial disease (6). Candida albicans is the most common cause of VVC, but non-Candida species have increased recently (7, 8). On the other hand, this has caused the emergence of species resistant to azole antifungal therapy due to inappropriate treatment (9-11). Therefore, by a rapid and specific diagnosis and prediction relevant to the risk factors of this disease, its social problems can be minimized.
In predicting, modeling, detecting, and classifying relational patterns among variables, artificial neural network (ANN) models have broad applications compared to conventional models, such as the response surface methodology (RSM) (12). The ANN models can identify patterns between variables (demographic factors and clinical symptoms) with minimum squared errors and high correlation coefficients without limiting assumptions such as normality (13-15). In medicine, psychology, and even humanities, ANN models' efficiency in finding a complicated relationship between variables has been demonstrated (16, 17). Therefore, different variables are used to predict predisposition to VVC by ANN modeling.
2. Objectives
There are few reports of the ANN model to predict fungal infections about risk factors. This study attempts to use the ANN model to predict VVC relative to predisposing risk factors such as age, clinical symptoms (itching, discharge, burning), lower abdominal pain, atypical hemorrhage, dyspareunia, history of infection, contraception methods, etc.
3. Methods
3.1. Study Design
This cross-sectional study was performed on 250 women (18 - 60 years) referred to gynecology clinics in Birjand, Iran. After receiving permission from the Ethics Committee of the Birjand University Medical Sciences, Iran (IR.BUMS.REC.1399.184), the patient’s demographic characteristics (age, occupation, location, education, history of infection, symptoms, and drug consumption) were recorded in the checklist confidentially. The inclusion criteria for the study were being married, pregnant or menstruating women, women with severe medical disorders, and those with a history of hysterectomy were excluded. Informed consent was obtained from all participants. No complication was detected after taking the vaginal samples.
3.2. Sample Collection
In this study, 250 vaginal samples were collected from the outer third of the vaginal wall with sterile cotton-tipped swabs and then were inoculated into 2 ml of normal saline for transport to the mycology laboratory. Subsequently, microscopic and colony morphology examination was conducted to diagnose the infection. The smear was prepared from these samples and put onto glass slides. A microscopic examination of each patient was conducted to detect yeasts or pseudo-hyphae by Gram staining. Further microscopic examination was performed using saline and 10% KOH preparations, and the samples were subjected to growth on Sabouraud Dextrose Agar (SDA Merck, Germany) medium after incubation for 24, 48, and 72 h at 37°C growth was observed and evaluated.
3.3. The Culture and Identification of Fungi
The positive culture was analyzed for species identification of yeast isolates by standard procedures, including morphology, germ tube test, chlamydospore formation (cornmeal agar test), and chromogenic medium CHROM agar (CAC, Becton Dickinson, Heidelberg, Germany), which was ready to use (32°C for 24 - 48h). Presumptive identification of C. albicans, C. glabrata, C. tropicalis, C. krusei, and C. parapsilosis were made based on the morphology and colors of the colonies. Further species level identifications were made by germ tube production at 3 h incubation in serum at 37°C and the chlamydospore formation on corn meal-Tween 80 agar (CTA; Difco, Detroit, MI, USA) (30°c for 48h).
3.4. Statistical Analysis
Parallel processing procedures like the neural network connect simple neurons in the input, hidden, and output layers. The independent variables are the input layer neurons, and the response variables are the output layer neurons. Therefore, the structure of the neural network is determined according to the number of neurons in the hidden layer, the type of connections, and the binding function between the neurons in the hidden layer and other layers (18). The multilayer feed-forward back-propagation neural network, a widely used and robust neural network, predicted vulvovaginal candidiasis in the present study. In the ANN model of this study, age, location, occupation status, education, clinical symptoms (itching, discharge, burning), lower abdominal pain, atypical hemorrhage, dyspareunia, history of infection, contraception methods, natural vaginal delivery (NVD), cesarean section (CS), history of infertility, recent antibiotic use, sexual intercourse, and abortion history were the input variables, and the output variable was vulvovaginal candidiasis. Data from all backgrounds were utilized in the study dataset with Adam optimizer, and Initial Lambda, Initial Sigma, Interval Center, Interval Offset, and Maximum Training Epochs were 5e-7, 5e-5, 0, ±0.5, and automatically, respectively. To achieve this, 90% of the data were merged into the network training procedure, and 10% were used for model assessment—more sigmoid and hyperbolic tangent functions connected to the input and hidden liner. Hyperbolic tangent or sigmoid transfer functions connect the hidden and output layer neurons (18). Afterward, convenient functions were performed to optimize the network by increasing the neuron numbers in the hidden layer from 2 to 15. Each network was repeated three times to avoid the effect of a random assignment of weights and random correlations, and the mean sum of squared errors was used as a suitable index for model evaluation. A total of 213 network data were used to train the model, and the model's performance was evaluated using the remaining 24 data. The performance of the optimal neural network model was assessed by the sensitivity, specificity, and accuracy area under the ROC curve (AUC). Descriptive statistics were used to describe the data statistically, chi-square test, t-test, and ANN analysis using SPSS (Statistical Product and Service Solutions) version 22 software.
4. Results
In this study, the mean age (± SD) of the 250 study subjects was 34.6 ± 9.99 years. Among 249 women, 102 (41.0%) were culture-positive for Candida species. Of the 102 Candida isolates, C. albicans was the most frequent isolate, which was recovered from 57 (55.9%), followed by C. parapsilosis 24 (23.5%), C. galabratain 20 (19.6%), and C. kruseiin 1 (1.0%).
Demographic and clinical characteristics of the study subjects are shown in Table 1. This study also investigated the relationship between demographic characteristics (e.g., location, urban/rural, occupation, education) and the prevalence of vulvovaginal candidiasis. Results demonstrated no significant relationship between the demographic characteristics and the prevalence of vaginal candidiasis (P > 0.05).
The Demographic Information and Clinical Symptoms of Study Subjects
Variables | Negative Culture (n = 147) | Positive Culture (n = 102) | Total (n = 250) | P-Value |
---|---|---|---|---|
Age | 0.4 | |||
< 20 | 7 (4.8) | 7 (6.9) | 14 (5.6) | |
20 - 35 | 74 (50.3) | 60 (58.8) | 134 (53.8) | |
35 - 50 | 52 (35.4) | 28 (27.5) | 80 (32.1) | |
> 50 | 14 (9.5) | 7 (6.9) | 21 (16.8) | |
location | 0.8 | |||
Urban | 102 (69.4) | 69 (67.6) | 171 (68.7) | |
Rural | 45 (30.6) | 33 (32.4) | 78 (31.3) | |
Occupation status | 0.842 | |||
Employed | 18 (12.2) | 11 (10.8) | 29 (11.6) | |
Housewife | 129 (87.8) | 91 (89.2) | 220 (88.3) | |
Education | 0.542 | |||
Academic | 30 (20.4) | 25 (24.5) | 55 (22.0) | |
Middle school | 102 (69.4) | 70 (68.6) | 172 (69.0) | |
Illiterate | 15 (10.2) | 7 (6.9) | 22 (8.8) | |
Number of deliveries | 0.539 | |||
No | 35 (23.8) | 20 (29.6) | 55 (22.0) | |
NVD | 79 (53.7) | 63 (61.8) | 142 (57.0) | |
CS | 21 (14.3) | 10 (9.8) | 31 (12.4) | |
NVD+CS | 12 (8.2) | 9 (8.8) | 21 (8.4) | |
Abortion history | 45 (30.6) | 25 (24.5) | 70 (28.1) | 0.621 |
History of infertility | 22 (15.0) | 10 (9.8) | 32 (12.8) | 0.254 |
History of infection (last year) | 0.229 | |||
Yes | 66 (44.9) | 38 (37.3) | 104 (41.8) | |
No | 81 (55.1) | 64 (62.7) | 145 (58.2) | |
Symptoms | 0.67 | |||
Yes | 103 (70.1) | 74 (72.5) | 177 (71.0) | |
No | 44 (29.0) | 28 (27.5) | 72 (29.0) | |
Discharge | 56 (38.1) | 34 (33.3) | 90 (36.1) | 0.442 |
Burning | 42 (28.6) | 28 (27.5) | 70 (28.1) | 0.847 |
Itching | 40 (27.2) | 22 (21.6) | 62 (25.0) | 0.311 |
Lower abdominal pain | 42 (28.6) | 28 (27.5) | 70 (28.1) | 0.847 |
Dyspareunia | 42 (28.6) | 16 (15.7) | 58 (23.3) | 0.018 |
Atypical hemorrhage | 15 (10.2) | 9 (8.8) | 24 (9.6) | 0.717 |
Contraception (OCP) | 18 (12.2) | 10 (9.8) | 28 (11.2) | 0.815 |
IUD | 1 (0.7) | 3 (2.9) | 0.30 | |
Condom | 19 (12.9) | 15 (14.7) | 4 (1.6) | 0.675 |
Injection | 3 (2.0) | 3 (2.9) | 34 (13.6) | 0.680 |
Tubectomy | 4 (2.7) | 4 (3.9) | 0.707 | |
Other methods | 65 (44.2) | 41 (40.2) | 6 (2.4) | 0.903 |
Non-contraceptive | 33 (23.8) | 23 (22.5) | 8 (3.2) | 0.795 |
Sexually active | 106 (42.6) | 102 (41.0) | 106 (42.6) | 0.183 |
Intercourse (on the week) | ||||
< 1 | 96 (65.3) | 67 (65.7) | 56 (22.5) | 0.671 |
1 - 3 | 50 (34.0) | 31 (30.4) | 208 (83.5) | 0.065 |
> 3 | 1 (0.7) | 4 (3.9) | 0.083 | |
Antibiotics (last 4 weeks) | 12 (8.2) | 11 (10.8) | 163 (65.4) | 0.339 |
Our results demonstrated that the prevalence of infection was higher among housewives with secondary education. It was observed that 32.4% of study subjects lived in a rural area, and there was no significant difference between the location of residence and the prevalence of VVC (P = 0.8).
The results of comparing clinical symptoms (e.g., burning, itching, lower abdominal pain, dyspareunia, atypical hemorrhage) with the incidence of Vulvovaginal candidiasis (VVC) candidiasis demonstrated that there was no significant relationship between clinical symptoms and the infection (P < 0.05). Among the clinical presentations, dyspareunia showed a significant difference (P = 0.01). Moreover, discharge was more frequent (33.3%) in Candida-positive cultures than other symptoms.
No significant difference was observed between the frequencies of Candida infection and all variables considered as risk factors, including contraceptive methods, having sexual intercourse (on the week), and antibiotic use in the previous month (P < 0.05).
4.1. Artificial Neural Network to Predict Model
We examined the neural network results with two neurons in the hidden layer to determine the best function to connect different layers of the neural network among the different functions in the connecting input, hidden, and output layers (Figure 1A). Nevertheless, as can be seen, the neural network with hyperbolic tangent function in the hidden and input layer connection and sigmoid function in the hidden and output layer connection had the least mean square error in both the training and validation sets. Moreover, as shown in Figure 1B, increasing the number of hidden layers into eight neurons improved the network's performance. Therefore, the neural network with eight neurons in the hidden layer and the hyperbolic tangent function for connecting the input and hidden layers, and the sigmoid function for connecting the hidden and output layers with 0.191 and 0.160 total mean square errors were optimal in training and validation sets, respectively (Figure 1). Figure 2 illustrates that this determined the specificity, sensitivity, and area under the curve values 76.2%, 79.3%, and 80.1% for predicting vulvovaginal candidiasis by an optimal neural network. Among the variables studied, age, miscarriage, dyspareunia, education, natural vaginal delivery (NVD), and lower abdominal pain with 17.02, 11.14, 8.55, 6.94, 5.70, 5.61, and 5.27 percent, were essential variables in the fit of the optimal model (Figure 3).
(A) The correct classification percentage of different ANN models in both training and testing phases for different transfer functions; (B) The correct classification percentage of selected ANN transfer function models in both training and testing phases for different hidden neurons.
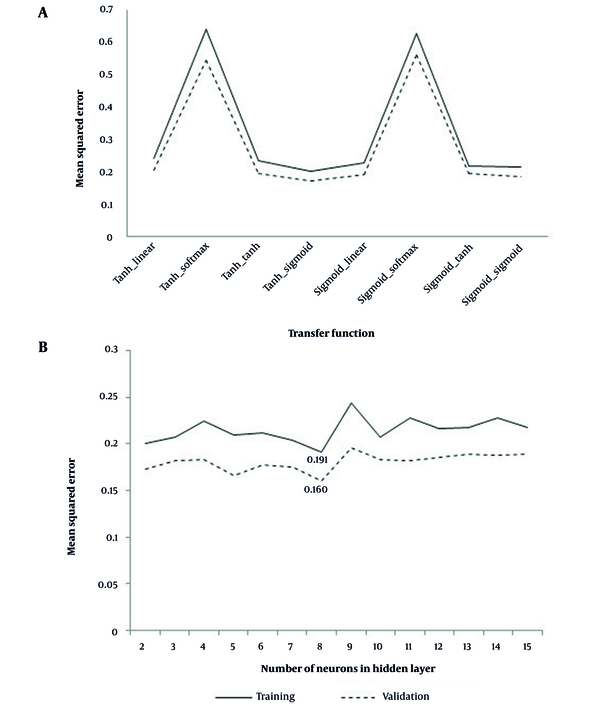
Roc curve of the ANN model
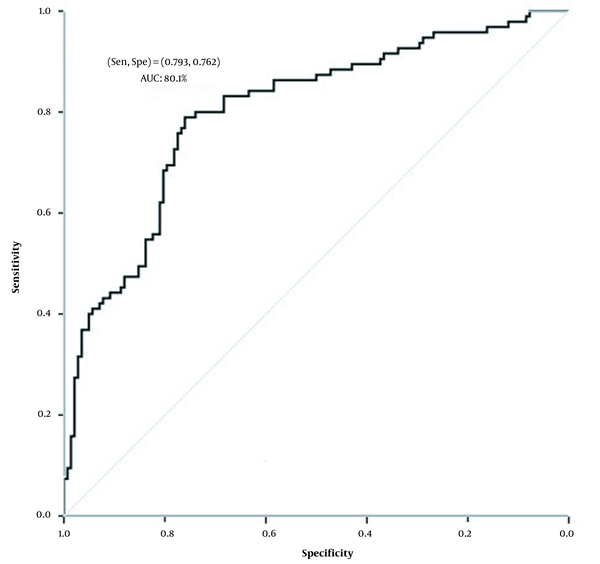
The variable importance of the selected artificial neural network.
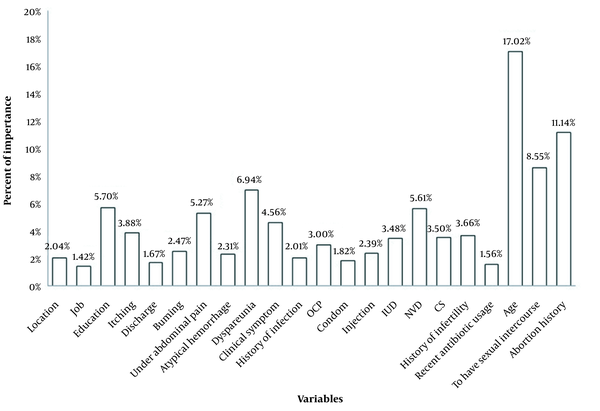
5. Discussion
Vulvovaginal candidiasis (VVC) is reported as one of the most worldwide mucosal infections of the genital tract of women (19). Several studies on the prevalence of candidiasis among fertile women demonstrated that the distribution of isolated Candida species varies among different countries. This greatly depends on risk factors such as age, hygienic habits, disease history, and social-cultural customs (20, 21). This study is the first to investigate the prevalence of vaginal candidiasis among women in Birjand, Iran.
In our study, the prevalence of VVC was 41.0% in urban and rural populations in Birjand. A similar study conducted in other cities of Iran reported the prevalence of the infection as Zahedan (47.14%), Shahrekord (32.8%), Shiraz (35%), and Kashan (45%), respectively (2, 22-24). In another study conducted by Rezaie et al., among 862 patients with suspected vaginitis, 72 vaginal candidiasis and 104 cases of bacterial vaginitis were detected (25). In this study, the most common species isolated was C. albicans, followed by C. paarapecilosis, C. glabrate, and C. krusei. Similar studies demonstrated that a proportion of VVC attributed to non-albicans Candida species was related to C. glabrate.
This study used statistical methods to predict the relationship between risk factors and clinical symptoms in predicting VVC infection. Using the ANN model, our study demonstrated that age, abortion history, sexual intercourse frequency, dyspareunia, education, NVD, and lower abdominal pain were the main predictors of VVC. According to ROC analysis, the specificity and sensitivity were 76.2% and 79.3%, respectively, to predict vulvovaginal candidiasis by the optimal neural network. Moreover, the descriptive statistics (chi-square test and t-test) revealed no significant correlation between VVC infection clinical symptoms and risk factors.
However, ANN contains parameters that may not be considered significant, limiting the use of conventional methods. This was demonstrated because age, abortion history, number of sexual intercourse, dyspareunia, education, NVD, and lower abdominal pain were not significant in descriptive statistical analysis.
Previous reports revealed that increased risk for VVC might be associated with several factors, such as patients' sociodemographic and clinical symptoms, previous genital tract infections, diabetes, and previous/current antibiotic therapy (6). In this study, we investigated the ANN model in predicting VVC about some demographic factors and clinical symptoms (age, abortion history, number of sexual intercourse, dyspareunia, etc.). Our results indicated that important variables to predict VVC infection are age, abortion history, number of sexual intercourse, dyspareunia, education, NVD, and lower abdominal pain.
Previous studies assessing the vulvovaginal prevalence among fertile women demonstrated that it greatly depends on factors including age, personal hygiene, underlying diseases, and social-cultural customs (3-5). The distribution of VVC infection frequencies according to age, living area, education, and job showed that older women and primary to secondary education and homemakers had higher infection rates. In agreement with other studies, C. albicans was predominantly isolated from women of childbearing age. Indeed, the deposition of glycogen due to the high estrogen levels in the vagina's epithelium can lead to the extreme growth of C. albicans (6, 9, 26).
Based on the literature, the most common symptoms observed in women with VVC include vaginal discharge, itching, and burning (1). Recently, in a study conducted by Akbarzadeh et al. on 280 patients, 105 were diagnosed with VVC, and the most common clinical symptom was vaginal discharge (88.6%) (27). They observed no significant relationship between the symptoms, infection rate, and risk factors. In another study by Naji et al., on 232 patients referred to a gynecological specialist, 105 were infected with VVC. The most common Candida species were C. albicans and C. glabrata; itching was the most common clinical presentation (82.9%) (28).
In 2013, a study by Saddek et al. predicted important bacterial species in nosocomial infections using the results obtained from factor analysis by a neural network analysis system (29). In 2015, another study by Chen et al. demonstrated that the ANN model with variables including age, antibiotics use, serum albumin concentrations, radiotherapy, surgery, hemoglobin, and hospitalization duration could predict deep fungal infection in lung cancer (30).
In this regard, our results demonstrated that conventional statistical methods did not correlate the number of risk factors with the occurrence of VVC. However, variables such as abortion history, number of sexual intercourses, dyspareunia, education, natural vaginal delivery (NVD), and lower abdominal pain included in our ANN model had significant differences (P < 0.05).
One advantage of the current study was using the ANN model, which does not require any pre-requisites. Other advantages include: (i) it was a model that simultaneously considered the effect of variables, (ii) compared to other models, it did not require restrictive assumptions such as normality.
One limitation of the present study was that the investigated variables only included clinical presentations and demographic information. Therefore, other factors, such as underlying diseases, diabetes, and hypothyroidism, should be included in future studies.
5.1. Conclusions
The result of the ANN model study risk factors for infection and clinical symptoms by a system of neural networks. So, it is allowed us to predict the effective factors in the occurrence of infection by considering the simultaneous effect of variables. Therefore, age, abortion history, number of sexual intercourse, dyspareunia, education, natural vaginal delivery (NVD), and lower abdominal efficiently predict the vulvovaginitis candidiasis infection in a suspicious subject in our study.
Acknowledgements
References
-
1.
Ahmad A, Khan AU. Prevalence of Candida species and potential risk factors for vulvovaginal candidiasis in Aligarh, India. Eur J Obstet Gynecol Reprod Biol. 2009;144(1):68-71. [PubMed ID: 19261369]. https://doi.org/10.1016/j.ejogrb.2008.12.020.
-
2.
Fouladi B, Yadegari MH, Rajabibazl M, Fazaeli A, Hashemzadeh Chaleshtori M. [Identification of Candida Species in Patients with Vulvovaginitis Presenting Different Clinical Symptoms]. J Adv Med Biomed Res. 2015;23(98):53-67. Persian.
-
3.
Denning DW, Kneale M, Sobel JD, Rautemaa-Richardson R. Global burden of recurrent vulvovaginal candidiasis: a systematic review. Lancet Infect Dis. 2018;18(11):e339-47. [PubMed ID: 30078662]. https://doi.org/10.1016/S1473-3099(18)30103-8.
-
4.
Zeng X, Zhang Y, Zhang T, Xue Y, Xu H, An R. Risk Factors of Vulvovaginal Candidiasis among Women of Reproductive Age in Xi'an: A Cross-Sectional Study. Biomed Res Int. 2018;2018:9703754. [PubMed ID: 29977925]. [PubMed Central ID: PMC6011108]. https://doi.org/10.1155/2018/9703754.
-
5.
Grigoriou O, Baka S, Makrakis E, Hassiakos D, Kapparos G, Kouskouni E. Prevalence of clinical vaginal candidiasis in a university hospital and possible risk factors. Eur J Obstet Gynecol Reprod Biol. 2006;126(1):121-5. [PubMed ID: 16256258]. https://doi.org/10.1016/j.ejogrb.2005.09.015.
-
6.
Ghaddar N, El Roz A, Ghssein G, Ibrahim JN. Emergence of Vulvovaginal Candidiasis among Lebanese Pregnant Women: Prevalence, Risk Factors, and Species Distribution. Infect Dis Obstet Gynecol. 2019;2019:5016810. [PubMed ID: 31467477]. [PubMed Central ID: PMC6699268]. https://doi.org/10.1155/2019/5016810.
-
7.
Konadu DG, Owusu-Ofori A, Yidana Z, Boadu F, Iddrisu LF, Adu-Gyasi D, et al. Prevalence of vulvovaginal candidiasis, bacterial vaginosis and trichomoniasis in pregnant women attending antenatal clinic in the middle belt of Ghana. BMC Pregnancy Childbirth. 2019;19(1):341. [PubMed ID: 31547803]. [PubMed Central ID: PMC6757405]. https://doi.org/10.1186/s12884-019-2488-z.
-
8.
Roudbary M, Roudbarmohammadi S, Bakhshi B, Farhadi Z, Nikoomanesh F. Identification of Candida species isolated from Iranian women with vaginal candidiasis by PCR-RFLP method. Eur J Exp Biol. 2013;3(6):365-9.
-
9.
Nasrollahi Z, Yadegari MH, Roudbar Mohammadi S, Roudbary M, Hosseini Poor M, Nikoomanesh F, et al. Fluconazole Resistance Candida albicans in Females With Recurrent Vaginitis and Pir1 Overexpression. Jundishapur J Microbiol. 2015;8(9). e21468. [PubMed ID: 26495107]. [PubMed Central ID: PMC4609386]. https://doi.org/10.5812/jjm.21468.
-
10.
Ghaddar N, Anastasiadis E, Halimeh R, Ghaddar A, Dhar R, AlFouzan W, et al. Prevalence and antifungal susceptibility of Candida albicans causing vaginal discharge among pregnant women in Lebanon. BMC Infect Dis. 2020;20(1):32. [PubMed ID: 31931738]. [PubMed Central ID: PMC6958632]. https://doi.org/10.1186/s12879-019-4736-2.
-
11.
Fan SR, Liu XP, Li JW. Clinical characteristics of vulvovaginal candidiasis and antifungal susceptibilities of Candida species isolates among patients in southern China from 2003 to 2006. J Obstet Gynaecol Res. 2008;34(4):561-6. [PubMed ID: 18937710]. https://doi.org/10.1111/j.1447-0756.2008.00817.x.
-
12.
Feng S, Li L, Cen L, Huang J. Using MLP networks to design a production scheduling system. Comput Oper Res. 2003;30(6):821-32. https://doi.org/10.1016/s0305-0548(02)00044-8.
-
13.
Betiku E, Ajala SO. Modeling and optimization of Thevetia peruviana (yellow oleander) oil biodiesel synthesis via Musa paradisiacal (plantain) peels as heterogeneous base catalyst: A case of artificial neural network vs. response surface methodology. Ind Crops Prod. 2014;53:314-22. https://doi.org/10.1016/j.indcrop.2013.12.046.
-
14.
Nourouzi MM, Chuah TG, Choong TS. Optimisation of reactive dye removal by sequential electrocoagulation-flocculation method: comparing ANN and RSM prediction. Water Sci Technol. 2011;63(5):984-94. [PubMed ID: 21411950]. https://doi.org/10.2166/wst.2011.280.
-
15.
Sundström A. Using the Rating Scale Model to Examine the Psychometric Properties of the Self-Efficacy Scale for Driver Competence. Eur J Psychol Assess. 2011;27(3):164-70. https://doi.org/10.1027/1015-5759/a000063.
-
16.
Ghaedi AM, Vafaei A. Applications of artificial neural networks for adsorption removal of dyes from aqueous solution: A review. Adv Colloid Interface Sci. 2017;245:20-39. [PubMed ID: 28473053]. https://doi.org/10.1016/j.cis.2017.04.015.
-
17.
Gardner JW, Craven M, Dow C, Hines EL. The prediction of bacteria type and culture growth phase by an electronic nose with a multi-layer perceptron network. Meas Sci Technol. 1998;9(1):120-7. https://doi.org/10.1088/0957-0233/9/1/016.
-
18.
Zhang Z, Barkoula NM, Karger-Kocsis J, Friedrich K. Artificial neural network predictions on erosive wear of polymers. Wear. 2003;255(1-6):708-13. https://doi.org/10.1016/s0043-1648(03)00149-2.
-
19.
Alfouzan W, Dhar R, Ashkanani H, Gupta M, Rachel C, Khan ZU. Species spectrum and antifungal susceptibility profile of vaginal isolates of Candida in Kuwait. J Mycol Med. 2015;25(1):23-8. [PubMed ID: 25534676]. https://doi.org/10.1016/j.mycmed.2014.10.021.
-
20.
Guan WJ, Ni ZY, Hu Y, Liang WH, Ou CQ, He JX, et al. Clinical Characteristics of Coronavirus Disease 2019 in China. N Engl J Med. 2020;382(18):1708-20. [PubMed ID: 32109013]. [PubMed Central ID: PMC7092819]. https://doi.org/10.1056/NEJMoa2002032.
-
21.
Guzel AB, Ilkit M, Burgut R, Urunsak IF, Ozgunen FT. An evaluation of risk factors in pregnant women with Candida vaginitis and the diagnostic value of simultaneous vaginal and rectal sampling. Mycopathologia. 2011;172(1):25-36. [PubMed ID: 21293929]. https://doi.org/10.1007/s11046-011-9392-z.
-
22.
Dehghan P, Kharazi M, Yazdani M, Zomorodian K, Chadeganipour M, Akbari M. [Diagnosis of Candida species isolated from patients with vaginal candidiasis and healthy individuals based on clinical symptoms and paraclinical evidences]. J Isfahan Med Sch. 2012;30(209):1662-9. Persian.
-
23.
Ghajari A, Lotfali E, Ahmadi NA, Nazer Fassihi P, Shahmohammadi N, Ansari S, et al. Isolation of Different Species of Candida in Patients with Vulvovaginal Candidiasis from Damavand, Iran. Arch Clin Infect Dis. 2018;13(6). https://doi.org/10.5812/archcid.59291.
-
24.
Habibian R, Jafarzadeh L, Shahriari K. Investigating the relationship between recurrent candidiasis with predisposing factors and symptoms of disease. J Shahrekord Univ Med Sci. 2013;15(5).
-
25.
Rezaei H, Foroughi-Parvar F, Maghsood AH, Fallah M, Saidijam M, Matini M. Prevalence of bacterial vaginosis and vaginal candidiasis in women referred to health centers of Hamadan City, West of Iran, 2014. Pars Jahrom Univ Med Sci. 2017;15(2):17-23. https://doi.org/10.29252/jmj.15.2.17.
-
26.
Adams ST, Leveson SH. Clinical prediction rules. BMJ. 2012;344:d8312. [PubMed ID: 22250218]. https://doi.org/10.1136/bmj.d8312.
-
27.
Akbarzadeh M, Bonyadpoure B, Pacshir K, Mohagheghzadeh A. [Causes and clinical symptoms of vaginal candidiasis in patients referring to selective clinics of Shiraz University of Medical Sciences (2009)]. J Arak Univ Med Sci. 2010;13(3):12-20. Persian.
-
28.
Naji S, Diba K, Javanmard N, Yoosefzadeh R. Interspecies differences of Candida species causing recurrent vulvovaginal candidiasis in response to fluconazole treatment. Curr Med Mycol. 2018;4.
-
29.
Saddek B, Hacen B, Fateh A, Chara B, Mustapha B. Artificial Neural Networks In Prevention of Nosocomials Infections. Int J Sci Technol Res. 2013;2(10).
-
30.
Chen J, Chen J, Ding HY, Pan QS, Hong WD, Xu G, et al. Use of an Artificial Neural Network to Construct a Model of Predicting Deep Fungal Infection in Lung Cancer Patients. Asian Pac J Cancer Prev. 2015;16(12):5095-9. [PubMed ID: 26163648]. https://doi.org/10.7314/apjcp.2015.16.12.5095.