Abstract
Background:
Today, the COVID-19 pandemic is ever-increasingly challenging healthcare systems globally with many uncertainties and ambiguities regarding disease behavior and outcome prediction. Thus, machine learning (ML) algorithms could be potentially demanding to tackle these challenges.Objectives:
The present study aimed to construct and compare two prediction models based on statistical and computational ML algorithms to predict mortality in COVID-19 hospitalized patients and, finally, adopt the best-performing algorithm, accordingly.Methods:
Having considered a single-center registry, we scrutinized 482 records of laboratory-confirmed COVID-19 hospitalized patients admitted from February 9, 2020, to December 20, 2020. The most important clinical parameters for COVID-19 mortality prediction were identified using the Phi coefficient technique. In the next step, two statistical and computational ML models, ie, logistic regression (LR) and artificial neural network (ANN), were evaluated through the metrics derived from the confusion matrix.Results:
Predictive models were trained using 16 validated features. The results indicated that the best performance pertained to the ANN classifier with a positive predictive value (PPV) of 0.96, a negative predictive value (NPV) of 0.86, the sensitivity of 0.94, specificity of 0.94, and accuracy of 0.93.Conclusions:
According to the results, ANN predicted mortality in hospitalized patients with COVID-19 with an acceptable level of accuracy. Therefore, it would be extremely reasonable to develop intelligent decision support systems to early detect high-risk patients, helping clinicians come up with proper interventions.Keywords
COVID‐19 Coronavirus Machine Learning Artificial Intelligence Neural Network Hospital Mortality
1. Background
In December 2019, the novel coronavirus disease (COVID-19), detected initially in Wuhan China, rapidly spread all over the world. As a result, the world health organization (WHO) declared this outbreak a pandemic in January 2020 (1, 2). Unfortunately, the aggressive dissemination of this contagious viral infection resulted in major global challenges such as the shortage of hospital resources and exhaustion of healthcare providers, which necessitates developing accurate prediction models to both effectively triage patients and also maximize the use of limited resources. Besides, healthcare administrators and clinicians count on different computational and statistical models to effectively and accurately predict uncertainties in hospital and medical settings (3-5).
The clinical outcomes of the novel virus range from asymptomatic or mild symptoms to serious complications and even death in some cases. For example, in some patients with a set of mild to moderate symptoms, a severe form of the disease emerges, which requires advanced risk, stratification models. Using prediction models, professionals promptly identify patients in the early stages of the disease, which, in turn, minimizes the mortality rate (6, 7).
Several different statistical and computational methods can be used to develop a mortality prediction model, including but not limited to logistic regression (LR), linear regression, Cox regression, and machine learning (ML) algorithms (8, 9). To diminish pandemic-related challenges, health care providers worldwide apply ML, and statistical models to early predict COVID-19 deterioration (7, 10, 11). Machine learning, a branch of Artificial Intelligence (AI), facilitates extracting high-quality prediction models from the mining of huge raw datasets (12) and serves as a valuable tool in medical research to improve predictive modeling and reveal new contributing factors to a specific outcome (12, 13). In addition, ML algorithms may minimize uncertainties and ambiguities by offering evidence-based medicine for risk analysis, screening, prediction, and care plans; they also support reliable clinical decision-making through health care quality improvement (14, 15). In this regard, supervised classification of learning algorithms (both statistical and computational) can be utilized to address these uncertainties by providing patient risk stratification for tailored clinical decision-making support (ie, measuring the probability of a disease, assessing disease likelihood, forecasting disease spread, and predicting fatality) (8, 9, 16-18).
Machine learning algorithms, which analyze the inputs and outputs of complex processes, can provide prediction models that perform better than traditional ones. Also, they can detect nonlinear connections, improve multifactor algorithms, and upgrade methodologies for model validation (19, 20). Machine learning-based prediction models have successfully achieved early detection of disease trends and outbreaks, forecasting of disease behaviors and outcomes, and estimation of patient survival and patients’ responses to supportive therapies during the pandemic (19, 21). In this regard, with the onset of the current pandemic, researchers used ML to design and improve models for predicting poor disease outcomes and early detection of patient condition deterioration (such as ICU admission, mechanical intubation, or even death). Hence, these models greatly helped frontline therapists and decision-makers opt for the most effective supportive and therapeutic plans at the right time and place (15, 22, 23).
2. Objectives
Since different laboratory, clinical, and epidemiological features affect the survival or death of COVID-19 patients, it is important to understand these factors to reduce the mortality rate to a greater extent (24). Besides, the large volume of multidimensional data may perplex therapists in identifying the most important factors (25). Therefore, ML algorithms ease developing prediction models due to their numerous feature selection and classification capabilities (13, 26). Therefore, we aimed to develop and compare two statistical and computational ML methods for predicting mortality among hospitalized patients with COVID-19.
3. Methods
This retrospective, single-center study aimed to develop a prediction model based on two ML algorithms for mortality risk classification in hospitalized COVID-19 patients. It was conducted in four stages as follows: 1- data understanding, 2- patient selection, 3- preprocessing and data reduction, and 4- model development and evaluation.
3.1. Data Understanding
In this retrospective study, a COVID-19 hospital-based registry database from Imam Khomeini hospital, Ilam city, west of Iran, was thoroughly reviewed. Needless to say, the study was approved by the ethical committee board of Ilam University of Medical Sciences (Ethics code: IR.MEDILAM.REC.1399.294). Plus, only the patients admitted from January 9, 2020 to January 20, 2021 with a positive RT-PCR test for COVID-19, who met our inclusion criteria, were included. Then three health information management (HIM) experts quantitatively and qualitatively investigated case records based on 54 risk factors under six main classes: patients’ demographics (five variables), clinical manifestations (14 variables), comorbidities (seven variables), laboratory parameters (26 variables), treatment (one variable), and outcome (one variable). The data fields for each class have been illustrated below:
- Demographics: Gender, age, weight, height, and blood type.
- Clinical manifestations: Cough, contusion, nausea, vomiting, headache, gastrointestinal (GI) symptoms, muscular pain, chills, fever, dyspnea, loss of taste, loss of smell, runny nose, and sore throat.
- Comorbidities: Pneumonia, cardiac disease, hypertension, diabetes, smoking, alcohol addiction, and other underline diseases.
- Laboratory parameters: Creatinine, red cell count, white cell count, hematocrit, hemoglobin, platelet count, absolute lymphocyte count, absolute neutrophil count, serum levels of calcium, phosphorus, magnesium, sodium, and potassium, blood urea nitrogen (BUN), total bilirubin, aspartate aminotransferase (ASP), alanine aminotransferase (ALT), albumin, glucose, lactate dehydrogenase (LDH), activated partial thromboplastin time, prothrombin time, alkaline phosphatase, erythrocyte sedimentation rate (ESR), C-reactive protein, and hypersensitive troponin.
- Treatment: Oxygen therapy.
- Outcome: Death (yes/no).
3.2. Patient Selection
Eligible patients who matched the inclusion criteria comprised a total of 12885 cases suspected to have COVID-19, referred to Imam Khomeini hospital’s ambulatory and emergency departments (EDs), of whom 3350 cases were confirmed by RT-PCR. Based on exclusion criteria, finally, 482 records entered the study (306 and 176 of whom would survive and die, respectively). The exclusion criteria were as follows: (1) non-COVID-19 or non-hospitalized cases or patients with unknown disposition, (2) patients who were 18 years old or younger, (3) incomplete case records (missing data more than 70%), and (4) admission time before January 9, 2020 or after January 20, 2021. To ensure privacy and confidentiality of information, we promised to use unique identification codes for every patient throughout data collection and data presentation.
3.3. Preprocessing and Data Reduction
First, incomplete case records with great missing data (more than 70%) were excluded from analysis. Also, the remaining missing values were imputed using the mean or mode of each variable. Noisy and abnormal values, errors, duplicates, and meaningless data were checked by two HIM experts (M. SH and L. E), two infectious disease specialists, and a hematologist. When there were different interpretations on data preprocessing, we contacted the corresponding physician. Moreover, we initially omitted some features that were not common for model construction for better and easier analysis of the database before training the prediction models. Feature selection is characterized by the process of identifying and removing unneeded or redundant variables from the original dataset without any major loss of information. In the case of high-dimensional data, feature selection is deemed to be one of the key steps in data mining and pattern recognition. It enhances learning efficiency, increases prediction performance, and minimizes the complexity of model training via input optimization (27-29). To determine the most important factors for predicting mortality among hospitalized patients, the Phi correlation coefficient was used at P < 0.01 to specify a meaningful relationship between each input variable (mortality determinants) and output variable (death or survival) (30, 31).
3.4. Model Development and Evaluation
In the present study, two types of prediction models, including statistical BLR and computational ANN, were implemented.
Logistic regression: This model can be utilized to determine probable relationships between independent and dependent variables. Frequently, they can be used in two-valued output classes (ie, dichotomous variables), such as the relationship between several factors affecting Coronavirus disease (COVID-19: code 0 or non-COVID-19: code 1). A simple formula for this method has been shown in Equation 1, where "P" denotes the possibility of output occurrence; b0 is the intercept, and b1 is the coefficient of the input (or X in the formula), which belonged to the training dataset (32, 33).
In this study, the Forward LR method of BLR was applied to develop the prediction model in IBM SPSS Statistical software, V 25. Then its performance was assessed using two quantitative criteria, including true positive (TP), false positive (FP), false negative (FN), true negative (TN), and log-likelihood (Equation 2).
The log-likelihood function is a method for determining the underlying model’s goodness of fit using sample data. It can also be defined as the difference between the probabilities of the expected and observed outputs’ occurrences. It is, of course, necessary to emphasize that the smaller this amount is, the better the algorithm can perform. In Equation 2, P (Yi) is the probability of the observed output occurrence, associated with the (i) value that belongs to the Y dependent variable. Also, L(P, Y) is Log-likelihood, and 1- P(Yi) is the probability of non-observed output occurrence.
Artificial neural networks: Artificial neural networks (ANNs) are a set of computing algorithms that emulate the functions of biological neural networks. The components of the models are nodes, weights, and layers (input, hidden, and output layers) (34, 35). The three structural layers of this model are associated with predictor variables, weighted nodes connecting the input and output layers, and dependent variables, respectively (36). Moreover, activation methods are necessary to determine the link between different nodes in ANNs’ functional configurations that can be simulated using different mathematical functions (37).
Multilayer perceptron-ANNs (MLP-ANNs) are the simplest and most commonly used ANN architectures thanks to their structural flexibility, good representational capabilities, and a large number of trainable algorithms (28, 29). In this research, therefore, the backpropagation type of MLP-ANN configuration was applied owing to its low error rate in predicting output variables, as well as its extensive use in the literature (38). Also, we applied the tansing activation method to connect weighted nodes in ANNs. In this study, the ANN was designed in MATLAB software R 2013 to predict mortality among hospitalized patients with COVID-19, using 70% of data for ANN training and 15% for each of validating and testing the model developed. We first determined the number of inputs and outputs in each two input and hidden layers, which were associated with the number of independent and dependent variables, respectively. Subsequently, we placed one node in one hidden layer and then measured its performance based on different criteria, including sensitivity, specificity, and accuracy. In each step, we added a node and measured its performance. Then the best configuration of ANNs was selected by comparing different nodes and the number of the hidden layer(s). Finally, training and validating mean squared errors (MSE) and the area under the ROC curve (AUC) of ANN were determined as final key parameters of the prediction models. Hence, to compare the performance of the models in predicting mortality, we applied some criteria such as positive predictive value (PPV), negative predictive value (NPV), sensitivity, specificity, and accuracy, which were obtained from the confusion matrix and AUC-ROC curve.
4. Results
4.1. Patient Profiles
By applying the exclusion criteria, finally, a total of 482 eligible hospitalized COVID-19 patients were chosen as follows: 288 (59.75%) were male, and 194 (40.24%) were female. Also, the median age of the participants was 57.25 years (interquartile: 18 - 100). Moreover, 150 (31.12%) and 332 (68.87%) were hospitalized in the ICU and general wards, respectively. Regarding the outcome, 382 (79.25%) recovered, and 100 (20.74%) succumbed to the disease.
4.2. Feature Selection
Based on the Phi coefficient correlation between each death determinant and the output variable (survival (0) and (death (1)), 16 variables were recognized as the most important predictors of mortality in hospitalized COVID-19 patients at P < 0.01), as shown in Table 1.
The Most Influential Factors in Predicting Mortality in Patients with COVID-19
No. | Variables | Type of Variable | Correlation Coefficient | P-Value |
---|---|---|---|---|
1 | Cough | Qualitative (binominal) | 0.168 | 0.001 |
2 | Contusion | Qualitative (binominal) | 0.144 | 0.001 |
3 | Oxygen therapy | Qualitative (binominal) | 0.169 | < 0.001 |
4 | Length of hospitalization | Quantitative | 0.432 | < 0.001 |
5 | Dyspnea | Qualitative (binominal) | 0.119 | < 0.001 |
6 | Runny nose | Qualitative (binominal) | 0.185 | < 0.001 |
7 | Loss of taste | Qualitative (binominal) | 0.244 | < 0.001 |
8 | Loss of smell | Qualitative (binominal) | 0.248 | < 0.001 |
9 | Erythrocyte sedimentation rate | Quantitative | 0.539 | 0.001 |
10 | Pleural fluid | Qualitative (binominal) | -0.534 | < 0.001 |
11 | ICU hospitalization | Qualitative (binominal) | 0.628 | < 0.001 |
12 | Blood sodium | Quantitative | 0.307 | 0.001 |
13 | Absolute lymphocyte count | Quantitative | 0.472 | < 0.001 |
14 | Activated partial thromboplastin time | Quantitative | 0.648 | < 0.001 |
15 | Other underlying diseases | Qualitative (binominal) | 0.153 | 0.001 |
16 | Sore throat | Qualitative (binominal) | 0.121 | 0.01 |
Based on the correlation coefficient, the variables of ICU hospitalization (φ = 0.628, P < 0.001), activated partial thromboplastin time (φ = 0.648, P < 0.001), length of hospitalization (φ = 0.432, P < 0.001), pleural fluid (φ = -0.534, P < 0.01), and absolute lymphocyte count (φ = 0.472, P < 0.001) represented much higher correlation coefficients than other variables (P < 0.01).
4.3. Logistic Regression
Using the Forward LR method of BLR, five steps were taken in the prediction model. Table 2 depicts the table of the Model of IF-Removed.
The Model of IF-Removed
Model IF Term Removed | ||||
---|---|---|---|---|
Variables | Model Log-Likelihood | Change in -2 Log-Likelihood | df | P-Value of the Change |
Step 1 | ||||
ICU hospitalization | -126.159 | 93.357 | 1 | 0.000 |
Step 2 | ||||
ICU hospitalization | -101.285 | 72.725 | 1 | 0.000 |
Pleural fluid | -79.481 | 29.117 | 1 | 0.000 |
Step 3 | ||||
ICU hospitalization | -86.167 | 53.983 | 1 | 0.000 |
Pleural fluid | -75.454 | 32.558 | 1 | 0.000 |
Absolute lymphocyte count | -64.922 | 11.494 | 1 | 0.001 |
Step 4 | ||||
Length of hospitalization | -59.175 | 10.402 | 1 | 0.001 |
ICU hospitalization | -82.764 | 57.580 | 1 | 0.000 |
Pleural fluid | -72.316 | 36.684 | 1 | 0.000 |
Absolute lymphocyte count | -61.584 | 15.219 | 1 | 0.000 |
Step 5 | ||||
Length of hospitalization | -54.961 | 9.640 | 1 | 0.002 |
Loss of smell | -53.974 | 7.667 | 1 | 0.006 |
ICU hospitalization | -76.821 | 53.361 | 1 | 0.000 |
Pleural fluid | -68.117 | 35.953 | 1 | 0.000 |
Absolute lymphocyte count | -57.403 | 14.523 | 1 | 0.000 |
As it can be seen in Table 2, entering the ICU hospitalization variable into the first step resulted in a log-likelihood of -126.59, assigning this variable as the first to enter into the model. Also, by entering other important variables in next phases into the model, we demonstrated that the log-likelihood rate reduced till the final step (ie, 5th step). In this step, the log-likelihood of the loss of smell, which was entered into the model as the latest variable, was obtained -53.974. Simultaneously, other variables suggested the minimal value of log-likelihood in this step at P < 0.01 (df = 1).
Figure 1 represents the predictive power of BLR in determining the probability of survival and death in hospitalized patients with COVID-19 based on the aforementioned five-step process described in the previous section.
Steps 1 and 5 of BLR
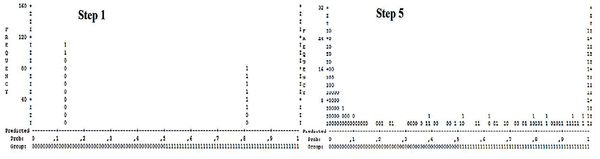
As shown in Figure 1, vertical vertices demonstrate the number of BLR’s iterations in each step for death prediction whereas horizontal vertices are possible BLR values at which the predicted cases would be categorized into certain groups. For example, a predicted probability of > 0.5 was regarded as "dead," while values less than 0.5 predicted the "survival" of hospitalized COVID-19 patients.
At the cut-off value of 0.5, the symbols of 0 and 1 represented survived, and dead cases, respectively, and each symbol equated to 10 cases of interest. Hence, we compared steps 1 and 5 of BLR and noticed that the addition of those five important variables to the BLR model caused the representative cases associated with survived hospitalized patients (0) to recede from the threshold (0.5), especially in high BLR iterations. Therefore, the difference between the probability predicted by the model and the expected rate reduced (ie, log-likelihood reduction). Also, the representative cases related to demised hospitalized patients (1) in step 5 were closer to 1 compared to their distance from the threshold in step 1 (ie, log-likelihood reduction and consequently, the model’s performance augmentation). Besides, comparing the final step of BLR with step 1 based on the TP, FP, TN, and FN values revealed that truly classified cases (TP = 133 and TN = 252) in step 5th significantly increased compared to respective values in step 1st (TP = 105 and TN = 173). However, the number of falsely classified cases was lower in step 5th (FP = 55 and FN = 43) than in step 1st (FP = 134 and FN = 71), indicating a much higher efficiency in step 5th than in step 1st regarding the correct classification of survived and dead COVID-19 hospitalized patients.
4.4. Artificial Neural Network
It should be noted that the addition of sigmoid nodes to the hidden layer(s) for ANN training and subsequently, measuring its performance showed that the architecture of 16-20-10-1 (16 input variables as the input layer, 20 and 10 sigmoid nodes in hidden layers of 1 and 2, respectively, and 1 output as the target layer) offered much better functionality compared to other architectures concerning different performance criteria.
The results of MSE measurement for ANN (Figure 2) showed that the ANN acquired the best performance at 16th training repetition (six steps before reaching the best validation (22nd epoch)). As noted, the best validation performance was achieved in 22nd training epoch (MSE = 0.037122). In this step thus, the MSE of ANN training and testing was close to the validation performance (0.01 < MSE < 0.1) (Figure 2). The error histogram for the ANN algorithm has been depicted in Figure 2.
The MSE of different states of the dataset and error histogram bar of the ANN
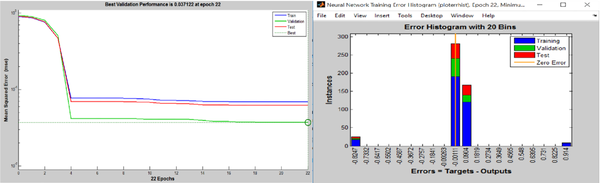
The error histogram with 20 bins was utilized to classify the training and validate and test various states of the ANN. As indicated in Figure 2, approximately 200 samples with the error rate of -0.00111 and more than 100 samples with the error rate of 0.0904 were used to train the ANN. Also, the error rate was set in these two amounts to validate and test the subset of the dataset. The presence of some outliers in the databases directed a few portions of training and testing data into 0.914 and -0.8247 points of the error histogram diagram, slightly decreasing the ANN’s performance.
4.5. Comparison of Two Selected Methods
The results of comparing the performance of two selected methods via the confusion matrix demonstrated that the ANN correctly predicted the outcome of 92.9% of hospitalized patients; however, 79% of the patients were correctly classified in the 5th step of the BLR technique. Also, the incorrectly classified samples in ANN and BLR’s 5th step included FP = 1.9% vs. 11.4% and FN = 5.2% vs. 8.9%, respectively, revealing the lower rate of incorrectly classified samples in the ANN method. The results of PPV, NPV, sensitivity, specificity, and accuracy of the ANN and BLR techniques have been presented in Figure 3.
Some performance criteria in the two selected methods
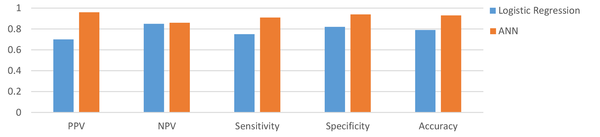
As shown in Figure 3, the ANN had a higher prediction performance than the BLR. The AUCs of the two different methods, AUC-ROC of the ANN, and the results of the confusion matrix have been shown in Figure 4.
The ANN’s confusion matrix and AUC-ROC
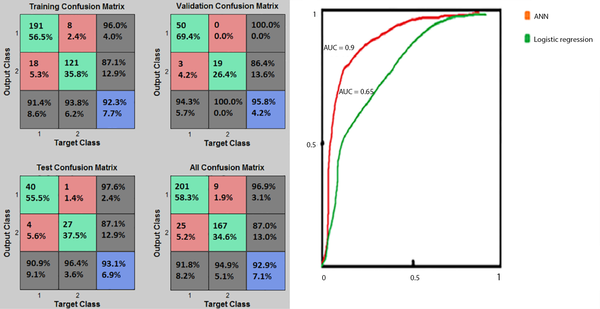
Based on Figure 4, the percentages of the cases correctly classified in the training, validation, and test phases were 92.3%, 95.8%, and 93.1%, respectively. The validation (with 95.8% of TP+TN) and training (with 92.3% of TP+TN) of the confusion matrix presented the best and poorest performances, respectively. Figure 4 also demonstrated that the ANN technique (AUC = 0.90) was better than the BLR technique (AUC = 0.65) in terms of prediction performance. Besides, the ROC diagram of the ANN was closer to the vertical axis (ie, the TP value). Overall, comparison of the two prediction models based on the confusion matrix, PPV, NPV, sensitivity, specificity, accuracy, and AUC revealed that the ANN model performed better in predicting death among hospitalized COVID-19 patients.
5. Discussion
It is believed that the early prediction of possible death due to COVID-19 based on novel, objective, and scientific techniques can help tackle the cumbersome burden of the disease on healthcare systems by effectively triaging critically ill patients and optimally managing the scarce hospital resources (15, 27). Therefore, the present study’s purpose was to retrospectively develop and validate two statistical and computational ML models based on the most relevant determinants of COVID-19 mortality. For this purpose, we applied two ML models (statistical: BLR and computational: ANN) to predict mortality among hospitalized confirmed COVID-19 patients based on the clinical data available in the registry database of Ilam University of Medical Sciences. The most important mortality predictors were determined using the Phi coefficient at P < 0.01, leading to the identification of sixteen final variables as the most important predictors.
Investigating the BLR model’s performance using the confusion matrix and log-likelihood demonstrated that in 5th step, the model’s performance was superior compared to step 1st, with TP = 133, TN = 252, FP = 55, FN = 43, and the average log-likelihood of -61.6. On the other hand, the best performance of the ANN model was obtained with the structure of 16-20-10-1 with the MSE of 0.037122, validated at the 22nd training iteration. At this point, the classification of a large number of samples delivered a near zero value, as observed in the error histogram, with TP = 281, TN = 167, FN = 9, and FP = 25 in the total confusion matrix. Comparison of the two selected algorithms regarding the confusion matrix (as a common performance criterion) demonstrated that the structure of 16-20-10-1 in ANN resulted in a better performance compared with the 5th step of BLR.
So far, several studies have evaluated the applicability of ML techniques in predicting mortality in patients with COVID-19. For instance, Karthikeyan et al. retrospectively studied the clinical data of 2779 confirmed or suspected COVID-19 patients to construct an intelligent prediction model via selected ML algorithms. Finally, the ANN model attained the best performance with an accuracy of 96% (39). Furthermore, Das et al. conducted a retrospective analysis on chest X-ray data of 3299 COVID-19 subjects and showed that the ANN model, with an accuracy of 0.981% and AUC- ROC of 0.886, claimed the best predictive ability (40). Also, Yadaw et al. assessed the performance of four ML algorithms, including LR, RF, SVM, and eXtreme Gradient Boosting (XGBoost), using a dataset of 3841 patients to predict COVID-19 mortality. In the above-mentioned model, XGBoost with an AUC of 91% and LR with AUC of 78% gained the best and worst performance among other models developed (24). Gao (2020) conducted a retrospective analysis on the data of 2520 COVID-19 hospitalized patients. The results showed that the ANN model yielded the best performance with an AUC-ROC of 0.9760 in predicting COVID-19 patients’ physiological deterioration and death compared with other models developed by LR, support vector machine (SVM), and gradient boosted decision tree (15). Vaid et al., (26) also compared the efficiency of the logistic regression with L1 regularization (LASSO) and ANN-MLP models in predicting mortality among 4029 confirmed COVID-19 patients. Ultimately, the best performance was reported for the modified ANN-MLP model with the sensitivity of 90.7%, specificity of 91.4%, and AUC-ROC of 0.963 (41). An et al. achieved the best COVID-19-related mortality predictive performance using the ANN technique with RMSE of 5.9451 and MAE of 4.6354 (41). Similarly, we observed the best performance for the ANN model with PPV of 0.96%, NPV of 0.86, sensitivity of 0.94, specificity of 0.94, and accuracy of 0.93.
Studies have also reported some important clinical variables (predictors) for COVID-19 patients’ mortality through leveraging a feature selection analysis technique. It should be noted that the features selected are regarded as inputs for developing ML-based models for predicting mortality among COVID-19 patients. Apparently, the strongest predictive variables include age (7, 19, 24, 40, 42-45), ICU hospitalization (15, 27, 40, 42), low oxygen saturation (decreased SPO2) (6, 7, 10, 19, 22, 27, 45), dyspnea (7, 10, 40, 43), loss of taste/smell, hypertension (19, 24, 27, 40, 44, 45), cardiovascular diseases (24, 27, 40, 42, 44, 46), raised ALT and/or AST (22, 24, 40, 46, 47), elevated LDH (21, 22, 40, 43), and raised leukocyte/neutrophil count (15, 22, 24, 40, 42, 44, 48). In the present study, the most important variables (COVID-19 mortality predictors) were identified based on correlation coefficients at the level of P < 0.01 (ie, feature selection). These variables included ICU hospitalization, activated partial thromboplastin time, length of hospitalization, pleural fluid, and absolute lymphocyte count. In general, these variables were relatively in line with the results of previous studies that have categorized and prioritized these parameters.
Interestingly, the selected computational ML algorithm (ie, the ANN model) could predict mortality in COVID-19 patients with acceptable performance, which, in turn, can help optimally use limited hospital resources for treating patients with more critical conditions and assist professionals to provide more qualitative care, reducing medical errors accordingly. The models proposed in this study may facilitate the early detection and effective management of COVID-19 patients, minimizing the death rate in them. Also, developing a valid anticipative model may enhance the quality of care and increase the survival rate of COVID-19 patients. Therefore, mortality prediction and risk analysis models can greatly contribute to identifying high-risk patients, followed by the adoption of the most effective and reliable support and treatment plans. Besides, quantitative, objective and evidence-based models for risk stratification, mortality prediction, and care plan development would efficiently obviate uncertainties and ambiguities. These models also offer a better strategy for clinicians to lessen disease complications and improve patient survival likelihoods.
Despite timely and accurate identification of high-risk cases, the present study faced some limitations that may have caused classification bias as follows. First, we dealt with a retrospective dataset that might lack any unfilled and imbalanced data fields. Second, this study was conducted at a single center and on merely 482 data, so the generalizability of the proposed model is subjected to certain limitations. Third, we used only two ML algorithms for clinical-based prediction analyses, and last but not least, the selected dataset lacked some important clinical variables such as radiological parameters. To sum up, the performance of our model will be enhanced if more classification techniques are tested using larger, multicenter, and prospective datasets in the future.
5.1. Conclusions
In this study, we developed and evaluated two ML-based prediction models for in-hospital mortality in COVID-19 patients using the most important clinical characteristics (16 predictors). It was observed that the ANN model performed best in terms of classification accuracy compared to the BLR algorithm. The proposed model can be suitably used to anticipate the mortality risk of hospitalized COVID-19 patients, allowing for the optimal allocation of restricted hospital resources. Also, this model could automatically identify high-risk patients as soon as they are admitted and hospitalized. To conclude, the ML algorithms coupled with qualitative and comprehensive hospital databases can be beneficial in the timely and accurate mortality risk stratification of COVID-19 patients.
Acknowledgements
References
-
1.
Peeri NC, Shrestha N, Rahman MS, Zaki R, Tan Z, Bibi S, et al. The SARS, MERS and novel coronavirus (COVID-19) epidemics, the newest and biggest global health threats: what lessons have we learned? Int J Epidemiol. 2020;49(3):717-26. [PubMed ID: 32086938]. [PubMed Central ID: PMC7197734]. https://doi.org/10.1093/ije/dyaa033.
-
2.
Shi H, Han X, Jiang N, Cao Y, Alwalid O, Gu J, et al. Radiological findings from 81 patients with COVID-19 pneumonia in Wuhan, China: a descriptive study. Lancet Infect Dis. 2020;20(4):425-34. https://doi.org/10.1016/s1473-3099(20)30086-4.
-
3.
Lai CC, Shih TP, Ko WC, Tang HJ, Hsueh PR. Severe acute respiratory syndrome coronavirus 2 (SARS-CoV-2) and coronavirus disease-2019 (COVID-19): The epidemic and the challenges. Int J Antimicrob Agents. 2020;55(3):105924. [PubMed ID: 32081636]. [PubMed Central ID: PMC7127800]. https://doi.org/10.1016/j.ijantimicag.2020.105924.
-
4.
Hussain A, Bhowmik B, do Vale Moreira NC. COVID-19 and diabetes: Knowledge in progress. Diabetes Res Clin Pract. 2020;162:108142. [PubMed ID: 32278764]. [PubMed Central ID: PMC7144611]. https://doi.org/10.1016/j.diabres.2020.108142.
-
5.
Moujaess E, Kourie HR, Ghosn M. Cancer patients and research during COVID-19 pandemic: A systematic review of current evidence. Crit Rev Oncol Hematol. 2020;150:102972. [PubMed ID: 32344317]. [PubMed Central ID: PMC7174983]. https://doi.org/10.1016/j.critrevonc.2020.102972.
-
6.
Zhao Z, Chen A, Hou W, Graham JM, Li H, Richman PS, et al. Prediction model and risk scores of ICU admission and mortality in COVID-19. PLoS One. 2020;15(7). e0236618. [PubMed ID: 32730358]. [PubMed Central ID: PMC7392248]. https://doi.org/10.1371/journal.pone.0236618.
-
7.
Hu H, Yao N, Qiu Y. Comparing Rapid Scoring Systems in Mortality Prediction of Critically Ill Patients With Novel Coronavirus Disease. Acad Emerg Med. 2020;27(6):461-8. [PubMed ID: 32311790]. [PubMed Central ID: PMC7264631]. https://doi.org/10.1111/acem.13992.
-
8.
Singh H, Dhar J. Mathematical Population Dynamics and Epidemiology in Temporal and Spatio-Temporal Domains. CRC Press; 2018.
-
9.
Bhandari S, Shaktawat AS, Tak A, Patel B, Shukla J, Singhal S, et al. Logistic regression analysis to predict mortality risk in COVID-19 patients from routine hematologic parameters. Ibnosina J Med Biomed Sci. 2020;12(2):123.
-
10.
Yan L, Zhang H, Goncalves J, Xiao Y, Wang M, Guo Y, et al. An interpretable mortality prediction model for COVID-19 patients. Nat Mach. 2020;2(5):1-6. https://doi.org/10.1038/s42256-020-0180-7.
-
11.
Malki Z, Atlam ES, Hassanien AE, Dagnew G, Elhosseini MA, Gad I. Association between weather data and COVID-19 pandemic predicting mortality rate: Machine learning approaches. Chaos Solitons Fractals. 2020;138:110137. [PubMed ID: 32834583]. [PubMed Central ID: PMC7367008]. https://doi.org/10.1016/j.chaos.2020.110137.
-
12.
Shanbehzadeh M, Nopour R, Kazemi-Arpanahi H. Comparison of Four Data Mining Algorithms for Predicting Colorectal Cancer Risk. J Adv Med Biomed. 2021;29(133):100-8. https://doi.org/10.30699/jambs.29.133.100.
-
13.
Hernandez-Suarez DF, Ranka S, Kim Y, Latib A, Wiley J, Lopez-Candales A, et al. Machine-Learning-Based In-Hospital Mortality Prediction for Transcatheter Mitral Valve Repair in the United States. Cardiovasc Revasc Med. 2021;22:22-8. [PubMed ID: 32591310]. [PubMed Central ID: PMC7736498]. https://doi.org/10.1016/j.carrev.2020.06.017.
-
14.
Shipe ME, Deppen SA, Farjah F, Grogan EL. Developing prediction models for clinical use using logistic regression: an overview. J Thorac Dis. 2019;11(Suppl 4):S574-84. [PubMed ID: 31032076]. [PubMed Central ID: PMC6465431]. https://doi.org/10.21037/jtd.2019.01.25.
-
15.
Gao Y, Cai GY, Fang W, Li HY, Wang SY, Chen L, et al. Machine learning based early warning system enables accurate mortality risk prediction for COVID-19. Nat Commun. 2020;11(1):1-10. [PubMed ID: 33024092]. [PubMed Central ID: PMC7538910]. https://doi.org/10.1038/s41467-020-18684-2.
-
16.
Medina-Mendieta JF, Cortes-Cortes M, Cortes-Iglesias M. COVID-19 Forecasts for Cuba Using Logistic Regression and Gompertz Curves. MEDICC Rev. 2020;22(3):32-9. [PubMed ID: 32812897]. https://doi.org/10.37757/MR2020.V22.N3.8.
-
17.
Xu K, Zhou M, Yang D, Ling Y, Liu K, Bai T, et al. Application of ordinal logistic regression analysis to identify the determinants of illness severity of COVID-19 in China. Epidemiol Infect. 2020;148. e146. [PubMed ID: 32631458]. [PubMed Central ID: PMC7369341]. https://doi.org/10.1017/S0950268820001533.
-
18.
Almeshal AM, Almazrouee AI, Alenizi MR, Alhajeri SN. Forecasting the Spread of COVID-19 in Kuwait Using Compartmental and Logistic Regression Models. Appl Sci. 2020;10(10). https://doi.org/10.3390/app10103402.
-
19.
Assaf D, Gutman Y, Neuman Y, Segal G, Amit S, Gefen-Halevi S, et al. Utilization of machine-learning models to accurately predict the risk for critical COVID-19. Intern Emerg Med. 2020;15(8):1435-43. [PubMed ID: 32812204]. [PubMed Central ID: PMC7433773]. https://doi.org/10.1007/s11739-020-02475-0.
-
20.
Brownlee J. Data preparation for machine learning: data cleaning, feature selection, and data transforms in Python. Machine Learning Mastery; 2020.
-
21.
Agieb R. Machine Learning Models for the Prediction the Necessity of Resorting to ICU of COVID-19 Patients. Int J Adv Trends Comput Sci Engineer. 2020;9(5):6980-4. https://doi.org/10.30534/ijatcse/2020/15952020.
-
22.
Booth AL, Abels E, McCaffrey P. Development of a prognostic model for mortality in COVID-19 infection using machine learning. Mod Pathol. 2020;34(3):1-10. [PubMed ID: 33067522]. [PubMed Central ID: PMC7567420]. https://doi.org/10.1038/s41379-020-00700-x.
-
23.
Parchure P, Joshi H, Dharmarajan K, Freeman R, Reich DL, Mazumdar M, et al. Development and validation of a machine learning-based prediction model for near-term in-hospital mortality among patients with COVID-19. BMJ Support Palliat Care. 2020. [PubMed ID: 32963059]. [PubMed Central ID: PMC8049537]. https://doi.org/10.1136/bmjspcare-2020-002602.
-
24.
Yadaw AS, Li Y, Bose S, Iyengar R, Bunyavanich S, Pandey G. Clinical features of COVID-19 mortality: development and validation of a clinical prediction model. Lancet Digit Health. 2020;2(10):e516-25. https://doi.org/10.1016/s2589-7500(20)30217-x.
-
25.
Chen Y, Linli Z, Lei Y, Yang Y, Liu Z, Xia Y, et al. Risk factors for mortality in critically ill patients with COVID‐19 in Huanggang, China: A single‐center multivariate pattern analysis. J Med Virol. 2020. https://doi.org/10.21203/rs.3.rs-29429/v1.
-
26.
Vaid A, Jaladanki SK, Xu J, Teng S, Kumar A, Lee S, et al. Federated Learning of Electronic Health Records to Improve Mortality Prediction in Hospitalized Patients With COVID-19: Machine Learning Approach. JMIR Med Inform. 2021;9(1). e24207. [PubMed ID: 33400679]. [PubMed Central ID: PMC7842859]. https://doi.org/10.2196/24207.
-
27.
Pan P, Li Y, Xiao Y, Han B, Su L, Su M, et al. Prognostic Assessment of COVID-19 in the Intensive Care Unit by Machine Learning Methods: Model Development and Validation. J Med Internet Res. 2020;22(11). e23128. [PubMed ID: 33035175]. [PubMed Central ID: PMC7661105]. https://doi.org/10.2196/23128.
-
28.
Sun L, Mo Z, Yan F, Xia L, Shan F, Ding Z, et al. Adaptive Feature Selection Guided Deep Forest for COVID-19 Classification With Chest CT. IEEE J Biomed Health Inform. 2020;24(10):2798-805. [PubMed ID: 32845849]. https://doi.org/10.1109/JBHI.2020.3019505.
-
29.
Abdullah A, Ramya C, Priyadharsini V, Reshma C, Selvakumar S. A survey on evolutionary techniques for feature selection. 2017 Conference on Emerging Devices and Smart Systems (ICEDSS). IEEE; 2017. p. 58-62.
-
30.
Kalgotra P, Sharda R, Luse A. Which similarity measure to use in network analysis: Impact of sample size on phi correlation coefficient and Ochiai index. Int J Inf Manage. 2020;55. https://doi.org/10.1016/j.ijinfomgt.2020.102229.
-
31.
Trafimow DAVID. Comparing the descriptive characteristics of the adjusted success rate to the phi coefficient, the odds ratio, and the difference between conditional proportions. Int J Stat Adv Theory Appl. 2017;1(1):1-19.
-
32.
Shah K, Patel H, Sanghvi D, Shah M. A comparative analysis of logistic regression, random forest and KNN models for the text classification. Augment Hum Res. 2020;5(1):1-16.
-
33.
Asenso Barnieh B, Jia L, Menenti M, Jiang M, Zhou J, Zeng Y, et al. Modeling the Underlying Drivers of Natural Vegetation Occurrence in West Africa with Binary Logistic Regression Method. Sustainability. 2021;13(9):4673. https://doi.org/10.3390/su13094673.
-
34.
Gholipour C, Rahim F, Fakhree A, Ziapour B. Using an Artificial Neural Networks (ANNs) Model for Prediction of Intensive Care Unit (ICU) Outcome and Length of Stay at Hospital in Traumatic Patients. J Clin Diagn Res. 2015;9(4):OC19-23. [PubMed ID: 26023581]. [PubMed Central ID: PMC4437096]. https://doi.org/10.7860/JCDR/2015/9467.5828.
-
35.
Pasini A. Artificial neural networks for small dataset analysis. J Thorac Dis. 2015;7(5):953.
-
36.
Walczak S. Artificial Neural Networks. Advanced Methodologies and Technologies in Artificial Intelligence, Computer Simulation, and Human-Computer Interaction. IGI Global; 2019. p. 40-53.
-
37.
Kaleeswaran V, Dhamodharavadhani S, Rathipriya R. A Comparative Study of Activation Functions and Training Algorithm of NAR Neural Network for Crop Prediction. 2020 4th International Conference on Electronics, Communication and Aerospace Technology (ICECA). IEEE; 2020. p. 1073-7.
-
38.
Amirsadri S, Mousavirad SJ, Ebrahimpour-Komleh H. A Levy flight-based grey wolf optimizer combined with back-propagation algorithm for neural network training. Neural Comput Appl. 2018;30(12):3707-20. https://doi.org/10.1007/s00521-017-2952-5.
-
39.
Karthikeyan A, Garg A, Vinod PK, Priyakumar UD. Machine Learning Based Clinical Decision Support System for Early COVID-19 Mortality Prediction. Front Public Health. 2021;9:626697. [PubMed ID: 34055710]. [PubMed Central ID: PMC8149622]. https://doi.org/10.3389/fpubh.2021.626697.
-
40.
Das AK, Mishra S, Saraswathy Gopalan S. Predicting CoVID-19 community mortality risk using machine learning and development of an online prognostic tool. PeerJ. 2020;8. e10083. [PubMed ID: 33062451]. [PubMed Central ID: PMC7528809]. https://doi.org/10.7717/peerj.10083.
-
41.
An C, Lim H, Kim DW, Chang JH, Choi YJ, Kim SW. Machine learning prediction for mortality of patients diagnosed with COVID-19: a nationwide Korean cohort study. Sci Rep. 2020;10(1):1-11. [PubMed ID: 33127965]. [PubMed Central ID: PMC7599238]. https://doi.org/10.1038/s41598-020-75767-2.
-
42.
Allenbach Y, Saadoun D, Maalouf G, Vieira M, Hellio A, Boddaert J, et al. Development of a multivariate prediction model of intensive care unit transfer or death: A French prospective cohort study of hospitalized COVID-19 patients. PLoS One. 2020;15(10). e0240711. [PubMed ID: 33075088]. [PubMed Central ID: PMC7571674]. https://doi.org/10.1371/journal.pone.0240711.
-
43.
Xia P, Gao K, Xie J, Sun W, Shi M, Li W, et al. Data Mining-Based Analysis of Chinese Medicinal Herb Formulae in Chronic Kidney Disease Treatment. Evid Based Complement Alternat Med. 2020;2020:9719872. [PubMed ID: 32047530]. [PubMed Central ID: PMC7003280]. https://doi.org/10.1155/2020/9719872.
-
44.
Zhang Y, Xin Y, Li Q, Ma J, Li S, Lv X, et al. Empirical study of seven data mining algorithms on different characteristics of datasets for biomedical classification applications. Biomed Eng Online. 2017;16(1):125. [PubMed ID: 29096638]. [PubMed Central ID: PMC5668968]. https://doi.org/10.1186/s12938-017-0416-x.
-
45.
Zhou Y, He Y, Yang H, Yu H, Wang T, Chen Z, et al. Exploiting an early warning Nomogram for predicting the risk of ICU admission in patients with COVID-19: a multi-center study in China. Scand J Trauma Resusc Emerg Med. 2020;28(1):1-13. [PubMed ID: 33109234]. [PubMed Central ID: PMC7590555]. https://doi.org/10.1186/s13049-020-00795-w.
-
46.
Chin V, Samia NI, Marchant R, Rosen O, Ioannidis JPA, Tanner MA, et al. A case study in model failure? COVID-19 daily deaths and ICU bed utilisation predictions in New York state. Eur J Epidemiol. 2020;35(8):733-42. [PubMed ID: 32780189]. [PubMed Central ID: PMC7417851]. https://doi.org/10.1007/s10654-020-00669-6.
-
47.
Wynants L, Van Calster B, Collins GS, Riley RD, Heinze G, Schuit E, et al. Prediction models for diagnosis and prognosis of covid-19: systematic review and critical appraisal. BMJ. 2020;369:m1328. [PubMed ID: 32265220]. [PubMed Central ID: PMC7222643]. https://doi.org/10.1136/bmj.m1328.
-
48.
Ryan L, Lam C, Mataraso S, Allen A, Green-Saxena A, Pellegrini E, et al. Mortality prediction model for the triage of COVID-19, pneumonia, and mechanically ventilated ICU patients: A retrospective study. Ann Med Surg (Lond). 2020;59:207-16. [PubMed ID: 33042536]. [PubMed Central ID: PMC7532803]. https://doi.org/10.1016/j.amsu.2020.09.044.