Abstract
Context:
Artificial intelligence (AI) Chatbots are computer programs that simulate human conversation and use artificial intelligence, including machine learning and natural language processing, to interact with users via natural language. With the outbreak of the COVID-19 pandemic, the use of digital health technologies such as chatbots has accelerated.Objectives:
This study aims to investigate the application of AI chatbots in combating the COVID-19 pandemic and explore their features.Methods:
We reviewed the literature on health chatbots during the COVID-19 pandemic. PubMed, Scopus, Web of Science, and Google Scholar were searched using relevant keywords such as “chatbot”, “conversational agent,” and “artificial intelligence”. To select the relevant articles, we conducted title, abstract, and full-text screening based on inclusion and exclusion criteria. Chatbots, their applications, and design features were extracted from the selected articles.Results:
Out of 673 articles initially identified, 17 articles were eligible for inclusion. We categorized the selected AI chatbots based on their roles, applications, and design characteristics. Around 70% of chatbots were designed to play a preventive role. Our review identified 8 key applications of the AI chatbots during the COVID-19 pandemic, which include (1) information dissemination and education, (2) self-assessment and screening, (3) connecting to health centers, (4) combating misinformation and fake news, (5) patient tracking and service delivery (6) mental health (7) monitoring exposure (8) vaccine information and scheduling. AI chatbots were deployed on various platforms, including mobile apps, web, and social media. Mobile-based chatbots were the most frequent. All chatbots used Natural Language Understanding (NLU) methods to understand natural language input and act on the user’s request. More than 50% of AI chatbots used NLU platforms, including Google Dialogflow, Rasa framework, and IBM Watson.Conclusions:
AI chatbots can play an effective role in combating the COVID-19 pandemic. Increasing people’s awareness, optimizing the use of health resources, and reducing unnecessary encounters are some of the advantages of using AI chatbots during the COVID-19 outbreak. Using NLU platforms can be a suitable solution for developing AI chatbots in the healthcare domain. With advancements in the field of artificial intelligence, it seems that AI chatbots will have a promising future in healthcare, particularly in public health, chronic disease management, and mental health.Keywords
Chatbot Conversational Agents Artificial Intelligence Covid-19 Coronavirus Pandemic
1. Context
With the spread of the COVID-19 pandemic around the world, significant demands were imposed on healthcare systems, and the effective use of available resources and capacity expansion became a vital matter (1). Various restrictions, such as social distancing to slow and prevent the transmission of the virus, created major challenges in the provision of traditional and face-to-face services (2). Furthermore, the rapid growth and update of information about the virus and the fast spread of misinformation and fake news created confusion and anxiety (3, 4).
A solution to these problems in healthcare is the employment of chatbots and other digital health technologies (5, 6). A chatbot, or conversational agent, is a computer software that simulates human interaction and communicates with people in natural language using voice instructions, text dialogues, or both (7, 8). Rule-based and artificial intelligence (NLP)-based chatbots are the two primary categories of chatbots (9). Rule-based chatbots communicate using predefined answers. These chatbots don’t comprehend the context of the conversation; instead, they respond according to a set of if-then rules. Only when users provide a predetermined, controlled keyword or command do they offer matched answers (like Telegram bots). The agent might not be able to match that question with an answer if something is supplied that is outside its scope, such as a different spelling or accent. AI chatbots to communicate with users in natural language employ artificial intelligence, including machine learning and natural language processing (NLP) (10). AI Chatbots use machine learning to make decisions, learn from prior conversations, and recognize patterns in user input. Natural language processing helps chatbots extract structured data from unstructured language input and enables them to replicate that behavior. Even when someone makes a spelling error, NLP still enables them to comprehend the content of the conversation. Users can freely communicate with the chatbot by speech or writing (11). AI chatbots may perform better than their rule-based counterparts (12, 13).
Recently, the use of chatbots has increased across industries and business functions such as customer service, sales, and marketing (14). Chatbots have been employed in the healthcare industry for several applications, including diet, physical activity, and mental health (15-17). Using chatbots can improve real-time access to health information and services for millions of people (18-20). In response to the COVID-19 pandemic and to provide accurate information about the virus, many organizations and governments have created health chatbots (18, 21). For example, the World Health Organization (WHO) has developed a chatbot on the WhatsApp platform to combat COVID-19, which disseminates news and new findings related to the virus and answers users’ questions about protection and care against the Coronavirus (22).
While several studies have investigated the use of chatbots in healthcare, few have focused specifically on AI chatbots and their application in combating COVID-19 (19, 21, 23). Safi et al. have specifically investigated the technical aspects of health chatbots and were not focused on their application in COVID-19 (19). Other studies have reviewed the application of chatbots in COVID-19 but did not specifically focus on AI chatbots and their technical aspects (21, 23). Moreover, these studies that have reviewed the use of chatbots in COVID-19 have only investigated chatbots developed in the first year of the outbreak, ignoring more recent developments.
Therefore, this scoping review aims to systematically review the recent literature on AI-based chatbots related to COVID-19, identify and describe their applications in combating the virus, and investigate the design features of these chatbots. Specifically, we aim to answer the following research questions:
a) How have AI-based chatbots been used to combat COVID-19?
b) What are the key features of these chatbots?
c) How have these technologies been designed?
d) What AI methods have been used in these chatbots?
This review will provide insights into current research on AI-based chatbots in healthcare, their applications in managing pandemics like COVID-19, and the technical aspects that can be effective in the design and utilization of these chatbots.
2. Objectives
In thus study we aim to contribute to the existing literature on the use of AI-based chatbots in healthcare and provide guidance for future research and development in this field.
3. Methods
3.1. Data Resources and Search Strategy
Based on Preferred Reporting Items for Systematic Reviews and Meta-Analyses (PRISMA), we performed a scoping review (24) (Figure 1). Electronic databases (PubMed, Scopus, Web of Science, and Google Scholar) were used for the literature searches. For Google Scholar, we only used the first 150 results because it gives the best results for a search query first. Between November 2019 and October 2022, the search was done. Articles were searched in databases by a combination of related terms, including “chatbot”, “conversational agent”, “voice bot”, “conversational bot”, “virtual assistant”, “conversational system”, “COVID-19”,” SARS-CoV-2”, and “coronavirus.” Search terms were categorized into two groups (Table 1). To combine search terms, we used the OR operator for inside each group and the AND operator for between groups. For management, all records were imported into Endnote 20, and duplicates were removed.
Summary of literature search

Combination of Search Terms
Groups | Search Terms |
---|---|
1 | Covid 19 OR covid-19 OR covid19 OR “corona virus” OR SARS-CoV-2 OR Coronavirus OR Corona |
2 | Chatbot*OR “conversational agent*” OR voicebot OR “virtual assistant*” OR “conversational bot*” OR “conversational system*” OR “conversational interface*” OR “chat bot*” OR chat-bot* OR “virtual agent*” |
3.2. Inclusion and Exclusion Criteria
To choose relevant studies, we applied the following inclusion criteria: peer-reviewed and original articles, full-text available, the studies focused on the application of chatbots in response to the COVID-19 pandemic, and the papers investigated the technical aspects of chatbots. We excluded studies that (a) did not investigate a chatbot, (b) chatbots were not related to the healthcare domain, (c) chatbots were used for other healthcare purposes, (d) were used non-AI or rule-based chatbots, (e) was published as a review article and (f) non-English articles.
3.3. Study Selection
Two reviewers independently screened all titles and abstracts, and full-text articles were screened for eligibility. Disagreements between the reviewers were resolved by discussion, and a third reviewer was consulted if necessary. We recorded the number of articles screened at each stage and the reasons for exclusion.
3.4. Data Extraction
Two investigators independently extracted data from studies. The data extracted included general study information (such as the authors’ name and publication year), a description of the chatbot (chatbot name, aim, and language), chatbot applications, and technical characteristics (such as platform, AI techniques, and user-chatbot interaction). Extracted data were recorded in a spreadsheet. Any disagreements about the data extracted were resolved by the third reviewer (Additional file 2).
4. Results
4.1. Search Results
Figure 1 illustrates the study selection process. A total of 673 articles were identified from the electronic database search. After removing 313 duplicate articles, the abstracts and titles of the remaining articles were screened, and 271 studies were excluded. After the full-text review phase and the removal of 72 studies, 17 articles were finally selected.
4.2. Chatbots Characteristics
The characteristics of 17 chatbots are presented in Table 2. Fourteen chatbots (88.2%) were programmed to converse in English (7, 25-37). Four chatbots were bilingual and supported languages such as French, Italian, Vietnamese, and Indian in addition to English. The United States, India, Australia, and Austria developed bilingual or multilingual chatbots. Arogya Setu, Watson Assistant, Symptoma, and COVIBOT were multi-language. These chatbots exhibit a commitment to inclusivity, potentially fostering satisfaction among diverse linguistic communities. For example, Arogya Setu and COVIBOT are used in India, which support more than 10 popular languages (7, 31).
Chatbots Characteristics
Country and Chatbot Name | Year | language a | Audience b | Chatbot Role (s) c |
---|---|---|---|---|
USA | ||||
Alexa (33) | 2022 | Single | General | Preventive, diagnostic |
CovidBot (36) | 2021 | Single | Older people | Preventive, diagnostic |
Watson Assistant (27) | 2021 | Multi-language | General | Preventive |
India | ||||
Woebot (32) | 2021 | Bilingual | General | Therapeutic |
Arogya setu (7) | 2020 | Multi-language | General | Diagnostic, therapeutic |
CoronaGO (29) | 2021 | Single | General | Preventive |
COVIBOT (31) | 2020 | Multi-language | General | Diagnostic, therapeutic |
WashKaro (30) | 2022 | Bilingual | General | Preventive, diagnostic |
Canada | ||||
Chloe (34) | 2021 | Bilingual | General | Preventive, diagnostic |
MIRA (38) | 2022 | Single | Health care providers | Preventive |
Australia | ||||
Cory COVID-Bot (35) | 2022 | Bilingual | General | Preventive |
Austria | ||||
Symptoma (26) | 2020 | Multi-language | General | Diagnostic |
Germany | ||||
Ada Health (28) | 2020 | Single | General | Diagnostic |
HongKong | ||||
Vac Chat, Fact Check (39) | 2022 | Single | Young people | Preventive |
NewZealand | ||||
Aroha (25) | 2022 | Single | Young people | Preventive |
Spain | ||||
SanIA (40) | 2021 | Single | General | Preventive, diagnostic |
UAE | ||||
VWise (37) | 2022 | Single | General | Preventive |
Thirteen out of the 17 chatbots were developed for the general population, and four were designed for specific populations. Chatbots designed for specific audiences had a preventive role (25, 36, 38, 39). Governments, large corporations (such as IBM and Amazon), and international organizations (such as WHO) produced the majority of AI chatbots aimed at the general public. For instance, the Indian government created CoronaGO and Arogya setu to enhance healthcare services and assist the population during the COVID-19 situation (7, 29).
America, Canada, Australia, and New Zealand focused more on prevention, while India and European countries focused more on diagnostics. Chatbots with a therapeutic role (3 cases) were developed in the US and Canada. Seven out of the 17 chatbots had multiple roles in health service delivery, with 5 cases being preventive and diagnostic and 2 cases being diagnostic and therapeutic. These chatbots were created by the US, India, Canada and Spain.
4.3. Chatbots Applications
The reviewed chatbots offered a range of services to users. We categorized their applications into eight groups (Table 3), which include (1) information dissemination and education, (2) self-assessment and screening, (3) connecting to health centers, (4) combating misinformation and fake news, (5) patient tracking and providing services, (6) mental health, (7) exposure monitoring, and (8) vaccine information and scheduling.
The Applications of Artificial Intelligence (AI) Chatbots
Chatbot Name | Chatbot Role | Chatbot Application | |||||||||
---|---|---|---|---|---|---|---|---|---|---|---|
Preventive | Diagnostic | Therapeutic | Information Dissemination | Self-assessment and Screening | Exposure Monitoring | Patient Tracking and Service Delivery | combating Misinformation and Fake News | Mental Health | Vaccination Information and Scheduling | Connecting to Health Care Center | |
Arogya setu (7) | √ | √ | √ | √ | √ | √ | √ | ||||
Alexa (33) | √ | √ | √ | √ | √ | √ | √ | ||||
Symptoma (26) | √ | √ | |||||||||
CovidBot (36) | √ | √ | √ | √ | √ | √ | |||||
Aroha (25) | √ | √ | √ | √ | |||||||
Chloe (34) | √ | √ | √ | √ | √ | √ | |||||
VWise (37) | √ | √ | √ | √ | |||||||
CoronaGO (29) | √ | √ | √ | √ | √ | ||||||
Cory COVID-Bot (35) | √ | √ | √ | √ | √ | √ | |||||
COVIBOT (31) | √ | √ | √ | √ | √ | ||||||
MIRA (38) | √ | √ | √ | √ | |||||||
Vac Chat, Fact Check (39) | √ | √ | √ | √ | |||||||
Watson Assistant (27) | √ | √ | |||||||||
WashKaro (30) | √ | √ | √ | √ | √ | ||||||
Woebot (32) | √ | √ | |||||||||
Ada Health (28) | √ | √ | √ | ||||||||
SanIA (40) | √ | √ | √ | √ | √ | √ | |||||
N (%) = | 12(70.58%) | 9(52.94%) | 3(17.64%) | 13(76.42%) | 9(52.94%) | 4(23.53%) | 5(29.41%) | 7(41.17%) | 5(29.41%) | 3(17.64%) | 8(47.05%) |
4.3.1. Information Dissemination and Education
The most common application of AI chatbots was disseminating information and providing education related to COVID-19 (13 cases) (7, 25, 27, 29, 30, 33-40). These chatbots provide various information, such as symptoms, disease diagnosis, disease stages, virus transmission, and the availability of healthcare services and how to access them. They also offer training on preventive practices, such as hand hygiene and wearing face masks.
4.3.2. Self-assessment and Screening
Nine AI chatbots were used for triaging and assessing users during the COVID-19 pandemic (7, 26, 28, 30, 31, 33, 34, 36, 40). These chatbots ask a series of questions to evaluate the likelihood of COVID-19 infection without the need to visit medical centers. Self-screening chatbots assess COVID-19 symptoms based on guidelines from governments and approved sources (e.g., CDC or WHO) and provide recommendations if necessary. For instance, Symptoma collects data on disease symptoms, age, and gender to determine the likelihood of COVID-19 infection. According to a study, Symptoma properly identified COVID-19 users in 96.32% of cases (26).
4.3.3. Connecting to Health Centers
Eight chatbots enabled users to communicate with health centers and healthcare providers (7, 28, 29, 31, 35, 36, 38, 40). Some chatbots, in addition to evaluating and triaging individuals, could identify the user’s location using GPS technology. They provide information about the nearest health centers and assist in making appointments for further investigations (7, 36). Some AI chatbots allow online communication with doctors and healthcare centers, enabling users to benefit from remote diagnostic and consulting services (7, 28). For instance, SanIA, developed in Spain, created a secure communication channel between the citizen and the health system during the COVID-19 outbreak. It provides round-the-clock patient advice, including psychological assessments (40).
4.3.4. Combating Misinformation and Fake News
Seven out of 17 AI chatbots were able to combat misinformation and fake news about COVID-19 (30, 33-37, 39). These chatbots provide users with reliable and accurate information, such as up-to-date statistical information, best practices, and health protocols (33, 36). They monitor and identify misinformation and rumors about COVID-19 and prevent its further spread by alerting users.
4.3.5. Patient Tracking and Service Delivery
In 5 cases of AI chatbots, it was possible to provide remote care services to people with COVID-19 (7, 25, 29, 31, 34). These chatbots were able to continuously and daily track the patient’s physical conditions and vital signs. They also answer patients’ questions round-the-clock during mandated self-isolation periods and, if necessary, help communicate with healthcare centers and receive advice and remote care services (31, 34). For example, COVIBOT helps patients take proper medication and provides remote consultation (31).
4.3.6. Mental Health
Supporting people’s mental health during the pandemic and providing necessary services was another application of AI chatbots (5 cases) (25, 32, 35, 38, 40). Mental and emotional problems such as stress, anxiety, and depression were prevalent during the COVID-19 pandemic. Five AI chatbots were able to monitor and support people’s mental health during the pandemic. By providing various mental health services to users, especially patients in home quarantine, these chatbots help reduce the psychological effects of COVID-19, such as anxiety, distress, and depression. For example, Aroha was an AI chatbot that provided services related to the management of anxiety and depression caused by COVID-19 to New Zealand citizens (25).
4.3.7. Exposure Monitoring
Four chatbots were deployed to monitor exposure to the coronavirus and provide notifications (7, 29, 33, 35). These chatbots provide alerts and necessary information to users by assessing the status of disease spread, as well as identifying high-risk and infected locations. For instance, based on GPS and Bluetooth technology, Arogya Setu attempts to assess risk based on the user’s location. Additionally, it informs the user if they are close to a patient with Covid-19 (7).
4.3.8. Vaccine Information and Scheduling
Three chatbots were designed to provide services about COVID-19 vaccines (33, 37, 39). These chatbots provide accurate and up-to-date information about COVID-19 vaccines and inform users about their advantages. Additionally, they provide information about vaccination centers and support users in making appointments for vaccination. These chatbots help reduce vaccine hesitancy and increase vaccination rates. For example, VWise is a free text-based AI chatbot that guides and informs the public about COVID-19 vaccination in the Eastern Mediterranean Region (EMR) (37).
More than 80% of chatbots had multiple applications. The combination of categorized applications with the highest frequency included information dissemination and education combined with combating misinformation and fake news (7 cases), information dissemination and education combined with self-assessment and screening (6 cases), information dissemination and education combined with connecting to health centers (6 cases), and self-assessment and screening combined with connecting to health centers (5 cases). In comparing the performance of the identified chatbots across several applications, it becomes clear that their various capabilities contribute significantly to health society satisfaction. Chatbots specializing in information dissemination and education, such as Cory COVID-Bot, CoronaGO, and Chloe, provide timely and accurate information, enhancing public awareness and subsequently fostering satisfaction within the health society. Additionally, chatbots such as SanIA facilitating connection to health centers not only bridge the gap between users and healthcare providers but also streamline access to vital services, positively impacting satisfaction levels. The ability of certain chatbots, such as Woebot and Aroha, to offer mental health support during the pandemic addresses a critical aspect of overall well-being, contributing to heightened satisfaction within the health society. The Arogya setu, Alexa, and Cory COVID-Bot had the most applications compared to other chatbots. The multi-functional capabilities of chatbots like Arogya Setu, Alexa, and Cory COVID-Bot, which offer a range of services, resonant with different user requirements and preferences and raise levels of satisfaction. In contrast, Symptoma and Woebot had only one-use case and were used to check covid-19 symptoms and support mental health, respectively.
4.4. Chatbots Design Characteristics
As shown in Figure 2, the reviewed AI chatbots differed in terms of design features, including platforms, AI techniques, and user chatbot interaction design, which are discussed below.
Artificial intelligence (AI) chatbot design characteristics
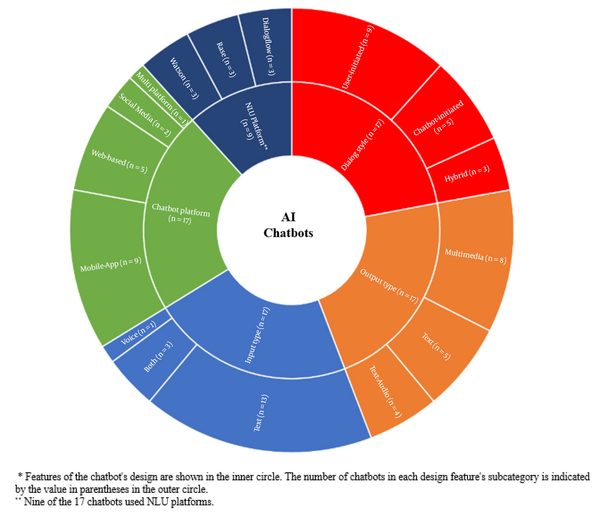
4.4.1. Chatbot Platforms
The AI chatbots were deployed on various platforms. Nine chatbots were mobile applications that could be installed on smartphones (Android or IOS) (7, 27, 30-34, 36, 40). Six chatbots were web-based and accessible through various devices such as computers, tablets, and mobile devices (25, 26, 28, 29, 38, 39). Three chatbots were deployed on social media platforms, including Facebook (2 cases) and Telegram (1 case) (25, 35, 37). One of the chatbots was deployed on both a web-based platform and Facebook (25).
4.4.2. AI Techniques
According to our objectives, all of the reviewed chatbots were based on artificial intelligence. MIRA and Vac Chat Fact Check chatbots were hybrid types that used rule-based and artificial intelligence methods (38, 39). All of the AI chatbots utilized Natural Language Understanding (NLU), a subset of Natural Language Processing (NLP), to understand user input and provide appropriate responses conversationally. For instance, WashKaro and Chloe employed LSTM and BERT methods to understand free-text input (30, 34). One of the chatbots used Artificial Intelligence Markup Language (AIML), an Extensible Markup Language (XML) based method, to understand user input (7). Some chatbots (6 cases) used machine learning methods such as decision trees and deep learning. NLU platforms, such as Google Dialogflow (3 cases), Rasa framework (3 cases), and IBM Watson (3 cases), were employed by more than 50% of the AI chatbots (9 cases). NLU platforms are prebuilt NLU models that are used for developing AI chatbots (42).
4.4.3. User-Chatbot Interaction Design
AI chatbots employ various user-chatbot interaction designs. In terms of input format, 13 chatbots were text-based, 1 was voice-based, and 3 cases offered both text and voice options. Among the 16 text-based chatbots, 13 allowed free-text input, while 3 used predefined and controlled text input. In terms of output format, 8 chatbots were capable of providing information in multimedia formats, including text, audio, images, and videos. Five cases were text-based, and four cases included both text and audio output. In terms of dialog style, 9 chatbots were designed to be user-initiated, where the user initiates the conversation, and the chatbot responds to the user’s queries related to COVID-19. This dialog style was commonly used in most preventive chatbots (9 cases). In 5 cases, the chatbots were initiators, and the user had to respond to the chatbot’s questions regarding demographic data, medical history, and COVID-19 symptoms. For example, Ada Health is a mobile-based diagnostic chatbot that asks users a series of questions for screening purposes (28).
Considering the high accessibility of smartphones, mobile applications such as Arogya Setu, Alexa, and CovidBot cater to different user preferences and accessibility, potentially increasing their access to health services and positively influencing satisfaction across diverse demographics. In terms of input/output format, CovidBot, COVIBOT, and Watson Assistant chatbots are text and voice-based. This possibility makes it easier for users, especially the elderly, to use the chatbot and thus increase their satisfaction (31, 36). Chatbots with free-text input capability, such as Cory COVID-Bot, provide more freedom of action for the user compared to chatbots with input control (35). Symptoma and Mira are more flexible than other chatbots with fixed and free text input (26, 38).
5. Discussion
In this scoping review, we investigated 17 AI chatbots related to the COVID-19 pandemic. The majority of chatbots spoke in English when interacting with users; other languages, such as German, Chinese, and French, were less frequently used. This is consistent with the fact that the United States, followed by India and Canada, had the greatest proportion of chatbot development. Chatbots created in India were often multilingual. This is due to the language diversity in the country (7, 29, 31). The majority of the chatbots were designed for the general population and were less targeted to specific groups. This generality can be attributed to the fact that COVID-19 is not limited to a specific group and has affected the general population (1). However, based on the features of AI chatbots, this novel technology can be useful in providing better health services to high-risk groups such as elderly people.
Our review identified the eight most common use cases of AI chatbots during the COVID-19 pandemic. Enhancing public awareness about COVID-19 has a significant effect on reducing and preventing the spread of the disease (43). More than 75% of chatbots had the task of disseminating information and educating users about COVID-19. These chatbots answered users’ questions round-the-clock and provided them with necessary information. Lack of readily available, accurate information from reliable sources during the COVID-19 outbreak led to the rapid spread of rumors and false information (30, 44). Some AI chatbots were created to address this issue by delivering trustworthy information and spotting rumors and false news (33, 36). Dissemination of up-to-date, reliable, correct, and high-quality information can greatly enhance the usefulness of chatbots. Information and findings related to COVID-19 are rapidly evolving, so the information provided by chatbots must be continuously updated and validated.
Given the importance of early identification of people with COVID-19 (45), some chatbots were developed for the assessment and triage of individuals. These chatbots were often created by governments and organizations such as WHO and CDC to screen people in the community. They usually assess the user’s risk of contracting COVID-19 based on common diagnostic guidelines and employ machine learning techniques. Using these chatbots has many benefits, including reducing unnecessary visits to healthcare centers, promoting social distancing, optimizing the use of health resources, preventing virus transmission, and enabling early identification of patients. However, the diagnostic accuracy of chatbots in COVID-19 diagnosis remains one of the main challenges. Munsch and colleagues have shown that chatbots exhibit different sensitivity and specificity in relation to the diagnosis of COVID-19 (46). Therefore, the diagnostic performance of chatbots must be evaluated.
Providing care services to patients who are in home quarantine and continuously monitoring their physical and mental condition is important for better management of COVID-19 (47). Some chatbots were designed to support COVID-19 patients. These chatbots provide telemonitoring and care services and allow users to communicate online with healthcare providers and medical centers (28). This can improve the quality of care services, enhance patient safety, and reduce violations of home quarantine.
COVID-19 vaccines play an effective role in reducing the mortality rate and the spread of the virus. However, rumors about the side effects of the vaccines and vaccine hesitancy pose significant challenges (46). According to the WHO, vaccine hesitancy is considered one of the 10 major threats to public health (39). Some chatbots are developed to provide services about the COVID-19 vaccine, such as answering user’s questions. These chatbots can be effective in reducing vaccine hesitancy and encouraging people to get vaccinated.
While AI chatbots have the potential to improve healthcare services during the pandemic, Using AI chatbots to help combat the prevalence of COVID-19 presented several main challenges (25, 28, 33, 34). These challenges include ensuring the accuracy of the information, accessibility, and equitable access, language barriers, handling a high volume of queries, integration with healthcare systems, privacy and data concerns, user trust, monitoring and evaluation of the chatbot’s performance, ongoing maintenance and updates, and user education. Addressing these challenges requires collaboration among AI developers, healthcare professionals, and public health agencies to develop effective solutions.
AI chatbots were also examined from technical aspects. On a variety of platforms, including mobile, web, and social media, AI chatbots have been implemented. The majority of chatbots were mobile-based (7, 27, 30-34, 36, 40). This can be due to the popularity of smartphones and easy access to this technology. NLU component is an essential part of every AI chatbot (42). Therefore, all chatbots use NLU techniques to comprehend user input in natural language and respond to their requests. Because it takes NLP skills to create an NLU from scratch, chatbot developers typically employ NLU platforms (42). Numerous NLU platforms have recently been made available as pre-built NLU components for chatbots. Google DialogFlow, Rasa, IBM Watson, and Microsoft LUIS platforms are the four most common platforms for creating chatbots (42). In 52% of the reviewed AI chatbots, the Rasa framework, IBM Watson, and Google DialogFlow platforms were used. Despite the advantages of using NLU platforms, each platform has its features and limitations that should be considered when developing a chatbot (42).
User-chatbot interaction design is an important factor in chatbot efficiency (48). Therefore, we investigated the conversational style of chatbots. The input type in most chatbots was text. In more than 80% of these chatbots, the user can use free text input and have a natural language conversation. AI chatbots use various NLP or NLU techniques to process and understand user requests. Therefore, the user can enter his request in the chatbot in natural language and in the form of free text. However, the results of a study have shown that most of the chatbots were not free text, and the user could only choose from predetermined and controlled options (23). This may be due to most of the reviewed chatbots being rule-based. Some chatbots were based on voice input in addition to text. These chatbots used different voice recognition techniques to understand the input information. For example, three chatbots used voice recognition technology from IBM (18, 19) and Amazon (24). The possibility of user interaction in the form of free text or voice can improve the usability of chatbots and increase ease of use, especially for older adults and disabled people. However, misunderstanding of user requests and language limitations are major issues in these chatbots. The output format of most chatbots was text, but other formats, such as audio, medical catalogs, and educational videos, were also used. Using different and appropriate output formats can help facilitate better and more effective user chatbot conversations, especially in educational and informational chatbots. CoronaGO is an example of these chatbots that provide necessary training related to self-care against COVID-19 to users in multimedia formats (29). The chatbot’s ability to understand user emotions is important, especially in mental health chatbots. With the advancements in artificial intelligence, it is envisaged that AI chatbots will be able to improve the social and emotional aspects of the conversation.
5.1. Conclusions
In conclusion, our scoping review identified eight common application categories of AI chatbots related to COVID-19. Most of the developed chatbots have a preventive role and are commonly utilized for information dissemination, education, and self-assessment. While AI chatbots have the potential to improve healthcare access and quality by providing personalized, accessible, and timely support and information to patients, it is important to acknowledge the potential limitations and challenges associated with their use. Further research is needed to investigate the usability and effectiveness of AI chatbots in different healthcare areas, including their impact on clinical outcomes and user experience. More than half of the AI chatbots were designed based on widely used NLU platforms. Because this method makes chatbot development easier and frees developers from having to deal with technological concerns, using NLU platforms can be advantageous for the development of AI chatbots in the healthcare industry.
Overall, while AI chatbots offer a promising approach to improving healthcare services, further research is needed to understand their benefits and limitations in more depth. With advancements in AI, it appears that AI chatbots such as ChatGPT can mark a bright future in the healthcare domain. However, their efficacy and effectiveness need to be evaluated through rigorous research.
References
-
1.
Nunez A, Sreeganga SD, Ramaprasad A. Access to Healthcare during COVID-19. Int J Environ Res Public Health. 2021;18(6). [PubMed ID: 33799417]. [PubMed Central ID: PMC7999346]. https://doi.org/10.3390/ijerph18062980.
-
2.
Han E, Tan MMJ, Turk E, Sridhar D, Leung GM, Shibuya K, et al. Lessons learnt from easing COVID-19 restrictions: An analysis of countries and regions in Asia Pacific and Europe. Lancet. 2020;396(10261):1525-34. [PubMed ID: 32979936]. [PubMed Central ID: PMC7515628]. https://doi.org/10.1016/S0140-6736(20)32007-9.
-
3.
Rocha YM, de Moura GA, Desiderio GA, de Oliveira CH, Lourenco FD, de Figueiredo Nicolete LD. The impact of fake news on social media and its influence on health during the COVID-19 pandemic: A systematic review. Z Gesundh Wiss. 2021:1-10. [PubMed ID: 34660175]. [PubMed Central ID: PMC8502082]. https://doi.org/10.1007/s10389-021-01658-z.
-
4.
Vijjali R, Potluri P, Kumar S, Teki S. Two stage transformer model for COVID-19 fake news detection and fact checking. arXiv preprint arXiv:2011.13253. 2020.
-
5.
Peek N, Sujan M, Scott P. Digital health and care in pandemic times: impact of COVID-19. BMJ Health Care Inform. 2020;27(1). [PubMed ID: 32565418]. [PubMed Central ID: PMC7307522]. https://doi.org/10.1136/bmjhci-2020-100166.
-
6.
He W, Zhang ZJ, Li W. Information technology solutions, challenges, and suggestions for tackling the COVID-19 pandemic. Int J Inf Manage. 2021;57:102287. [PubMed ID: 33318721]. [PubMed Central ID: PMC7724285]. https://doi.org/10.1016/j.ijinfomgt.2020.102287.
-
7.
Battineni G, Chintalapudi N, Amenta F. Ai chatbot design during an epidemic like the novel coronavirus. Healthcare (Basel). 2020;8(2). [PubMed ID: 32503298]. [PubMed Central ID: PMC7349073]. https://doi.org/10.3390/healthcare8020154.
-
8.
Rodsawang C, Thongkliang P, Intawong T, Sonong A, Thitiwatthana Y, Chottanapund S. Designing a competent chatbot to counter the COVID-19 pandemic and empower risk communication in an emergency response system. OSIR J. 2020;13(2).
-
9.
Abd-Alrazaq AA, Alajlani M, Alalwan AA, Bewick BM, Gardner P, Househ M. An overview of the features of chatbots in mental health: A scoping review. Int J Med Inform. 2019;132:103978. [PubMed ID: 31622850]. https://doi.org/10.1016/j.ijmedinf.2019.103978.
-
10.
A S, John D. Survey on chatbot design techniques in speech conversation systems. Int Advanced Computer Sci Applications. 2015;6(7). https://doi.org/10.14569/ijacsa.2015.060712.
-
11.
Montenegro JLZ, da Costa CA, da Rosa Righi R. Survey of conversational agents in health. Expert Systems with Applications. 2019;129:56-67. https://doi.org/10.1016/j.eswa.2019.03.054.
-
12.
Dahiya M. A tool of conversation: Chatbot. Int J computer sci engineering. 2017;5(5):158-61.
-
13.
Hussain S, Ameri Sianaki O, Ababneh N. A Survey on Conversational Agents/Chatbots Classification and Design Techniques. Web, Artificial Intelligence and Network Applications. 2019. p. 946-56. https://doi.org/10.1007/978-3-030-15035-8_93.
-
14.
Van den Broeck E, Zarouali B, Poels K. Chatbot advertising effectiveness: When does the message get through? Computers in Human Behavior. 2019;98:150-7. https://doi.org/10.1016/j.chb.2019.04.009.
-
15.
Adamopoulou E, Moussiades L. An Overview of Chatbot Technology. Artificial Intelligence Applications and Innovations. 2020. p. 373-83. https://doi.org/10.1007/978-3-030-49186-4_31.
-
16.
Bulla C, Parushetti C, Teli A, Aski S, Koppad S. A review of AI based medical assistant chatbot. Res Applications Web Develop Design. 2020;3(2):1-14.
-
17.
Inkster B, Sarda S, Subramanian V. An Empathy-Driven, Conversational Artificial Intelligence Agent (Wysa) for Digital Mental Well-Being: Real-World Data Evaluation Mixed-Methods Study. JMIR Mhealth Uhealth. 2018;6(11). e12106. [PubMed ID: 30470676]. [PubMed Central ID: PMC6286427]. https://doi.org/10.2196/12106.
-
18.
Herriman M, Meer E, Rosin R, Lee V, Washington V, Volpp KG. Asked and answered: Building a chatbot to address covid-19-related concerns. NEJM Catalyst Innovations in Care Delivery. 2020;1(3).
-
19.
Safi Z, Abd-Alrazaq A, Khalifa M, Househ M. Technical aspects of developing chatbots for medical applications: Scoping review. J Med Internet Res. 2020;22(12). e19127. [PubMed ID: 33337337]. [PubMed Central ID: PMC7775817]. https://doi.org/10.2196/19127.
-
20.
Thorat SA, Jadhav V. A Review on Implementation Issues of Rule-based Chatbot Systems. SSRN Electronic J. 2020. https://doi.org/10.2139/ssrn.3567047.
-
21.
Almalki M, Azeez F. Health Chatbots for Fighting COVID-19: A scoping review. Acta Inform Med. 2020;28(4):241-7. [PubMed ID: 33627924]. [PubMed Central ID: PMC7879453]. https://doi.org/10.5455/aim.2020.28.241-247.
-
22.
Walwema J. The WHO Health Alert: Communicating a Global Pandemic with WhatsApp. J Business Technic Communication. 2020;35(1):35-40. https://doi.org/10.1177/1050651920958507.
-
23.
Amiri P, Karahanna E. Chatbot use cases in the Covid-19 public health response. J Am Med Inform Assoc. 2022;29(5):1000-10. [PubMed ID: 35137107]. [PubMed Central ID: PMC8903403]. https://doi.org/10.1093/jamia/ocac014.
-
24.
Moher D, Liberati A, Tetzlaff J, Altman DG, Prisma Group. Preferred reporting items for systematic reviews and meta-analyses: The PRISMA statement. Ann Intern Med. 2009;151(4):264-9. W64. [PubMed ID: 19622511]. https://doi.org/10.7326/0003-4819-151-4-200908180-00135.
-
25.
Ludin N, Holt-Quick C, Hopkins S, Stasiak K, Hetrick S, Warren J, et al. A chatbot to support New Zealand young people during the COVID-19 pandemic: Evaluation of a real world roll out of an open trial. J Med Internet Res. 2022. https://doi.org/10.2196/preprints.38743.
-
26.
Martin A, Nateqi J, Gruarin S, Munsch N, Abdarahmane I, Zobel M, et al. An artificial intelligence-based first-line defence against COVID-19: digitally screening citizens for risks via a chatbot. Sci Rep. 2020;10(1):19012. [PubMed ID: 33149198]. [PubMed Central ID: PMC7643065]. https://doi.org/10.1038/s41598-020-75912-x.
-
27.
McKillop M, South BR, Preininger A, Mason M, Jackson GP. Leveraging conversational technology to answer common COVID-19 questions. J Am Med Inform Assoc. 2021;28(4):850-5. [PubMed ID: 33517402]. [PubMed Central ID: PMC7798957]. https://doi.org/10.1093/jamia/ocaa316.
-
28.
Morse KE, Ostberg NP, Jones VG, Chan AS. Use characteristics and triage acuity of a digital symptom checker in a large integrated health system: Population-based descriptive study. J Med Internet Res. 2020;22(11). e20549. [PubMed ID: 33170799]. [PubMed Central ID: PMC7717918]. https://doi.org/10.2196/20549.
-
29.
Pandey AK, Janghel RR, Sujatha R, Kumar SS, Kumar TS, Chatterjee JM. Coronago website integrated with chatbot for COVID-19 Tracking. ISIC. 2021. p. 521-7.
-
30.
Pandey R, Gautam V, Pal R, Bandhey H, Dhingra LS, Misra V, et al. A machine learning application for raising WASH awareness in the times of COVID-19 pandemic. Sci Rep. 2022;12(1):810. [PubMed ID: 35039533]. [PubMed Central ID: PMC8764038]. https://doi.org/10.1038/s41598-021-03869-6.
-
31.
Patil A, Patil K, Shimpi G, Kulkarni M. COVIBOT: An efficient AI-based Chatbot with Voice Assistance and Multilingualism for COVID-19. Department Inf Technol. 2020;17.
-
32.
Prochaska JJ, Vogel EA, Chieng A, Baiocchi M, Maglalang DD, Pajarito S, et al. A randomized controlled trial of a therapeutic relational agent for reducing substance misuse during the COVID-19 pandemic. Drug Alcohol Depend. 2021;227:108986. [PubMed ID: 34507061]. [PubMed Central ID: PMC8423936]. https://doi.org/10.1016/j.drugalcdep.2021.108986.
-
33.
Sharevski F, Slowinski A, Jachim P, Pieroni E. "Hey Alexa, what do you know about the COVID-19 vaccine?"- (Mis)perceptions of mass immunization and voice assistants. Internet Things (Amst). 2022;19:100566. [PubMed ID: 37520838]. [PubMed Central ID: PMC9264809]. https://doi.org/10.1016/j.iot.2022.100566.
-
34.
Siedlikowski S, Noel LP, Moynihan SA, Robin M. Chloe for COVID-19: Evolution of an intelligent conversational agent to address infodemic management needs during the COVID-19 pandemic. J Med Internet Res. 2021;23(9). e27283. [PubMed ID: 34375299]. [PubMed Central ID: PMC8457340]. https://doi.org/10.2196/27283.
-
35.
Van Baal ST, Le S, Fatehi F, Hohwy J, Verdejo-Garcia A. Cory COVID-Bot: An evidence-based behavior change chatbot for COVID-19. Stud Health Technol Inform. 2022;289:422-5. [PubMed ID: 35062181]. https://doi.org/10.3233/SHTI210948.
-
36.
Wang X, Liang T, Li J, Roy S, Pandey V, Du Y, et al. Artificial Intelligence-Empowered Chatbot for Effective COVID-19 Information Delivery to Older Adults. Int J E-Health Med Communications (IJEHMC). 2021;12(6):1-18.
-
37.
Zidoun Y, Kaladhara S, Kotp Y, Powell L, Nour R, Al Suwaidi H, et al. Conversational Agent to Address COVID-19 infodemic: A design-based research approach. Challenges of Trustable AI and Added-Value on Health. 2022. https://doi.org/10.3233/shti220421.
-
38.
Noble JM, Zamani A, Gharaat M, Merrick D, Maeda N, Lambe Foster A, et al. Developing, implementing, and evaluating an artificial intelligence-guided mental health resource navigation chatbot for health care workers and their families during and following the COVID-19 Pandemic: Protocol for a cross-sectional study. JMIR Res Protoc. 2022;11(7). e33717. [PubMed ID: 35877158]. [PubMed Central ID: PMC9361145]. https://doi.org/10.2196/33717.
-
39.
Luk TT, Lui JHT, Wang MP. Efficacy, usability, and acceptability of a chatbot for promoting COVID-19 vaccination in unvaccinated or booster-hesitant young adults: Pre-post pilot study. J Med Internet Res. 2022;24(10). e39063. [PubMed ID: 36179132]. [PubMed Central ID: PMC9534274]. https://doi.org/10.2196/39063.
-
40.
Serra CM. Utility of sania chatbot to maintain continuity of care and psychological support during COVID-19 Pandemic. Biomed J of Sci Technical Res. 2021;33(5). https://doi.org/10.26717/bjstr.2021.33.005474.
-
41.
Jovanovic M, Baez M, Casati F. Chatbots as conversational healthcare services. IEEE Internet Computing. 2021;25(3):44-51. https://doi.org/10.1109/mic.2020.3037151.
-
42.
Abdellatif A, Badran K, Costa DE, Shihab E. A comparison of natural language understanding platforms for chatbots in software engineering. IEEE Transactions on Software Engineering. 2022;48(8):3087-102. https://doi.org/10.1109/tse.2021.3078384.
-
43.
Sun CX, Bin HE, Di MU, Li PL, Zhao HT, Li ZL, et al. Public awareness and mask usage during the COVID-19 epidemic: a survey by China CDC new media. Biomedi Environmental Sci. 2020;33(8):639-45.
-
44.
Vraga EK, Jacobsen KH. Strategies for Effective Health Communication during the Coronavirus Pandemic and Future Emerging Infectious Disease Events. World Med Health Policy. 2020;12(3):233-41. https://doi.org/10.1002/wmh3.359.
-
45.
Zhao Y, Cui C, Zhang K, Liu J, Xu J, Nisenbaum E, et al. COVID19: A systematic approach to early identification and healthcare worker protection. Front Public Health. 2020;8:205. [PubMed ID: 32574296]. [PubMed Central ID: PMC7248242]. https://doi.org/10.3389/fpubh.2020.00205.
-
46.
Rahmani K, Shavaleh R, Forouhi M, Disfani HF, Kamandi M, Oskooi RK, et al. The effectiveness of COVID-19 vaccines in reducing the incidence, hospitalization, and mortality from COVID-19: A systematic review and meta-analysis. Front Public Health. 2022;10:873596. [PubMed ID: 36091533]. [PubMed Central ID: PMC9459165]. https://doi.org/10.3389/fpubh.2022.873596.
-
47.
Xu H, Huang S, Qiu C, Liu S, Deng J, Jiao B, et al. Monitoring and management of home-quarantined patients with COVID-19 using a wechat-based telemedicine system: Retrospective cohort study. J Med Internet Res. 2020;22(7). e19514. [PubMed ID: 32568727]. [PubMed Central ID: PMC7333794]. https://doi.org/10.2196/19514.
-
48.
Chaves AP, Gerosa MA. How should my chatbot interact? A survey on social characteristics in human–chatbot interaction design. Int J Human–Computer Interaction. 2020;37(8):729-58. https://doi.org/10.1080/10447318.2020.1841438.