Abstract
Background:
Chronic diarrhea in children poses a significant clinical challenge and can lead to adverse health outcomes. Among various causes, fat malabsorption is particularly concerning, as it may lead to inadequate nutrient absorption, malnutrition, and impaired growth. Prompt and precise diagnosis is crucial for implementing effective treatments.Objectives:
The goal of this study is to utilize deep learning to create a superior diagnostic tool that exceeds traditional methods, facilitating the early identification of fat malabsorption in children suffering from chronic diarrhea.Methods:
In a preliminary study involving 100 pediatric patients, 25 machine learning algorithms were evaluated. The convolutional neural network (CNN) was identified as the most effective and subsequently refined through hyperparameter tuning.Results:
The CNN model exhibited exceptional performance, attaining a test accuracy of 97% and an area under the curve (AUC) score of 99.4%. These results underscore its reliability in accurately identifying cases of fat malabsorption.Conclusions:
This research represents noteworthy progress in pediatric gastroenterology, merging deep learning techniques with medical expertise to develop a dependable and rapid diagnostic tool. This innovative method promises significant improvements in detecting fat malabsorption, potentially transforming clinical practices and enhancing patient outcomes in children with chronic diarrhea.Keywords
Pediatric Chronic Diarrhea Fat Malabsorption Deep Learning Artificial Intelligence Diagnostic Tool Machine Learning Algorithms CNN
1. Background
Chronic diarrhea poses a significant clinical challenge worldwide in pediatric populations (1). This condition, characterized by persistent and frequent bowel movements over a prolonged period, can lead to serious health consequences for affected children. Fat malabsorption stands out as a major concern among the various causes. It occurs when the digestive system is unable to efficiently absorb dietary fats, leading to their excessive excretion in stools (2). This impairs the absorption of essential nutrients, contributing to malnutrition and growth delays in these children. The prompt and accurate diagnosis of fat malabsorption is critical for starting appropriate treatments and ensuring the best possible health outcomes for pediatric patients suffering from chronic diarrhea. Traditional diagnostic methods for fat malabsorption, which involve collecting and analyzing stool samples, are not only burdensome and time-consuming but also produce variable results and are prone to errors (3-5). There is a pressing need for more innovative, efficient, and reliable diagnostic methods for pediatric patients with chronic diarrhea, highlighting the importance of advancing diagnostic technologies.
In recent years, deep learning, a branch of artificial intelligence, has initiated a transformative shift in medical imaging and diagnostics (6). These sophisticated algorithms excel at identifying complex patterns and features within intricate datasets, leading to the development of potent diagnostic tools.
Machine learning algorithms have made significant strides in processing and analyzing biomedical data, aided by advancements in computational power and the growing availability of datasets. These algorithms are broadly classified into three categories: Supervised, self-supervised, and reinforcement learning (7). Supervised learning algorithms are preferred when dealing with labeled input and output data, as they learn the relationship between inputs and outputs through optimization techniques. Deep learning, which is a type of supervised learning, is included in this category. It encompasses variants like long short-term memory (LSTM) or convolutional neural networks (CNN), which adjust their parameters through iterative updates using gradient descent methods (8-10). This process enables the model to enhance its accuracy progressively.
The CNN algorithm, specifically, is designed for analyzing datasets in 3D, 2D, or 1D. It utilizes kernels of various sizes to extract vital features from the data, aiding in the identification of the intended target. Activation functions within CNNs are crucial for tackling non-linear challenges across different datasets (11-13). Factors such as the type of activation function, kernel size, number of kernels, and the number of hidden layers are critical for achieving desired outcomes. Optimizing these parameters is essential and should be customized to address the specific problem being tackled.
In our study, we aimed to leverage the capabilities of advanced technology for the early detection of fat malabsorption in children suffering from chronic diarrhea. Our goal is to create a reliable and quick diagnostic tool by integrating cutting-edge computational methods with established medical expertise. This innovation is expected to significantly enhance the accuracy and speed of detecting fat malabsorption.
This paper outlines the methodology, results, and implications of our pilot study, which involves training and validating a CNN model on a meticulously compiled dataset of pediatric patients with chronic diarrhea. We evaluate the model's ability to differentiate between cases of fat malabsorption and those without, and we explore the potential impact of our findings on clinical practices. The developed deep learning model identifies fat malabsorption without necessitating stool samples, offering three key advantages over conventional diagnostic methods: rapid and early diagnosis, avoidance of the inconvenience and discomfort associated with collecting stool samples, and cost-effectiveness. The CNN model represents a significant advancement in pediatric gastroenterology, providing a novel and efficient diagnostic approach to address the critical need for accurate and prompt diagnosis in children with chronic diarrhea.
The study involved 100 patients who visited the Pediatric Gastroenterology outpatient clinic at Gaziantep University Medical Faculty Hospital with diarrhea lasting more than four weeks, previously undiagnosed. Diagnostic tests such as stool reductant, hematocrit, sugar chromatography, hemoglobin (Hb), platelet count, white blood cell count, alanine aminotransferase (ALT), aspartate aminotransferase (AST), albumin, glucose, sodium (Na), potassium (K), chloride (Cl), calcium (Ca), magnesium (Mg), phosphorus (P), vitamin B12, ferritin, folate, vitamin D, lipid profiles, immunoglobulins, and a sweat test were performed for a comprehensive assessment and to rule out other conditions.
We employed 25 different machine-learning algorithms, among which the CNN emerged as the most effective. This model underwent fine-tuning of hyperparameters tailored to the specific problem. The data fed into these algorithms included 21 key features like gender, age, weight, and height, along with various clinical parameters such as Hb and platelet count. The objective was to predict the presence or absence of fat malabsorption. The CNN model's efficacy was gauged through accuracy, F1 score, precision, recall, and Area Under the Curve (AUC) score (14-16). The model demonstrated a remarkable 97% accuracy, an AUC score of 99.4%, and an F1 score of 96%. In clinical validation at Cengiz Gökçek Gynecology and Pediatrics Hospital and the Department of Pediatrics at Gaziantep University, the model achieved 100% accuracy in detecting fat malabsorption in pediatric patients with chronic diarrhea.
2. Objectives
This study aimed to utilize deep learning, particularly CNN models, to improve the early detection of fat malabsorption in pediatric patients with chronic diarrhea. Chronic diarrhea is a significant clinical challenge, and the presence of fat malabsorption further complicates the health of affected children. Traditional diagnostic methods, which rely on the collection and analysis of stool samples, are cumbersome and prone to variability. Our research seeks to identify innovative and efficient diagnostic methods by harnessing the power of advanced computational techniques, especially deep learning. By training and validating a CNN model using a specially curated dataset of pediatric patients, our objective is to evaluate the model's effectiveness in distinguishing between cases of fat malabsorption and those without. The ultimate aim is to develop a fast, non-invasive, and cost-effective diagnostic tool for pediatric gastroenterology, meeting the critical need for accurate and prompt diagnosis in children experiencing chronic diarrhea.
3. Materials
The study included patients from Gaziantep University's Department of Pediatrics who presented with diarrhea lasting more than four weeks without a previous diagnosis. All individuals attending the Pediatric Gastroenterology Clinic from January 2022 to December 2022 for chronic diarrhea were considered for the study, totaling 100 patients.
A thorough physical examination was conducted for each patient, during which height and weight were measured. Dates of birth and the onset of diarrhea were collected from patients and their caregivers. Data on fecal reductant, steatocrit, sugar chromatography, hemoglobin, platelet count, white blood cell count, ALT, AST, albumin, glucose, Na, K, Cl, Ca, Mg, P, vitamin B12, ferritin, folate, vitamin D tests, lipid profiles, immunoglobulins, and sweat test results were gathered and used in the differential diagnosis.
For reductant analysis, stool samples were diluted with distilled water in a graduated centrifuge tube to form a slurry, which was then centrifuged. The supernatant obtained was analyzed for reductant content using the Clinitest method. This test involved adding fifteen drops of the supernatant to a 15 mL tube, followed by a Clinitest tablet. The mixture was then heated to boiling for 15 seconds, after which a color change was observed and compared with a reference scale. A green-brown coloration of the remaining liquid indicated the presence of reductant substances, marking the test as positive. A reductant level of 0.5 mg/dL or higher in the stool was deemed abnormal.
The fecal steatocrit test measures the ratio of fecal fat to the total fecal matter in a stool sample. For this test, about 0.5 grams of feces were processed, homogenized, and centrifuged at 12,000 rpm for 15 minutes. The fat layer's length was then measured as a percentage of the total solid layer. For infants under six months, values exceeding 85% were indicative of fat malabsorption, while for infants older than six months, values between 93 - 95% were considered significant.
Initially, standards for stool and sugar chromatography were set using glucose, galactose, fructose, lactose, and sucrose, each at a concentration of 1 g/L. This process involved dissolving 10 mg of each sugar in 10 mL of distilled water and mixing it with 2 mL of acetone in a graduated centrifuge tube, leaving a 1 cm space from the top. Sample application points on chromatography paper were marked at intervals of 1.25 cm. The samples, mixed with 1 mL of stool, were vortexed and centrifuged to obtain a clear acetone supernatant for the next step. A line was drawn with a pencil 1 cm from the bottom of the chromatography plate, which was then positioned 0.5 cm from the edge, and samples were applied using a chromatography syringe with 10 µL. The plate was placed in a solvent tank and allowed to run until the solvent reached the top. Patient samples were then analyzed by comparing the migration distances of their spots with those of the standard spots.
3.1. Dataset Preparation
The dataset for the machine learning algorithms included 21 inputs: gender, age, weight, height, Hb, platelet count, white blood cell count, ALT, AST, albumin, glucose, Na, K, Cl, Ca, Mg, P, vitamin B12, ferritin, folate, and vitamin D test results. These criteria and variables were chosen based on the exploration of potential causes of chronic diarrhea, with blood tests conducted to ensure a thorough evaluation. The goal was to determine the presence or absence of fat malabsorption. Among the collected data, 19 patients were identified as having positive fat malabsorption.
To address the challenge of an imbalanced dataset, where the minority class (positive fat malabsorption cases) was significantly underrepresented compared to the majority class (negative cases), the synthetic minority over-sampling technique (SMOTE) was employed (17). This technique enhances the dataset by generating synthetic examples of the minority class. It selects a sample from the minority class along with its k nearest neighbors and then creates new samples by interpolating these points in the feature space. Using the SMOTE algorithm, we augmented the dataset to include up to 81 positive cases, resulting in a balanced dataset of 162 samples, with 81 negative and 81 positive cases.
For model training, 122 samples were used, while 10 samples were set aside for validation. Additionally, 30 original data samples not generated by the SMOTE algorithm were reserved for testing to accurately assess the performance of the developed models. This approach ensures that the testing phase reflects the models' effectiveness on genuine data, free from synthetic augmentation.
3.2. Methods
Convolutional neural networks are a fundamental category within deep learning, designed primarily for processing images and signals. They utilize specialized kernels to efficiently extract relevant features from the input data. Following the operation of these kernels, activation functions introduce nonlinearity into the model, enhancing its ability to understand complex data relationships. Functions such as sigmoid or ReLU are commonly used for this purpose, enabling the network to capture complex patterns in the data (12).
A critical element of CNN architecture is the Maxpooling layer (18). This layer identifies and retains the most significant features within specific areas or pools, greatly aiding the network in pattern recognition. The integration of a Dropout layer instrumental in mitigating overfitting, a common challenge in machine learning (19). Overfitting occurs when a model excessively learns from the training data to the detriment of its generalization to new, unseen data (20). Overfitting is addressed by the Dropout layer, which temporarily disables a fraction of the kernels in each training epoch, encouraging the model to generalize better across various data aspects.
The effectiveness of CNNs relies on the precise tuning of parameters, such as the size and number of kernels (21). Adjusting these parameters to suit the particular challenge at hand is crucial. It is important to note that different CNN architectures may be more suited to specific types of problems, demonstrating the model's flexibility and capability to tackle diverse tasks. Figure 1 provides a visual representation of a CNN model's architecture for further clarification.
The architecture of the CNN Model. This diagram outlines the essential components and layers of the CNN model, highlighting its structure.
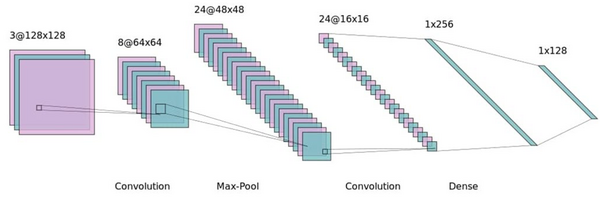
The learning rate is another critical parameter that requires careful adjustment. An improperly set learning rate can lead the model to get stuck in local minima or exhibit erratic behavior.
Equally important is the selection of optimizers (22-24). These gradient-based optimization algorithms are pivotal for various tasks. Choosing the right loss function is also essential. In the context of deep learning models that utilize gradient-based optimizers, the loss function directs the updates of kernels by calculating the derivative of the loss concerning the kernel weights. For example, Mean Absolute Error is apt for regression issues, Binary Cross Entropy fits binary classification tasks, and Categorical Cross Entropy is suited for multi-class classification scenarios (25). Each choice must be made with careful consideration to maximize model efficacy. In our case, the Binary Cross Entropy loss function was chosen due to the binary classification nature of our problem.
Padding is a key concept in image processing, especially within the realm of CNNs (26). It involves appending extra pixels to the image borders before filter application or convolution. Padding serves two main purposes. First, it aims to mitigate the potential loss of edge information during convolution, which, without padding, could result in a reduced output feature map size, possibly overlooking important details. Second, padding helps maintain the spatial dimensions of the input image through the network layers, which is crucial for applications such as object detection and localization, where accurate spatial information is vital. Different padding strategies exist, with "valid" indicating no padding and "same" implying padding that retains the original input size. By carefully implementing padding, CNNs can effectively learn and extract significant features from images, thereby enhancing their performance in a variety of computer vision tasks. For our project, 'same' padding was utilized to ensure the input size was maintained.
Several supervised machine learning algorithms were applied in the pursuit of detecting fat malabsorption, with the CNN model proving to be the most effective. Given the feature size of 1 × 21, 1-dimensional kernels were used in the CNN to extract relevant features. The model's parameters were initially fine-tuned to address this particular issue. Table 1 provides a detailed list of parameters targeted for optimization.
Optimized Parameters of Convolutional Neural Network (CNN)
Hyperparameters | Values |
---|---|
Learning rate | 0.01, 0.001, and 0.0001 |
Number of hidden layers | 1 to 20, the stride is 1 |
Number of dense hidden layers | 1 to 5, the stride is 1 |
Number of neurons | 4 to 512, the stride is 4 |
Number of kernels | 16 to 2048, the stride is 16 |
Size of kernels | 1 × 3, 1 × 5, and 1 × 7 |
Activation functions | ReLU, Sigmoid, Tanh, Selu, and Linear |
Batch size | 1 to 16 |
Optimizers | Adam, RMSprop, Momentum, and Adadelta |
Loss function | Binary cross entropy |
A grid search method was employed to determine the optimal parameters, recording validation accuracy across 5 epochs. The model that achieved the highest validation accuracy was then selected for further training, as outlined in Table 2.
Layer and Kernel -Neuron Count | Activation Function | Trainable Parameters |
---|---|---|
Conv1D | Linear | 256 |
Kernel count = 128 | ||
Kernel size = (3, 1) | ||
Maxpooling1D (Pool size = 2) | ||
Conv1D | ReLU | 24704 |
Kernel count = 128 | ||
Kernel size = (3, 1) | ||
Maxpooling1D (Pool size = 2) | ||
Conv1D | ReLU | 98560 |
Kernel count = 256 | ||
Kernel size = (3, 1) | ||
Maxpooling1D (Pool size = 2) | ||
Conv1D | ReLU | 393728 |
Kernel count = 512 | ||
Kernel size = (3, 1) | ||
Maxpooling1D (Pool size = 2) | ||
Flatten | ||
Dense | ||
Neuron count = 1 | Sigmoid | 513 |
The study incorporated a total of 162 samples. To evaluate the model's effectiveness, 21 negative and 9 positive original samples were reserved, ensuring these 30 datasets comprised actual, non-synthetic data. This allocation involved using 122 samples for training, 10 for validation, and 30 for testing the model's accuracy.
4. Results
The distribution of the patients' age, gender, weight, and height percentiles is presented in Table 3. Table 4 displays a comparison of weight and height percentile values, stool steatocrit, reducing substances in stool, and mortality based on gender. Furthermore, Table 5 contrasts certain blood parameters between deceased patients and survivors.
Age, Gender, Weight, and Height Percentile Distribution of the Patients
Parameter | Values a |
---|---|
Age (y) | |
< 1 | 42 (42.0) |
1 - 5 | 46 (46.0) |
> 5 | 12 (12.0) |
Gender | |
Female | 45 (45.0) |
Male | 55 (55.0) |
Weight percentile | |
< 3 | 26 (26.0) |
3 | 3 (3.0) |
3 - 10 | 15 (15.0) |
10 | 2 (2.0) |
10 - 25 | 24 (24.0) |
25 - 50 | 15 (15.0) |
50 | 2 (2.0) |
50 - 75 | 8 (8.0) |
75 - 90 | 1 (1.0) |
90 - 97 | 3 (3.0) |
> 97 | 1 (1.0) |
Height percentile | |
< 3 | 26 (26.0) |
3 | 2 (2.0) |
3 - 10 | 14 (14.0) |
10p | 4 (4.0) |
10 - 25 | 16 (16.0) |
25 | 6 (6.0) |
25 - 50 | 12 (12.0) |
50 | 3 (3.0) |
50 - 75 | 13 (13.0) |
75 - 90 | 3 (3.0) |
90 - 97 | 1 (1.0) |
Total | 100 (100.0) |
The Comparison of Weight and Height Percentile Values, Steatocrit in Stool, Reducing Substance in Stool, and Death Status According to Gender a
Parameter | Female | Male | P-Value b |
---|---|---|---|
Weight percentile | |||
< 3 | 10 (22.2) | 16 (29.1) | 0.418 |
3 - 97 | 34 (75.6) | 39 (70.9) | |
> 97 | 1 (2.2) | 0 (0.0) | |
Height percentile | 0.891 | ||
< 3 | 12 (26.7) | 14 (25.5) | |
3 - 97 | 33 (73.3) | 41 (74.5) | |
Steatocrit in stool | 0.508 | ||
Negative | 37 (82.2) | 44 (80.0) | |
Trace/rare | 4 (8.9) | 8 (14.5) | |
1 + | 1 (2.2) | 1 (1.8) | |
2 + | 1 (2.2) | 2 (3.6) | |
3 + | 2 (4.4) | 0 (0.0) | |
Reductant in stool | 0.050 | ||
Negative | 24 (53.3) | 33 (60.0) | |
Trace/rare | 18 (40.0) | 11 (20.0) | |
2 + | 1 (2.2) | 7 (12.7) | |
3 + | 1 (2.2) | 4 (7.3) | |
4 + | 1 (2.2) | 0 (0.0) | |
Current status of the patient | 0.657 | ||
Alive | 42 (93.3) | 50 (90.9) | |
Dead | 3 (6.7) | 5 (9.1) |
Parameters | Dead (n = 8) | Living (n = 92) | P -Value |
---|---|---|---|
Hb, g/dL | 9.9 (8.1 - 15.6) | 10.7 (4.6 - 15.5) | 0.965 |
Leukocytes, 103/µ | 13310 (3360 - 20070) | 9690 (3360 - 45350) | 0.141 |
Platelets, 103/µ | 285500 (26000 - 650000) | 388500 (23000 - 1159000) | 0.384 |
Glucose, mg/dL | 82 (62 - 134) | 86 (17 - 416) | 0.954 |
Albumin, g/L | 33 (12 - 44) | 39 (19 - 52.1) | 0.063 |
ALT, U/L | 14.5 (7 - 61) | 21.5 (5 - 109) | 0.266 |
AST, U/L | 44 (25 - 78) | 42.5 (12 - 215) | 0.990 |
Ferritin, ug/L | 207.8 (9.8 - 5324) | 31.6 (2.7 - 6036) | 0.030 |
B12, ng/L | 238 (84 - 930) | 275 (40 - 1550) | 0.608 |
Folate, ug/L | 9.8 (7 - 14.1) | 12.7(3.6 - 24) | 0.070 |
Vitamin D, ug/L | 11.0 (0 - 35) | 18.9 (0 - 95.5) | 0.086 |
Na, mmol/L | 135 (133 - 153) | 136 (126 - 159) | 0.740 |
K, mmol/L | 4.2 (3.4 - 6.4) | 4.5 (2.6 - 7.7) | 0.879 |
Cl, mmol/L | 113 (104 - 117) | 105 (70 - 405) | 0.011 |
Ca, mmol/L | 8.8 (7.9 - 12.1) | 9.9 (7.3 - 11.3) | 0.042 |
P, mmol/L | 3.7 (2.5 - 5.1) | 5 (2.8 - 10.3) | 0.004 |
Mg, mmol/L | 1.7 (1.4 - 2.3) | 2.1 (1.1 - 5.7) | 0.016 |
Figure 2 illustrates the CNN model's training performance. Subfigure (A) shows the reduction in loss for both validation and training data, while subfigure (B) highlights the improvement in accuracy across epochs.
A: Training and validation loss; B: Training and validation accuracy.
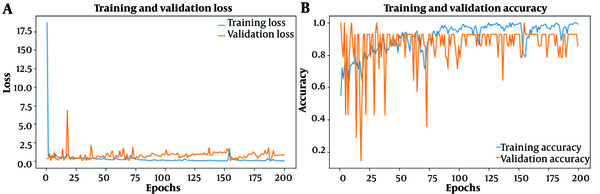
The performance of the CNN model was evaluated using several metrics, including accuracy, precision, recall, F1 score, and AUC score (15). After a thorough assessment of the test dataset, our model exhibited impressive performance metrics. Notably, it achieved an accuracy of 97%, indicating a high level of correct predictions. The F1 score, which harmonizes precision and recall, was outstanding, reaching 95% for class 1 and 98% for class 0. The metrics, including precision, recall, F1 score, and accuracy for the test data, are detailed in Table 6.
Model Evaluation Metrics
Precision (Positive Predictive Value) | Recall (Sensitivity) | z | F1-Score | Support | |
---|---|---|---|---|---|
Class 0 | 1.00 | 0.95 | 1.00 | 0.98 | 21 |
Class 1 (fat-malabsorbtion) | 0.90 | 1.00 | 0.95 | 0.95 | 9 |
Accuracy | 0.97 | 30 | |||
Macro avg | 0.95 | 0.98 | 0.95 | 0.96 | 30 |
Weighted avg | 0.97 | 0.97 | 0.95 | 0.97 | 30 |
The macro average calculates the unweighted mean of precision, recall, and F1 score across all classes (14), treating each class equally without considering its frequency or distribution in the dataset. Conversely, the weighted average takes into account class imbalance by calculating the metrics weighted by the number of samples in each class, providing a more nuanced evaluation by emphasizing the performance in larger classes. The "macro avg" values show exemplary performance across classes, with a precision of 95%, indicating that 95% of positive predictions were accurate. Moreover, a recall score of 98% suggests that 98% of actual positives were correctly identified. The resultant F1 score of 96% indicates a balanced integration of precision and recall. The "weighted avg" values, accounting for class distribution, similarly highlight strong overall model efficacy, with precision, recall, and F1 score all at 97%, demonstrating the model's adeptness at generalizing across different classes. These outcomes affirm the model's robust and reliable classification capability, highlighting its potential for real-world applications.
Furthermore, the AUC score, assessing the model's capacity to differentiate between positive and negative classes, reached an outstanding 99.4%. These findings emphasize the model's strength and efficiency in accurately classifying instances.
Several machine learning algorithms were tested to achieve the best outcome, with CNN emerging as the top performer. The results, displayed in Table 7, are organized in descending order based on test accuracy, highlighting the CNN model's superior performance.
Algorithm | Accuracy | AUC |
---|---|---|
CNN | 97.0 | 99.4 |
Quadratic discriminant | 87.9 | 92.0 |
Medium gaussian SVM | 85.6 | 93.1 |
Kernel naive Bayes | 80.3 | 84.1 |
Quadratic SVM | 80.3 | 88.1 |
Gaussian naive bayes | 79.5 | 86.2 |
Cubic SVM | 79.5 | 87.0 |
Ensemble subspace KNN | 78.8 | 86.1 |
Fine tree | 76.5 | 75.6 |
Medium tree | 76.5 | 75.6 |
Coarse tree | 76.5 | 74.5 |
Fine Gaussian SVM | 76.5 | 85.0 |
Bagged trees | 75.8 | 88.0 |
Fine KNN | 73.5 | 72.9 |
Cosine KNN | 72.0 | 80.1 |
Linear SVM | 70.5 | 74.7 |
Ensemble RUS-boosted trees | 69.7 | 81.8 |
Ensemble subspace discriminant | 68.2 | 75.3 |
Logistic regression | 65.9 | 63.2 |
Linear discriminant | 65.2 | 67.2 |
Cubic KNN | 62.9 | 79.4 |
Medium KNN | 61.4 | 79.4 |
Weighted KNN | 60.6 | 86.4 |
Coarse Gaussian SVM | 58.3 | 81.3 |
Coarse KNN | 54.5 | 50.8 |
Layer-wise relevance propagation (LRP) is an interpretability technique for neural networks, designed to shed light on how individual input features influence the model's predictions. As an explainability method, LRP assigns relevance scores to each input feature, elucidating their significance in the neural network's decision-making process.
The fundamental concept of LRP involves redistributing the model's output relevance back to its input features in a layer-wise fashion. This process assigns relevance scores to each feature, demonstrating their effect on the final prediction. Such interpretability is vital for establishing trust and understanding in the workings of complex neural network models, particularly in domains requiring model transparency and accountability.
The relevance formula is described in Equation 1, where Ri represents the relevance of the ith neuron in the analyzed layer, Rj the relevance of the jth neuron in the subsequent layer, aij the activation between the ith and jth neurons, and
Interpreting LRP scores entails identifying each feature's impact on the model's output. Positive scores indicate a beneficial influence, negative scores a detrimental effect, and their magnitude is the strength of their contribution. A comparative analysis identifies the most impactful features, where higher positive relevance scores contribute favorably to the predictions.
Figure 3 showcases the relevance scores of features. Using the test dataset, LRP scores for each feature across samples were calculated and averaged. The bar graph in Figure 3 illustrates the average LRP scores, highlighting that while all features contribute positively, Platelet Count (501.6), ferritin (323.16), and vitamin B12 (292.01) show exceptionally high positive relevance, marking them as significantly influential on the model. The relevance scores are listed in descending order in Table 8.
Relevance scores of features.
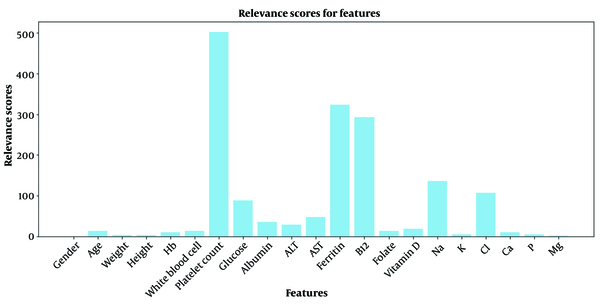
Relevance Scores of Features in Descending Order
Parameter | Value |
---|---|
Platelet count | 501.6 |
Ferritin | 323.16 |
B12 | 292.01 |
Na | 136.58 |
Cl | 107.2233 |
Glucose | 87.733 |
AST | 47.83333 |
Albumin | 34.98667 |
ALT | 29.08333 |
Vitamin D | 19.47267 |
Age | 14.06667 |
Folate | 12.921 |
White blood cell | 12.8433 |
Hb | 10.88667 |
Ca | 9.48 |
P | 4.96 |
K | 4.43 |
Weight | 2.8333 |
Height | 2.8 |
Mg | 2.106667 |
Gender | 0.5667 |
The clinical validation of our model was carried out in collaboration with patients from both Cengiz Gökçek Gynecology and Pediatrics Hospital and the Department of Pediatrics at Gaziantep University, covering the period from June 1, 2023, to January 30, 2024. The study included 27 patients diagnosed with chronic diarrhea, of whom 6 tested positive and 21 tested negative for fat malabsorption. Of these patients, 20 were selected from a repository of historical cases collected at predetermined intervals, while 7 were diagnosed in real time by our researchers. The model showcased perfect prediction accuracy, correctly determining the fat malabsorption status of all 27 patients in the cohort. This exceptional performance affirms our model's reliability as a precise diagnostic tool for pediatric patients with chronic diarrhea, highlighting its potential clinical value.
5. Discussion
5.1. Interpretation of Results
Our study's results offer strong support for the effectiveness of our innovative diagnostic approach to fat malabsorption in pediatric patients with chronic diarrhea. The high precision, recall, and F1-score values achieved by our CNN model attest to its capability to accurately identify cases of fat malabsorption versus those without. Notably, the model demonstrated a Sensitivity of 100%, successfully detecting all instances of fat malabsorption. With merely 1 false positive, the model exhibited a remarkable specificity of 95.2%, effectively minimizing incorrect diagnoses and accurately identifying cases without malabsorption. The positive predictive value (PPV) of 90% further underscores the model's dependability in making accurate positive diagnoses. Achieving 100% true positives and 95.2% true negatives, the model balanced its performance impressively.
Throughout its clinical evaluation, which included 6 positive and 21 negative cases, the model maintained flawless accuracy in predicting both fat malabsorption and its absence, achieving an astounding 100% accuracy rate. This outstanding performance underscores the model's robustness and reliability in a clinical context, marking it as an invaluable diagnostic tool for pediatric patients suffering from chronic diarrhea.
The LRP results offer detailed insights into the model's decision-making process by highlighting the significant impact of specific features, such as 'Platelet count,' 'Glucose,' and 'Ferritin,' in line with medical understanding of their connection to fat malabsorption. The high positive relevance scores of these features emphasize their vital role in the model's predictive success. Conversely, features like 'Vitamin D' and 'Mg' showed lower positive relevance, indicating a lesser impact on detecting fat malabsorption.
5.2. Comparison with Previous Studies
To the best of our knowledge, no CNN or AI model has been developed specifically for detecting fat malabsorption using a range of blood tests and features. Nonetheless, our findings are in harmony with prior research exploring deep learning and CNN applications in medical diagnostics. The efficacy of our model in distinguishing fat malabsorption cases aligns with outcomes in related domains, demonstrating the adaptability and dependability of deep learning techniques. While traditional diagnostic approaches have yielded variable outcomes, our method offers a promising alternative, enhancing both accuracy and efficiency.
5.3. Addressing Limitations
It's critical to acknowledge our study's limitations. The relatively small sample size, despite being meticulously selected, might introduce a level of variability. Moreover, our study was limited to pediatric patients from a particular clinical setting. Future studies involving larger and more varied groups will be crucial to ascertain the durability and applicability of our diagnostic method across broader contexts.
The class imbalance and the limitations inherent in the SMOTE algorithm, which was employed to mitigate this imbalance, pose additional challenges. Although SMOTE helps in creating synthetic samples to enhance the representation of the minority class, we addressed its potential downsides by judiciously adjusting parameters and ensuring a balance between original and synthetic data during model training to avoid overfitting. The susceptibility of clinical datasets to noise and outliers was countered by implementing stringent preprocessing measures before applying SMOTE. To prevent information leakage, we ensured that synthetic samples were generated solely from the training dataset. Despite these obstacles, our method resulted in a successful model for detecting fat malabsorption, evidencing accuracy in handling both synthetic and real-world clinical data.
5.4. Proposed Explanations
The remarkable efficacy of our CNN model can be credited to its capability to discern complex patterns in clinical metrics indicative of fat malabsorption. The model's progressive learning and adaptation, driven by the iterative refinement of weights via gradient descent optimization, enhance its precision over time. The optimization of hyperparameters, such as activation functions and kernel sizes, plays a significant role in the model's success.
5.5. Discussion of Implications
Our study's results have profound implications for clinical practice and research. The non-invasive nature of our diagnostic method reduces patient discomfort and streamlines the diagnosis process, potentially transforming pediatric gastroenterology by providing a more accessible and patient-friendly diagnostic option. Additionally, the cost-efficiency of our approach eases the financial strain on healthcare systems and patients, presenting it as a feasible solution for broad adoption.
In conclusion, our study marks a significant advancement in pediatric gastroenterology by utilizing deep learning, specifically a CNN, to identify fat malabsorption. Traditional diagnostic approaches often involve tedious stool sample collection and analysis, which are subject to variability and potential for human error. Our developed deep-learning model obviates the need for stool samples, presenting three key benefits. First, it facilitates rapid and early diagnosis, which is crucial for timely intervention. Second, it avoids the discomfort of collecting stool samples, addressing a significant concern for patients. Third, our model is cost-effective compared to traditional diagnostic methods. Given its practicality, swift response, and cost efficiency, it's crucial to integrate our model into clinical settings. For effective integration, the model should be incorporated within existing hospital information systems, enhancing accessibility for clinicians and allowing seamless incorporation into their daily practice.
5.6. Conclusions
5.6.1. Summarizing Main Findings
This research signifies a breakthrough in diagnosing fat malabsorption in pediatric patients suffering from chronic diarrhea. Employing deep learning techniques, we've achieved remarkable accuracy and efficiency in differentiating fat malabsorption cases from non-malabsorption cases. The performance of our CNN model underscores its potential as a significant diagnostic tool for this challenging clinical scenario.
5.6.2. Reiterating Importance
The need for accurate and prompt diagnosis of fat malabsorption in pediatric patients is paramount. This condition can lead to severe health implications, such as malnutrition and impaired growth, making early detection vital for ensuring the best possible outcomes for patients. Our study meets this critical demand by offering a non-invasive, efficient, and reliable diagnostic alternative that is also cost-effective.
5.6.3. Recommendations
Based on our findings, we advocate for the adoption of our diagnostic method in routine clinical practice within pediatric gastroenterology. Its non-invasive nature and high accuracy render it an indispensable asset for healthcare practitioners. Healthcare facilities should consider this approach to enhance diagnostic accuracy and speed in detecting fat malabsorption, thereby elevating the standard of care for affected children.
5.6.4. Future Research Directions
While our study constitutes a significant step forward, future research should aim to further validate our diagnostic method across broader and more diverse patient groups to confirm its effectiveness and applicability in various clinical contexts. Exploring the application of this methodology to other gastrointestinal disorders could also extend its utility in pediatric gastroenterology.
In summary, our study represents a pivotal development in diagnosing fat malabsorption in children with chronic diarrhea. Leveraging deep learning, we have introduced a groundbreaking diagnostic tool with substantial promise for clinical application. Integrating this method could fundamentally transform pediatric gastroenterology, ensuring timely, accurate diagnoses and ultimately enhancing the health outcomes of affected children globally.
References
-
1.
Thiagarajah JR, Kamin DS, Acra S, Goldsmith JD, Roland JT, Lencer WI, et al. Advances in Evaluation of Chronic Diarrhea in Infants. Gastroenterology. 2018;154(8):2045-2059 e6. [PubMed ID: 29654747]. [PubMed Central ID: PMC6044208]. https://doi.org/10.1053/j.gastro.2018.03.067.
-
2.
Saslow SB, Camilleri M, Thomforde GM, Van Dyke CT, Pitot HC, Rubin J. Relation between fat malabsorption and transit abnormalities in human carcinoid diarrhea. Gastroenterology. 1996;110(2):405-10. [PubMed ID: 8566586]. https://doi.org/10.1053/gast.1996.v110.pm8566586.
-
3.
Teh LB, Stopard M, Anderson S, Grant A, Quantrill D, Wilkinson RH, et al. Assessment of fat malabsorption. J Clin Pathol. 1983;36(12):1362-6. [PubMed ID: 6655068]. [PubMed Central ID: PMC498569]. https://doi.org/10.1136/jcp.36.12.1362.
-
4.
Widodo AD, Kadim M, Timan IS, Susanti NI, Alatas FS, Firmansyah A. Effectivity of microscopic test as a simple diagnostic method to detect fat malabsorption in children. Med J Indones. 2019;28(4):338-44. https://doi.org/10.13181/mji.v28i4.3640.
-
5.
Mascarenhas MR, Mondick J, Barrett JS, Wilson M, Stallings VA, Schall JI. Malabsorption blood test: Assessing fat absorption in patients with cystic fibrosis and pancreatic insufficiency. J Clin Pharmacol. 2015;55(8):854-65. [PubMed ID: 25689042]. [PubMed Central ID: PMC4496318]. https://doi.org/10.1002/jcph.484.
-
6.
Sawant N, Bansal K. An Overview of Deep Learning in Medical Imaging. SSRN Electron J. 2022;Preprint:1-27. https://doi.org/10.2139/ssrn.4031820.
-
7.
Dey A. Machine Learning Algorithms: A Review. Int J Comput Sci Inf Technol. 2016;7(3):1174-9.
-
8.
Dogo EM, Afolabi OJ, Nwulu NI, Twala B, Aigbavboa CO. A Comparative Analysis of Gradient Descent-Based Optimization Algorithms on Convolutional Neural Networks. International Conference on Computational Techniques, Electronics and Mechanical Systems (CTEMS). Belagavi, Karnataka, India. IEEE; 2018. p. 92-9.
-
9.
Jiawei Z. Gradient Descent based Optimization Algorithms for Deep Learning Models Training. arXiv. 2019;preprint(preprint).
-
10.
Van Houdt G, Mosquera C, Nápoles G. A review on the long short-term memory model. Artif Intell Rev. 2020;53(8):5929-55. https://doi.org/10.1007/s10462-020-09838-1.
-
11.
Nwankpa C, Ijomah W, Gachagan A, Marshall S. Activation functions: Comparison of trends in practice and research for deep learning. arXiv. 2018;preprint(preprint):1-20.
-
12.
Szandała T. Review and Comparison of Commonly Used Activation Functions for Deep Neural Networks. Bio-inspired Neurocomputing. Singapore: Springer; 2021. p. 203-24. https://doi.org/10.1007/978-981-15-5495-7_11.
-
13.
Hao W, Yizhou W, Yaqin L, Zhili S. The Role of Activation Function in CNN. 2nd International Conference on Information Technology and Computer Application (ITCA), 18-20 December 2020. Guangzhou, China. IEEE; 2020. p. 429-32.
-
14.
Takahashi K, Yamamoto K, Kuchiba A, Koyama T. Confidence interval for micro-averaged F (1) and macro-averaged F (1) scores. Appl Intell (Dordr). 2022;52(5):4961-72. [PubMed ID: 35317080]. [PubMed Central ID: PMC8936911]. https://doi.org/10.1007/s10489-021-02635-5.
-
15.
Powers DM. Evaluation: from precision, recall and F-measure to ROC, informedness, markedness and correlation. arXiv. 2020;preprint(preprint).
-
16.
Ling CX, Huang J, Zhang H. AUC: A Better Measure than Accuracy in Comparing Learning Algorithms.In: Xiang Y, Chaib-draa B, editors. Advances in Artificial Intelligence, 16th Conference of the Canadian Society for Computational Studies of Intelligence. Halifax, Canada. Springer, Berlin, Heidelberg; 2003. p. 329-41.
-
17.
Maldonado S, López J, Vairetti C. An alternative SMOTE oversampling strategy for high-dimensional datasets. Appl Soft Comput. 2019;76:380-9. https://doi.org/10.1016/j.asoc.2018.12.024.
-
18.
Nagi J, Ducatelle F, Di Caro GA, Ciresan D, Meier U, Giusti A, et al. Max-pooling convolutional neural networks for vision-based hand gesture recognition. International Conference on Signal and Image Processing Applications (ICSIPA). Kuala Lumpur, Malaysia. IEEE; 2011. p. 342-7.
-
19.
Nandini GS, Kumar APS, K C. Dropout technique for image classification based on extreme learning machine. Glob Transit Proc. 2021;2(1):111-6. https://doi.org/10.1016/j.gltp.2021.01.015.
-
20.
Ying X. An Overview of Overfitting and its Solutions. J Phys Conf Ser. 2019;1168. https://doi.org/10.1088/1742-6596/1168/2/022022.
-
21.
Wang J, Xu J, Wang X. Combination of hyperband and Bayesian optimization for hyperparameter optimization in deep learning. arXiv. 2018;preprint(preprint):1-10.
-
22.
Kingma DP, Ba J. Adam: A method for stochastic optimization. 3rd International Conference for Learning Representations. San Diego. arXiv; 2014.
-
23.
Zeiler MD. Adadelta: An adaptive learning rate method. arXiv. 2012;preprint(preprint).
-
24.
Ruder S. An overview of gradient descent optimization algorithms. arXiv. 2016;preprint(preprint).
-
25.
Wang Q, Ma Y, Zhao K, Tian Y. A Comprehensive Survey of Loss Functions in Machine Learning. Ann Data Sci. 2020;9(2):187-212. https://doi.org/10.1007/s40745-020-00253-5.
-
26.
Dwarampudi M, Reddy NV. Effects of padding on LSTMs and CNNs. arXiv. 2019;preprint(preprint).